Natural language processing-based analysis of the level of adoption by expert radiologists of the ASSR, ASNR and NASS version 2.0 of lumbar disc nomenclature: an eight-year survey
Introduction
In 2014, the combined task forces of the American Society of Spine Radiology (ASSR), American Society of Neuroradiology (ASNR), and North American Spine Society (NASS) published a consensus paper summarizing their current recommendations for lumbar disc nomenclature reports (1). This statement is an update of a previous one, published by the same task force in 2001, for promoting consistent, robust, and precise terminology for lumbar disc nomenclature (2).
However, the adoption of this suggested terminology and incorporation of this new lexicon into radiology reports requires an active commitment from both radiologists and radiology departments. In this scenario, there are some ways to evaluate the grade of adoption of this new terminology by radiologists and radiology departments (3,4). Questioning or screening radiologists about their commitment grade with the new lexicon may be insufficient and inaccurate, attached to different biases (forgetfulness, lack of objectivity…) (5). Word-based search engines may help in this task; however, these kinds of close-word approaches are limited since they do not consider the context of sentences, such as negation or data outside the core of radiology reports, such as clinical information (6). Besides, the large number of radiological reports, the massive amount of information available in radiology reports, the type of applied format (structured vs. unstructured) or the variability between the way radiology reports are performed by the same or different radiologists make this task almost impossible for human beings (7).
Artificial intelligence (AI) algorithms can help in the challenging task of reviewing radiology reports and detecting changes in the way magnetic resonance imaging (MRI) lumbar spine reports are performed. Natural language processing (NLP), has widely demonstrated its analyzing and evaluating radiological reports, helping to extract additional information from them, tasks otherwise not possible by conventional word-based search engine approaches (8-10).
The purpose of this study is to assess the degree of adherence in our radiology department to the NASS, ASSR and ASNR version 2.0 recommendations on lumbar disc nomenclature by using an NLP approach after the publication of the consensus paper by the ASSR, ASNR and NASS. We hypothesized that NLP may help to find relevant differences between the terminology used before and after the consensus paper publication for each radiologist evaluated. We present this article in accordance with the TRIPOD reporting checklist (available at https://qims.amegroups.com/article/view/10.21037/qims-23-1294/rc).
Methods
Study design
The study was conducted in accordance with the Declaration of Helsinki (as revised in 2013). The study was approved by our local Ethics Committee and individual consent for this retrospective analysis was waived by the local Ethics Committee in view of the retrospective nature based on anonymized radiology reports. A retrospective analysis of lumbar spine MRI radiological reports was carried out. These reports were performed and analyzed in the Spanish language. However, for better comprehension and readability of this paper, all the Spanish terms used are followed by their translation to English between parentheses.
As the consensus paper was published in November 2014 and an internal clinical lecture in our radiology department at HT Medica in Jaén (Spain) explaining this paper was conducted in March 2015 in our radiology department. For this reason, we analyzed the reports retrospectively in two groups: reports between May 2010 and February 2015 and reports from March 2015 to February 2022.
Reports were selected from three different radiologists: two experts in neuroradiology and one in musculoskeletal radiology (in our radiology department, both groups report spine imaging), that have been continuously reporting spine MRI studies in our radiology department since May 2010 to date, also referred to as radiologist A (20 years of experience), radiologist B (12 years of experience) and radiologist C (12 years of experience) for the present study. For the NLP analysis, the non-structured findings and conclusion sections of each radiology report were selected.
Selected terms related to MRI lumbar spine nomenclature were divided into four main categories: disc with fissures of the annulus, degenerated disc, herniated disc and disc location following the ASSR, ASNR and NASS consensus paper structure. Old terms are defined by the non-recommendable or non-specific lexicon detailed in the consensus paper. New terms are defined by the lexicon recommended by the consensus papers. Table 1 summarizes the selected terms to be analyzed in both groups of reports. Given the large number of MRI lumbar spine reports selected (more than 34,000) and the number of terms to search for (29 terms) within these reports, we opted for NLP extraction approach and rule-based methodology for evaluating the degree of adherence of radiologists to ASSR, ASNR and NASS recommendations instead of using other NLP techniques.
Table 1
Category | Terms | Descriptions |
---|---|---|
1. Disc with fissures of the annulus | Old | Annular tear |
New | Annular fissure | |
2. Degenerated disc | Old | Spondylosis deformans |
Intervertebral osteochondrosis | ||
Dehydration | ||
Loss of height | ||
New | Degenerated disc | |
Degenerative disc changes | ||
3. Herniated disc | Old | Herniated nucleous pulposus (HNP) |
Ruptured disc | ||
Protruded disc | ||
Prolapsed disc | ||
Bulging disc | ||
New | Protrusion | |
Extrusion | ||
Bulging | ||
4. Disc location | Old | Right paracentral |
Right posterolateral | ||
Left paracentral | ||
Left posterolateral | ||
New | Central | |
Subarticular | ||
Foraminal | ||
Right central | ||
Right subarticular | ||
Right foraminal | ||
Left central | ||
Left subarticular | ||
Left foraminal |
Data pre-processing and algorithm development
A processing pipeline, which uses the terminology as a knowledge source for automatic annotation terms in radiology reports, was applied (Figure 1). Since radiology reports were highly unstructured and contained much noise data, NLP techniques were utilized to clean the text (e.g., for misspelling correction). Each step of this NLP pipeline is detailed below:
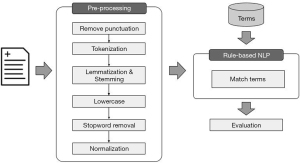
Remove punctuation
Our first step in the NLP pipeline has been to remove all punctuation marks to standardize terms such as “central-derecha” (right-central) to “central derecha” (right central).
Tokenization
Tokenization is the process of breaking a stream of textual data into words, terms, sentences, symbols, or some other meaningful elements called tokens (11). A tokenizer breaks unstructured data and natural language text into chunks of information that can be considered discrete elements. For this purpose, we use the FreeLing library that incorporates analysis functionalities for various languages, including Spanish (12). Tokens selection was decided based on the old and new terms detailed at the ASSR, ASNR and NASS consensus paper and summarized in Table 1.
Lemmatization & stemming
NLP uses lemmatization and stemming to transform inflected forms of a single word back to their root form. For instance, “discos protruidos” (protruded discs), will be reduced to “disco protruido” (protruded disc) and the root form of “protrusion” is protrusion. As in the previous step, we use the NLP Freeling tool that provides the root words for Spanish (13).
Lower case
Lowering the text is one of the most common pre-processing steps where the text is converted into the same case, preferably lowercase.
Stop word removal
Removing stop words is an essential step in NLP text processing. It involves filtering out high-frequency words that add little or no semantic value to a sentence, for example, a (an), con (with), de (of/from), el (the)... For example, sometimes, instead of finding “pérdida de altura” (loss of height), the term “pérdida altura” (height loss) is found without “de” (of) and this process eliminates this terminology variability. The list of Spanish stop-word was extracted from the NLTK package and can be found at https://github.com/nltk/nltk_data/blob/gh-pages/packages/corpora/stopwords.zip (14).
Normalization
The last step in pre-processing and text cleaning is text normalization. This requires actions such as removing accents that can be found in Spanish, removing line breaks, and converting several blank spaces into one.
Match terms
A rule-based term extraction algorithm was implemented to identify terms through the pre-processing steps described above. The proposed algorithm is based on a comparison between terminology and pre-processed texts of radiological reports. The terminology contains, including old and new ones, 29 terms. In NLP, matching techniques aim to compare two sentences to determine whether they have a similar meaning.
Standard reference
A separate group of reports was included as a validation set to generate a gold standard to evaluate the NLP tool. Six hundred lumbar spine MRI reports from the three radiologists evaluated (200 from each one), were reviewed by a radiologist with 14 years of experience. Table S1 shows the distribution of labels in the validation set.
Statistical analysis
Standard metrics were used to evaluate the performance of the NLP algorithm for terminology extraction such as recall, precision and F1-measure considering the number of true positives (TPs), false positives (FPs) and false negatives (FNs). All analyses were performed in Python 3.8.
In the labeled corpus of 600 reports, to assess the evolution in the use of both, the old and the new terms, for the four categories by each radiologist between the periods considered, a statistical analysis was performed using SciPy version 1.5.4. Since the number of occurrences of the terms in the report does not follow a normal distribution, the Mann-Whitney U test was used. The level of significance was set at 0.05.
Results
NLP analysis
In total, 34,064 lumbar spine MRI reports were included (no report was excluded from the analysis) in the study from the radiologists selected from May 2010 to February 2022, separated into two main groups. The first group of lumbar spine MRI reports from May 2010 to February 2015 (15,813 studies), and the second group of lumbar spine MRI reports from March 2015 to February 2022 (18,251 studies). Table S2 shows percentages and number of reports for each radiologist from each period. Each radiological report had a mean number of 176 words with a standard deviation of 88 words. In a brief analysis, we found that 2% of the reports did not contain pathologies, as the conclusion of the study was “study without findings of interest”, “no significant alterations”, “study within normality”, and so on.
Validation set
Regarding the 600 MRI lumbar spine radiology reports labeled by a radiology expert, a total of 4,606 terms (both old and new) were included in the set. The minimum number of lexical terms found in the reports is 1, the maximum is 21, the mean is 7 terms, and the standard deviation is 4. This dataset of 600 reports constituted the gold standard for our evaluation of the algorithm.
NLP algorithm performance
The evaluation step counts the number of terms annealed by the NLP algorithm against the gold standard validation set. 4094 TP, 243 FP and 269 FN were reported, resulting in a precision of 94.4%, recall of 93.8% and finally an F1-score of 94.1%.
Terms such as “degenerative phenomena”, “spondylarthrosis”, “left foraminal” and “right foraminal” are the most problematic for recognition by the NLP (FN) algorithm. After analyzing these FNs, we have concluded that the system is vulnerable to radiologists’ misspellings and often forgets to label bigrams. For example, “left foraminal” is labeled as “foraminal”, forgetting to include laterality.
Regarding the FPs, the algorithm erroneously recognized terms such as “central” and “height loss”. Often the system fails in the detection of “central” because it frequently recognizes “central canal” in radiology reports which is related to spinal canal stenosis, a category not evaluated for the purpose of this study. Regarding the term “height loss” it usually appears in the reports referring to vertebrae fracture or collapse instead of disc degeneration.
NLP results for MRI lumbar spine reports evaluated
Results were obtained for two different periods (May 2010–February 2015 and March 2015–February 2022) and for both old and new terminology proposed by the ASSR, ASNR and NASS v2.0 consensus statement. The percentage and distribution of each term related to each main category (disc with fissures of the annulus /fissure, degenerated disc, herniated disc and disc location) are available in Table S3.
The percentage of decrement and increment of old and new terms used ranges from a decrement of 44.63% (old terms related to degenerated disc category for radiologist B) to an increment of 40.64% (old term related to degenerated disc category for radiologist A (Table 2). The most relevant increments in the percentage of use of new terms proposed by the ASSR, ASNR and NASS (Table 3) for radiologist A were terms related to herniated disc (7.27%), and degenerated disc (32.48%), and disc localization (36.53%) for radiologist C. The most relevant decreases in the percentage of use of old terms were for degenerated disc category (44.63% for radiologist B) and disc location (18.86% for radiologist A) and disc location and degenerated disc (27.73% and 18.95% respectively for radiologist C) (Figure 2).
Table 2
Radiologist | Category† | May 2010–Feb 2015 | Mar 2015–Feb 2022 | Increase/decrease in use, %‡ | |||||||
---|---|---|---|---|---|---|---|---|---|---|---|
No. of reports | No. of reports with terms | Frequency of terms in reports | % of use | No. of reports | No. of reports with terms | Frequency of terms in reports | % of use | ||||
A | 1 | 8,519 | 0 | 0 | 0 | 5,381 | 0 | 0 | 0 | 0 | |
2 | 1,306 | 1,404 | 15.33 | 3,012 | 3,375 | 55.97 | 40.64 | ||||
3 | 20 | 20 | 0.23 | 6 | 6 | 0.11 | −0.12 | ||||
4 | 1,656 | 3,389 | 19.44 | 31 | 52 | 0.58 | −18.86 | ||||
B | 1 | 2,948 | 0 | 0 | 0 | 10,342 | 0 | 0 | 0 | 0 | |
2 | 1,677 | 4,118 | 56.89 | 1,266 | 2,118 | 12.26 | −44.63 | ||||
3 | 88 | 120 | 2.99 | 22 | 31 | 0.21 | −2.78 | ||||
4 | 500 | 998 | 16.96 | 619 | 1,016 | 5.99 | −10.97 | ||||
C | 1 | 4,229 | 1 | 1 | 0.02 | 2,398 | 0 | 0 | 0 | −0.02 | |
2 | 3,119 | 4,512 | 73.75 | 1,312 | 1,832 | 54.8 | −18.95 | ||||
3 | 7 | 8 | 0.17 | 6 | 6 | 0.25 | −0.08 | ||||
4 | 1,201 | 1,704 | 28.4 | 16 | 19 | 0.67 | −27.73 |
†, 1: disc with fissures of the annulus; 2: degenerated disc; 3: herniated disc; 4: location of the disc. ‡, negative values mean the percentage decreased in the use of old terms; positive values mean percentage increased in the use of new terms.
Table 3
Radiologist | Category† | May 2010–Feb 2015 | Mar 2015–Feb 2022 | Increase/decrease in use‡ | |||||||
---|---|---|---|---|---|---|---|---|---|---|---|
No. of reports | No. of reports with terms | Frequency of terms in reports | % of use | No. of reports | No. of reports with terms | Frequency of terms in reports | % of use | ||||
A | 1 | 8,519 | 1 | 1 | 0.01 | 5,381 | 0 | 0 | 0.00 | −0.01 | |
2 | 764 | 835 | 8.97 | 25 | 27 | 0.46 | −8.51 | ||||
3 | 7,636 | 19,461 | 89.63 | 5,214 | 17,794 | 96.9 | 7.27 | ||||
4 | 7,569 | 22,886 | 88.85 | 4,824 | 23,981 | 89.65 | 0.8 | ||||
B | 1 | 2,948 | 0 | 0 | 0.00 | 10,342 | 2 | 2 | 0.02 | 0.02 | |
2 | 68 | 86 | 2.31 | 44 | 55 | 0.43 | −1.88 | ||||
3 | 2,339 | 6,110 | 79.34 | 10,272 | 44,851 | 67.65 | −11.69 | ||||
4 | 816 | 3,202 | 27.68 | 6,996 | 27,072 | 14.21 | −13.47 | ||||
C | 1 | 4,229 | 175 | 195 | 4.14 | 2,398 | 205 | 221 | 8.55 | 4.41 | |
2 | 196 | 222 | 4.63 | 890 | 1,093 | 37.11 | 32.48 | ||||
3 | 4,044 | 11,550 | 95.63 | 2,308 | 7,209 | 96.25 | 0.62 | ||||
4 | 1,665 | 3,935 | 39.37 | 1,820 | 6,197 | 75.9 | 36.53 |
†, 1: disc with fissures of the annulus; 2: degenerated disc; 3: herniated disc; 4: location of the disc. ‡, negative values mean the percentage decreased in the use of old terms; positive values mean percentage increased in the use of new terms.
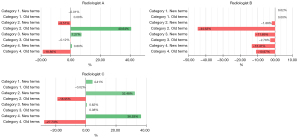
Regarding the herniated disc category, the use of the term “bulging disc” increased from 0.81% to 55.56% for radiologist B and from 3.24% to 24.35% for radiologist C, and the term “extrusion” increased for radiologist B in its use from 3.43% to 33.17%.
Discussion
In this study, we propose the use of NLP tools for the assessment of the level of adherence to the new recommendations (v 2.0) performed in 2014 by the ASSR, ASNR and NASS consensus paper for lumbar spine disc nomenclature in our radiology department (1). The use of a standardized and unified lexicon for reporting lumbar spine MRI is required to minimize variability, improve communication with spine surgeons and reduce potential mistakes.
Our results indicate a positive global impact over the terminology used for reporting MRI lumbar spine exams after the publication of the ASSR, ASNR and NASS consensus paper and its divulgation through an internal formative lecture in March 2015. This impact was more evident related to categories such as disc location, disc herniation or degenerated disc, especially for less experienced radiologists (B and C) which would probably be more permeative to the adoption of new lexicon related to lumbar disc nomenclature as well as to reduce or remove the use of old non-specific terms. However, regarding the paradoxical increase in the use of old terms related to disc degeneration found for the highest experienced radiologist A would probably be related to a misunderstanding of what old terms should no longer be used in the degenerated disc category. This outlier demonstrates how NLP tools can help to identify and explain an incorrect use of the new lexicon proposed helping to resolve the situation (that would otherwise remain unnoticed) (15,16) (Figure 3).
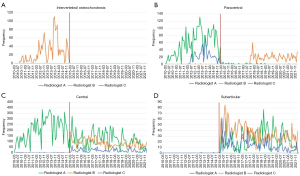
The main contribution of this study is to apply a rule-based NLP method to assess the adoption of the ASSR, ASNR and NASS white paper recommendations using NLP for the first time in the scientific literature. In the literature published, scarce studies have evaluated the potential impact of NLP algorithms for evaluating the level of adherence to internal or external recommendations, even less in the field of MRI lumbar spine reporting whereas no prior benchmarks have been established, so it is hard to establish a direct comparison with our results. Travis Caton et al. used NLP tools to determine patterns of degenerative spinal stenosis on lumbar MRI based on free-text descriptors and establish potential relationships with lumbar spine level, age, and sex (17). They found that spinal canal and neuroforaminal stenosis increase caudally from T12 to L5 being more prevalent in men and finding a higher prevalence in younger patients (under 50 years) and at lower spine levels (L5–S1) compared with older patients. The same group evaluated the effect on reporting time to account for the global severity of lumbar degenerative disease using NLP tools using a composite severity score (18). They extracted information about the severity of lumbar spine and neuroforaminal stenosis from raw radiological report text and created this score, which resulted in a significant and independent predictor of radiologist reporting time. One of the main applications of this score is to weigh qualitative metrics, such as relative value units depending on the study’s complexity. In the same line (evaluation of clinical and financial impact), NLP tools were used by Duszak et al. to assess deficiencies in abdominal ultrasound reports (19). They used as a standard the Current Procedural Terminology (CPT®) that establishes eight elements required for performing abdominal ultrasound reports (liver, pancreas, gallbladder, inferior vein cava, pancreas, kidneys, aorta and bile ducts). They found that deficiencies in abdominal ultrasound reports (i.e., spleen was neglected in up to 40% of the cases) are common and contribute to substantial losses in legitimate professional revenues. They proposed the use of the structured report and to improve physician training to alleviate the rate of these deficiencies. Regarding lumbar spine reports, Huhdanpaa et al. evaluated the potential of NLP tools applied to free-text radiological reports for identifying type 1 Modic endplate changes (20). They selected this type of endplate changes as it may be an important target for potential intervention. They obtain high specificity (99%) but low sensitivity (70%) with a high negative predictive value (0.96%) for this task.
NLP has recently shown its capability for capture, extract and standardize information from free radiology reports and electronic health records in different clinical scenarios (21-23). There is a growing interest in the use of rule-based NLP approaches for extracting relevant information from radiology reports, as we have used for our study. Recently, Laurent et al. evaluated the capability of rule-based NLP for automatic classification of tumor response from radiology reports and how to integrate it into clinical oncology workflow with an overall accuracy of 0.82 (24). An algorithm for extraction of information from abdominal aortic aneurysms from radiology reports has lately been developed by Gaviria-Valencia et al. using a ruled-based algorithm with an accuracy of 0.97 (25). They suggest that this kind of approach would support automatic input for patient care. Although we show the ability of NLP to evaluate the degree of adherence of radiologists to the ASSR, ASNR and NASS recommendations for lumbar disc nomenclature, we also acknowledge several limitations of our work. One major limitation in this study is that all the MRI reports are from the same radiology site which potentially introduces bias in the results. Evaluation of our NLP algorithms at other radiology institutions would provide a better insight about changes in the way MRI lumbar spine studies are reported. Regarding methodology, we have used MRI lumbar spine reports with and without pathological findings. However, since the goal of the manuscript is mainly to evaluate changes in terms related to lumbar disc pathology, the existence of reports without relevant findings does not influence the way radiologists use new terms describing pathology. Since the system matches terms with radiological reports to extract the information from the text, it cannot interpret lexical ambiguities such as homonymy and polysemy. In future work, the use of machine learning (ML) models should be a challenge to be met (26). We are aware that some less frequent categories, such as those related to containment, continuity, migration, volume, or composition of displaced material as well as spinal canal stenosis, have not been considered for analysis in this work. These categories could be included in further analysis.
Application of our NLP research tool with a larger number of radiology reports and other institutions may help to approach a global degree of adherence to the ASSR, ASNR and NASS recommendations for lumbar disc nomenclature. Most importantly, it could also help to identify strengths or potential weaknesses of the actual 2.0 version to elaborate a newer and updated version. Besides, our approach can be used to assess the degree of adherence to other recommendations and guidelines for reporting, for example, the Reporting and Data System (RADS) scales promoted by American College of Radiology (ACR) (27,28). Regarding radiological department workflow, these kinds of NLP applications may help to identify radiologists with scarce adherence to lexicon recommendations encouraging them to use these guidelines or promoting specific radiological lectures for teaching and learning purposes.
Conclusions
The results from this study suggest that the use of NLP tools may play a valuable role in the evaluation of the degree of adoption of radiology departments to the recommendations performed by the ASSR, ASNR and NASS regarding the MRI lumbar spine nomenclature. Our NLP approach helps to evaluate the degree of adherence to new preferred terms by radiologists, detects non-preferred terms in lumbar spine radiology reports, and encourages radiologists to adapt the proposed lexicon. This approach could potentially be used to assess other recommendations in radiology reports.
Acknowledgments
Funding: This study was partially funded by the Ministry of Science and Innovation (MCIN/AEI/10.13039/501100011033) under grant number PTQ2021-012120.
Footnote
Provenance and Peer Review: With the arrangement by the Guest Editors and the editorial office, this article has been reviewed by external peers.
Reporting Checklist: The authors have completed the TRIPOD reporting checklist. Available at: https://qims.amegroups.com/article/view/10.21037/qims-23-1294/rc
Conflicts of Interest: All authors have completed the ICMJE uniform disclosure form (available at https://qims.amegroups.com/article/view/10.21037/qims-23-1294/coif). The special issue “Advances in Diagnostic Musculoskeletal Imaging and Image-guided Therapy” was commissioned by the editorial office without any funding or sponsorship. All authors are employees of HT Medica. A.L. is also the Chair of Radiology and Shareholder in HT Medica. Besides, A.L. reports payments to institution from Springer as book editor, GE Healthcare as lecturer and Siemens Healthineers as member of Digital oncology Advisory Board. The authors have no other conflicts of interest to declare.
Ethical Statement: The authors are accountable for all aspects of the work in ensuring that questions related to the accuracy or integrity of any part of the work are appropriately investigated and resolved. The study was conducted in accordance with the Declaration of Helsinki (as revised in 2013). The study was approved by our local Ethics Committee and individual consent for this retrospective analysis was waived by the local Ethics Committee in view of the retrospective nature based on anonymized radiology reports.
Open Access Statement: This is an Open Access article distributed in accordance with the Creative Commons Attribution-NonCommercial-NoDerivs 4.0 International License (CC BY-NC-ND 4.0), which permits the non-commercial replication and distribution of the article with the strict proviso that no changes or edits are made and the original work is properly cited (including links to both the formal publication through the relevant DOI and the license). See: https://creativecommons.org/licenses/by-nc-nd/4.0/.
References
- Fardon DF, Williams AL, Dohring EJ, Murtagh FR, Gabriel Rothman SL, Sze GK. Lumbar disc nomenclature: version 2.0: Recommendations of the combined task forces of the North American Spine Society, the American Society of Spine Radiology and the American Society of Neuroradiology. Spine J 2014;14:2525-45.
- Appel B. Nomenclature and classification of lumbar disc pathology. Neuroradiology 2001;43:1124-5.
- Shinagare AB, Lacson R, Boland GW, Wang A, Silverman SG, Mayo-Smith WW, Khorasani R. Radiologist Preferences, Agreement, and Variability in Phrases Used to Convey Diagnostic Certainty in Radiology Reports. J Am Coll Radiol 2019;16:458-64.
- Lee R, Cohen MD, Jennings GS. A new method of evaluating the quality of radiology reports. Acad Radiol 2006;13:241-8.
- Yang C, Kasales CJ, Ouyang T, Peterson CM, Sarwani NI, Tappouni R, Bruno M. A succinct rating scale for radiology report quality. SAGE Open Med 2014;2:2050312114563101.
- Steinkamp J, Chambers C, Lalevic D, Cook T. Automatic Fully-Contextualized Recommendation Extraction from Radiology Reports. J Digit Imaging 2021;34:374-84.
- Jungmann F, Arnhold G, Kämpgen B, Jorg T, Düber C, Mildenberger P, Kloeckner R. A Hybrid Reporting Platform for Extended RadLex Coding Combining Structured Reporting Templates and Natural Language Processing. J Digit Imaging 2020;33:1026-33.
- Pons E, Braun LM, Hunink MG, Kors JA. Natural Language Processing in Radiology: A Systematic Review. Radiology 2016;279:329-43.
- López-Úbeda P, Martín-Noguerol T, Juluru K, Luna A. Natural Language Processing in Radiology: Update on Clinical Applications. J Am Coll Radiol 2022;19:1271-85.
- Casey A, Davidson E, Poon M, Dong H, Duma D, Grivas A, Grover C, Suárez-Paniagua V, Tobin R, Whiteley W, Wu H, Alex B. A systematic review of natural language processing applied to radiology reports. BMC Med Inform Decis Mak 2021;21:179.
- Celi LA, Chen C, Gruhl D, Shivade C, Wu JTY. Introduction to Clinical Natural Language Processing with Python. In: Celi L, Majumder M, Ordóñez P, Osorio J, Paik K, Somai M. editors. Leveraging Data Sci Glob Heal Cham: Springer; 2020:229-50.
- Padró L, Stanilovsky E. FreeLing 3.0 : Towards Wider Multilinguality. Proceedings of the Eighth International Conference on Language Resources and Evaluation (LREC'12). 2012:2473-9. Available online: http://nlp.lsi.upc.edu/publications/papers/padro12.pdf
- López-Úbeda P, Díaz-Galiano MC, Montejo-Ráez A, Martín-Valdivia MT, Ureña-López LA. An integrated approach to biomedical term identification systems. Appl Sci 2020;10:1726.
- Wagner W. Natural Language Processing with Python, Analyzing Text with the Natural Language Toolkit. Lang. Resour. Eval. 2010;
- Crombé A, Seux M, Bratan F, Bergerot JF, Banaste N, Thomson V, Lecomte JC, Gorincour G. What Influences the Way Radiologists Express Themselves in Their Reports? A Quantitative Assessment Using Natural Language Processing. J Digit Imaging 2022;35:993-1007.
- López-Úbeda P, Martín-Noguerol T, Luna A. Radiology, explicability and AI: closing the gap. Eur Radiol 2023;33:9466-8.
- Travis Caton M Jr, Wiggins WF, Pomerantz SR, Andriole KP. Effects of age and sex on the distribution and symmetry of lumbar spinal and neural foraminal stenosis: a natural language processing analysis of 43,255 lumbar MRI reports. Neuroradiology 2021;63:959-66.
- Caton MT Jr, Wiggins WF, Pomerantz SR, Andriole KP. The Composite Severity Score for Lumbar Spine MRI: a Metric of Cumulative Degenerative Disease Predicts Time Spent on Interpretation and Reporting. J Digit Imaging 2021;34:811-9.
- Duszak R Jr, Nossal M, Schofield L, Picus D. Physician documentation deficiencies in abdominal ultrasound reports: frequency, characteristics, and financial impact. J Am Coll Radiol 2012;9:403-8.
- Huhdanpaa HT, Tan WK, Rundell SD, Suri P, Chokshi FH, Comstock BA, Heagerty PJ, James KT, Avins AL, Nedeljkovic SS, Nerenz DR, Kallmes DF, Luetmer PH, Sherman KJ, Organ NL, Griffith B, Langlotz CP, Carrell D, Hassanpour S, Jarvik JG. Using Natural Language Processing of Free-Text Radiology Reports to Identify Type 1 Modic Endplate Changes. J Digit Imaging 2018;31:84-90.
- Raza S, Schwartz B. Constructing a disease database and using natural language processing to capture and standardize free text clinical information. Sci Rep 2023;13:8591.
- Jorg T, Kämpgen B, Feiler D, Müller L, Düber C, Mildenberger P, Jungmann F. Efficient structured reporting in radiology using an intelligent dialogue system based on speech recognition and natural language processing. Insights Imaging 2023;14:47.
- Perera N, Dehmer M, Emmert-Streib F. Named Entity Recognition and Relation Detection for Biomedical Information Extraction. Front Cell Dev Biol 2020;8:673.
- Laurent G, Craynest F, Thobois M, Hajjaji N. Automatic Classification of Tumor Response From Radiology Reports With Rule-Based Natural Language Processing Integrated Into the Clinical Oncology Workflow. JCO Clin Cancer Inform 2023;7:e2200139.
- Gaviria-Valencia S, Murphy SP, Kaggal VC, McBane Ii RD, Rooke TW, Chaudhry R, Alzate-Aguirre M, Arruda-Olson AM. Near Real-time Natural Language Processing for the Extraction of Abdominal Aortic Aneurysm Diagnoses From Radiology Reports: Algorithm Development and Validation Study. JMIR Med Inform 2023;11:e40964.
- López-Úbeda P, Martín-Noguerol T, Luna A. Radiology in the era of large language models: the near and the dark side of the moon. Eur Radiol 2023;33:9455-7.
- Sefidbakht S, Jalli R, Izadpanah E. Adherence of Academic Radiologists in a Non-English Speaking Imaging Center to the BI-RADS Standards of Reporting Breast MRI. J Clin Imaging Sci 2015;5:66.
- An JY, Unsdorfer KML, Weinreb JC. BI-RADS, C-RADS, CAD-RADS, LI-RADS, Lung-RADS, NI-RADS, O-RADS, PI-RADS, TI-RADS: Reporting and Data Systems. Radiographics 2019;39:1435-6.