Comparison of artificial intelligence, elastic imaging, and the thyroid imaging reporting and data system in the differential diagnosis of suspicious nodules
Introduction
Thyroid nodule is a prevalent endocrine disease. Previous studies have shown that palpable thyroid nodules are present in about 5% of the iodine-sufficient population, while the prevalence rate of thyroid nodules is as high as 67% (1,2). On the other hand, only approximately 5% of thyroid nodules are malignant (3,4), and most patients have no symptoms. These characteristics make the disease easily overlooked by patients, leading to delayed treatment or conversely, overtreatment.
Ultrasound is currently the imaging method of choice for the thyroid gland and is essential in diagnosing and treating of thyroid nodules (5,6). Sonographic features of malignant thyroid nodules include solid component, very hypoechoic patterns, taller-than-wide dimensions, irregular or lobulated borders, and microcalcifications, among others. However, the sensitivity and specificity of these features vary. Currently, no single feature can reliably identify the nature of thyroid nodules (7). To standardize clinicians’ interpretation of ultrasound images and reduce intraobserver and interobserver discrepancies, researchers in different countries and regions have successively proposed different versions of the thyroid imaging reporting and data system (TIRADS) (8), of which the American College of Radiology TIRADS (ACR-TIRADS) (9) published by the ACR in 2017 is now widely used. The system scores thyroid nodules based on composition, echogenicity, shape, margin, and echogenic foci. Finally, the malignancy risk of the nodule is stratified according to the ACR-TIRADS categories (TR) according to the total score as follows: TR1, benign; TR2, no suspicion; TR3, mild suspicion; TR4, moderate suspicion; and TR5, high suspicion. TR3, TR4, and TR5 all involve suspected malignancy but differ in malignancy risk: the malignancy risk is 5% for TR3 nodules, 5–20% for TR4 nodules, and no less than 20% for TR5 nodules. It follows that even the highest-rated TR5 nodules have a large possible range of risk, and further evaluation of nodules is warranted.
The use of artificial intelligence (AI) involves reducing human intervention as much as possible while using computers to simulate intelligent behavior (10). Breakthrough advances have been made in recent years with deep learning, a technology that enables the construction of models with input samples, producing systems that can learn without explicit programming (11). Supported by this technology, AI is rapidly evolving and being widely used in many fields. S-Detect (Samsung Medison Co., Seoul, South Korea), a computer-aided diagnostic system for ultrasonography using AI (12) based on deep learning algorithms, has demonstrated reliable diagnostic ability in previous studies and is currently used for differentiating between benign and malignant thyroid and breast nodules (13).
Palpation is another standard method for diagnosing thyroid nodules in clinic, and malignant nodules are generally stiffer than are benign ones (14). In 1991, Ophir et al. first proposed two-dimensional elastography to quantify tissue stiffness (15), and the underlying principle of this technique can be explained as follows: an excitation is applied to the tissue to produce a response, and the response is probed and analyzed by ultrasound to calculate physical parameters such as the Young’s modulus, which reflect the elastic situation of the tissue (16). Elastography can be used as a supplement to gray-scale ultrasound to increase diagnostic accuracy (17). A novel elastography technique that calculates the tissue elasticity contrast index (ECI) using carotid pulsation as a pressure source was demonstrated to be capable of determining the nature of thyroid nodules (18-20). The ECI index is based on the Elastoscan method, which is a steady-state quasistatic physiological excitation technique used for obtaining a quantitative stiffness evaluation (19). In contrast to the conventional strain elastography, this technology does not require external compression on the neck area, thus providing more operator-independent thyroid strain images.
In clinical practice, thyroid nodules with evident benign features on ultrasonography are relatively easy to diagnose, but suspicious nodules with malignant ultrasonographic traits frequently confound doctors and patients. Although the diagnostic value of S-Detect and ECI has been confirmed in some studies, the subjects of these research were largely all thyroid nodules or nodules of certain specific pathological characteristics (21-23); thus, the role of these two techniques in identifying the nature of suspicious thyroid nodules is still unclear. Moreover, these two new technologies can be implemented on a single ultrasound diagnostic instrument, and combining them with TIRADS is straightforward and feasible, but no relevant research has been conducted in this area.
The aim of this retrospective study was thus to examine the diagnostic efficacy of ACR-TIRADS, S-Detect, and ECI for suspicious thyroid nodules and to explore whether their combination could improve the quality of assessment. We present this article in accordance with the STARD reporting checklist (available at https://qims.amegroups.com/article/view/10.21037/qims-23-788/rc).
Methods
The study was conducted in accordance with the Declaration of Helsinki (as revised in 2013) and was approved by the Ethics Committee of the First Hospital of China Medical University (No. 2023540). Informed consent was obtained from all individual participants.
Patients
According to the pre-experiment results, the sensitivity of ACR-TIRADS was 0.72, the sensitivity of S-Detect was 0.97, and the discordant proportion was 0.29. However, there was no significant correlation between ECI and the nature of nodules. The prevalence of thyroid nodules was set to 0.45 (24). The inspection efficiency (1−β) was 0.9, and the inspection level (α) was 0.05. According to PASS 2021 software (NCSS, LLC. Kaysville, UT, USA), the sample size was estimated to be 98. Between January 2021 and November 2021, 101 consecutive patients with 138 thyroid nodules were enrolled in our center. The inclusion criteria were the following: (I) TR3, TR4, and TR5 nodules defined as “suspicious nodules”; (II) patients who had surgical or cytopathological results after ultrasound examination; and (III) voluntary enrollment in this study. Meanwhile, the exclusion criteria were the following: (I) TR1 and TR2 nodules, (II) patients who had undergone fine-needle aspiration (FNA) before ultrasound examination, and (III) inconclusive cytologic findings.
Inconclusive FNA results were excluded based on the Bethesda system (25). Finally, a nodule was diagnosed as benign if any of the following criteria were met: (I) benign according to repeated FNA examinations or postoperative pathology; and (II) one benign result on FNA examination and no significant change in the nodule on ultrasonography after 1 year of follow-up. A thyroid nodule was diagnosed as malignant if malignancy was evident in the surgical specimen or when Bethesda V (suspicion of malignancy) or VI (malignant) was determined on cytology. The doctors involved in the pathological analysis were not aware of the results of the ultrasound examination.
Ultrasound examination and analysis
Thyroid ultrasonography was performed before FNA or thyroidectomy in patients. Patients were in the supine position, and a 3 to 12-MHz linear transducer (Samsung Ultrasound RS80A, Samsung Medison Co.) was used in this study. The ultrasound gel was smeared on the probe and gently affixed to the patient’s neck for examination, resulting in an image of the thyroid nodule with appropriate gray scale, contrast, and lesion location.
The examiner (Y.F.Z.) and another sonographer (X.M.W.) respectively followed ACR-TIRADS in the interpretation of gray-scale ultrasound images according to composition (cystic or almost completely cystic, spongiform, mixed cystic and solid, solid or almost completely solid), echogenicity (anechoic, hyperechoic or isoechoic, hypoechoic, very hypoechoic), shape (wider-than-tall or taller-than-wide), margin (smooth, ill-defined, lobulated or irregular, extrathyroidal extension), echogenic foci (none or large comet-tail artifacts, macrocalcifications, peripheral [rim] calcifications, punctate echogenic foci). If the assessment by the two doctors was not concordant, a discussion was held to determine the result. Moreover, two observers were blinded to the results of S-Detect and ECI. TR5 was considered positive, whereas TR3 and TR4 were considered negative.
S-Detect (Samsung Medison Co.) was then used to delineate the nodule contour automatically, manual drawing was adopted when the boundary of the nodule drawn by the software was in error, and finally, S-Detect made a judgment of “possibly benign” or “possibly malignant” regarding the quality of thyroid nodules.
Elastography was next performed on each nodule, during which the examiner applied no external pressure and only carotid pulsations were used as the pressure source, with good elastography being indicated when the bar graph on the right side of the screen stabilized to green. The examiner selected solid areas of the nodule (avoiding macrocalcifications, peripheral [rim] calcifications, and anechoic areas), manually set the region of interest (ROI) within the lesion, and then the software automatically calculated the ECI value (Figure 1). The elastography procedure lasted approximately 5 seconds, and the patients were asked to hold their breath during the examination. This process was repeated three times, and the median was recorded.
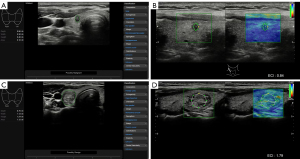
Except for the participation of X.M.W. when evaluating thyroid nodules according to ACR-TIRADS, the rest of the work was performed by Y.F.Z. during the same examination. Both had more than 10 years of clinical experience in thyroid ultrasonography.
Statistical analysis
The data in this study were analyzed with SPSS 25.0 (IBM Corp., Armonk, NY, USA) and MedCalc 18.2.1 (MedCalc Software Ltd, Ostend, Belgium). The enumeration data were contrasted using the two-sided chi-squared test. A two-tailed independent-sample t test was used to observe differences in the measurement data. For the combined assessment of gray-scale ultrasonography and S-Detect, the predicted probability was generated by fitting the diagnostic yield of ACR-TIRADS and S-Detect via binary logistic regression. Moreover, receiver operating characteristic (ROC) curves were used to compare the diagnostic levels of different methods. P<0.05 indicated a statistically significant difference.
Results
Of 101 enrolled patients, 30 were excluded for the following reasons: unclear pathological findings (n=19), incomplete data on elastography (n=6), and lack of consent for FNA or surgery (n=5). Ultimately, 71 patients (12 men and 59 women; mean age 43±13 years; age range, 12–72 years), comprising a total of 94 thyroid nodules (42 benign nodules and 52 malignant nodules) were included in the study (Figure 2). The nodules’ diameter ranged from 0.19 to 3.61 cm, with an average of 0.98±0.70 cm. Among the malignant nodules, 40 were pathologically confirmed by surgical resection, which included 39 papillary carcinomas and 1 follicular carcinoma, and the remaining 12 malignant nodules were diagnosed based on FNA results, including 11 of Bethesda class V and 1 of class VI. Five benign nodules were surgically confirmed, and the remaining 37 were diagnosed according to the results of FNA and subsequent follow-up.
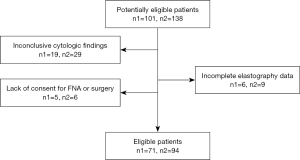
The characteristics of the thyroid nodules included in this study are summarized in Table 1. Gender, age, diameter, composition, echogenicity, shape, and echogenic foci were not statistically different between patients with benign and malignant nodules. The irregular or lobulated margin of the nodule was significantly associated with thyroid cancer (P=0.004). ACR-TIRADS and S-Detect could differentially diagnosis thyroid nodules (P=0.007 and P=0.014, respectively), but ECI could not (P=0.592).
Table 1
Characteristic | Benign (n=42) | Malignant (n=52) | P value |
---|---|---|---|
Gender | 0.053 | ||
Male | 4 | 13 | |
Female | 38 | 39 | |
Age (years), mean [SD] | 46 [14] | 40 [11] | 0.093 |
Diameter (cm), mean [SD] | 1.03 [0.70] | 0.94 [0.70] | 0.561 |
Composition | 0.464 | ||
Solid | 39 | 51 | |
Mixed cystic and solid | 3 | 1 | |
Echogenicity | 0.556 | ||
Hyperechoic or isoechoic | 7 | 5 | |
Hypoechoic | 24 | 34 | |
Very hypoechoic | 11 | 13 | |
Shape | 0.177 | ||
Wider-than-tall | 22 | 20 | |
Taller-than-wide | 20 | 32 | |
Margin | 0.004 | ||
Smooth or ill-defined | 24 | 14 | |
Lobulated or irregular | 18 | 35 | |
Extrathyroidal extension | 0 | 3 | |
Echogenic foci | 0.497 | ||
Non or large comet-tail artifacts | 22 | 27 | |
Macrocalcifications | 5 | 4 | |
Peripheral calcifications | 5 | 3 | |
Punctate echogenic foci | 10 | 18 | |
ACR-TIRADS | 0.007 | ||
TR3 | 3 | 0 | |
TR4 | 14 | 8 | |
TR5 | 25 | 44 | |
S-Detect | 0.014 | ||
Possibly benign | 8 | 1 | |
Possibly malignant | 34 | 51 | |
ECI, mean [SD] | 1.73 [1.35] | 1.48 [1.00] | 0.592 |
ACR-TIRADS, American College of Radiology Thyroid Imaging Reporting and Data System; TR, ACR-TIRADS category; ECI, elasticity contrast index.
Table 2 summarizes the diagnostic efficacy of ACR-TIRADS, S-Detect, and their combination. S-Detect had a significantly better sensitivity than did ACR-TIRADS (P=0.036), but its specificity was lower (P=0.032), and there was no difference between the accuracy of the two (P>0.05). The specificity, positive predictive value (PPV), and overall accuracy of the combination of S-Detect and ACR-TIRADS were higher than either used alone, but these differences were not statistically significant.
Table 2
Parameters | ACR-TIRADS | S-Detect | S-Detect combined with ACR-TIRADS | P value1 | P value2 | P value3 |
---|---|---|---|---|---|---|
Sensitivity (%) | 84.6 | 98.1 | 82.7 | 0.036 | 0.791 | 0.034 |
Specificity (%) | 40.5 | 19.0 | 45.2 | 0.032 | 0.659 | 0.010 |
PPV (%) | 63.8 | 60.0 | 65.2 | – | – | – |
NPV (%) | 68.0 | 88.9 | 67.9 | – | – | – |
Accuracy (%) | 64.9 | 62.8 | 66.0 | 0.761 | 0.878 | 0.648 |
P value1: comparison of S-Detect vs. ACR-TIRADS. P value2: comparison of S-Detect combined with ACR-TIRADS vs. ACR-TIRADS. P value3: comparison of S-Detect combined with ACR-TIRADS vs. S-Detect. ACR-TIRADS, American College of Radiology Thyroid Imaging Reporting and Data System; PPV, positive predictive value; NPV, negative predictive value.
Figure 3 compares the area under the curve (AUC) of the ROC curve for ACR-TIRADS, S-Detect, and their combination. The AUC was 0.631 [95% confidence interval (CI): 0.525–0.728] for ACR-TIRADS, 0.586 (95% CI: 0.479–0.686) for S-Detect, and 0.640 (95% CI: 0.534–0.736) for the combined assessment. Although the AUC of S-Detect combined with ACR-TIRADS was slightly higher than that of either used alone, this difference was not statistically significant.
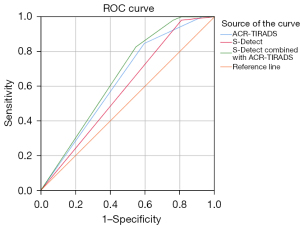
Discussion
This study investigated the value of S-Detect and ECI in differentiating the nature of suspicious thyroid nodules. The findings suggested that S-Detect had a similar discriminatory power for suspicious thyroid nodules to ACR-TIRADS, whereas ECI had relatively limited efficacy, and that of ACR-TIRADS and S-Detect combined was comparable but not superior to that of ACR-TIRADS alone. To the best of our knowledge, compared with previous reports, our study was the first to consider the combination of ACR-TIRADS, S-Detect, and ECI in the differential diagnosis of thyroid nodules. Furthermore, due to the difficulty in distinguishing suspicious thyroid nodules, only TR3, TR4, and TR5 nodules as assessed by ACR-TIRADS were selected for further clinical need. Additionally, to avoid the influence of artifacts, we merely used the above three methods to evaluate thyroid nodules without considering other clinical data of the patients and without relying on the examiners’ empirical judgment.
In recent years, with the advancement of instruments and continuous improvement of ultrasound image quality, an increasing number of thyroid nodules are being detected. For patients with thyroid nodules detected by ultrasound, active follow-up or FNA examination is often recommended to clarify the property of nodules. Although FNA reduces thyroid surgery in patients with benign nodules (26), it can still not avoid aggravating the psychological and financial burden of many patients. One study showed that 17.54–28.67% of FNAs based on different versions of the TIRADS were unnecessary (27). Therefore, developing a means to determining the nature of thyroid nodules more accurately with ultrasound images is a crucial need in clinical work.
The integration of AI is expected to improve the accuracy and consistency of ultrasonography, reduce unnecessary FNA, and optimize the management of thyroid nodules. There are presently numerous AI technologies in the field of thyroid ultrasound, among which S-Detect is the most widely used (28-31). According to Choi et al.’s study of 89 patients, the sensitivity of S-Detect was similar to that of experienced sonographers (90.7% vs. 88.4%), but both the specificity and AUC were lower (specificity: 74.6% vs. 94.9%; AUC: 0.83 vs. 0.92) (12). Barczyński et al. reported that the sensitivity and negative predictive value (NPV) of S-Detect for identifying the nature of thyroid nodules were similar to those with surgeons with specialized ultrasound skills (sensitivity: 90% vs. 90%; NPV: 96.97% vs. 97.44%), while the specificity and PPV were significantly lower (specificity: 80% vs. 95%; PPV: 52.94% vs. 81.82%), but still superior to surgeons with basic ultrasound skills (32). Wei et al. reported that the accuracy, specificity, PPV, and AUC of S-Detect were superior to those of less experienced sonographers (accuracy: 77.0% vs. 63.7–65.2%; specificity: 65.2% vs. 37.5–49.1%; PPV: 68.3% vs. 55.7–57.8%; AUC: 0.782 vs. 0.666–0.669), but it was not significantly helpful for experienced ultrasound doctors. The level of this technology was roughly equivalent to that of ultrasound doctors with 9 years of work experience (33). Li et al.’s investigation also found similar outcomes (34). A prospective study of 88 patients by Szczepanek-Parulska et al. showed that the specificity, PPV, and accuracy of S-Detect were superior to those of experienced sonographers (specificity: 80.6% vs. 61.2%; PPV: 81.9% vs. 69.8%; accuracy: 85% vs. 75.9%), while the sensitivity and NPV were similar (sensitivity: 89.4% vs. 90.0%; NPV: 88.5% vs. 87.2%) (35).
In contrast, our study found that S-Detect had a better sensitivity (98.1%) than did ACR-TIRADS (84.6%), but the specificity of S-Detect was low at only 19.0%. S-Detect had a similar PPV to that of ACR-TIRADS (60.0% vs. 63.8%), and the NPV of the former (88.9%) was higher than that of the latter (68.0%). Additionally, S-Detect was similar to ACR-TIRADS in terms of accuracy (62.8% vs. 64.9%).
The specificity (45.2%), PPV (65.2%), overall accuracy (66.0%), and AUC (0.640) of the combination of S-Detect and ACR-TIRADS were higher compared with the sole use of S-Detect or ACR-TIRADS, but these differences were not statistically significant.
Compared to other researches (12,32-35), our study found a lower diagnostic ability of S-Detect and TIRADS. This may be because we only selected suspicious thyroid nodules, whose ultrasound images all had one or more malignant features. S-Detect and TIRADS essentially analyze the image characteristics of nodules, so their ability to distinguish the nature of these nodules may be reduced. Furthermore, compared to ACR-TIRADS, S-Detect had a much lower specificity and was more volatile. Excessive reliance on S-Detect for differentiating suspicious thyroid nodules may therefore result in benign nodules being misdiagnosed as malignancies.
Ultrasound elastography can noninvasively assess the mechanical properties of tissues and utilize the changes in the elasticity of diseased tissues to generate qualitative and quantitative information that can be used for diagnosis (36). It can thus be leveraged as a supplement to conventional ultrasound images and is a valuable method for differentiating the nature of lesions. In this study, we employed a novel elastography technique, which used the carotid pulse as an internal pressure source to calculate the ECI value, reducing influence of observers and providing greater objectivity than would be possible by artificially applying external pressure (37). Several studies have reported the diagnostic value of elastography using the pulse of the carotid artery for thyroid nodules. According to Cho et al.’s study, the accuracy of gray-scale ultrasound combined with ECI in diagnosis (78.6%) was higher than that of gray-scale ultrasound alone (76.9%) or ECI (67.1%) (38). A retrospective study of 102 patients by Choi et al. found that the ECI value of thyroid malignant nodules was significantly higher than that of benign nodules. The AUC of gray-scale ultrasound and ECI were 0.755 (95% CI: 0.660–0.835) and 0.835 (95% CI: 0.748–0.901), respectively, and the AUC of the combined diagnosis of the two methods was 0.853 (95% CI: 0.769–0.915), which was significantly higher than that of gray-scale ultrasound alone (P=0.022) (39). Dighe et al. explored the efficacy of ultrasound elastography in the differential diagnosis of small thyroid nodules. Their results showed that the AUC of ECI for the diagnosis of small papillary thyroid carcinoma (PTMC) was 0.812 (95% CI: 0.653–0.920), and with an ECI of 3.6 as the cutoff value, the sensitivity for the diagnosis of PTMC was 100% while the specificity was 60% (40).
However, in our study, the difference in ECI between benign and malignant nodules was not significant, which may be due to the following: (I) the nodules included in our study were small in size, of which 59 (62.8%) were less than 1 cm in diameter and difficult to be detected with elastography. (II) Our study aimed at evaluating suspicious nodules, the risk of included nodules was generally high, with 69 (73.4%) being TR5, and these high-risk nodules might not have had a substantial difference in hardness.
It is worth noting that the thyroid nodules included in this study were strictly selected, and differences in imaging characteristics were relatively small (Table 1). The study was likely underpowered due to this reason, and the true effect will likely not be discerned until more studies are performed. Additionally, the study’s external validity was confined to thyroid nodules with suspicious ultrasound features only.
Our study has several limitations which should be mentioned. First, we only included thyroid nodules that had undergone FNA or surgery, so there might have been selection bias. Second, Bethesda V cytology was classified as malignant since these nodules subsequently underwent BRAF (V600E) tests, and the results were all positive. According to Trimboli et al.’s study, the PPV of the BRAF test is excellent (99%) (41). However, there was still a risk of treating these nodules as malignancies. After excluding these 11 nodules, we repeated the calculation and discovered that while the figures changed somewhat, the ultimate conclusion remained the same. Third, the evaluation of suspicious nodules according to ACR-TIRADS was performed by two experienced doctors, and the evaluation level was probably higher than the average of the sonographers in general. In addition, the nodules included in this study were generally small, potentially leading to an underestimation of the diagnostic capabilities of the respective techniques. Furthermore, the number of cases included in this study was small, which might have rendered the statistical difference nonsignificant. Finally, we did not analyze factors that could have affected the ultrasound elastography results, such as patient age, weight, blood pressure, presence of underlying diseases such as atherosclerosis, and the distance of the nodule from the carotid artery.
Conclusions
For differentiating suspicious thyroid nodules, S-Detect has a high sensitivity, but its specificity is inferior to that of ACR-TIRADS. The diagnostic ability of the combination is comparable but not superior to that of ACR-TIRADS alone, while that of ECI is uncertain. At present, S-Detect cannot replace manual diagnosis, and the value of elastography using the carotid artery for determining the nature of suspected thyroid nodules remains unclear.
Acknowledgments
Funding: This study was funded by
Footnote
Reporting Checklist: The authors have completed the STARD reporting checklist. Available at https://qims.amegroups.com/article/view/10.21037/qims-23-788/rc
Conflicts of Interest: All authors have completed the ICMJE uniform disclosure form (available at https://qims.amegroups.com/article/view/10.21037/qims-23-788/coif). Y.F.Z. reports that this work was funded by the Health and Medical Big Data Research Institute of China Medical University (No. HMB201901103). The other authors have no conflicts of interest to declare.
Ethical Statement: The authors are accountable for all aspects of the work in ensuring that questions related to the accuracy or integrity of any part of the work are appropriately investigated and resolved. The study was conducted in accordance with the Declaration of Helsinki (as revised in 2013) and was approved by the Ethics Committee of the First Hospital of China Medical University (No. 2023540). Informed consent was obtained from all individual participants.
Open Access Statement: This is an Open Access article distributed in accordance with the Creative Commons Attribution-NonCommercial-NoDerivs 4.0 International License (CC BY-NC-ND 4.0), which permits the non-commercial replication and distribution of the article with the strict proviso that no changes or edits are made and the original work is properly cited (including links to both the formal publication through the relevant DOI and the license). See: https://creativecommons.org/licenses/by-nc-nd/4.0/.
References
- Durante C, Grani G, Lamartina L, Filetti S, Mandel SJ, Cooper DS. The Diagnosis and Management of Thyroid Nodules: A Review. JAMA 2018;319:914-24. [Crossref] [PubMed]
- Uppal N, Collins R, James B. Thyroid nodules: Global, economic, and personal burdens. Front Endocrinol (Lausanne) 2023;14:1113977. [Crossref] [PubMed]
- Kobaly K, Kim CS, Mandel SJ. Contemporary Management of Thyroid Nodules. Annu Rev Med 2022;73:517-28. [Crossref] [PubMed]
- Wong R, Farrell SG, Grossmann M. Thyroid nodules: diagnosis and management. Med J Aust 2018;209:92-8. [Crossref] [PubMed]
- Alexander EK, Cibas ES. Diagnosis of thyroid nodules. Lancet Diabetes Endocrinol 2022;10:533-9. [Crossref] [PubMed]
- Zhou J, Yin L, Wei X, Zhang S, Song Y, Luo BSuperficial Organ and Vascular Ultrasound Group of the Society of Ultrasound in Medicine of the Chinese Medical Association, et al. Chinese Artificial Intelligence Alliance for Thyroid and Breast Ultrasound. 2020 Chinese guidelines for ultrasound malignancy risk stratification of thyroid nodules: the C-TIRADS. Endocrine 2020;70:256-79.
- Grani G, Sponziello M, Pecce V, Ramundo V, Durante C. Contemporary Thyroid Nodule Evaluation and Management. J Clin Endocrinol Metab 2020;105:2869-83. [Crossref] [PubMed]
- Levine RA. History of Thyroid Ultrasound. Thyroid 2023;33:894-902. [Crossref] [PubMed]
- Tessler FN, Middleton WD, Grant EG, Hoang JK, Berland LL, Teefey SA, Cronan JJ, Beland MD, Desser TS, Frates MC, Hammers LW, Hamper UM, Langer JE, Reading CC, Scoutt LM, Stavros AT. ACR Thyroid Imaging, Reporting and Data System (TI-RADS): White Paper of the ACR TI-RADS Committee. J Am Coll Radiol 2017;14:587-95. [Crossref] [PubMed]
- Hamet P, Tremblay J. Artificial intelligence in medicine. Metabolism 2017;69S:S36-40. [Crossref] [PubMed]
- The Lancet. Artificial intelligence in health care: within touching distance. Lancet 2017;390:2739. [Crossref] [PubMed]
- Choi YJ, Baek JH, Park HS, Shim WH, Kim TY, Shong YK, Lee JH. A Computer-Aided Diagnosis System Using Artificial Intelligence for the Diagnosis and Characterization of Thyroid Nodules on Ultrasound: Initial Clinical Assessment. Thyroid 2017;27:546-52. [Crossref] [PubMed]
- Zhang D, Jiang F, Yin R, Wu GG, Wei Q, Cui XW, Zeng SE, Ni XJ, Dietrich CF. A Review of the Role of the S-Detect Computer-Aided Diagnostic Ultrasound System in the Evaluation of Benign and Malignant Breast and Thyroid Masses. Med Sci Monit 2021;27:e931957. [Crossref] [PubMed]
- Monpeyssen H, Tramalloni J, Poirée S, Hélénon O, Correas JM. Elastography of the thyroid. Diagn Interv Imaging 2013;94:535-44. [Crossref] [PubMed]
- Ophir J, Céspedes I, Ponnekanti H, Yazdi Y, Li X. Elastography: a quantitative method for imaging the elasticity of biological tissues. Ultrason Imaging 1991;13:111-34. [Crossref] [PubMed]
- Gennisson JL, Deffieux T, Fink M, Tanter M. Ultrasound elastography: principles and techniques. Diagn Interv Imaging 2013;94:487-95. [Crossref] [PubMed]
- Chambara N, Lo X, Chow TCM, Lai CMS, Liu SYW, Ying M. Combined Shear Wave Elastography and EU TIRADS in Differentiating Malignant and Benign Thyroid Nodules. Cancers (Basel) 2022.
- Lim DJ, Luo S, Kim MH, Ko SH, Kim Y. Interobserver agreement and intraobserver reproducibility in thyroid ultrasound elastography. AJR Am J Roentgenol 2012;198:896-901. [Crossref] [PubMed]
- Cantisani V, Lodise P, Di Rocco G, Grazhdani H, Giannotti D, Patrizi G, Medvedyeva E, Olive M, Fioravanti C, Giacomelli L, Chiesa C, Redler A, Catalano C, D'Ambrosio F, Ricci P. Diagnostic accuracy and interobserver agreement of Quasistatic Ultrasound Elastography in the diagnosis of thyroid nodules. Ultraschall Med 2015;36:162-7. [Crossref] [PubMed]
- Hahn SY, Shin JH, Ko EY, Bae JM, Choi JS, Park KW. Complementary Role of Elastography Using Carotid Artery Pulsation in the Ultrasonographic Assessment of Thyroid Nodules: A Prospective Study. Korean J Radiol 2018;19:992-9. [Crossref] [PubMed]
- Zhou L, Zheng LL, Zhang CJ, Wei HF, Xu LL, Zhang MR, Li Q, He GF, Ghamor-Amegavi EP, Li SY. Comparison of S-Detect and thyroid imaging reporting and data system classifications in the diagnosis of cytologically indeterminate thyroid nodules. Front Endocrinol (Lausanne) 2023;14:1098031. [Crossref] [PubMed]
- De Fiori E, Lanza C, Carriero S, Tettamanzi F, Frassoni S, Bagnardi V, Mauri G. The European Institute of Oncology Thyroid Imaging Reporting and Data System for Classification of Thyroid Nodules: A Prospective Study. J Clin Med 2022;11:3238. [Crossref] [PubMed]
- Xie X, Yu Y. Effect of the location and size of thyroid nodules on the diagnostic performance of ultrasound elastography: A retrospective analysis. Clinics (Sao Paulo) 2020;75:e1720. [Crossref] [PubMed]
- Li Y, Jin C, Li J, Tong M, Wang M, Huang J, Ning Y, Ren G. Prevalence of Thyroid Nodules in China: A Health Examination Cohort-Based Study. Front Endocrinol (Lausanne) 2021;12:676144. [Crossref] [PubMed]
- Cibas ES, Ali SZ. The 2017 Bethesda System for Reporting Thyroid Cytopathology. Thyroid 2017;27:1341-6. [Crossref] [PubMed]
- Cibas ES. Fine-needle aspiration in the work-up of thyroid nodules. Otolaryngol Clin North Am 2010;43:257-71. vii-viii. [Crossref] [PubMed]
- Zhu H, Yang Y, Wu S, Chen K, Luo H, Huang J. Diagnostic performance of US-based FNAB criteria of the 2020 Chinese guideline for malignant thyroid nodules: comparison with the 2017 American College of Radiology guideline, the 2015 American Thyroid Association guideline, and the 2016 Korean Thyroid Association guideline. Quant Imaging Med Surg 2021;11:3604-18. [Crossref] [PubMed]
- Peng S, Liu Y, Lv W, Liu L, Zhou Q, Yang H, et al. Deep learning-based artificial intelligence model to assist thyroid nodule diagnosis and management: a multicentre diagnostic study. Lancet Digit Health 2021;3:e250-9. [Crossref] [PubMed]
- Zhu J, Zhang S, Yu R, Liu Z, Gao H, Yue B, Liu X, Zheng X, Gao M, Wei X. An efficient deep convolutional neural network model for visual localization and automatic diagnosis of thyroid nodules on ultrasound images. Quant Imaging Med Surg 2021;11:1368-80. [Crossref] [PubMed]
- Akkus Z, Cai J, Boonrod A, Zeinoddini A, Weston AD, Philbrick KA, Erickson BJ. A Survey of Deep-Learning Applications in Ultrasound: Artificial Intelligence-Powered Ultrasound for Improving Clinical Workflow. J Am Coll Radiol 2019;16:1318-28. [Crossref] [PubMed]
- Zhong L, Wang C. Diagnostic accuracy of S-Detect in distinguishing benign and malignant thyroid nodules: A meta-analysis. PLoS One 2022;17:e0272149. [Crossref] [PubMed]
- Barczyński M, Stopa-Barczyńska M, Wojtczak B, Czarniecka A, Konturek A. Clinical validation of S-Detect mode in semi-automated ultrasound classification of thyroid lesions in surgical office. Gland Surg 2020;9:S77-S85. [Crossref] [PubMed]
- Wei Q, Zeng SE, Wang LP, Yan YJ, Wang T, Xu JW, Zhang MY, Lv WZ, Cui XW, Dietrich CF. The value of S-Detect in improving the diagnostic performance of radiologists for the differential diagnosis of thyroid nodules. Med Ultrason 2020;22:415-23. [Crossref] [PubMed]
- Li Y, Liu Y, Xiao J, Yan L, Yang Z, Li X, Zhang M, Luo Y. Clinical value of artificial intelligence in thyroid ultrasound: a prospective study from the real world. Eur Radiol 2023;33:4513-23. [Crossref] [PubMed]
- Szczepanek-Parulska E, Wolinski K, Dobruch-Sobczak K, Antosik P, Ostalowska A, Krauze A, Migda B, Zylka A, Lange-Ratajczak M, Banasiewicz T, Dedecjus M, Adamczewski Z, Slapa RZ, Mlosek RK, Lewinski A, Ruchala M. S-Detect Software vs. EU-TIRADS Classification: A Dual-Center Validation of Diagnostic Performance in Differentiation of Thyroid Nodules. J Clin Med 2020;9:2495. [Crossref] [PubMed]
- Sigrist RMS, Liau J, Kaffas AE, Chammas MC, Willmann JK. Ultrasound Elastography: Review of Techniques and Clinical Applications. Theranostics 2017;7:1303-29. [Crossref] [PubMed]
- Bae U, Dighe M, Dubinsky T, Minoshima S, Shamdasani V, Kim Y. Ultrasound thyroid elastography using carotid artery pulsation: preliminary study. J Ultrasound Med 2007;26:797-805. [Crossref] [PubMed]
- Cho YJ, Ha EJ, Han M, Choi JW. US Elastography Using Carotid Artery Pulsation May Increase the Diagnostic Accuracy for Thyroid Nodules with US-Pathology Discordance. Ultrasound Med Biol 2017;43:1587-95. [Crossref] [PubMed]
- Choi WJ, Park JS, Koo HR, Kim SY, Chung MS, Tae K. Ultrasound elastography using carotid artery pulsation in the differential diagnosis of sonographically indeterminate thyroid nodules. AJR Am J Roentgenol 2015;204:396-401. [Crossref] [PubMed]
- Dighe M, Luo S, Cuevas C, Kim Y. Efficacy of thyroid ultrasound elastography in differential diagnosis of small thyroid nodules. Eur J Radiol 2013;82:e274-80. [Crossref] [PubMed]
- Trimboli P, Scappaticcio L, Treglia G, Guidobaldi L, Bongiovanni M, Giovanella L. Testing for BRAF (V600E) Mutation in Thyroid Nodules with Fine-Needle Aspiration (FNA) Read as Suspicious for Malignancy (Bethesda V, Thy4, TIR4): a Systematic Review and Meta-analysis. Endocr Pathol 2020;31:57-66. [Crossref] [PubMed]