A radiomics model of contrast-enhanced computed tomography for predicting post-acute pancreatitis diabetes mellitus
Introduction
Acute pancreatitis (AP) is a common acute abdominal condition with an increasing incidence worldwide (1). Although AP is often considered a self-limiting disease, there is growing evidence that a new-onset disturbance in glucose metabolism can occur following AP (2). Recently, there has been growing interest in post-acute pancreatitis diabetes mellitus (PPDM-A). According to a 2014 systematic review and meta-analysis, 23% of AP patients develop new-onset diabetes during follow-up (3). A 2015 national population-based study showed that AP patients had greater than twofold higher risk of diabetes mellitus than individuals without AP (4). The prevalence of PPDM-A has almost tripled during the past 10 years, and its incidence is expected to increase by 2.8% per year, reaching 15.8 per 100,000 general population by 2050 (5,6). PPDM-A confers a higher risk of pancreatic cancer mortality and shorter life expectancy than type 2 diabetes (7-9). Therefore, it would be useful to develop an effective method for screening PPDM-A.
Previous studies have mainly examined the clinical risk factors for predicting PPDM-A (10-13). However, the simple clinical models showed low accuracy and stability and lacked individual specificity, which could be caused by the differences in research methods and clinical factors. Although imaging examinations performed well in diagnosing AP, there have been few imaging studies on the risk factors of PPDM-A. These studies have only analyzed the effects of intra-pancreatic fat deposition, Balthazar’s score, and pancreatic necrosis on the risk of developing PPDM-A (14-16). It has found that excess intra-pancreatic fat deposition is present in patients with PPDM-A, which can destroy pancreatic cells, impair pancreatic endocrine function, and ultimately increase the risk of pancreatic cancer (17). However, conventional imaging examinations are unable to analyze pancreatic microscopic changes at the molecular and cellular levels. Moreover, the existing predictive models only contain the traditional morphological features visible to the naked eye and do not include the potential subtle features that reflect disease heterogeneity. Therefore, accurate quantitative methods to predict PPDM-A are still lacking.
Quantitative imaging features are extracted from digital images in a high-throughput manner and changes in the human body at the tissue, cellular, and gene level can therefore be reflected by radiomics (18,19). Studies have found that radiomics models can be used to evaluate the severity of AP and indicate the prognosis and evolution of pancreatitis (20-22). One previous study developed a computed tomography (CT)-based radiomics nomogram to predict the incidence of PPDM-A, which was a single-center study with a small sample size, and only radiomic features were extracted from unenhanced plain CT images, ignoring potentially valuable information (23). Therefore, we established a radiomics model based on the arterial- and venous-phase contrast-enhanced CT (CECT) images at the first onset of AP from two tertiary care centers and compared it with a clinical model and a combined model based on the clinical and radiomic features to evaluate its capability to predict PPDM-A. We present this article in accordance with the TRIPOD reporting checklist (available at https://qims.amegroups.com/article/view/10.21037/qims-23-1232/rc).
Methods
Patients
This retrospective, bicentric study was conducted in accordance with the Declaration of Helsinki (as revised in 2013). The study was approved by the Institutional Review Board of the Second Affiliated Hospital of Chongqing Medical University and the First Affiliated Hospital of Chongqing Medical University (No. 315. dated December 30, 2021), and individual consent for this retrospective analysis was waived. We retrospectively recruited AP patients who had been hospitalized at institution 1 (the Second Affiliated Hospital of Chongqing Medical University) in the period from August 2014 to August 2022 and at institution 2 (the First Affiliated Hospital of Chongqing Medical University) from January 2018 to August 2022. The baseline information and imaging data were collected and analyzed.
Depending on the occurrence of diabetes after AP, the patients were divided into PPDM-A group and non-PPDM-A group (24,25). In PPDM-A group, the patients with a first onset of AP were followed up for the development of diabetes mellitus from August 2014 to August 2023. In non-PPDM-A group, the patients with a first AP onset were enrolled between August 2014 and August 2022 and were followed up through August 2023 via the analysis of medical records and telephone interviews to verify that diabetes mellitus did not occur during the follow-up period. In PPDM-A group, the mean interval time between the first onset of AP and the diagnosis of diabetes was 29±24.6 months (3–120 months). In non-PPDM-A group, the mean follow-up time was 59.8±28.1 months (12.3–134.8 months).
According to the 2012 Atlanta consensus criteria (26), AP was diagnosed based on the identification of at least two of the following three signs: (I) the presence of typical abdominal pain; (II) elevated serum amylase or lipase levels at least three times greater than the upper limit of normal; and (III) characteristic imaging findings of AP.
Diabetes was diagnosed by recording at least one of the following (27): (I) fasting plasma glucose levels ≥126 mg/dL (7.0 mmol/L); (II) plasma glucose ≥11.1 mmol/L 2 hours after a 75 g oral glucose load; (III) random blood glucose ≥200 mg/dL (11.1 mmol/L) accompanied by diabetes symptoms; and (IV) glycosylated haemoglobin ≥6.5% (48 mmol/dL).
The inclusion and exclusion criteria for PPDM-A group were as follows: inclusion criteria: (I) patients were diagnosed with diabetes mellitus >90 days after the date of first pancreatitis diagnosis (24,25,28); (II) upper abdominal CECT examinations performed within 7 days of the onset of AP; and (III) inpatients. Exclusion criteria: (I) having any history of diabetes; (II) elevated blood glucose during hospitalization; (III) high blood glucose within 90 days after discharge; (IV) acute onset of chronic pancreatitis; (V) cancer or severe chronic wasting diseases; (VI) loss to follow-up; (VII) unsatisfactory images or incomplete medical records; and (VIII) less than 18 years of age.
The inclusion criteria applied to the non-PPDM-A were based on the diagnosis of AP and (I) a detailed admission index at the first AP onset and (II) there was no abnormal blood sugar index, after a detailed review of medical records or follow-up telephone consultations through August 2023. The exclusion criteria were as follows: (I) acute onset of chronic pancreatitis; (II) cancer or severe chronic wasting diseases; (III) complications resulting from abnormal pancreatic anatomical features; (IV) loss to follow-up; and (V) unsatisfactory images or incomplete medical records.
A total of 374 patients with first onset AP were recruited (mean age, 48±15 years; age range, 18–86 years; 231 men), comprising 239 participants in the non-PPDM-A group and 135 participants in the PPDM-A group. The patients from institution 1 were randomly assigned to the training cohort and the internal validation cohort at a ratio of 8:2, while those from institution 2 served as the external validation cohort. Twelve clinical characteristics, namely age, gender, disease severity based on the 2012 Revised Atlanta Criteria (26), CT severity index (CTSI), extra-pancreatic inflammation on CT (EPIC) (29), etiology, hospital stay, pancreatic necrosis, the number of recurrences, follow-up time, and smoking and drinking status, were collected from the hospital information system. The classification of etiological factors and the definition of recurrent AP are shown in Section S1 in Appendix 1, and the study flowchart is presented in Figure 1.
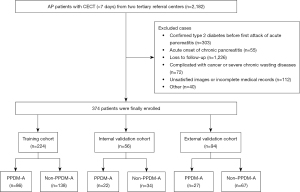
CECT image collection
All of the patients underwent an abdominal CECT examination within 7 days after the onset of AP symptoms with one of three multidetector row CT systems. We retrieved anonymous arterial- and venous-phase CECT images from the picture archiving and communication system for feature extraction. The detailed image collection information is provided in Section S2 in Appendix 1.
Image segmentation, preprocessing, and feature extraction
Two abdominal radiologists with 8 and 12 years of experience used the IntelliSpace Discovery platform (ISD, Philips Healthcare, Best, the Netherlands) independently, without knowing the patient’s clinical outcomes. On the ISD platform, a three-dimensional region of interest (3DROI) containing the whole pancreas was semiautomatically generated by delineating the pancreatic boundaries on the CECT image, including pancreatic necrosis but avoiding the bile ducts and blood vessels (30). Then, the plugin-pyradiomics 2.1 on the ISD platform was used to extract radiomics features for each 3DROI. A total of 1,199 radiomics features were extracted, as shown in Section S3 in Appendix 1 and Figure S1. For each patient, we used two different 3DROIs from CECT images of the arterial and portal venous phases and integrated all 2,398 radiomics features from the two 3DROIs. To ensure the repeatability of the results, the Min–Max normalization and resampling were used to preprocess the data and images, respectively (Section S4 in Appendix 1). The detailed radiomics process is presented in Figure 2.
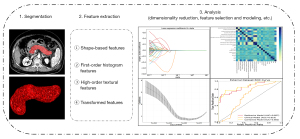
Intraobserver reliability and interobserver agreement
Two abdominal radiologists selected CECT images of random 30 patients, without knowing the patient’s clinical outcomes, to assess intraobserver and interobserver agreement for feature extraction. To evaluate intraobserver reliability, observer 1 used the same method to delineate 3DROI and extract radiomics features twice within 7 days. To evaluate interobserver agreement, observer 2 delineated the 3DROI only once, and we compared the obtained features with the features extracted after the first delineation by observer 1. Intraclass correlation coefficients (ICCs) were used to assess the intraobserver reliability and interobserver agreement. The features with an ICC value of above 0.75 were considered stable, while the remaining features were considered unstable and eliminated.
Dimensionality reduction and feature selection
To avoid the dimensionality disarray and reduce the bias of radiomics features, the following measures were taken to select features in the training group. First, random forest-based recursive feature elimination was used to select features. Then, collinearity analysis was performed on the features selected by the random forest algorithm. The features with Pearson correlation coefficient (r) greater than 0.9 were randomly deleted to remove redundancy. Finally, the least absolute shrinkage and selection operator (LASSO) was used for dimensionality reduction to select the optimal features by performing regularization and variable selection so as to improve the predictive accuracy and reliability of the radiomics model. The idea was to control the amounts of features by introducing L1 regularization terms and to reduce the coefficients of some unimportant features. By adjusting the regularization parameter λ, the weight of regularization terms can be controlled. And the best regression function of the model can be selected while filtered out the sparse features.
Modeling
Three classification methods [eXtreme Gradient Boosting (XGBoost), Adaptive Boosting (AdaBoost), and Decision Tree] were built in three models to compare the performances. Fivefold cross-validation was applied to tune hyperparameters for better model performance. The predictive performances of the models were compared based on the area under the receiver operating characteristic (ROC) curve (AUC), accuracy, sensitivity, specificity, positive predictive value, negative predictive value, and F1-score. Each of the three models was modeled with three algorithms, resulting in a total of nine classifiers. The performances were compared both within and between the models.
Statistical analysis
Statistical analyses were performed using SPSS for Windows (version 22.0, Chicago, IL, USA). The data in different groups were tested for normality using the Shapiro-Wilk test. Continuous variables that approximated a normal distribution were expressed as mean ± standard deviation; continuous variables that were skewed were expressed as median (interquartile range). Independent-sample t-test or Mann-Whitney U test was applied to compare continuous variables. Chi-squared test or Fisher’s exact test was used to compare categorical variables. Radiomics feature analysis, modeling and visual analysis were performed using Python 3.9.13 and R (version 4.3.1, https://www.r-project.org/). Pandas 1.4.4 and Numpy 1.21.5 were used for data preprocessing and normalization. Scikit-learn 1.0.2 was used for feature selection methods, establishment of machine learning models, and drawing the ROC curve. R was used for visual analysis and basic data analysis since it has a powerful graphing function. DeLong test was used to compare the AUCs of these models. The P values were two-sided, and P values lower than 0.05 were considered statistically significant.
Results
Patient characteristics
The detailed clinical characteristics of the AP patients in the training, internal validation, and external validation cohorts are shown in Table 1. The prevalence of PPDM-A was 38.39%, 39.29%, and 28.72% in the training, internal validation, and external validation cohorts, respectively, with no statistically significant difference among the three cohorts (P=0.236). Of the 12 clinical characteristics measured, only the follow-up time and the number of recurrences significantly differed between the PPDM-A group and the non-PPDM-A group for the training cohort (P=0.018 and P<0.001, respectively) and the internal validation cohort (P<0.001 and P=0.004, respectively). The gender (P=0.017) and smoking status (P=0.042) significantly differed between the PPDM-A group and the non-PPDM-A group only in the training cohort. All of the clinical characteristics were normalized to values between 0 and 1 using Min–Max normalization, and nine features were selected by LASSO. Three clinical characteristics, namely, etiology, disease severity, and pancreatic necrosis, were excluded.
Table 1
Characteristics | Institution 1 | Institution 2 | |||||||||
---|---|---|---|---|---|---|---|---|---|---|---|
Training cohort (n=224) | Internal validation cohort (n=56) | External validation cohort (n=94) | |||||||||
PPDM-A (n=86) |
Non-PPDM-A (n=138) | P | PPDM-A (n=22) |
Non-PPDM-A (n=34) | P | PPDM-A (n=27) |
Non-PPDM-A (n=67) | P | |||
Gender | 0.017* | 0.757 | 0.074 | ||||||||
Male | 60 (69.77) | 74 (53.62) | 14 (63.64) | 23 (67.65) | 21 (77.78) | 39 (58.21) | |||||
Female | 26 (30.23) | 64 (46.38) | 8 (36.36) | 11 (32.35) | 6 (22.22) | 28 (41.79) | |||||
Age (years) | 46.97±15.31 | 48.88±15.18 | 0.362 | 50.32±13.54 | 49.03±15.04 | 0.746 | 51.37±15.88 | 46.15±16.03 | 0.16 | ||
Etiology | 0.058 | 0.089 | 0.111 | ||||||||
Biliary | 21 (24.42) | 56 (40.58) | 4 (18.18) | 15 (44.12) | 11 (40.74) | 33 (49.25) | |||||
Hypertriglyceridemia | 29 (33.72) | 29 (21.01) | 7 (31.82) | 11 (32.35) | 10 (37.04) | 18 (26.87) | |||||
Alcoholic | 6 (6.98) | 9 (6.52) | 1 (4.55) | 2 (5.88) | 2 (7.41) | 0 | |||||
Idiopathic | 30 (34.88) | 44 (31.88) | 10 (45.45) | 6 (17.65) | 4 (14.81) | 16 (23.88) | |||||
CTSI | 4 [3–6] | 4 [3–4] | 0.992 | 3.5 [3–4] | 4 [2.75–4] | 0.889 | 4 [3–6] | 4 [3–6] | 0.403 | ||
Disease severity | 0.868 | 0.516 | 0.325 | ||||||||
Mild | 18 (20.93) | 26 (18.84) | 4 (18.18) | 10 (29.41) | 6 (22.22) | 11 (16.42) | |||||
Moderate | 63 (73.26) | 102 (73.91) | 18 (81.82) | 23 (67.65) | 20 (74.07) | 46 (68.65) | |||||
Severe | 5 (5.81) | 10 (7.25) | 0 | 1 (2.94) | 1 (3.8) | 10 (14.93) | |||||
EPIC | 5 [2–7] | 5 [2–7] | 0.447 | 4.5 [2.75–6] | 4.5 [1–7] | 0.858 | 5 [2–6] | 5 [3–7] | 0.449 | ||
Hospital stay (days) | 13 [8–20] | 15 [9–21] | 0.243 | 13 [8.75–16.25] | 11 [7–17.25] | 0.608 | 12 [9–22] | 18 [11–30] | 0.083 | ||
Follow-up time (months) | 22 [9–45] | 15 [6–29] | 0.018* | 24.5 [18.5–37.5] | 5.5 [3–20] | <0.001* | 20 [8–36] | 11 [5–27] | 0.071 | ||
Pancreatic necrosis | 0.428 | 0.613 | 0.595 | ||||||||
Yes | 24 (27.91) | 32 (23.19) | 4 (18.18) | 6 (17.65) | 8 (29.63) | 20 (29.85) | |||||
No | 62 (72.09) | 106 (76.81) | 18 (81.82) | 28 (82.35) | 19 (70.37) | 47 (70.15) | |||||
Number of recurrences | <0.001* | 0.004* | 0.266 | ||||||||
0 recurrence | 23 (26.74) | 91 (65.94) | 5 (22.73) | 22 (64.71) | 11 (40.75) | 40 (59.7) | |||||
1 recurrence | 41 (47.67) | 35 (25.36) | 6 (27.27) | 8 (23.53) | 14 (51.85) | 22 (32.84) | |||||
2 recurrences | 12 (13.95) | 6 (4.35) | 6 (27.27) | 3 (8.82) | 1 (3.7) | 3 (4.48) | |||||
≥3 recurrences | 10 (11.63) | 6 (4.35) | 5 (22.73) | 1 (2.94) | 1 (3.7) | 2 (2.99) | |||||
Smoking | 0.042* | 0.752 | 0.02* | ||||||||
Yes | 39 (45.35) | 44 (31.88) | 10 (45.45) | 14 (41.18) | 15 (55.56) | 20 (29.85) | |||||
No | 47 (54.65) | 94 (68.12) | 12 (54.55) | 20 (58.82) | 12 (44.44) | 47 (70.15) | |||||
Drinking | 0.296 | 0.004* | 0.542 | ||||||||
Yes | 26 (30.23) | 33 (23.91) | 7 (31.82) | 24 (70.59) | 6 (22.22) | 19 (28.36) | |||||
No | 60 (69.77) | 105 (76.09) | 15 (68.18) | 10 (29.41) | 21 (77.78) | 48 (71.64) |
The age is approximate a normal distribution which are expressed as mean ± standard deviation; CTSI, EPIC, hospital stay and follow-up time are skewed distribution which are expressed as median [interquartile range]. The gender, etiology, disease severity, pancreatic necrosis, number of recurrences, smoking and drinking status are expressed as number (frequency). *, P<0.05. PPDM-A, post-acute pancreatitis diabetes mellitus; CTSI, computed tomography severity index; EPIC, extra-pancreatic inflammation on computed tomography.
Intraobserver reliability and interobserver agreement
Regarding intraobserver reliability, a total of 2,130 features reached satisfactory agreement, with a mean ICC value of 0.957 and a range of 0.752–1.000, as shown in Figure 3A. The remaining 268 features were eliminated. For interobserver agreement, 1,807 features reached satisfactory agreement, with a mean ICC value of 0.946 and a range of 0.75–1.000, as shown in Figure 3B. The 591 features with unsatisfactory agreement were eliminated. Among the eliminated features, 140 features were identical between the intraobserver and interobserver agreement. Finally, 719 features were excluded, and the remaining 1,679 features were kept for further analyses.
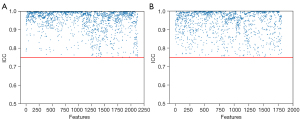
Dimensionality reduction and feature selection
First, 505 radiomics features were selected by random forest-based recursive feature elimination. Next, 193 radiomics features remained after checking the collinearity. Then, 11 radiomics features were selected by LASSO regression, and the value of the best-tuned regularization parameter (λ) was 0.01. Finally, eleven radiomics features were selected (Section S5 in Appendix 1 for detailed feature information).
Modeling
Overall, among the three classification methods, the XGBoost classifier showed better and more consistent performances. In the external validation cohort, the XGBoost method achieved an AUC of 0.857 [95% confidence interval (CI): 0.855–0.981] in the radiomics model and an AUC of 0.882 (95% CI: 0.844–0.981) in the combined model. The AdaBoost method achieved AUC values of 0.837 (95% CI: 0.870–0.985) and 0.788 (95% CI: 0.852–0.981) in the radiomics and combined models, respectively. The Decision Tree method showed some AUC values of 0.5, meaning that the corresponding classification model was worthless. The detailed information on the AdaBoost and Decision Tree methods is shown in Tables S1,S2. Therefore, we further focused only on the best method’s (XGBoost) performance among the three models.
The XGBoost’s radiomics model performed well in predicting PPDM-A (Table 2). In the training cohort, the AUC and accuracy were 0.964 (95% CI: 0.896–0.967) and 0.964, respectively. In the internal validation cohort, the AUC and accuracy were 0.901 (95% CI: 0.849–0.964) and 0.875, respectively. In the external validation cohort, the AUC and accuracy were 0.857 (95% CI: 0.855–0.981) and 0.968, respectively.
Table 2
Model | Training cohort | Internal validation cohort | External validation cohort | ||||||||
---|---|---|---|---|---|---|---|---|---|---|---|
Clinical | Radiomics | Combined | Clinical | Radiomics | Combined | Clinical | Radiomics | Combined | |||
AUC (95% CI) | 0.685 (0.613, 0.719) |
0.964 (0.896, 0.967) |
0.980 (0.916, 0.982) |
0.733 (0.5, 0.5) |
0.901 (0.849, 0.964) |
0.901 (0.789, 0.963) |
0.619 (0.50, 0.64) |
0.857 (0.855, 0.981) |
0.882 (0.844, 0.981) |
||
Accuracy | 0.701 | 0.964 | 0.978 | 0.696 | 0.875 | 0.893 | 0.596 | 0.968 | 0.840 | ||
Sensitivity | 0.616 (53/86) | 0.965 (83/86) | 0.988 (85/86) | 0.773 (17/22) | 0.773 (17/22) | 0.955 (21/22) | 0.407 (11/27) | 0.963 (26/27) | 0.778 (21/27) | ||
Specificity | 0.754 (104/138) | 0.964 (133/138) | 0.971 (134/138) | 0.647 (22/34) | 0.941 (32/34) | 0.853 (29/34) | 0.672 (45/67) | 0.970 (65/67) | 0.866 (58/67) | ||
PPV | 0.609 (53/87) | 0.943 (83/88) | 0.955 (85/89) | 0.586 (17/29) | 0.895 (17/19) | 0.808 (21/26) | 0.333 (11/33) | 0.929 (26/28) | 0.700 (21/30) | ||
NPV | 0.759 (104/137) | 0.978 (133/136) | 0.993 (134/135) | 0.815 (22/27) | 0.865 (32/37) | 0.967 (29/30) | 0.738 (45/61) | 0.985 (65/66) | 0.906 (58/64) | ||
F1-score | 0.613 | 0.953 | 0.971 | 0.667 | 0.844 | 0.875 | 0.367 | 0.945 | 0.737 |
The brackets in the table show the numerator and denominator of the sensitivity, specificity, PPV and NPV. AUC, area under the receiver operating characteristic curve; CI, confidence interval; PPV, positive predictive value; NPV, negative predictive value.
The XGBoost’s clinical model did not perform well in predicting PPDM-A (Table 2). In the training cohort, the AUC and accuracy were 0.685 (95% CI: 0.613–0.719) and 0.701, respectively. In the internal validation cohort, the AUC and accuracy were 0.733 (95% CI: 0.5–0.5) and 0.696, respectively. In the external validation cohort, the AUC and accuracy were 0.619 (95% CI: 0.50–0.64) and 0.596, respectively.
The XGBoost’s combined model also showed good performance (Table 2). In the training cohort, the AUC and accuracy were 0.980 (95% CI: 0.916–0.982) and 0.978, respectively. In the internal validation cohort, the AUC and accuracy were 0.901 (95% CI: 0.789–0.963) and 0.893, respectively. In the external validation cohort, the AUC and accuracy were 0.882 (95% CI: 0.844–0.981) and 0.840, respectively.
By comparing the AUCs among the three XGBoost’s models, we found that the radiomics model was significantly better than the clinical model in the external validation cohort (0.857 vs. 0.619, P<0.001), but there was no significant difference between the combined and radiomics models (0.882 vs. 0.857, P=0.317). The ROC evaluation of the models is shown in Figure 4.
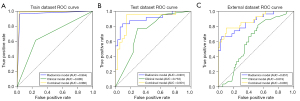
Discussion
In this study, a quantitative radiomics model based on CECT images was developed and validated, which provided a noninvasive and individualized method to predict PPDM-A. The radiomics model showed good performance in the external validation cohort, with an accuracy of 0.968, suggesting that the clinical application of radiomics is promising in predicting PPDM-A.
The risk of PPDM-A increases with increasing follow-up time, the number of recurrences, and other clinical factors (31-33). In this study, we found significant differences in the follow-up time and the number of recurrences between the PPDM-A and non-PPDM-A groups in the training and internal validation cohorts. However, in the external validation cohort, the follow-up time was longer in the PPDM-A group than in the non-PPDM-A group, but the number of recurrences was not significantly different. In addition, the gender and smoking status were significantly different only in the training cohort. We speculate that the reason for these results may be the small sample size. According to large population-based studies, the severity of the initial AP episode, pancreatic necrosis, and etiology are not related to the risk of PPDM-A (3,4,32), which is consistent with our findings. However, several studies have reported that pancreatic necrosis is an independent risk factor for PPDM-A (16,34). In clinical practice, PPDM-A can occur without pancreatic necrosis, suggesting that pancreatic necrosis is not the only reason for developing PPDM-A. Therefore, LASSO regression was used to exclude etiology, pancreatic necrosis, and severity so as to improve the accuracy of the clinical model. However, the accuracy of the clinical model to predict PPDM-A was only approximately 0.7, which was far from satisfactory.
In contrast, our proposed radiomics model showed obvious advantages in predicting PPDM-A compared with the clinical model. In this study, we analyzed CECT radiomics in patients with AP, and the results indicated that some patients were already at high risk of PPDM-A during the first episode of AP. There is evidence that stress hyperglycemia occurs in up to 70% of patients during the course of AP, and diabetes mellitus occurs in nearly 25% of patients after AP, suggesting that some patients experience a temporary or permanent impairment of pancreatic endocrine function during AP attacks (35,36). In recent years, it has been suggested that the pathogenesis of PPDM-A involves multiple factors. Previous studies have confirmed that chronic low-grade inflammation caused by elevated levels of proinflammatory cytokines during AP attacks is the key pathway of PPDM-A (37). In addition, proinflammatory cytokines-stimulated lipolysis plays an important role in the pathogenesis of PPDM-A (38,39). It has been shown that intra-pancreatic fat deposition occurs in patients with PPDM-A, which may damage the Langerhans islet cells and impair insulin secretion (14,15). The dysfunction of the pancreas-gut-brain axis may also lead to PPDM-A (40,41). Both the enteroendocrine and brain cells can secrete the glucoregulatory peptides that affect the pancreatic islet cells, and the glucoregulatory peptides can affect the interaction between the nervous system and the gastrointestinal tract and regulate glucose metabolism (40). Therefore, PPDM-A may be caused by the changes in various factors in the microenvironment in vivo, and radiomics can reflect these changes to predict PPDM-A.
Zhong et al. (23) developed a CT-based radiomics nomogram for predicting PPDM-A incidence. The paper’s authors conducted a single-center study with a small sample size and extracted radiomics features from only the unenhanced plain CT images, so potentially valuable information had been overlooked. And the AUC and accuracy of the CT-based radiomics nomogram to predict the PPDM-A incidence was only 0.743 and 0.815, respectively. Whereas our study collected arterial- and venous-phase CECT images from two tertiary referral centers, and provided an independent external validation cohort, providing more abundant image information and increasing the advantages of our results for clinical application. Besides, to reduce the influence of variable CT parameters on the stability of the radiomics features, we used resampling to preprocess the images. It has been shown that resampling can maintain feature stability by optimizing gray-level discretization (42,43).
In our study, the radiomics model in the external validation cohort had a higher accuracy not only than the internal validation cohort but also the training cohort. We speculate that the reason for this situation may be due to the class imbalance. The prevalence of PPDM-A was higher in both the training and internal validation group (38.39% and 39.29%, respectively) than in the external validation group (28.72%). Besides, only the accuracy of the radiomics model was slightly higher. Therefore, we thought this was a normal phenomenon, which also can be found in the studies by the Du et al. and Ouyang et al. (44,45).
Our study has several limitations. First, the result might be slightly affected due to class imbalance. The imbalance learning techniques, such as the synthetic minority oversampling technique, undersampling, oversampling, are prone to overfitting problems and the generated sample may introduce noises (46). Although we did not adopt methods to deal with the class imbalance, the AUC values of our model were good eventually. In addition, many studies also did not take the approach of dealing with class imbalances and got satisfactory results (47,48). Second, although the follow-up period was relatively long, it is possible that PPDM-A did not occur within the follow-up period in some patients, which could have led to false-negative predictions. Besides, the clinical characteristics of AP patients were limited, which may have affected the prediction effect of PPDM-A in the comprehensive evaluation. Finally, the sample size was not large enough, which may have led to a slight selection bias. In the future, we will conduct a large prospective study to address these issues.
Conclusions
In conclusion, we proposed a radiomics model based on CECT images, providing a convenient and noninvasive method for predicting PPDM-A.
Acknowledgments
The authors would like to thank Dr. Zhixuan Song (Philips Healthcare, Guangzhou, China) for the assistance with statistics and data visualization.
Funding: This work was sponsored by
Footnote
Reporting Checklist: The authors have completed the TRIPOD reporting checklist. Available at https://qims.amegroups.com/article/view/10.21037/qims-23-1232/rc
Conflicts of Interest: All authors have completed the ICMJE uniform disclosure form (available at https://qims.amegroups.com/article/view/10.21037/qims-23-1232/coif). R.H. reports that this research was funded by the Chengdu University of Traditional Chinese Medicine “Xinglin Scholars” Discipline Talents Research Promotion Plan (grant No. YYZX2021059). D.Z. reports that this research was sponsored by Natural Science Foundation of Chongqing, China (grant No. CSTB2023NSCQ-MSX0154) and the First Affiliated Hospital of Chongqing Medical University (grant No. CYYY-BSHPYXM-202305). The other authors have no conflicts of interest to declare.
Ethical Statement: The authors are accountable for all aspects of the work in ensuring that questions related to the accuracy or integrity of any part of the work are appropriately investigated and resolved. The study was conducted in accordance with the Declaration of Helsinki (as revised in 2013). The study was approved by the institutional review board of the Second Affiliated Hospital of Chongqing Medical University and the First Affiliated Hospital of Chongqing Medical University (No. 315. Dated December 30, 2021), and individual consent for this retrospective analysis was waived.
Open Access Statement: This is an Open Access article distributed in accordance with the Creative Commons Attribution-NonCommercial-NoDerivs 4.0 International License (CC BY-NC-ND 4.0), which permits the non-commercial replication and distribution of the article with the strict proviso that no changes or edits are made and the original work is properly cited (including links to both the formal publication through the relevant DOI and the license). See: https://creativecommons.org/licenses/by-nc-nd/4.0/.
References
- Forsmark CE, Vege SS, Wilcox CM. Acute Pancreatitis. N Engl J Med 2016;375:1972-81. [Crossref] [PubMed]
- Bharmal SH, Cho J, Ko J, Petrov MS. Glucose variability during the early course of acute pancreatitis predicts two-year probability of new-onset diabetes: A prospective longitudinal cohort study. United European Gastroenterol J 2022;10:179-89. [Crossref] [PubMed]
- Das SL, Singh PP, Phillips AR, Murphy R, Windsor JA, Petrov MS. Newly diagnosed diabetes mellitus after acute pancreatitis: a systematic review and meta-analysis. Gut 2014;63:818-31. [Crossref] [PubMed]
- Shen HN, Yang CC, Chang YH, Lu CL, Li CY. Risk of Diabetes Mellitus after First-Attack Acute Pancreatitis: A National Population-Based Study. Am J Gastroenterol 2015;110:1698-706. [Crossref] [PubMed]
- Pendharkar SA, Mathew J, Petrov MS. Age- and sex-specific prevalence of diabetes associated with diseases of the exocrine pancreas: A population-based study. Dig Liver Dis 2017;49:540-4. [Crossref] [PubMed]
- Cho J, Petrov MS. Pancreatitis, Pancreatic Cancer, and Their Metabolic Sequelae: Projected Burden to 2050. Clin Transl Gastroenterol 2020;11:e00251. [Crossref] [PubMed]
- Cho J, Pandol SJ, Petrov MS. Risk of cause-specific death, its sex and age differences, and life expectancy in post-pancreatitis diabetes mellitus. Acta Diabetol 2021;58:797-807. [Crossref] [PubMed]
- Cho J, Scragg R, Petrov MS. Postpancreatitis Diabetes Confers Higher Risk for Pancreatic Cancer Than Type 2 Diabetes: Results From a Nationwide Cancer Registry. Diabetes Care 2020;43:2106-12. [Crossref] [PubMed]
- Petrov MS. DIAGNOSIS OF ENDOCRINE DISEASE: Post-pancreatitis diabetes mellitus: prime time for secondary disease. Eur J Endocrinol 2021;184:R137-49. [Crossref] [PubMed]
- Soo DHE, Pendharkar SA, Jivanji CJ, Gillies NA, Windsor JA, Petrov MS. Derivation and validation of the prediabetes self-assessment screening score after acute pancreatitis (PERSEUS). Dig Liver Dis 2017;49:1146-54. [Crossref] [PubMed]
- Man T, Seicean R, Lucaciu L, Istrate A, Seicean A. Risk factors for new-onset diabetes mellitus following acute pancreatitis: a prospective study. Eur Rev Med Pharmacol Sci 2022;26:5745-54. [Crossref] [PubMed]
- Zhang J, Lv Y, Hou J, Zhang C, Yua X, Wang Y, Yang T, Su X, Ye Z, Li L. Machine learning for post-acute pancreatitis diabetes mellitus prediction and personalized treatment recommendations. Sci Rep 2023;13:4857. [Crossref] [PubMed]
- Ma JH, Yuan YJ, Lin SH, Pan JY. Nomogram for predicting diabetes mellitus after the first attack of acute pancreatitis. Eur J Gastroenterol Hepatol 2019;31:323-8. [Crossref] [PubMed]
- Singh RG, Nguyen NN, DeSouza SV, Pendharkar SA, Petrov MS. Comprehensive analysis of body composition and insulin traits associated with intra-pancreatic fat deposition in healthy individuals and people with new-onset prediabetes/diabetes after acute pancreatitis. Diabetes Obes Metab 2019;21:417-23. [Crossref] [PubMed]
- Singh RG, Cervantes A, Kim JU, Nguyen NN, DeSouza SV, Dokpuang D, Lu J, Petrov MS. Intrapancreatic fat deposition and visceral fat volume are associated with the presence of diabetes after acute pancreatitis. Am J Physiol Gastrointest Liver Physiol 2019;316:G806-15. [Crossref] [PubMed]
- Tu J, Yang Y, Zhang J, Yang Q, Lu G, Li B, Tong Z, Ke L, Li W, Li J. Effect of the disease severity on the risk of developing new-onset diabetes after acute pancreatitis. Medicine (Baltimore) 2018;97:e10713. [Crossref] [PubMed]
- Petrov MS. Post-pancreatitis diabetes mellitus and excess intra-pancreatic fat deposition as harbingers of pancreatic cancer. World J Gastroenterol 2021;27:1936-42. [Crossref] [PubMed]
- Lambin P, Leijenaar RTH, Deist TM, Peerlings J, de Jong EEC, van Timmeren J, Sanduleanu S, Larue RTHM, Even AJG, Jochems A, van Wijk Y, Woodruff H, van Soest J, Lustberg T, Roelofs E, van Elmpt W, Dekker A, Mottaghy FM, Wildberger JE, Walsh S. Radiomics: the bridge between medical imaging and personalized medicine. Nat Rev Clin Oncol 2017;14:749-62. [Crossref] [PubMed]
- Zhao Y, Wei J, Xiao B, Wang L, Jiang X, Zhu Y, He W. Early prediction of acute pancreatitis severity based on changes in pancreatic and peripancreatic computed tomography radiomics nomogram. Quant Imaging Med Surg 2023;13:1927-36. [Crossref] [PubMed]
- Lin Q, Ji YF, Chen Y, Sun H, Yang DD, Chen AL, Chen TW, Zhang XM. Radiomics model of contrast-enhanced MRI for early prediction of acute pancreatitis severity. J Magn Reson Imaging 2020;51:397-406. [Crossref] [PubMed]
- Chen Y, Chen TW, Wu CQ, Lin Q, Hu R, Xie CL, Zuo HD, Wu JL, Mu QW, Fu QS, Yang GQ, Zhang XM. Radiomics model of contrast-enhanced computed tomography for predicting the recurrence of acute pancreatitis. Eur Radiol 2019;29:4408-17. [Crossref] [PubMed]
- Tang L, Ma L, Chen Y, Hu Y, Chen X, Huang X, Liu N. Radiomics analysis of contrast-enhanced T1W MRI: predicting the recurrence of acute pancreatitis. Sci Rep 2023;13:2762. [Crossref] [PubMed]
- Zhong S, Du Q, Liu N, Chen Y, Yang T, Qin S, Jiang Y, Huang X. Developing a CT-based radiomics nomogram for predicting post-acute pancreatitis diabetes mellitus incidence. Br J Radiol 2023;96:20230382. [Crossref] [PubMed]
- Petrov MS, Yadav D. Global epidemiology and holistic prevention of pancreatitis. Nat Rev Gastroenterol Hepatol 2019;16:175-84. [Crossref] [PubMed]
- Petrov MS, Basina M. DIAGNOSIS OF ENDOCRINE DISEASE: Diagnosing and classifying diabetes in diseases of the exocrine pancreas. Eur J Endocrinol 2021;184:R151-63. [Crossref] [PubMed]
- Banks PA, Bollen TL, Dervenis C, Gooszen HG, Johnson CD, Sarr MG, Tsiotos GG, Vege SSAcute Pancreatitis Classification Working Group. Classification of acute pancreatitis--2012: revision of the Atlanta classification and definitions by international consensus. Gut 2013;62:102-11. [Crossref] [PubMed]
- American Diabetes Association Professional Practice Committee. 2. Classification and Diagnosis of Diabetes: Standards of Medical Care in Diabetes-2022. Diabetes Care 2022;45:S17-S38. [Crossref] [PubMed]
- Dugic A, Hagström H, Dahlman I, Rutkowski W, Daou D, Kulinski P, Löhr JM, Vujasinovic M. Post-pancreatitis diabetes mellitus is common in chronic pancreatitis and is associated with adverse outcomes. United European Gastroenterol J 2023;11:79-91. [Crossref] [PubMed]
- De Waele JJ, Delrue L, Hoste EA, De Vos M, Duyck P, Colardyn FA. Extrapancreatic inflammation on abdominal computed tomography as an early predictor of disease severity in acute pancreatitis: evaluation of a new scoring system. Pancreas 2007;34:185-90. [Crossref] [PubMed]
- Alhussaini AJ, Steele JD, Nabi G. Comparative Analysis for the Distinction of Chromophobe Renal Cell Carcinoma from Renal Oncocytoma in Computed Tomography Imaging Using Machine Learning Radiomics Analysis. Cancers (Basel) 2022;14:3609. [Crossref] [PubMed]
- Tu X, Liu Q, Chen L, Li J, Yu X, Jiao X, Wang N, Hu L, Yuan Y, Gong W, Ding Y, Shi X, Xiao W, Lu G. Number of recurrences is significantly associated with the post-acute pancreatitis diabetes mellitus in a population with hypertriglyceridemic acute pancreatitis. Lipids Health Dis 2023;22:82. [Crossref] [PubMed]
- Singh A, Aggarwal M, Garg R, Stevens T, Chahal P. Post-pancreatitis diabetes mellitus: insight on optimal management with nutrition and lifestyle approaches. Ann Med 2022;54:1776-86. [Crossref] [PubMed]
- Bharmal SH, Cho J, Alarcon Ramos GC, Ko J, Stuart CE, Modesto AE, Singh RG, Petrov MS. Trajectories of glycaemia following acute pancreatitis: a prospective longitudinal cohort study with 24 months follow-up. J Gastroenterol 2020;55:775-88. [Crossref] [PubMed]
- Yu BJ, Li NS, He WH, He C, Wan JH, Zhu Y, Lu NH. Pancreatic necrosis and severity are independent risk factors for pancreatic endocrine insufficiency after acute pancreatitis: A long-term follow-up study. World J Gastroenterol 2020;26:3260-70. [Crossref] [PubMed]
- Jivanji CJ, Asrani VM, Windsor JA, Petrov MS. New-Onset Diabetes After Acute and Critical Illness: A Systematic Review. Mayo Clin Proc 2017;92:762-73. [Crossref] [PubMed]
- Zhi M, Zhu X, Lugea A, Waldron RT, Pandol SJ, Li L. Incidence of New Onset Diabetes Mellitus Secondary to Acute Pancreatitis: A Systematic Review and Meta-Analysis. Front Physiol 2019;10:637. [Crossref] [PubMed]
- Petrov MS. Panorama of mediators in postpancreatitis diabetes mellitus. Curr Opin Gastroenterol 2020;36:443-51. [Crossref] [PubMed]
- Gillies NA, Pendharkar SA, Singh RG, Asrani VM, Petrov MS. Lipid metabolism in patients with chronic hyperglycemia after an episode of acute pancreatitis. Diabetes Metab Syndr 2017;11:S233-41. [Crossref] [PubMed]
- Pendharkar SA, Singh RG, Petrov MS. Pro-inflammatory cytokine-induced lipolysis after an episode of acute pancreatitis. Arch Physiol Biochem 2018;124:401-9. [Crossref] [PubMed]
- Pendharkar SA, Asrani VM, Murphy R, Cutfield R, Windsor JA, Petrov MS. The Role of Gut-brain Axis in Regulating Glucose Metabolism After Acute Pancreatitis. Clin Transl Gastroenterol 2017;8:e210. [Crossref] [PubMed]
- Pendharkar SA, Walia M, Drury M, Petrov MS. Calcitonin gene-related peptide: neuroendocrine communication between the pancreas, gut, and brain in regulation of blood glucose. Ann Transl Med 2017;5:419. [Crossref] [PubMed]
- Larue RTHM, van Timmeren JE, de Jong EEC, Feliciani G, Leijenaar RTH, Schreurs WMJ, Sosef MN, Raat FHPJ, van der Zande FHR, Das M, van Elmpt W, Lambin P. Influence of gray level discretization on radiomic feature stability for different CT scanners, tube currents and slice thicknesses: a comprehensive phantom study. Acta Oncol 2017;56:1544-53. [Crossref] [PubMed]
- Shafiq-Ul-Hassan M, Zhang GG, Latifi K, Ullah G, Hunt DC, Balagurunathan Y, Abdalah MA, Schabath MB, Goldgof DG, Mackin D, Court LE, Gillies RJ, Moros EG. Intrinsic dependencies of CT radiomic features on voxel size and number of gray levels. Med Phys 2017;44:1050-62. [Crossref] [PubMed]
- Du Y, Cai M, Zha H, Chen B, Gu J, Zhang M, Liu W, Liu X, Liu X, Zong M, Li C. Ultrasound radiomics-based nomogram to predict lymphovascular invasion in invasive breast cancer: a multicenter, retrospective study. Eur Radiol 2024;34:136-48. [Crossref] [PubMed]
- Ouyang ZQ, He SN, Zeng YZ, Zhu Y, Ling BB, Sun XJ, Gu HY, He B, Han D, Lu Y. Contrast enhanced magnetic resonance imaging-based radiomics nomogram for preopera-tively predicting expression status of Ki-67 in meningioma: a two-center study. Quant Im-aging Med Surg 2023;13:1100-1114. [Crossref] [PubMed]
- Wongvorachan T, He S, Bulut O. A Comparison of Undersampling, Oversampling, and SMOTE Methods for Dealing with Imbalanced Classification in Educational Data Mining. Information 2023;14:54.
- Li Y, Huang X, Xia Y, Long L. Value of radiomics in differential diagnosis of chromophobe renal cell carcinoma and renal oncocytoma. Abdom Radiol (NY) 2020;45:3193-201. [Crossref] [PubMed]
- Zhong L, Shi L, Zhou L, Liu X, Gu L, Bai W. Development of a nomogram-based model combining intra- and peritumoral ultrasound radiomics with clinical features for differentiating benign from malignant in Breast Imaging Reporting and Data System category 3-5 nodules. Quant Imaging Med Surg 2023;13:6899-910. [Crossref] [PubMed]