Value of dual-layer spectral detector CT in predicting lymph node metastasis of non-small cell lung cancer
Introduction
Early detection and diagnosis can reduce lung cancer mortality rates, however, lung cancer remains the most common malignant tumor globally. As the main cause of cancer-related deaths worldwide, lung cancer places a huge burden on public health and the economy (1). Non-small cell lung cancer (NSCLC) is the major histological subtype of lung cancer, accounting for 80–85% of all lung cancers, and has a 5-year survival rate of only 23.6% (2). Lymph node metastasis (LNM) is the most common and major metastatic pathway in patients with NSCLC, approximately 16.6% of patients with stage-T1 NSCLC subsequently develop LNMs (3). According to the National Comprehensive Cancer Network Guidelines (4,5), surgery should be the first choice of treatment for early NSCLC, and radiotherapy and chemotherapy should be the first choice of treatment for advanced cases. For patients with resectable NSCLC, intraoperative radical mediastinal, hilar, and intrapulmonary lymph node dissection play key roles in improving tumor-free and overall survival rates. The accurate evaluation of LNM is an important factor in lung cancer staging, treatment, and prognosis (6).
Clinically, lymph nodes with a short axial diameter >10 mm measured using computed tomography (CT) were considered to be metastatic. Almeida et al. (7) studied this diagnostic criterion and found that their sensitivity and specificity were 51% and 85%, respectively, and the predicted results were unsatisfactory. As a non-invasive imaging technique, positron emission tomography/CT (PET/CT) is relatively accurate in diagnosing metastatic lymph nodes. More and more multidisciplinary lung cancer research teams recommended using PET-CT to assess LNM. However, false positives due to lymph node enlargement caused by infection, inflammation, or high metabolism, as well as expensive examination costs and large radiation exposure, limit the use of this examination. Thus, PET/CT has not been widely applied in clinical practice (8,9). Invasive methods, including thoracoscopic surgery and fiberoptic bronchoscopy-guided biopsy, are highly accurate. However, as they may lead to the risk of other complications, such as chest hematoma, infection, pneumothorax, or nerve damage, they are not the preferred diagnostic method for determining LNM (10).
Dual-layer spectral detector CT (SDCT) uses a multilayer detection system that eliminates the requirement for a predetermined energy CT. Compared with conventional CT, SDCT can provide multiple quantitative parameters of the essential characteristics of a substance. It can also provide more objective and accurate information on histological features and hemodynamics (11-13). Iodine concentration (IC), normalized iodine concentration (NIC), and the slope of the spectral curve (λ) can be used to quantify the concentration of the tissue contrast agent (iodine) associated with tumor blood supply and blood vessels. The effective atomic number (Zeff), electron density (ED), uric acid charts, and calcium suppression can be used to better reflect the composition and characteristics of the substances. Relevant studies have confirmed that SDCT is highly accurate in differentiating between benign and malignant tumors and in predicting LNM (14-16). However, previous studies on the SDCT prediction of LNM have mainly focused on whether the lymph nodes of various malignant tumors were metastatic by extracting quantitative information about the lymph nodes themselves (14-16). Unfortunately, it is difficult to achieve a one-to-one correspondence between SDCT and pathology in the selection and evaluation of lymph nodes in clinical practice, which often leads to deviations in analysis results. Thus, the prediction of LNM by analyzing the relevant features of the primary tumors instead of the lymph nodes has attracted increasing attention and has proven effective in some malignant tumor lymph node studies (17-21). There are few reports on predicting LNM by exploring the primary cancer foci of NSCLC using SDCT. Comprehensive evaluation systems involving multiple combinations of lesion morphology, clinical hematological indicators, and spectral quantitative parameters in NSCLC are rare.
Thus, this study aimed to explore the importance of SDCT quantitative parameters and their derivatives, combined with morphological information of the lesion and the clinical data of patients, in predicting LNM in NSCLC. We also established a predictive model for LNM to help develop the best treatment strategy.
Methods
Study population
The study was approved by the Institutional Ethics Committee of Jiangsu Cancer Hospital, and the requirement for individual consent for this retrospective analysis was waived. The study was conducted in accordance with the Declaration of Helsinki (as revised in 2013). A retrospective analysis was performed using the data of patients admitted to our hospital from September 2021 to November 2022 who underwent radical surgery and systematic lymph node dissection. To be eligible for inclusion in this study, the patients had to meet the following inclusion criteria: (I) present with solid solitary pulmonary nodules (SPNs), 8 mm < diameter ≤30 mm; (II) have undergone a SDCT chest-enhanced scan at our hospital; and (III) have NSCLC confirmed by postoperative pathology. Patients were excluded from the study if they met any of the following exclusion criteria: (I) had multiple primary lesions in the lung (n=3); (II) had received preoperative chemoradiotherapy or other anti-tumor therapy (n=7); (III) had incomplete clinical examination data (including clinical data, tumor index detection data, and spectral enhanced CT image data within 1 month before surgery) (n=15); and/or (IV) had images of suboptimal quality and/or images that were difficult to analyze (n=2). Ultimately, 91 patients were included in this study. They were divided into the non-LNM group (n=57) and the LNM group (n=34) according to the pathological findings for the lymph nodes. A flowchart of the specific inclusion and exclusion criteria is presented in Figure 1. Data of the clinicopathological characteristics, including age, sex, smoking status, tumor markers, and pathological type, of all the patients were collected.
SDCT image acquisition
The patients underwent SDCT (IQon; Philips Healthcare, Best, The Netherlands), following the same routine scanning protocol as that used for chest enhancement. All the patients were placed in the supine position, and the scan range was from the thoracic inlet to the costophrenic angle. The scanning parameters were as follows: tube voltage: 120 kVp; tube current modulation: three-dimensional; matrix: 512×512; collimator width: 64×0.625 mm; scan field of view: 372 mm; spacing: 0.90; and rotation time: 0.50 seconds. The thickness of the scanning layer was 5 mm, and that of the reconstruction layer was 1 mm. Contrast agent (iodofol: 1.0–1.5 mL/kg, iodine: 350 mg/mL; Jiangsu Hengrui Medicine, Lianyungang, China) was injected in the antecubital vein at a flow rate of 2.5–3.0 mL/s, and normal saline (20 mL) was then injected at the same flow rate. After the injection, the chest enhancement scan was delayed by 50 seconds.
Radiological analysis of the morphological characteristics on SDCT
Images of all the enrolled patients with NSCLC were interpreted and analyzed jointly by two diagnostic radiologists (A with four years of experience, and B with eight years of experience). Both physicians were unaware of the pathological results. When analyzing the morphological characteristics of the lesions, they were fully combined with thin-layer scanning and multi-planar reconstruction techniques to interpret the signs, such as lobulation, burr, pleural indentation, vessel convergence, vacuoles, and cavities (22). If any disagreements arose between the physicians, a consensus was reached by discussion.
Acquisition and analysis of SDCT quantitative parameters
All the images were uploaded to the IntelliSpace Portal (Philips Healthcare, Best, The Netherlands) for analysis and processing using a Spectral CT Viewer (Philips Healthcare) that was provided with the workstation. The image analysis was performed by a radiologist (A) and supervised by a senior radiologist (B). The image data of all the patients were obtained via surgery in the mediastinal window. After the lesions were found in the film reading, the region of interest (ROI) was manually delineated at three consecutive levels, including the largest lesion level and its adjacent upper and lower levels. Areas of calcification, blood vessels, voids, atelectasis, and necrosis were avoided, as these may affect the measurements. The average of three measurements for each patient was used as the final analytical data. The ROI of the aorta with similar layers was obtained using the same method for standardizing the SDCT quantitative parameters. Subsequently, a series of parameters was obtained.
The following values were recorded in our study: The CT values of the lesions at virtual non-contrast (VNC) and enhancement (40 and 70 keV), which were recorded as CTSPN-VNC, CTSPN-40keV, and CTSPN-70keV, respectively; and the CT values of the aorta at VNC (CTaorta-VNC) and enhancement at 70 keV (CTaorta), which were recorded and used as references. The following formulas were used to calculate the lesion to aorta virtual plain scan ratio (SARVNC), the lesion to aorta enhancement ratios (SAR40keV and SAR70keV), the differences between the lesion enhancement and virtual plain scan CT value (Δ40keV and Δ70keV), the contrast enhancement ratios (CER40keV and CER70keV), the standardized reinforcement scores (NEF40keV and NEF70keV), and λ (23,24):
The IC (mg/mL), Z values, and ED values were normalized to the aorta to account for hemodynamic changes between the patients. The NIC, normalized electron density (NED), and normalized Z (NZeff) were calculated using the following formulas:
To assess interobserver repeatability and variability, 50.5% (46/91) of the study participants were randomly selected, and the previous measurement procedure was repeated by another radiologist (C, with 14 years of experience in radiology). Interobserver agreement was assessed using Bland-Altman plots and intraclass correlation coefficients (ICCs).
Statistical analysis
All the data were statistically analyzed using SPSS 22 (SPSS, Inc., Chicago, IL, USA) and MedCalc15 (MedCalc Software, Mariakerke, Belgium). The continuous variables are expressed as the mean ± standard deviation. The chi-square test or Fisher’s exact test was used to analyze the differences in the basic clinical data and the morphological characteristics of the primary cancer lesions between the non-LNM and LNM groups. The Kolmogorov-Smirnov method was used to test the normality of tumor markers, the SDCT quantitative parameters of the primary cancer lesions, and the derived parameters. Levene’s test was used to test the homogeneity of variance. The differences between the multiple SDCT quantitative parameters and their derived quantitative parameters between the non-LNM and LNM groups were analyzed using the Mann-Whitney U and independent samples t-tests, and a false discovery rate (FDR) correction for multiple tests was used in the univariate analysis. The diagnostic performance of LNM in NSCLC patients was evaluated by plotting the receiver operating characteristic (ROC) curves for the corresponding parameters. To identify the independent predictive factors of LNM in NSCLC patients and establish the best multi-parameter regression prediction model, we subjected the following factors to a multi-factor logistic regression analysis: the statistically significant different clinical data, tumor indicators, morphological signs of primary cancer lesions on SDCT, and each quantitative parameter of SDCT, and its derived parameters. Interobserver agreement for the SDCT-related parameters was assessed using Bland-Altman plots and ICCs (an ICC of 0.000–0.200 was considered poor, 0.201–0.400 was considered fair, 0.401–0.600 was considered moderate, 0.601–0.800 was considered good; and 0.801–1.000 was considered excellent).
Results
Statistical analysis of the basic clinical data
A total of 91 patients presenting with solid SPNs who underwent radical surgery and systematic lymphatic dissection were included in this study. In the non-LNM group (n=57, 29 males and 28 females), the patients had a mean age of 65.667±9.238 years, and 26 patients had a smoking history. In the LNM group (n=34, 20 males and 14 females), the patients had a mean age of 62.647±8.131 years, and 17 patients had a smoking history. We analyzed the differences between the two groups in terms of age, sex, and smoking history, but no statistically significant differences were found (P=0.119, 0.462, and 0.685, respectively) (Table 1).
Table 1
Characteristics | Non-LNM group (n=57) | LNM group (n=34) | P value |
---|---|---|---|
Age (years), mean ± SEM | 65.667±9.238 | 62.647±8.131 | 0.119 |
Sex, N | 0.462 | ||
Male | 29 | 20 | |
Female | 28 | 14 | |
Smoking, N | 0.685 | ||
Yes | 26 | 17 | |
No | 31 | 17 |
The P values were obtained by the independent t-test the chi-square test. P<0.05 indicates statistical significance. LNM, lymph node metastasis; SEM, standard error of the mean.
Statistical analysis of the SDCT imaging signs
Table 2 shows the variability in interpreting the morphological features of the primary lesions on SDCT between the non-LNM and LNM groups of NSCLC patients. We analyzed the CT presentation of the primary cancer lesions in the two groups in relation to the following 10 aspects: location, size, lesion morphology, lobulation sign, short burr sign, pleural indentation sign, vessel convergence sign, air bronchogram sign, vacuole sign, and calcification. There were significant differences between the two groups in terms of the lesion size and vessel convergence sign (P<0.001 and P=0.025, respectively), which were more obvious in the LNM group than in the non-LNM group. There were no statistically significant differences between the non-LNM and LNM groups in terms of the location, morphology, short burr sign, lobulation sign, pleural indentation sign, air bronchogram sign, vacuole sign, and calcification (P>0.05).
Table 2
Characteristics | Non-LNM group (n=57) | LNM group (n=34) | P value |
---|---|---|---|
Location | 0.641 | ||
Upper left | 12 | 6 | |
Lower left | 13 | 8 | |
Upper right | 18 | 9 | |
Middle right | 2 | 4 | |
Lower right | 12 | 7 | |
Size (mm), mean ± SEM | 17.351±6.175 | 22.697±4.314 | <0.001 |
Lesion morphology | 0.311 | ||
Round-like | 8 | 2 | |
Irregular | 49 | 32 | |
Lobulation sign | 0.143 | ||
Yes | 41 | 29 | |
No | 16 | 5 | |
Short burr sign | 0.139 | ||
Yes | 35 | 26 | |
No | 22 | 8 | |
Pleural indentation sign | 0.183 | ||
Yes | 27 | 21 | |
No | 30 | 13 | |
Vessel convergence sign | 0.025 | ||
Yes | 32 | 27 | |
No | 25 | 7 | |
Air bronchogram sign | 0.248 | ||
Yes | 20 | 8 | |
No | 37 | 26 | |
Vacuole sign | 0.353 | ||
Yes | 6 | 6 | |
No | 51 | 28 | |
Calcification | >0.99 | ||
Yes | 4 | 3 | |
No | 53 | 31 |
The P values were obtained by the chi-square test and Mann-Whitney U-test. P<0.05 indicates statistical significance. SDCT, dual-layer spectral detector computed tomography; LNM, lymph node metastasis; SEM, standard error of the mean.
Analysis of the tumor markers in the non-LNM and LNM groups
The following six common tumor indicators between the non-LNM and LNM groups were collected and compared: carcinoembryonic antigen (CEA), carbohydrate antigen 724 (CA724), CA125, CA199, α-fetoprotein (AFP), and neuron-specific enolase (NSE). There were no statistically significant differences in the tumor markers between the two groups (P>0.05) (Table 3).
Table 3
Parameters | Non-LNM group (n=57) | LNM group (n=34) | P value |
---|---|---|---|
CEA (ng/mL) | 3.634±3.493 | 8.146±11.665 | 0.080 |
CA724 (U/mL) | 3.244±1.447 | 2.846±1.650 | 0.061 |
CA125 (U/mL) | 25.217±19.987 | 32.760±39.093 | 0.694 |
CA199 (U/mL) | 12.781±7.271 | 10.162±6.069 | 0.103 |
AFP (ng/mL) | 2.596±1.421 | 2.734±1.041 | 0.103 |
NSE (ng/mL) | 12.459±2.929 | 13.529±4.889 | 0.297 |
The data are presented as the mean ± standard deviation. The P values were obtained by the Mann-Whitney U-test. P<0.05 indicates statistical significance. LNM, lymph node metastasis; CEA, carcinoembryonic antigen; CA, carbohydrate antigen; AFP, α-fetoprotein; NSE, neuron-specific enolase.
Comparison of the SDCT quantitative parameters between the non-LNM and LNM groups
The acquisition of the quantitative parameters of the primary lesions and the outlines of the ROIs between the two groups are shown schematically in Figure 2 and Figure 3, respectively. The SDCT quantitative parameters and their derived quantitative parameters were obtained using ROI delineation and normalized uniformly. A total of 13 quantitative and derived quantitative indicators were included in the statistical analysis. Table 4 sets out the analysis results of the differences in the quantitative parameters related to the primary lesions in the two groups. In total, 11 quantitative parameters (i.e., SAR40keV, SAR70keV, Δ40keV, Δ70keV, CER40keV, CER70keV, NEF40keV, NEF70keV, λ, NIC, and NZeff) were significantly higher in the non-LNM group than the LNM group (P<0.05). However, no significant differences between the two groups were observed in relation to the other two quantitative parameters; that is, SARVNC and NED (P=0.258 and 0.223, respectively).
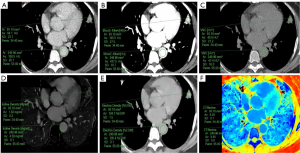
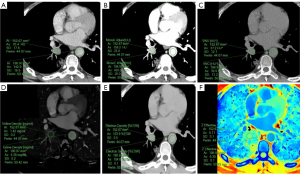
Table 4
Parameters | Non-LNM group (n=57) | LNM group (n=34) | P value |
---|---|---|---|
SARVNC | 0.772±0.264 | 0.829±0.173 | 0.258 |
SAR40keV | 1.064±0.356 | 0.790±0.234 | <0.001 |
SAR70keV | 0.438±0.159 | 0.363±0.097 | 0.018 |
Δ40keV | 177.556±47.277 | 128.839±44.173 | <0.001 |
Δ70keV | 52.715±14.310 | 38.683±14.808 | <0.001 |
CER40keV | 6.230±3.862 | 3.576±1.238 | <0.001 |
CER70keV | 1.858±1.163 | 1.073±0.407 | <0.001 |
NEF40keV | 1.189±0.542 | 0.789±0.283 | <0.001 |
NEF70keV | 0.352±0.158 | 0.236±0.089 | <0.001 |
λ | 4.159±1.107 | 3.004±1.003 | <0.001 |
NIC | 0.340±0.112 | 0.230±0.082 | <0.001 |
NED | 0.974±0.061 | 0.984±0.009 | 0.223 |
NZeff | 0.855±0.149 | 0.802±0.048 | 0.004 |
The data are presented as the mean ± standard deviation. The P values were obtained by the independent t-test or Mann-Whitney U-test. P<0.05 indicates statistical significance. SDCT, dual-layer spectral detector computed tomography; LNM, lymph node metastasis; SARVNC, solitary pulmonary nodule at VNC to arterial VNC ratio; VNC, virtual non-contrast; SAR40keV/70keV, solitary pulmonary nodule in the 40 keV/70 keV to arterial enhancement ratio; Δ40keV/70keV, attenuation difference of the solitary pulmonary nodule between the 40 keV/70 keV and VNC; CER, contrast enhancement ratio; NEF, normalized arterial enhancement fraction; λ, slope of the spectral attenuation curve; NIC, normalized iodine concentration; NED, normalized electron density; NZeff, normalized effective atomic number.
Diagnostic performance of the SDCT quantitative parameters in LNM prediction
The diagnostic efficacy of the quantitative parameters associated with SDCT in predicting LNM in patients with NSCLC that differed significantly between the non-LNM and LNM groups are detailed in Table 5. The statistical analysis showed that the three quantitative SDCT parameters with the highest diagnostic efficacy in distinguishing between LNM and non-LNM cases were CER40keV, NIC, and CER70keV [areas under the curve (AUCs) =0.794, 0.791, and 0.783, respectively] (Figure 4); the highest of which was CER40keV. When the CER40keV cut-off value was 3.752, it had a sensitivity of 64.7%, a specificity of 82.5%, a positive prediction rate (PPR) of 68.8%, a negative prediction rate (NPR) of 79.7%, and an accuracy of 75.0%. When the NIC cut-off value was 0.266, it had a sensitivity of 79.4%, a specificity of 71.9%, a PPR of 62.8%, a NPR of 85.4%, and an accuracy of 73.9%. When the CER70keV cut-off value was 1.117, it had a sensitivity of 64.7%, a specificity of 82.5%, a PPR of 68.8%, a NPR of 79.7%, and an accuracy of 75.0%.
Table 5
Parameters | AUC | Cut-off value | Sensitivity (%) | Specificity (%) | PPR (%) | NPR (%) | Accuracy (%) | P value |
---|---|---|---|---|---|---|---|---|
SAR40keV | 0.741 | 0.761 | 55.9 | 80.7 | 62.1 | 74.2 | 69.6 | <0.001 |
SAR70keV | 0.649 | 0.278 | 26.5 | 98.2 | 88.9 | 68.3 | 69.6 | 0.014 |
Δ40keV | 0.772 | 150.567 | 70.6 | 77.2 | 64.9 | 81.5 | 73.9 | <0.001 |
Δ70keV | 0.761 | 45.933 | 76.5 | 70.2 | 59.5 | 81.6 | 70.7 | <0.001 |
CER40keV | 0.794 | 3.752 | 64.7 | 82.5 | 68.8 | 79.7 | 75.0 | <0.001 |
CER70keV | 0.783 | 1.117 | 64.7 | 82.5 | 68.8 | 79.7 | 75.0 | <0.001 |
NEF40keV | 0.759 | 0.739 | 55.9 | 86.0 | 70.4 | 76.6 | 73.9 | <0.001 |
NEF70keV | 0.755 | 0.220 | 55.9 | 89.5 | 76.0 | 77.3 | 76.1 | <0.001 |
λ | 0.777 | 3.523 | 70.6 | 77.2 | 63.9 | 80.0 | 72.8 | <0.001 |
NIC | 0.791 | 0.266 | 79.4 | 71.9 | 62.8 | 85.4 | 73.9 | <0.001 |
NZeff | 0.680 | 0.814 | 70.6 | 64.9 | 53.5 | 77.1 | 65.2 | 0.0014 |
Receiver operating characteristic curve analysis. P<0.05 indicates statistical significance. SDCT, dual-layer spectral detector computed tomography; LNM, lymph node metastasis; AUC, area under the curve; PPR, positive prediction rate; NPR, negative prediction rate; SAR40keV/70keV, solitary pulmonary nodule in the 40 keV/70 keV to arterial enhancement ratio; Δ40keV/70keV, attenuation difference of the solitary pulmonary nodule between the 40 keV/70 keV and VNC; CER, contrast enhancement ratio; NEF, normalized arterial enhancement fraction; λ, slope of the spectral attenuation curve; NIC, normalized iodine concentration; NZeff, normalized effective atomic number.
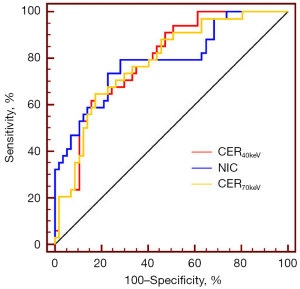
Combining the morphological features and SDCT quantitative parameters to predict LNM
A binary logistic regression analysis was performed in which the factors that differed significantly between the non-LNM and LNM groups, including the lesion size, vessel convergence sign, and 11 quantitative parameters (i.e., SAR40keV, SAR70keV, Δ40keV, Δ70keV, CER40keV, CER70keV, NEF40keV, NEF70keV, λ, NIC, and NZeff) were the independent variables, and LNM was the dependent variable. The results showed that size [odds ratio (OR): 1.157, 95% confidence interval (CI): 1.046–1.281, P=0.005], λ (OR: 0.473, 95% CI: 0.253–0.882, P=0.018), and NIC (OR: 0.396, 95% CI: 0.195–0.802, P=0.010) were independent predictive factors of LNM. The ROC curve analysis showed that the AUCs of size, λ, NIC, and their combination in diagnosing LNM were 0.748, 0.777, 0.791, and 0.892, respectively. The AUC of their combination (size + λ + NIC) was the largest (0.892). When the cut-off value was 0.292, the sensitivity, specificity, PPR, NPR, and accuracy were 82.4%, 84.2%, 73.7%, 88.7%, and 81.5%, respectively. The probability prediction model was expressed as follows:
The detailed data mentioned above are presented in Table 6 and Figure 5, respectively.
Table 6
Parameters | AUC | Cut-off value | Sensitivity (%) | Specificity (%) | PPR (%) | NPR (%) | Accuracy (%) | P value |
---|---|---|---|---|---|---|---|---|
Size | 0.748 | 16.500 | 88.2 | 56.1 | 54.5 | 88.9 | 67.4 | <0.001 |
λ | 0.777 | 3.523 | 70.6 | 77.2 | 63.9 | 80.0 | 72.8 | <0.001 |
NIC | 0.791 | 0.266 | 79.4 | 71.9 | 62.8 | 85.4 | 73.9 | <0.001 |
Combination model | 0.892 | 0.292 | 82.4 | 84.2 | 73.7 | 88.7 | 81.5 | <0.001 |
Multi-factor logistic regression analysis. P<0.05 indicates statistical significance. Combination model, the combination of size + λ + NIC. AUC, area under the curve; PPR, positive prediction rate; NPR, negative prediction rate; λ, slope of the spectral attenuation curve; NIC, normalized iodine concentration.
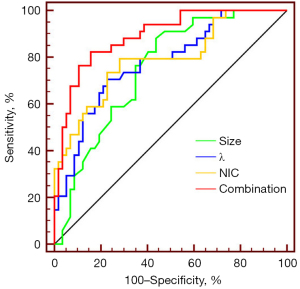
Interobserver consistency assessment
We randomly selected 50.5% (46/91) of the study participants for assessment, and the previous SDCT-related quantitative parameter measurement and acquisition process was repeated by radiologist C. The ICC values ranged from 0.801–0.935, indicating that the SDCT quantitative parameters and their related derived quantitative parameters had excellent interobserver agreement (Table 7). The Bland-Altman plots showed that all or a large proportion of the measurements were within the 95% CI, indicating excellent interobserver agreement in the assessment (Figure 6).
Table 7
Parameters | ICC (95% CI) |
---|---|
SARVNC | 0.920 (0.860–0.955) |
SAR40keV | 0.887 (0.805–0.936) |
SAR70keV | 0.935 (0.885–0.963) |
Δ40keV | 0.847 (0.740–0.912) |
Δ70keV | 0.824 (0.703–0.899) |
CER40keV | 0.819 (0.695–0.895) |
CER70keV | 0.801 (0.667–0.885) |
NEF40keV | 0.901 (0.828–0.944) |
NEF70keV | 0.914 (0.849–0.951) |
λ | 0.833 (0.717–0.904) |
NIC | 0.873 (0.782–0.928) |
NED | 0.928 (0.874–0.960) |
NZeff | 0.899 (0.825–0.943) |
ICC, intraclass correlation coefficient; SDCT, dual-layer spectral detector computed tomography; CI, confidence interval; SARVNC, solitary pulmonary nodule at VNC to arterial VNC ratio; VNC, virtual non-contrast; SAR40keV/70keV, solitary pulmonary nodule in the 40 keV/70 keV to arterial enhancement ratio; Δ40keV/70keV, attenuation difference of the solitary pulmonary nodule between the 40 keV/70 keV and VNC; CER, contrast enhancement ratio; NEF, normalized arterial enhancement fraction; λ, slope of the spectral attenuation curve; NIC, normalized iodine concentration; NED, normalized electron density; NZeff, normalized effective atomic number.
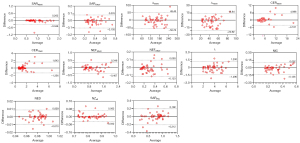
Discussion
LNM is the most common form of metastasis in NSCLC, and the presence or absence of LNM is of great significance in determining the surgical modality, disease stage, and patient prognosis (25). It is difficult to achieve a one-to-one correspondence between SDCT and pathology in the selection and evaluation of lymph nodes in clinical practice, which often leads to inconsistent results. Consequently, many studies have sought to obtain relatively stable information by analyzing and extracting the relevant features of primary tumors to predict LNM risk or metastasis (17-21).
In this study, a morphological analysis was performed on the following 10 SDCT signs of primary lesions: location, size, morphology, lobulation sign, short burr sign, pleural indentation sign, vessel convergence sign, air bronchogram sign, vacuole sign, and calcification. Significant differences in the lesion size and vessel convergence sign were observed between the non-LNM and LNM groups. The diameter of the primary lesion of NSCLC was larger in the LNM group than the non-LNM group (22.697 vs. 17.351 mm). This might be due to the relatively higher malignancy and faster growth of the primary lesions in the LNM group (26). Our study also showed that the size of the primary NSCLC lesions could be used as an independent risk factor in predicting LNM and had an AUC of 0.748. The proportion of vessel convergence signs was significantly higher in the LNM group than the non-LNM group [27/34 (79.41%) vs. 32/57 (56.14%), respectively], which suggests that malignant lesions require more nutrients to grow and also exhibit stronger metastatic properties (27).
Tumor markers are important in detecting malignant tumors for indications, prognostic monitoring, recurrence, and metastasis (28,29). We analyzed the differences in six tumor markers (i.e., CEA, CA724, CA125, CA199, AFP, and NSE) between the non-LNM and LNM groups. There were no statistically significant differences in these tumor indicators between the two groups. The included patients had solid SPNs (8 mm < diameter ≤30 mm), and the lesions in the non-LNM and LNM groups were relatively small (22.697 vs. 17.351 mm, respectively). Notably, CEA, CA724, CA125, CA199, and AFP are often highly correlated with digestive system tumors and have a weak role in lung cancer. NSE is the preferred marker for detecting SCLC (30,31) and was also found to be weakly associated with NSCLC in this study. Cytokeratin 211 and squamous cell carcinoma antigen are considered the most valuable serum markers for NSCLC. However, as not all the patients were examined before surgery, the correlation between these two tumor markers and LNM could not be further examined in the present study.
SDCT can be used to obtain various energy spectrum parameter images in addition to traditional CT images. It provides valuable information for detecting lesions, accurately determining the range of lesions, differentiating between benign and malignant lesions, and determining the likelihood of recurrence and metastasis. NIC and λ have been widely used to diagnose and predict LNM in oral, rectal, and thyroid cancers (14,19,32-34). NIC is a relatively stable parameter and an objective and quantitative reflection of tumor iodinated contrast intake, which is proportional to the tumor vessel density. In the present study, the NIC of the primary cancer lesions was lower in the LNM group than the non-LNM group, which is consistent with the findings of previous studies (14,33,35-37). This result may be due to the higher malignancy of the lesions in the LNM group, the invasion and destruction of adjacent blood vessels, and the immaturity and low functional efficiency of tumor neovascularization. Thus, poor blood supply leads to reduced iodine content in a tumor, while some internal tumor necrosis is also responsible for the absence of significant contrast enhancement. The λ values reflect the characteristics of the CT value of each tissue changing with X-ray energy. In this study, the λ values obtained from the lesions in the LNM group were significantly lower than those obtained from the non-LNM group, as λ also primarily characterizes the iodine content in the tissue; this trend is similar to that of NIC. Additionally, this study found that NIC and λ were independent predictive factors of LNM in NSCLC. Their diagnostic efficacies were 0.791 and 0.777, respectively, which further demonstrates the stability of these two quantitative parameters.
In addition, in examining which SDCT multi-parameters can be used to predict LNM in NSCLC, this study showed that NZeff had diagnostic value. The NZeff value of the lesions in the non-LNM group was slightly higher than that in the LNM group (0.855 vs. 0.802, respectively), which has not been reported in previous studies and may provide new ideas for predicting LNM in lung cancer. Nagano et al. (38) studied mediastinal LNM in NSCLC and showed that the ED of the metastatic lymph nodes was significantly lower than that of the non-metastatic lymph nodes. The present study found no significant difference in the NED between the LNM and non-LNM groups. Our study differs from Nagano et al.’s in a number of respects. First, the study populations differ. We used the primary lesions (solid SPNs) as the ROIs rather than the lymph nodes. Second, the NED was normalized to the aorta rather than the original value. Our study also found that 11 SDCT value-related derived parameters (i.e., SAR40keV, SAR70keV, Δ40keV, Δ70keV, CER 40keV, CER70keV, NEF40keV, NEF70keV, λ, NIC, and NZeff) differed significantly between the two groups. However, no statistically significant differences were observed between the two groups in terms of the two quantitative parameters; that is, SARVNC and NED (P=0.258 and 0.223, respectively). This suggests that the parameters associated with SDCT values can also reflect tumor information and provide a reference for clinical diagnosis. Similarly, Ma et al. (23) showed that parameters related to CT values, such as the CER, enhancement value in the venous phase, and enhancement value in the delayed phase, can be used as alternative markers for preoperatively detecting lymphovascular invasion in advanced gastric cancer. Liu et al. (39) also achieved good results in predicting serosal invasion in gastric cancer using CT value-related parameters, such as arterial phase, venous phase, and delayed phase enhancement values.
Unlike previous studies that focused solely on the value of SDCT multi-parameters in predicting LNM, this study also incorporated patients’ existing examination data. We explored the available resources to construct the most superior model for predicting LNM. The results showed that lesion size, λ, and NIC were independent predictive factors of LNM in NSCLC. Among all the independent predictive factors, NIC had the best diagnostic efficacy for LNM (AUC =0.791). However, the combined prediction model of NIC, λ, and lesion size was more effective than any single parameter alone (AUC =0.892). This study provides a new technique for predicting LNM in patients with NSCLC.
This study had several limitations. First, in this study, the patients had pathological diagnoses of NSCLC and solid SPNs of 8 mm < diameter ≤30 mm, and patients with masses larger than 30 mm were not included in the study. Second, the study used retrospective data collected from a single center; to improve the reliability of evidence, we would need a larger sample size and the acquisition parameters of multi-institution CT scanners. Third, while tumor indicators have been used for disease diagnosis and LNM prediction (39-41), there was no significant difference between the two groups in this study. This may be related to the small sample size and the lack of a comprehensive collection of tumor indicators. This issue will be addressed in subsequent multicenter experiments with larger sample sizes. Fourth, deep-learning methods have been extensively used in various prediction and classification tasks related to various types of diseases, including breast, lung, and melanoma cancers (42-44), and related research needs to be conducted to extract more useful and comprehensive information to assist in cancer diagnosis and treatment.
Conclusions
In conclusion, we found that the SDCT quantitative parameters combined with the clinical data showed promise in predicting LNM in NSCLC and had satisfactory diagnostic performance and reproducibility.
Acknowledgments
Funding: This work was supported by
Footnote
Conflicts of Interest: All authors have completed the ICMJE uniform disclosure form (available at https://qims.amegroups.com/article/view/10.21037/qims-23-447/coif). The authors have no conflicts of interest to declare.
Ethical Statement: The authors are accountable for all aspects of the work in ensuring that questions related to the accuracy or integrity of any part of the work are appropriately investigated and resolved. The study was conducted in accordance with the Declaration of Helsinki (as revised in 2013). The study was approved by the Institutional Ethics Committee of Jiangsu Cancer Hospital, and the requirement of individual consent for this retrospective analysis was waived.
Open Access Statement: This is an Open Access article distributed in accordance with the Creative Commons Attribution-NonCommercial-NoDerivs 4.0 International License (CC BY-NC-ND 4.0), which permits the non-commercial replication and distribution of the article with the strict proviso that no changes or edits are made and the original work is properly cited (including links to both the formal publication through the relevant DOI and the license). See: https://creativecommons.org/licenses/by-nc-nd/4.0/.
References
- Sung H, Ferlay J, Siegel RL, Laversanne M, Soerjomataram I, Jemal A, Bray F. Global Cancer Statistics 2020: GLOBOCAN Estimates of Incidence and Mortality Worldwide for 36 Cancers in 185 Countries. CA Cancer J Clin 2021;71:209-49. [Crossref] [PubMed]
- Nasim F, Sabath BF, Eapen GA. Lung Cancer. Med Clin North Am 2019;103:463-73. [Crossref] [PubMed]
- Chen B, Wang X, Yu X, Xia WJ, Zhao H, Li XF, Liu LX, Liu Y, Hu J, Fu XN, Li Y, Xu YJ, Liu DR, Yang HY, Xu L, Jiang F. Lymph node metastasis in Chinese patients with clinical T1 non-small cell lung cancer: A multicenter real-world observational study. Thorac Cancer 2019;10:533-42. [Crossref] [PubMed]
- Ettinger DS, Wood DE, Aisner DL, Akerley W, Bauman J, Chirieac LR, et al. Non-Small Cell Lung Cancer, Version 5.2017, NCCN Clinical Practice Guidelines in Oncology. J Natl Compr Canc Netw 2017;15:504-35. [Crossref] [PubMed]
- Ettinger DS, Wood DE, Aggarwal C, Aisner DL, Akerley W, Bauman JR, et al. NCCN Guidelines Insights: Non-Small Cell Lung Cancer, Version 1.2020. J Natl Compr Canc Netw 2019;17:1464-72. [Crossref] [PubMed]
- Ma X, Xia L, Chen J, Wan W, Zhou W. Development and validation of a deep learning signature for predicting lymph node metastasis in lung adenocarcinoma: comparison with radiomics signature and clinical-semantic model. Eur Radiol 2023;33:1949-62. [Crossref] [PubMed]
- Almeida FA, Uzbeck M, Ost D. Initial evaluation of the nonsmall cell lung cancer patient: diagnosis and staging. Curr Opin Pulm Med 2010;16:307-14. [Crossref] [PubMed]
- Kandathil A, Kay FU, Butt YM, Wachsmann JW, Subramaniam RM. Role of FDG PET/CT in the Eighth Edition of TNM Staging of Non-Small Cell Lung Cancer. Radiographics 2018;38:2134-49.
- Pak K, Park S, Cheon GJ, Kang KW, Kim IJ, Lee DS, Kim EE, Chung JK. Update on nodal staging in non-small cell lung cancer with integrated positron emission tomography/computed tomography: a meta-analysis. Ann Nucl Med 2015;29:409-19. [Crossref] [PubMed]
- Reck M, Rabe KF. Precision Diagnosis and Treatment for Advanced Non-Small-Cell Lung Cancer. N Engl J Med 2017;377:849-61. [Crossref] [PubMed]
- Wang P, Tang Z, Xiao Z, Hong R, Wang R, Wang Y, Zhan Y. Dual-energy CT in differentiating benign sinonasal lesions from malignant ones: comparison with simulated single-energy CT, conventional MRI, and DWI. Eur Radiol 2022;32:1095-105. [Crossref] [PubMed]
- Kang HJ, Kim SH, Bae JS, Jeon SK, Han JK. Can quantitative iodine parameters on DECT replace perfusion CT parameters in colorectal cancers? Eur Radiol 2018;28:4775-82. [Crossref] [PubMed]
- Gordic S, Puippe GD, Krauss B, Klotz E, Desbiolles L, Lesurtel M, Müllhaupt B, Pfammatter T, Alkadhi H. Correlation between Dual-Energy and Perfusion CT in Patients with Hepatocellular Carcinoma. Radiology 2016;280:78-87. [Crossref] [PubMed]
- Luo YH, Mei XL, Liu QR, Jiang B, Zhang S, Zhang K, Wu X, Luo YM, Li YJ. Diagnosing cervical lymph node metastasis in oral squamous cell carcinoma based on third-generation dual-source, dual-energy computed tomography. Eur Radiol 2023;33:162-71. [Crossref] [PubMed]
- Su GY, Xu XQ, Zhou Y, Zhang H, Si Y, Shen MP, Wu FY. Texture analysis of dual-phase contrast-enhanced CT in the diagnosis of cervical lymph node metastasis in patients with papillary thyroid cancer. Acta Radiol 2021;62:890-6. [Crossref] [PubMed]
- Gao L, Lu X, Wen Q, Hou Y. Added value of spectral parameters for the assessment of lymph node metastasis of lung cancer with dual-layer spectral detector computed tomography. Quant Imaging Med Surg 2021;11:2622-33. [Crossref] [PubMed]
- Li F, Huang F, Liu C, Pan D, Tang X, Wen Y, Chen Z, Qin Y, Chen J. Parameters of dual-energy CT for the differential diagnosis of thyroid nodules and the indirect prediction of lymph node metastasis in thyroid carcinoma: a retrospective diagnostic study. Gland Surg 2022;11:913-26. [Crossref] [PubMed]
- Yang X, Hu H, Zhang F, Li D, Yang Z, Shi G, Lu G, Jiang Y, Yang L, Wang Y, Duan X, Shen J. Preoperative Prediction of the Aggressiveness of Oral Tongue Squamous Cell Carcinoma with Quantitative Parameters from Dual-Energy Computed Tomography. Front Oncol 2022;12:904471. [Crossref] [PubMed]
- Zou Y, Zhang H, Li W, Guo Y, Sun F, Shi Y, Gong Y, Lu X, Wang W, Xia S. Prediction of ipsilateral lateral cervical lymph node metastasis in papillary thyroid carcinoma: a combined dual-energy CT and thyroid function indicators study. BMC Cancer 2021;21:221. [Crossref] [PubMed]
- Cong M, Feng H, Ren JL, Xu Q, Cong L, Hou Z, Wang YY, Shi G. Development of a predictive radiomics model for lymph node metastases in pre-surgical CT-based stage IA non-small cell lung cancer. Lung Cancer 2020;139:73-9. [Crossref] [PubMed]
- Chen W, Xu M, Sun Y, Ji C, Chen L, Liu S, Zhou K, Zhou Z. Integrative Predictive Models of Computed Tomography Texture Parameters and Hematological Parameters for Lymph Node Metastasis in Lung Adenocarcinomas. J Comput Assist Tomogr 2022;46:315-24. [Crossref] [PubMed]
- Austin JH, Müller NL, Friedman PJ, Hansell DM, Naidich DP, Remy-Jardin M, Webb WR, Zerhouni EA. Glossary of terms for CT of the lungs: recommendations of the Nomenclature Committee of the Fleischner Society. Radiology 1996;200:327-31. [Crossref] [PubMed]
- Ma Z, Liang C, Huang Y, He L, Liang C, Chen X, Huang X, Xiong Y, Liu Z. Can lymphovascular invasion be predicted by preoperative multiphasic dynamic CT in patients with advanced gastric cancer? Eur Radiol 2017;27:3383-91. [Crossref] [PubMed]
- Liu K, Wang M, Xu Y, Chen Q, Li K, Zhang L, Xie X, Shen W. Value of spectral computed tomography-derived quantitative parameters based on full volume analysis in the diagnosis of benign/malignant and pathological subtypes of solitary pulmonary nodules. Quant Imaging Med Surg 2023;13:3827-40. [Crossref] [PubMed]
- Popper HH. Progression and metastasis of lung cancer. Cancer Metastasis Rev 2016;35:75-91. [Crossref] [PubMed]
- Shi CZ, Zhao Q, Luo LP, He JX. Size of solitary pulmonary nodule was the risk factor of malignancy. J Thorac Dis 2014;6:668-76. [Crossref] [PubMed]
- Birau A, Ceausu RA, Cimpean AM, Gaje P, Raica M, Olariu T. Assessement of angiogenesis reveals blood vessel heterogeneity in lung carcinoma. Oncol Lett 2012;4:1183-6. [Crossref] [PubMed]
- Chen C, Chen Q, Zhao Q, Liu M, Guo J. Value of Combined Detection of Serum CEA, CA72-4, CA19-9, CA15-3 and CA12-5 in the Diagnosis of Gastric Cancer. Ann Clin Lab Sci 2017;47:260-3.
- Duffy MJ. Tumor markers in clinical practice: a review focusing on common solid cancers. Med Princ Pract 2013;22:4-11. [Crossref] [PubMed]
- Wang C, Jin S, Xu S, Cao S. The combination of pretreatment prognostic nutritional index and neuron-specific enolase enhances prognosis predicting value of small cell lung cancer. Clin Respir J 2021;15:264-71. [Crossref] [PubMed]
- Chen Z, Liu X, Shang X, Qi K, Zhang S. The diagnostic value of the combination of carcinoembryonic antigen, squamous cell carcinoma-related antigen, CYFRA 21-1, neuron-specific enolase, tissue polypeptide antigen, and progastrin-releasing peptide in small cell lung cancer discrimination. Int J Biol Markers 2021;36:36-44. [Crossref] [PubMed]
- Liu H, Yan F, Pan Z, Lin X, Luo X, Shi C, Chen X, Wang B, Zhang H. Evaluation of dual energy spectral CT in differentiating metastatic from non-metastatic lymph nodes in rectal cancer: Initial experience. Eur J Radiol 2015;84:228-34. [Crossref] [PubMed]
- Zou Y, Zheng M, Qi Z, Guo Y, Ji X, Huang L, Gong Y, Lu X, Ma G, Xia S. Dual-energy computed tomography could reliably differentiate metastatic from non-metastatic lymph nodes of less than 0.5 cm in patients with papillary thyroid carcinoma. Quant Imaging Med Surg 2021;11:1354-67. [Crossref] [PubMed]
- Li L, Cheng SN, Zhao YF, Wang XY, Luo DH, Wang Y. Diagnostic accuracy of single-source dual-energy computed tomography and ultrasonography for detection of lateral cervical lymph node metastases of papillary thyroid carcinoma. J Thorac Dis 2019;11:5032-41. [Crossref] [PubMed]
- Huang S, Meng H, Cen R, Ni Z, Li X, Suwal S, Chen H. Use quantitative parameters in spectral computed tomography for the differential diagnosis of metastatic mediastinal lymph nodes in lung cancer patients. J Thorac Dis 2021;13:4703-13. [Crossref] [PubMed]
- Hu X, Gu Q, Zhang K, Deng D, Li L, Li P, Shen H. Dual-Energy Computed Tomography for the Diagnosis of Mediastinal Lymph Node Metastasis in Lung Cancer Patients: A Preliminary Study. J Comput Assist Tomogr 2021;45:490-4. [Crossref] [PubMed]
- Li X, Meng X, Ye Z. Iodine quantification to characterize primary lesions, metastatic and non-metastatic lymph nodes in lung cancers by dual energy computed tomography: An initial experience. Eur J Radiol 2016;85:1219-23. [Crossref] [PubMed]
- Nagano H, Takumi K, Nakajo M, Fukukura Y, Kumagae Y, Jinguji M, Tani A, Yoshiura T. Dual-Energy CT-Derived Electron Density for Diagnosing Metastatic Mediastinal Lymph Nodes in Non-Small Cell Lung Cancer: Comparison With Conventional CT and FDG PET/CT Findings. AJR Am J Roentgenol 2022;218:66-74. [Crossref] [PubMed]
- Liu S, Xu M, Qiao X, Ji C, Li L, Zhou Z. Prediction of serosal invasion in gastric cancer: development and validation of multivariate models integrating preoperative clinicopathological features and radiographic findings based on late arterial phase CT images. BMC Cancer 2021;21:1038. [Crossref] [PubMed]
- Yang X, Pan X, Liu H, Gao D, He J, Liang W, Guan Y. A new approach to predict lymph node metastasis in solid lung adenocarcinoma: a radiomics nomogram. J Thorac Dis 2018;10:S807-19. [Crossref] [PubMed]
- Liu S, Qiao X, Xu M, Ji C, Li L, Zhou Z. Development and Validation of Multivariate Models Integrating Preoperative Clinicopathological Parameters and Radiographic Findings Based on Late Arterial Phase CT Images for Predicting Lymph Node Metastasis in Gastric Cancer. Acad Radiol 2021;28:S167-78. [Crossref] [PubMed]
- El Adoui M, Drisis S, Benjelloun M. Multi-input deep learning architecture for predicting breast tumor response to chemotherapy using quantitative MR images. Int J Comput Assist Radiol Surg 2020;15:1491-500. [Crossref] [PubMed]
- Aonpong P, Iwamoto Y, Han XH, Lin L, Chen YW. Improved Genotype-Guided Deep Radiomics Signatures for Recurrence Prediction of Non-Small Cell Lung Cancer. Annu Int Conf IEEE Eng Med Biol Soc 2021;2021:3561-4. [Crossref] [PubMed]
- Brinker TJ, Kiehl L, Schmitt M, Jutzi TB, Krieghoff-Henning EI, Krahl D, et al. Deep learning approach to predict sentinel lymph node status directly from routine histology of primary melanoma tumours. Eur J Cancer 2021;154:227-34. [Crossref] [PubMed]