Association between body mass index and glymphatic function using diffusion tensor image-along the perivascular space (DTI-ALPS) in patients with Parkinson’s disease
Introduction
The glymphatic system has been recently identified as a glial-based metabolic waste removal network (1). It facilitates fluid exchange between cerebrospinal fluid (CSF) and interstitial fluid (ISF), enabling the clearance of extracellular soluble proteins and metabolites. Many studies have suggested that impaired glymphatic flow may be implicated in various types of brain dysfunctions, such as normal pressure hydrocephalus (2), ischemic stroke (3), and Alzheimer’s disease (AD) (4,5).
Similar to AD, Parkinson’s disease (PD) is a prevalent neurodegenerative disorder that affects more than six million individuals worldwide (6). However, the underlying pathophysiology and progression of PD remain poorly understood. Recently, Zhang et al. demonstrated in animal experiments that the glymphatic system contributes to the clearance of recombinant human α-synuclein (α-syn) from the brain (7). Therefore, the glymphatic system is supposed to play an important role in the removal of α-syn in the human brain and may contribute to the progression of PD.
Obesity is commonly measured with body mass index (BMI), which is widely recognized as a primary risk factor of several neurodegenerative diseases (8). In recent years, the relationship between obesity and PD has also been gradually explored. Several studies demonstrated that higher BMI is associated with higher risk of PD, while other studies found no solid correlation between these two diseases (9-11). Weitman et al. reported that high fat diet could result in obesity and peripheral inflammation, and obesity has significant negative effects on lymphatic transport and lymph node architecture (12). In addition, obesity is associated with a pro-inflammatory state and has been linked to perivascular inflammation, oxidative stress, and mitochondrial dysfunction (13). These factors can potentially disrupt the glymphatic pathway by impairing the convective flow and CSF-to-ISF turnover, thereby potentially playing a significant role in the glymphatic dysfunction and development of PD (14). However, the effect of obesity on the glymphatic function of PD patients has not yet been established.
Magnetic resonance imaging (MRI) with intrathecal injection of gadolinium-based contrast agents can directly assess glymphatic system activity in humans (15). However, its application is limited because it is invasive and requires repeated MRI scans before and after the intrathecal injection of contrast agents. In addition, the off-label use of gadolinium-based contrast agents may result in severe neurotoxic complications. Recently, diffusion tensor image analysis along the perivascular space (DTI-ALPS) has emerged as an alternative non-invasive method for evaluating the glymphatic function in human brain (5,16). Several studies have reported the ability of the DTI-ALPS method to characterize glymphatic function in PD patients, and the lower ALPS index may reflect impairment of the glymphatic system (17-19).
The aim of this study was to investigate the association between BMI and glymphatic function in PD patients using the DTI-ALPS method. PD patients were categorized into three subgroups based on the World Health Organization criteria: normal weight PD (18.5 kg/m2 ≤ BMI <25 kg/m2), overweight PD (25 kg/m2 ≤ BMI <30 kg/m2), and obese PD (BMI ≥30 kg/m2) (20). Furthermore, the study examined the relationship between the different BMI statuses and ALPS index in PD patients. We hypothesized that higher BMI would be associated with impaired glymphatic function, as measured by ALPS indices, in PD patients. We present this article in accordance with the STROBE reporting checklist (available at https://qims.amegroups.com/article/view/10.21037/qims-23-1032/rc).
Methods
Participants
This was a retrospective, observational cross-sectional study. Participants in this study were obtained from the online Parkinson’s Progression Markers Initiative (PPMI) database (http://www.ppmi-info.org) in October 2022. The PPMI, a robust open-access data set, is a large scale ongoing observational, international, multicenter clinical study that aims to identify biological, genetic, and imaging biomarkers for the progression of PD (21,22). The inclusion and exclusion criteria adopted for this study were outlined in the PPMI database. In short, the criteria for enrollment for participants with PD at baseline were (I) aged 30 years or more; (II) scale of I or II on the Hoehn and Yahr (H&Y) scale at baseline; (III) confirmation of dopamine transporter deficit in the putamen on the DaTscan by central reading; (IV) no expectation of PD medication within six months from baseline. All PD patients were enrolled in PPMI from November 2010–April 2013. In this study, 131 sporadic PD patients, 12 PD patients with genetic mutations, and two PD patients with unknown type were analyzed. The study was conducted in accordance with the Declaration of Helsinki (as revised in 2013). More details regarding PPMI criteria are available on its website (http://www.ppmi-info.org/study-design/).
Assessment of BMI
Height and weight measurements were measured at baseline for all participants, and BMI was calculated using the formula: weight (in kilograms)/[height (in meters)]2. To investigate the association of BMI with clinical measurements, participants were categorized into three subgroups based on the BMI value according to World Health Organization criteria, i.e., normal weight (18.5 kg/m2 ≤ BMI <25 kg/m2), overweight (25 kg/m2 ≤ BMI <30 kg/m2), and obese (BMI ≥30 kg/m2) (20). Individuals with a BMI <18.5 kg/m2 were classified as underweight.
Clinical assessments
The participants were assessed with a wide spectrum of clinical tests, which included the Movement Disorders Society-sponsored Unified Parkinson’s Disease Rating Scale-Part III (MDS-UPDRS-III) for movement rating scales, and the Montreal Cognitive Assessment (MoCA) for global assessment of cognition. MDS-UPDRS-III was specifically used because it addresses PD motor symptoms for diagnosing bradykinesia, tremor, and gait and balance issues (23). All assessments were performed by movement disorders specialists following the PPMI protocol.
CSF sample measures
CSF samples were collected using standardized lumbar puncture procedures from all participants at the baseline visit of the PPMI study. Next, concentrations of α-syn, Aβ42, total tau, and p-tau were measured using appropriate commercially available sandwich-type ELISA kits (Covance, Dedham, MA, USA). The detailed method is described comprehensively on the PPMI website (http://www.ppmi-info.org/study-design/researchdocuments-and-sops/).
DTI data acquisition and preprocessing
The diffusion MRI scans used in the current study were acquired at various sites on the Siemens Tim Trio and Siemens Verio 3 Tesla MRI machines with a 12-channel Matrix head coil. All of 200 PD patients with DTI scanned with identical parameters at baseline visit were included. Details of the DTI data acquisition are available on the PPMI website (http://www.ppmi-info.org/study-design/research-documents-and-sops/). Briefly, the cardiac-triggered diffusion MRI acquisition sequence used the following parameters: diffusion gradient directions =64; repetition/echo time (TR/TE) =900/88 ms; flip angle =90°; b-values: 0, 1,000 s/mm2; the number of contiguous slices =72; slice thickness =2 mm. The individual raw MRI data in the digital imaging and communications in medicine (DICOM) file format were converted to the NIFTI file format using the MRIcron program. Subsequently, DTI data preprocessing and analysis were carried out using the FMRIB Software Library (FSL, version 6.0.5.1, http://www.fmrib.ox.ac.uk/fsl) to conduct processing steps, including correcting for head motion, eddy current distortion correction, DTIFIT, registration to the FSL-provided Montreal Neurological Institute (MNI) template.
Calculation of ALPS index
The activity of the glymphatic function was evaluated by the DTI-ALPS method, as previously described (5,19). Briefly, both fractional anisotropy (FA) and diffusivities along the direction of the x-, y-, and z-axis (Dx, Dy and Dz, respectively) maps acquired from preprocessed DTI were co-registered to the FSL provided FA map template. Four 6-mm-diameter regions of interest (ROIs) were designed in the Montreal Neurological Institute (MNI) space. The coordinates centers of ROIs were (24, −12, 24), (−28, −12, 24), (36, −12, 24) and (−40, −12, 24), respectively. Finally, manual correction was carried out to confirm the accuracy of registration and the location of ROIs for each patient. For each ROI, the diffusivities along different directions were extracted, including x-axis of projection (Dxproj), the association (Dxassoc) fibers areas, the y-axis of projection fibers (Dyproj) and the z-axis of association fibers (Dzassoc). The DTI-ALPS index was calculated as shown below: ALPS index = mean (Dxproj, Dxassoc)/mean (Dyproj, Dzassoc). The ALPS indices in both hemispheres were calculated, and the average values of left and right ALPS indices were also evaluated in the present study.
Statistical analysis
Statistical analyses were employed using IBM SPSS Statistics software (version 26, SPSS, Inc., Chicago, IL, USA). The Shapiro-Wilk test was initially used to test the normality of continuous variables and guide the selection of a parametric or nonparametric test for the comparison of variables. Normally distributed continuous variables were analyzed using one-way analysis of variance (ANOVA), followed by Bonferroni post-hoc tests when applicable. Nonparametric data were assessed using Kruskal-Wallis test. The sex and handedness data were compared using the Pearson Chi-squared test. The statistical significance levels of the report were two-sided, with the statistical significance set at 0.05. Spearman correlation analysis was used to assess relationships between the ALPS index, demographic and clinical characteristics, and CSF biomarkers, with p values corrected using the false discovery rate (FDR) approach. Partial correlation analysis was conducted to evaluate the correlations between ALPS index and BMI, while controlling for age, sex, years of education, handedness, and disease duration. To increase confidence in the interpretation of correlation, the mean correlation and its distribution of correlation coefficients was evaluated based on a large number of bootstrap iterations (n=5,000). Partial correlation analysis was also performed across all groups, which had been randomly resampled 27 data points from both the normal and overweight groups while retaining all data points from the obese group. Multivariable linear regression models were then used to calculate the independent contribution of each factor to the ALPS index. The multicollinearity of predictor variables was ruled out by assessing the variance inflation factor (VIF) values. The ALPS index was set as dependent variable. In the first model, age, sex, years of education, MDS-UPDRS-III total score, CSF Aβ42, and CSF p-tau/total tau were all entered as independent variables. In the second model, the effect of BMI was entered. The correlation heatmap and violin plot were drawn by using plotting tools in Hiplot Pro (https://hiplot.com.cn/), a comprehensive web service for biomedical data analysis and visualization. The scatter plots were created using OriginPro software (OriginPro learning edition, version 2024SP1 learning edition).
Results
Participants characteristics at baseline
A graphical overview of the participant selection is presented in Figure 1. In this study, a total of 200 PD participants with DTI scans at the baseline visit were recruited from the PPMI cohort. Regarding the importance of gating in the acquisition of the diffusion imaging, 32 subjects who had only non-gated and low-quality DTI scans were excluded. Among the remaining 168 participants, 148 met the inclusion criteria for our study, while 20 were excluded due to missing data on height, weight, CSF α-syn, Aβ42, total tau, or p-tau data. Additionally, three participants who were underweight (BMI <18.5 kg/m2) were excluded due to potentially higher likelihood of underlying health conditions which may impact the results. Therefore, a total of 145 subjects were included in the subsequent analyses.
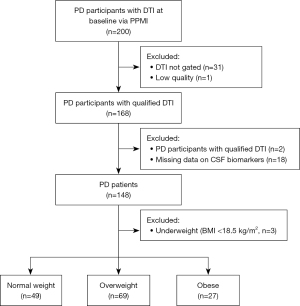
Additional demographic characteristics and clinical features at baseline are listed in Table 1. There were 145 participants with PD presented with average age of 61.40 years [standard deviation (SD) =9.12 years], with a female/male ratio of 96/49. Among these participants, individuals classified as normal weight, overweight and obese accounted for 33.79% (n=49), 47.59% (n=69), 18.62% (n=27), respectively. No significant difference was found in age, sex, years of education, disease duration, handedness, height, the scores of MoCA scores, MDS-UPDRS-III, CSF α-syn, total tau, Aβ42, and p-tau among the different BMI statuses groups. Compared with normal weight groups, overweight individuals exhibited higher levels of CSF p-tau/Aβ42 and CSF p-tau/total tau at baseline. However, there was no significant difference between overweight and obese group about the levels of CSF p-tau/Aβ42 and CSF p-tau/total tau.
Table 1
Characteristics | Total | Normal weight | Overweight | Obese | P value | |||
---|---|---|---|---|---|---|---|---|
A | B | C | D | |||||
No. of patients | 145 | 49 | 69 | 27 | ||||
Age (years) | 61.40±9.12 | 59.07±10.17 | 62.52±9.05 | 62.75±6.33 | 0.088a | – | – | – |
Sex (male/female) | 96/49 | 28/21 | 48/21 | 20/7 | 0.235b | – | – | – |
Education (years) | 15.41±2.91 | 15.59±2.68 | 15.16±3.11 | 15.70±2.85 | 0.641c | – | – | – |
Disease duration (month) | 9.31±12.89 | 7.76±9.06 | 9.41±13.65 | 11.87±16.43 | 0.511c | – | – | – |
Handedness (right/left/ambidextrous) | 127/13/5 | 43/4/2 | 59/8/2 | 25/1/1 | 0.839b | – | – | – |
Height (cm) | 174.37±8.91 | 173.49±8.62 | 174.65±8.41 | 175.22±10.72 | 0.675a | – | – | – |
Weight (kg) | 82.96±15.86 | 69.95±8.91 | 83.59±9.62 | 104.97±13.46 | <0.001a | <0.001a | <0.001a | <0.001a |
BMI (kg/m2) | 27.19±4.28 | 23.15±1.38 | 27.33±1.48 | 34.18±3.27 | <0.001c | <0.001c | <0.001c | <0.001c |
MoCA | 27.62±2.02 | 27.78±2.16 | 27.62±2.01 | 27.33±1.82 | 0.379c | – | – | – |
MDS-UPDRS-III total score | 20.70±9.63 | 19.69±9.26 | 20.94±10.39 | 21.93±8.32 | 0.508c | – | – | – |
CSF α-synuclein (pg/mL) | 1,460.48±683.91 | 1,495.48±604.32 | 1,459.86±746.11 | 1,398.53±673.83 | 0.820c | – | – | – |
CSF total tau (pg/mL) | 158.51±51.18 | 165.05±54.59 | 156.45±52.00 | 151.91±42.37 | 0.800c | – | – | – |
CSF p-tau (pg/mL) | 13.49±4.73 | 13.78±5.08 | 13.59±4.87 | 12.71±3.67 | 0.849c | – | – | – |
CSF Aβ42 (pg/mL) | 833.70±321.05 | 902.88±325.18 | 798.03±330.90 | 799.29±275.06 | 0.233c | – | – | – |
CSF Aβ42/α-synuclein | 0.610±0.186 | 0.643±0.200 | 0.591±0.193 | 0.599±0.129 | 0.305a | – | – | – |
CSF p-tau/Aβ42 | 0.018±0.007 | 0.016±0.007 | 0.019±0.008 | 0.017±0.006 | 0.048c | 0.042c | 0.980c | 0.969c |
CSF p-tau/α-synuclein | 0.010±0.002 | 0.010±0.002 | 0.010±0.003 | 0.010±0.002 | 0.661c | – | – | – |
CSF p-tau/total tau | 0.085±0.008 | 0.083±0.006 | 0.087±0.007 | 0.084±0.011 | 0.009c | 0.021c | 1.000c | 0.062c |
CSF total tau/Aβ42 | 0.206±0.079 | 0.197±0.079 | 0.213±0.083 | 0.204±0.068 | 0.137c | – | – | – |
CSF total tau/α-synuclein | 0.117±0.028 | 0.117±0.027 | 0.117±0.028 | 0.117±0.030 | 0.998c | – | – | – |
Data are expressed as number or mean ± standard deviation. a, one-way ANOVA; b, Pearson χ2 test; c, Kruskal-Wallis test. A, comparison among PD patients with normal weight, overweight and obese groups; B, normal weight vs. overweight; C, normal weight vs. obese; D, overweight vs. obese. BMI, body mass index; MoCA, Montreal Cognitive Assessment; MDS-UPDRS-III, Movement Disorders Society-sponsored Unified Parkinson’s Disease Rating Scale-Part III; CSF, cerebrospinal fluid; ANOVA, analysis of variance.
Differences between the ALPS index of the PD patients with different BMI statuses
Figure 2 shows the violin and box plots of the ALPS indices between the different BMI status groups. As there were three comparisons tested, Bonferroni corrected P value was set for 0.0167 (0.05/3), and P<0.0167 was considered significant. The results of the post hoc analysis suggested that the ALPS index for patients with obesity was statistically significantly lower than that for patients with normal weight (P<0.001). However, no significant difference in the ALPS index was observed in other groups (normal weight vs. overweight, P=0.097; overweight vs. obese, P=0.028).
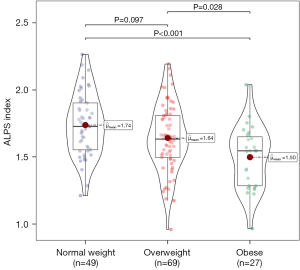
Correlation between ALPS index and demographics, BMI statuses, and CSF biomarkers
The results of correlation analysis between the ALPS index and various factors, including age, education, disease duration, BMI, MoCA score, MDS-UPDRS-III total score, and CSF biomarkers are presented in Spearman’s correlation matrix (Figure 3). The original and FDR-adjusted P values of correction matrix are showed in Table S1. The ALPS index exhibited significant negative correlations with age (−0.35, PFDR<0.01), education (−0.30, PFDR<0.01), BMI (−0.31, PFDR<0.01), CSF p-tau/Aβ42 (−0.26, PFDR=0.01), and CSF total tau/Aβ42 (−0.23, PFDR=0.02).
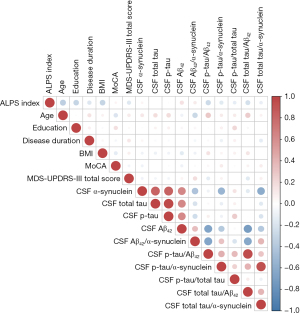
As shown in Figure 4, after correcting for covariates of age, sex, years of education, handedness, and disease duration, we found a significant negative correlation between BMI and the ALPS index among all PD participants (R=−0.275, P<0.001). However, no significant correlation was found between BMI and the ALPS index in each subgroup with different BMI statuses. The differences in subgroup participants numbers can influence the reliability of the correlation. To increase confidence in the interpretation, we randomly resampled (bootstrapping) the larger subgroups to the smallest subgroup size. Twenty-seven data points from both the normal and overweight groups were randomly resampled, while all data points from the obese group were retained. Partial correlation analysis was then conducted to evaluate the correlations between ALPS index and BMI across all groups for 5,000 iterations, ensuring that a new random sample was taken from the normal and overweight groups for each iteration. The mean correlation of all groups together was −0.2429 [95% confidence interval (CI): −0.3685, −0.1171], which did not pass through 0. Its distribution of correlation coefficients is showed in Figure S1. Our results demonstrated the presence of a robust, consistent correlation when the variation of samples were considered. In addition, among the PD patients classified as overweight, the ALPS index showed a significant negative correlation with the MDS-UPDRS-III total score (Figure 5, R=−0.318, P=0.01). There was no significant correlation found between ALPS index and the MDS-UPDRS-III total score in the overall PD group or its other subgroups, including the normal weight and obese groups.
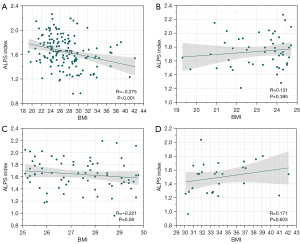
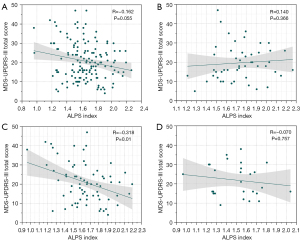
Multivariable linear regression for association with ALPS index
The results of the linear regression models for association with the ALPS index are summarized in Table 2. All independent variables had VIF values of <5, indicating the absence of multicollinearity. Model 1, which included age, sex, education, MDS-UPDRS-III total score, CSF Aβ42, and CSF p-tau/total tau, accounted for 30.1% of the variance in the ALPS index. The addition of BMI (model 2) accounted for an additional 4.5% of the variance. The multivariable linear regression analysis revealed that BMI [standardized β=−0.217; standard error (SE): 0.004, P=0.003] was a significant predictor of ALPS index, indicating that higher BMI was associated with a lower ALPS index.
Table 2
Variable | Model 1 | Model 2 | |||||||
---|---|---|---|---|---|---|---|---|---|
B | SE (B) | β | P | B | SE (B) | β | P | ||
Age (years) | −0.008 | 0.002 | −0.297 | <0.001*** | −0.008 | 0.002 | −0.291 | <0.001*** | |
Sex (male/female) | −0.083 | 0.039 | −0.156 | 0.033* | −0.068 | 0.038 | −0.127 | 0.076 | |
Education | −0.022 | 0.006 | −0.254 | 0.001** | −0.023 | 0.006 | −0.269 | <0.001*** | |
MDS-UPDRS-III total score | −0.004 | 0.002 | −0.148 | 0.042* | −0.003 | 0.002 | −0.132 | 0.062 | |
CSF Aβ42 | <0.001 | 0.000 | 0.157 | 0.032* | <0.001 | <0.001 | 0.135 | 0.058 | |
CSF p-tau/total tau | −3.301 | 2.359 | −0.103 | 0.164 | −2.755 | 2.297 | −0.086 | 0.232 | |
BMI (kg/m2) | – | – | – | – | −0.013 | 0.004 | −0.217 | 0.003** | |
R2 | – | 0.301 | – | – | – | 0.346 | – | – | |
Model F | – | 9.922*** | – | – | – | 10.370*** | – | – |
Significant difference defined as *, P<0.05; **, P<0.01; ***, P<0.001. ALPS, analysis along the perivascular space; PD, Parkinson’s disease; B, unstandardized coefficient; SE, standard error; β, standardized coefficient; MDS-UPDRS-III, Movement Disorders Society-sponsored Unified Parkinson’s Disease Rating Scale-Part III; CSF, cerebrospinal fluid; BMI, body mass index.
Discussion
Using DTI imaging data from 145 PD patients from the PPMI study, we investigated the association between BMI and ALPS index, as well as the relationship among ALPS index, CSF biomarkers, cognitive outcome, and motor outcome in PD patients with different BMI statuses. Our findings revealed a significant difference between the normal weight and obese groups for the ALPS index. With age, sex, education, handedness, and disease duration as the covariate, the partial correlation analysis revealed a significant negative correlation between the ALPS index and BMI for all PD patients. In addition, a negative correlation between ALPS index and the severity of motor symptoms measured by MDS-UPDRS-III total score was observed in the overweight group. Furthermore, higher levels of toxic CSF proteins (CSF p-tau/Aβ42 and CSF p-tau/total tau) was found in the overweight group, comparing with normal weight and obese groups. Finally, the multivariate linear regression results related to BMI should be interpreted as relatively directly related to ALPS index.
The glymphatic system, a recently discovered transport system in the brain, plays a crucial role in maintaining brain homeostasis by eliminating proteins and toxic metabolites (1). The classical neuropathologic characteristic of PD involves the excessive accumulation of α-syn and dopaminergic neuron degeneration, resulting in a variety of clinical symptoms such as bradykinesia, tremor, rigidity, and postural instability (24). Recently, Zou et al. reported that glymphatic influx of CSF tracer was reduced in a transgenic mouse model of PD, and blocking meningeal lymphatic drainage could aggravate PD-like pathology (25). Subsequently, Ding et al. found that patients with idiopathic PD exhibited significantly meningeal lymphatic dysfunction and reduced flow via dynamic contrast-enhanced MRI (26). DTI-ALPS has been increasingly used to non-invasively assess the brain’s glymphatic system, which has since been employed in the study of several conditions, including AD (5), migraine (27), and type 2 diabetes (28). The ALPS index has been proposed as an indirect indicator of the glymphatic system, and reduced ALPS index indicates less efficient fluid transport in the directionally perivascular pathway and compromised glymphatic clearance. Shen et al. reported that glymphatic system dysfunction is involved in the pathophysiology of PD, and ALPS index may be a promising biomarker of the glymphatic system activity in PD (14).
Exploring the adverse effects of risk factors on the glymphatic system in PD patients can significantly contribute to improving preventive and therapeutic interventions to target specific behavioral changes. Previous studies have identified several risk factors associated with the decreased glymphatic activity that is reflected by reduced ALPS index in PD individuals, such as ageing (17,29), oxidative stress (30), and sleep disorders (19). Overweight or obese, mainly due to a less healthy diet and lifestyle, is an increasingly serious and prevalent health issue both in developed and developing countries. It is a major risk factor for many diseases including cardiovascular disease, type 2 diabetes, AD, and several types of cancers (31). BMI is an easy and inexpensive screening method to assess obesity using an individual’s weight and height. Prior reports suggest that higher BMI, waist-to-hip ratio, and waist circumference are associated with reduced cerebral blood flow in older adults (age 69.0±7.4 years) (32). In recent years, several prospective studies have assessed the association between BMI and the risk of PD, but the results have been inconsistent. Some studies found that no association between BMI and risk of PD, while some suggested a trend towards increased risk in patients with high BMI, and some suggested a reduced risk of PD associated with lower BMI (9-11,33). The underlying mechanism linking obesity to PD risk remains unclear, and the relationship between BMI and glymphatic system activity in PD has been rarely investigated. Our results suggest that BMI is an important clinical biomarker of glymphatic activity in PD, and higher BMI may contribute to glymphatic system dysfunction in PD patients. In addition, we also showed that there is a significant negative correlation between age and ALPS index, which is in accordance with previous studies (17). Theoretically, the association between obesity and glymphatic system dysfunction in PD patients may be partially explained by the effect of increased systemic oxidative stress. Yu et al. showed that obese animals had higher expression of proinflammatory cytokines including interleukin (IL)-1β and IL-6 in brain structures, indicating the presence of a proinflammatory response (34). Furthermore, in animal models combining obesity and PD, chronic inflammation and oxidative damage were observed, resulting in DNA damage in both brain and peripheral structures (35). As a result, increased oxidative stress may lead to malfunctioning of the glymphatic system by decreasing the convective flow, and CSF-to-ISF turnover, resulting in impaired waste clearance.
The present study has several limitations that need to be considered. Firstly, our study was cross-sectional, which had a small sample size with an unequal number of PD participants of different BMI statuses, which might have limited the statistical power. Identifying PD participants with obesity proved to be challenging. Longitudinal studies of larger population samples with a balanced proportion are needed in the future to further explore the temporal effect of BMI on glymphatic system function in the progression of PD. Secondly, although it is very convenient for measuring the degree of obesity using BMI, it does not take into account the importance and heterogeneity of body fat distribution to evaluate the risk of metabolic alterations at the individual level (36). Waist circumference is a better marker of metabolic alterations and is associated with obesity-related comorbidity (37). Further studies should incorporate alternative obesity-related measurements to gain a better understanding of ALPS index in relation to obesity. Thirdly, control subjects were not included as a comparator group in this study, because we focused on the association between BMI and glymphatic function in PD patients, and the study’s design did not originally intend to include control subjects. Recently, Bae et al. reported that the glymphatic alteration in PD could be effectively measured by ALPS index, which demonstrated reduced glymphatic function in PD compared to the controls (38). Fourthly, as the image analysis process includes ROI mapping, it is important to acknowledge that the ROI mapping was performed by a single radiologist. Ideally, involving multiple radiologists to independently perform ROI mapping could enhance the robustness of our results and reduce potential bias. Additionally, high resolution imaging protocols such as susceptibility-weighted imaging (SWI) are need to precisely overlay with DTI to measure diffusion values in the correct regions in future study. Fifthly, it is noted that DTI-ALPS provides only an approximate measure of glymphatic flow, which could indirectly represent the transport function of the glymphatic system. Its validity as a proxy measure of CSF-ISF exchange has not been definitively established. Some assumptions, such as complete suppression of blood signals at a b-value of 1,000 s/mm2, could lead the DTI-ALPS index to represent factors other than fluid flow, such as tissue motion, slow blood flow, or white matter changes, which could all be affected by main effect of PD, BMI and their interactions. Further comparisons to gold standard techniques such as CSF tracer studies will be needed to determine the robustness of DTI-ALPS for assessing glymphatic dysfunction. Finally, the underlying mechanisms linking BMI, ALPS index, and CSF biomarkers should be explored in prospective studies involving large cohorts in the future.
Conclusions
Using the noninvasive DTIALPS method to measure glymphatic activity, our study demonstrates a negative association between higher BMI and decreased ALPS indices, suggesting high BMI may contribute to impaired glymphatic function in PD patients. In addition, with age, sex, education, handedness, and disease duration as the covariate, subgroup analysis revealed a negative correlation between ALPS index and the severity of motor symptoms measured by MDS-UPDRS-III in the overweight PD group, rather than in the normal weight and obese groups. Future studies should aim to investigate the longitudinal associations between BMI and glymphatic activity in PD patients, as well as some factors that can affect BMI, such as dopamine dysregulation, medication and diet over the course of PD.
Acknowledgments
We thank Shanghai Tengyun Biotechnology Co., Ltd. for developing Hiplot Pro platform (https://hiplot.com.cn/) and providing technical assistance and valuable tools for data analysis and visualization.
Funding: This research was supported by
Footnote
Reporting Checklist: The authors have completed the STROBE reporting checklist. Available at https://qims.amegroups.com/article/view/10.21037/qims-23-1032/rc
Conflicts of Interest: All authors have completed the ICMJE uniform disclosure form (available at https://qims.amegroups.com/article/view/10.21037/qims-23-1032/coif). The authors have no conflicts of interest to declare.
Ethical Statement: The authors are accountable for all aspects of the work in ensuring that questions related to the accuracy or integrity of any part of the work are appropriately investigated and resolved. The study was conducted in accordance with the Declaration of Helsinki (as revised in 2013).
Open Access Statement: This is an Open Access article distributed in accordance with the Creative Commons Attribution-NonCommercial-NoDerivs 4.0 International License (CC BY-NC-ND 4.0), which permits the non-commercial replication and distribution of the article with the strict proviso that no changes or edits are made and the original work is properly cited (including links to both the formal publication through the relevant DOI and the license). See: https://creativecommons.org/licenses/by-nc-nd/4.0/.
References
- Mestre H, Mori Y, Nedergaard M. The Brain's Glymphatic System: Current Controversies. Trends Neurosci 2020;43:458-66. [Crossref] [PubMed]
- Bae YJ, Choi BS, Kim JM, Choi JH, Cho SJ, Kim JH. Altered glymphatic system in idiopathic normal pressure hydrocephalus. Parkinsonism Relat Disord 2021;82:56-60. [Crossref] [PubMed]
- Toh CH, Siow TY. Glymphatic Dysfunction in Patients With Ischemic Stroke. Front Aging Neurosci 2021;13:756249. [Crossref] [PubMed]
- Ota M, Sato N, Nakaya M, Shigemoto Y, Kimura Y, Chiba E, Yokoi Y, Tsukamoto T, Matsuda H. Relationships Between the Deposition of Amyloid-β and Tau Protein and Glymphatic System Activity in Alzheimer's Disease: Diffusion Tensor Image Study. J Alzheimers Dis 2022;90:295-303. [Crossref] [PubMed]
- Taoka T, Masutani Y, Kawai H, Nakane T, Matsuoka K, Yasuno F, Kishimoto T, Naganawa S. Evaluation of glymphatic system activity with the diffusion MR technique: diffusion tensor image analysis along the perivascular space (DTI-ALPS) in Alzheimer's disease cases. Jpn J Radiol 2017;35:172-8. [Crossref] [PubMed]
- Armstrong MJ, Okun MS. Diagnosis and Treatment of Parkinson Disease: A Review. JAMA 2020;323:548-60. [Crossref] [PubMed]
- Zhang Y, Zhang C, He XZ, Li ZH, Meng JC, Mao RT, Li X, Xue R, Gui Q, Zhang GX, Wang LH. Interaction Between the Glymphatic System and α-Synuclein in Parkinson's Disease. Mol Neurobiol 2023;60:2209-22. [Crossref] [PubMed]
- Mazon JN, de Mello AH, Ferreira GK, Rezin GT. The impact of obesity on neurodegenerative diseases. Life Sci 2017;182:22-8. [Crossref] [PubMed]
- Logroscino G, Sesso HD, Paffenbarger RS Jr, Lee IM. Body mass index and risk of Parkinson's disease: a prospective cohort study. Am J Epidemiol 2007;166:1186-90. [Crossref] [PubMed]
- Chen H, Zhang SM, Schwarzschild MA, Hernán MA, Willett WC, Ascherio A. Obesity and the risk of Parkinson's disease. Am J Epidemiol 2004;159:547-55. [Crossref] [PubMed]
- Hu G, Jousilahti P, Nissinen A, Antikainen R, Kivipelto M, Tuomilehto J. Body mass index and the risk of Parkinson disease. Neurology 2006;67:1955-9. [Crossref] [PubMed]
- Weitman ES, Aschen SZ, Farias-Eisner G, Albano N, Cuzzone DA, Ghanta S, Zampell JC, Thorek D, Mehrara BJ. Obesity impairs lymphatic fluid transport and dendritic cell migration to lymph nodes. PLoS One 2013;8:e70703. [Crossref] [PubMed]
- de Heredia FP, Gómez-Martínez S, Marcos A. Obesity, inflammation and the immune system. Proc Nutr Soc 2012;71:332-8. [Crossref] [PubMed]
- Shen T, Yue Y, Ba F, He T, Tang X, Hu X, Pu J, Huang C, Lv W, Zhang B, Lai HY. Diffusion along perivascular spaces as marker for impairment of glymphatic system in Parkinson's disease. NPJ Parkinsons Dis 2022;8:174. [Crossref] [PubMed]
- Taoka T, Naganawa S. Glymphatic imaging using MRI. J Magn Reson Imaging 2020;51:11-24. [Crossref] [PubMed]
- Taoka T, Ito R, Nakamichi R, Kamagata K, Sakai M, Kawai H, Nakane T, Abe T, Ichikawa K, Kikuta J, Aoki S, Naganawa S. Reproducibility of diffusion tensor image analysis along the perivascular space (DTI-ALPS) for evaluating interstitial fluid diffusivity and glymphatic function: CHanges in Alps index on Multiple conditiON acquIsition eXperiment (CHAMONIX) study. Jpn J Radiol 2022;40:147-58. [Crossref] [PubMed]
- Cai X, Chen Z, He C, Zhang P, Nie K, Qiu Y, Wang L, Wang L, Jing P, Zhang Y. Diffusion along perivascular spaces provides evidence interlinking compromised glymphatic function with aging in Parkinson's disease. CNS Neurosci Ther 2023;29:111-21. [Crossref] [PubMed]
- Ma X, Li S, Li C, Wang R, Chen M, Chen H, Su W. Diffusion Tensor Imaging Along the Perivascular Space Index in Different Stages of Parkinson's Disease. Front Aging Neurosci 2021;13:773951. [Crossref] [PubMed]
- Si X, Guo T, Wang Z, Fang Y, Gu L, Cao L, Yang W, Gao T, Song Z, Tian J, Yin X, Guan X, Zhou C, Wu J, Bai X, Liu X, Zhao G, Zhang M, Pu J, Zhang B. Neuroimaging evidence of glymphatic system dysfunction in possible REM sleep behavior disorder and Parkinson's disease. NPJ Parkinsons Dis 2022;8:54. [Crossref] [PubMed]
- Obesity: preventing and managing the global epidemic. Report of a WHO consultation. World Health Organ Tech Rep Ser 2000;894:i-253.
- The Parkinson Progression Marker Initiative (PPMI). Prog Neurobiol 2011;95:629-35. [Crossref] [PubMed]
- Marek K, Chowdhury S, Siderowf A, Lasch S, Coffey CS, Caspell-Garcia C, et al. The Parkinson's progression markers initiative (PPMI) - establishing a PD biomarker cohort. Ann Clin Transl Neurol 2018;5:1460-77.
- Vassar SD, Bordelon YM, Hays RD, Diaz N, Rausch R, Mao C, Vickrey BG. Confirmatory factor analysis of the motor unified Parkinson's disease rating scale. Parkinsons Dis 2012;2012:719167. [Crossref] [PubMed]
- Khan AU, Akram M, Daniyal M, Zainab R. Awareness and current knowledge of Parkinson's disease: a neurodegenerative disorder. Int J Neurosci 2019;129:55-93. [Crossref] [PubMed]
- Zou W, Pu T, Feng W, Lu M, Zheng Y, Du R, Xiao M, Hu G. Blocking meningeal lymphatic drainage aggravates Parkinson's disease-like pathology in mice overexpressing mutated α-synuclein. Transl Neurodegener 2019;8:7. [Crossref] [PubMed]
- Ding XB, Wang XX, Xia DH, Liu H, Tian HY, Fu Y, Chen YK, Qin C, Wang JQ, Xiang Z, Zhang ZX, Cao QC, Wang W, Li JY, Wu E, Tang BS, Ma MM, Teng JF, Wang XJ. Impaired meningeal lymphatic drainage in patients with idiopathic Parkinson's disease. Nat Med 2021;27:411-8. [Crossref] [PubMed]
- Lee DA, Lee HJ, Park KM. Normal glymphatic system function in patients with migraine: A pilot study. Headache 2022;62:718-25. [Crossref] [PubMed]
- Yang G, Deng N, Liu Y, Gu Y, Yao X. Evaluation of Glymphatic System Using Diffusion MR Technique in T2DM Cases. Front Hum Neurosci 2020;14:300. [Crossref] [PubMed]
- McKnight CD, Trujillo P, Lopez AM, Petersen K, Considine C, Lin YC, Yan Y, Kang H, Donahue MJ, Claassen DO. Diffusion along perivascular spaces reveals evidence supportive of glymphatic function impairment in Parkinson disease. Parkinsonism Relat Disord 2021;89:98-104. [Crossref] [PubMed]
- Chen HL, Chen PC, Lu CH, Tsai NW, Yu CC, Chou KH, Lai YR, Taoka T, Lin WC. Associations among Cognitive Functions, Plasma DNA, and Diffusion Tensor Image along the Perivascular Space (DTI-ALPS) in Patients with Parkinson's Disease. Oxid Med Cell Longev 2021;2021:4034509. [Crossref] [PubMed]
- Lin X, Li H. Obesity: Epidemiology, Pathophysiology, and Therapeutics. Front Endocrinol (Lausanne) 2021;12:706978. [Crossref] [PubMed]
- Knight SP, Laird E, Williamson W, O'Connor J, Newman L, Carey D, De Looze C, Fagan AJ, Chappell MA, Meaney JF, Kenny RA. Obesity is associated with reduced cerebral blood flow - modified by physical activity. Neurobiol Aging 2021;105:35-47. [Crossref] [PubMed]
- Noyce AJ, Kia DA, Hemani G, Nicolas A, Price TR, De Pablo-Fernandez E, Haycock PC, Lewis PA, Foltynie T, Davey Smith G, Schrag A, Lees AJ, Hardy J, Singleton A, Nalls MA, Pearce N, Lawlor DA, Wood NW. Estimating the causal influence of body mass index on risk of Parkinson disease: A Mendelian randomisation study. PLoS Med 2017;14:e1002314. [Crossref] [PubMed]
- Yu R, Kim CS, Kwon BS, Kawada T. Mesenteric adipose tissue-derived monocyte chemoattractant protein-1 plays a crucial role in adipose tissue macrophage migration and activation in obese mice. Obesity (Silver Spring) 2006;14:1353-62. [Crossref] [PubMed]
- Cavalheiro EKFF, da Silva LE, Oliveira MP, Silva MG, Damiani AP, Ribeiro CB, Magenis ML, Cucker L, Michels M, Joaquim L, Machado RS, Vilela TC, Bitencourt RM, Andrade VM, Dal-Pizzol F, Petronilho F, Tuon T, Rezin GT. Effects of obesity on neuroinflammatory and neurochemical parameters in an animal model of reserpine-induced Parkinson's disease. Behav Brain Res 2022;434:114019. [Crossref] [PubMed]
- Tchernof A, Després JP. Pathophysiology of human visceral obesity: an update. Physiol Rev 2013;93:359-404. [Crossref] [PubMed]
- Janssen I, Katzmarzyk PT, Ross R. Waist circumference and not body mass index explains obesity-related health risk. Am J Clin Nutr 2004;79:379-84.
- Bae YJ, Kim JM, Choi BS, Choi JH, Ryoo N, Song YS, Cho SJ, Kim JH. Glymphatic function assessment in Parkinson's disease using diffusion tensor image analysis along the perivascular space. Parkinsonism Relat Disord 2023;114:105767. [Crossref] [PubMed]