Low 18F-fluorodeoxyglucose dose positron emission tomography assisted by a deep-learning image-denoising technique in patients with lymphoma
Introduction
Fluorine-18 (18F) fluorodeoxyglucose positron emission tomography/computed tomography ([18F]FDG PET/CT) is widely used for tumor staging and therapy evaluation in patients with lymphoma. Despite the favorable overall survival rate and consequent prolongation of life span in patients with lymphoma, the cumulative radiation dose of multiple PET/CT exams and its associated cancer risk cannot be ignored, especially in pediatric patients who are sensitive to radiation or those who need multiple PET/CT exams to monitor the therapeutic response (1). Recommendations of minimal [18F]FDG dose and acquisition time were established by nuclear medicine societies (2-4) to help PET centers implement good imaging practices following an as-low-as-reasonably-achievable (ALARA) principle. With new imaging technologies being developed, a greater number of studies are being conducted for further dose reduction.
Lower injected activity or shorter scan duration without sacrificing diagnostic image quality represents a desirable goal for PET imaging protocol optimization (5). However, a lower injected dose leads to higher image noise and suboptimal diagnostic image quality. In recent years, deep learning (DL) has been widely investigated in medical imaging fields (6-8). DL denoising techniques can significantly reduce image noise (9,10), which allows for a decrease in injected activity in oncology patients. However, the effects of the DL denoising technique on the diagnostic image quality and tumor evaluation of low-dose (LD) [18F]FDG PET/CT in patients with lymphoma has not been fully investigated. Therefore, the aim of this study was to investigate the impact of the DL denoising technique on image quality, tumor quantification, and Deauville score (DS) of half-dose [18F]FDG PET in patients with lymphoma. To this end, two nuclear medicine radiologists assessed the visual image quality score, DS, and quantitative parameters of PET images to characterize the stability and reproducibility of the technique.
Methods
Patients
This retrospective study enrolled 62 patients with lymphoma confirmed via the pathology from April 2021 to October 2022 in the Affiliated Hospital of Qingdao University. The patients were referred to [18F]FDG PET/CT exams for initial staging or follow-up studies. The patients were enrolled if their PET images were of diagnostic quality without artifacts and if the list-mode PET data were available for additional reconstructions. The mean age of the patients was 55±18 years, the mean body weight was 65±13 kg, and the mean injected [18F]FDG dose was 6.9±1.4 mCi (0.11±0.01 mCi/kg). The other patient characteristics are listed in Table 1. The study was conducted in accordance with the Declaration of Helsinki (as revised in 2013). This study was approved by the Institutional Ethics Committee of the Affiliated Hospital of Qingdao University and the informed consent was waived due to the retrospective nature of this study.
Table 1
Characteristics | Value |
---|---|
Female/male* | 33/29 |
Age (years) | 55±18 [13, 76] |
Weight (kg) | 65±13 [42, 120] |
Height (cm) | 166±12 [108, 185] |
Body mass index (kg/m2) | 23.6±3.4 [15.1, 35.1] |
Injected dose (MBq) | 256.0±52.6 [160.2, 455.1] |
Injected dose per patient weight (MBq/kg) | 3.9±0.2 [3.4, 4.8] |
Blood glucose (mmol/L) | 6.0±1.0 [4.4, 10.6] |
Uptake time (min) | 62±11 [50, 85] |
Treatment naïve/chemotherapy cases* | 38/24 |
Cases with Deauville score of 1/2/3/4/5* | 24/2/5/4/27 |
Lymphoma type* | |
Diffuse large B-cell lymphoma | 35 |
Marginal zone lymphoma | 7 |
Hodgkin lymphoma | 6 |
NK/T-cell lymphoma | 4 |
Follicular lymphoma | 3 |
Other non-Hodgkin lymphomas | 7 |
The values are presented as the mean ± standard deviation [range]. *, the values are counts. NK, natural killer.
Image acquisition protocol
The patients were instructed to fast for 6 hours without extreme exercise before the PET/CT scans. When the patient arrived at the imaging center, the level of blood glucose was measured to ensure a level of less than 11.0 mmol/L. The [18F]FDG solution was administered intravenously based on the patient’s weight (0.1 mCi/kg). The patients then rested in a waiting room with a quiet and warm environment for approximately 55 minutes (min).
All images were acquired with a United Imaging uMI Vista PET/CT scanner (United Imaging Healthcare, Shanghai, China) that integrated a 24-cm axial field-of-view (FOV) lutetium-yttrium oxyorthosilicate (LYSO) crystal-based digital PET and an 80-detector CT scanner. A CT scan was performed for attenuation correction and anatomic localization using a 120-kVp tube voltage and an 80-mAs tube current. The PET images were acquired in a three-dimensional (3D) list mode from the upper thighs to the skull base with an emission time of 2 min per patient bed.
The standard-of-care PET images were generated using the 2-min list-mode PET data with ordered subset expectation-maximization (OSEM) reconstruction. The OSEM reconstruction used two iterations, 20 subsets, 150×150 matrix dimensions, a 600-mm FOV, a 2.85-mm slice thickness, 4.5-mm Gaussian postfiltering, time of flight (TOF), attenuation correction, scatter correction, decay correction, and other vendors’ default corrections. The LD PET was simulated by using the first minute of the PET list-mode data, which allows for intrapatient comparison. The simulated LD PET images were generated by applying a convolutional denoising neural network (HYPER DLR, United Imaging Healthcare) after OSEM reconstruction using the same settings as those of the standard-of-care PET. The HYPER DLR is a US Food and Drug Administration (FDA) 510 k cleared denoising neural network for PET imaging. This denoising neural network is applied after OSEM reconstruction as a DL-based image processing function, specifically developed to reduce image noise in [18F]FDG PET. It is based on a U-Net architecture with residual network (ResNet)-style blocks and dense convolutional network (DenseNet)-like connections. In this study, the training datasets included 393 PET scans from four centers with United Imaging Healthcare PET/CT scanners (the data of our center was not involved in the training procedure). More details of the neural network architecture illustration and training procedure can be found in the supplementary file (Appendix 1) and the related literature (9). Hereafter, we refer to the standard-of-care PET images with OSEM reconstruction as the routine-dose (RD) group and the simulated LD PET images after applying the denoising neural network as the LD group.
Image evaluation
Two nuclear radiologists, reader 1 and reader 2 with 4 and 6 years of experience in oncology PET/CT, respectively, assessed the visual image quality of PET images using a 5-point scale. A score of 1 to 5 was given to the images with nondiagnostic, acceptable, moderate, good, or excellent image quality, respectively. The readers also reported the DSs for the visual interpretation of [18F]FDG uptake in the same reading session. The readers scored the images independently without knowing the other’s results, and the case reading orders were randomized to reduce the memory effects. The readers were blind to patient information and the reconstruction settings. The visual image assessment was performed on a commercial medical image workstation (uWS-MI, United Imaging Healthcare). The axial, coronal, sagittal, and fusion views of PET/CT images were available, and the windowing [target/background (T/B)] could be freely adjusted by the raters. To evaluate intrareader repeatability, reader 1 repeated the assessment of the visual image quality and the DS 2 weeks after the initial assessment.
Two nuclear radiologists with 4 and 6 years of experience in oncology PET/CT performed the quantitative evaluation. The evaluation included two parts: the quantitative evaluation in the liver and the mediastinum and the quantification evaluation of the tumor. In the first part, a circular region of interest (ROI) was placed in a homogeneous area of the right liver lobe parenchyma on PET images. The area of the ROI was 1,040 mm2 and was used for all patients. Similar to the ROI placement in the liver, another ROI was placed in the mediastinum blood pool (aorta arch) with a fixed size of 480 mm2. The maximum standardized uptake value (SUVmax), mean SUV (SUVmean), and SD in the liver ROI and the mediastinal ROI were measured. The coefficient of variation (COV) was calculated by dividing the SD by the SUVmean of the liver or the mediastinum ROI and presented in percentages.
In the second part of the quantitative evaluation, the SUVmax, SUVmean, metabolic tumor volume (MTV), and tumor lesion glycolysis (TLG) were measured at the selected lesions using 3D Slicer software (v. 5.0.2). The lesion was selected by the radiologist according to the following criteria: the lesion can be segmented using a SUV threshold larger than 2.5, the volume of the lesions was larger than 1.0 cm3, and the border of the lesion could be clearly defined to make a valid paired comparison between the RD and LD group. If there were more than multiple candidate lesions in a patient, a maximum of four lesions with the largest size and higher SUVs were included. Before starting quantitative measurement, the two nuclear radiologists reached a consensus regarding the lesion selection and then performed the segmentation individually.
The structural similarity index measure (SSIM) and peak signal to noise ratio (PSNR) were respectively used as the measurements of the fidelity and quality of images that had been adopted in DL clinical study workflows (11). The SSIM and PSNR were calculated on LD images using RD images as the original images. Furthermore, the DL denoising neural network was applied on RD images to create the reference images for calculating SSIM and PSNR for RD and LD images such that the reference images had lower noise than did RD images. The code is available on online (https://github.com/msyan/QD_DLR).
Statistical analysis
The data were analyzed with the R v. 4.2.0 software (The R Foundation of Statistical Computing) and Microsoft Excel (2016 edition). The visual image quality scores were compared between the RD and LD group with the Wilcoxon signed-rank test. The DS was analyzed with the contingency table. The inter- and intrareader agreements of the categorical scores were assessed with Cohen κ. The correlation and limits of agreement (LoA) of SUVmax and SUVmean in the liver and the mediastinum between the RD and LD groups were analyzed with the Spearman correlation coefficient and Bland-Altman plots. The LoA was defined as the mean difference between the RD and LD group and its 95% confidence interval (CI). The correlation, linear relationship, and LoA of the lesions’ measurements (SUVmax, SUVmean, MTV, and TLG) between the RD and LD group were analyzed with the Spearman correlation coefficient, linear regression, and Bland-Altman plots. Because the lesions’ measurements varied in a large range, the percentages of the LoA were calculated to determine their relative differences. The percentage mean difference was calculated by dividing the difference between the RD and LD group by the measurement of the RD group. LoA and intraclass correlation coefficient (ICC) were applied to ascertain the interreader agreement of the quantitative data.
Results
Among the 62 participants enrolled in this study, 78 lesions in 30 patients were included in the quantitative tumor evaluation. There was no lesion or the lesions did not meet the criteria in the other 32 patients. The MTVs of the lesions were 27.52±48.18 cm3, and the lesion’s SUVmax was 14.26±9.11.
The visual image quality score was comparable or better in the LD group compared with the RD group (Figure 1). In the RD group, the rater scored 0, 24, 32, 6, and 0 cases with a visual image quality score of 1, 2, 3, 4, and 5 respectively. In contrast, in the LD group, 0, 3, 23, 36, and 0 cases received a score of 1 to 5, respectively. Therefore, cases in the LD group attained higher scores compared with the RD group, and this result was statistically significant (RD 2.7±0.6 vs. LD 3.5±0.6; P<0.001). The inter- and intrareader agreements were excellent in the visual image quality score (κ=0.92 and κ=0.99).
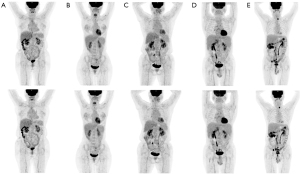
Using the LD images instead of the RD images did not change the patient management but did have a user-dependent impact on the decision of the DS. In the RD group, one of the readers rated 24, 2, 5, 4, and 27 cases with a DS of 1 to 5, respectively, and the assignment of the DS was not changed when the LD group was used instead (Figures 2,3). Meanwhile, the other reader assigned 24, 2, 5, 4, and 27 cases to the RD group and 24, 3, 4, 4, and 27 cases to the LD group with a DS of 1 to 5, respectively. Reader 1 obtained the same DS in the RD and LD groups as that of the reader 2 after 2 weeks. The discrepancy in the DS between the RD and LD groups occurred in a case after follow-up of three cycles of chemotherapy. In this case, a score of 2 was assigned using the RD images, but a score of 3 was assigned using the LD images. Further data analysis showed that the variance in the mediastinal SUVmax between the RD and LD groups might have caused this discrepancy. The SUVmax in the liver, mediastinum, and lesion were 3.28, 2.38, and 2.29 in the RD group, respectively, and were 2.80, 2.19, and 2.24 in the LD group, respectively. However, this discrepancy did not change patient management. The inter- and intrarater agreements were excellent for the DS (κ=0.99 and κ=0.99).
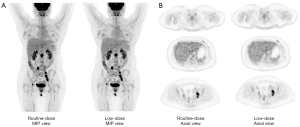
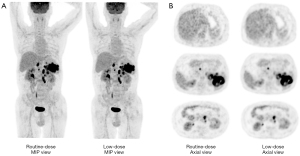
The data of SUVmax, SUVmean, SD, and COV in the liver and mediastinum are shown in Table 2. The SUVmax and SUVmean in the liver and mediastinum were highly correlated between the RD and LD groups, with a correlation coefficient of 0.86 to 0.97. The LoA between the RD and LD group in the SUVmax and SUVmean were good. The mean difference of the LoA between the RD and LD group was less than 0.22 for SUVmax and 0.02 for SUVmean, and the 95% CI of the differences was –0.19 to 0.64 for SUVmax and –0.17 to 0.20 for SUVmean. The SD was smaller in the LD group than in the RD group. In line with the SD result, the COV was also smaller in the LD group, suggesting the image noise measurement of the LD group was better compared with the RD group in both the liver and mediastinum.
Table 2
Measurement | RD group | LD group | LoA | Correlation coefficient |
---|---|---|---|---|
Mediastinal SUVmax | 2.09±0.34 | 1.96±0.30 | 0.13 [−0.17, 0.42] | 0.86 |
Mediastinal SUVmean | 1.65±0.28 | 1.63±0.28 | 0.02 [−0.12, 0.16] | 0.97 |
Mediastinal SD | 0.20±0.08 | 0.15±0.03 | 0.05 [−0.10, 0.19] | – |
Mediastinal COV (%) | 12.03±4.93 | 9.21±2.02 | 2.82 [−6.23, 11.86] | – |
Liver SUVmax | 2.83±0.42 | 2.60±0.35 | 0.22 [−0.19, 0.64] | 0.88 |
Liver SUVmean | 2.19±0.28 | 2.17±0.28 | 0.02 [−0.17, 0.20] | 0.95 |
Liver SD | 0.25±0.06 | 0.18±0.05 | 0.06 [−0.01, 0.14] | – |
Liver COV (%) | 11.29±2.26 | 8.45±2.03 | 2.84 [−0.50, 6.17] | – |
The data of SUV, SD, and COV in the RD and LD groups are the mean ± SD. The values of the LoA are the mean difference with 95% confidence intervals between the RD and LD groups. SUV, standardized uptake value; SD, standard deviation; COV, coefficient of variation; RD, routine dose; LD, low dose; LoA, limits of agreement; SUVmax, maximum SUV; SUVmean, mean SUV.
The SUVmax, SUVmean, MTV, and TLG of the lesions were highly correlated between the RD and LD groups. All correlation coefficients were larger than 0.99. The linear regression analysis indicated that the relationship of the lesions’ SUVmax, SUVmean, MTV, and TLG between the RD and LD group could be well fit with straight lines (Figure 4A-4D). The slopes of the lines were 1.05, 1.03, 0.97, and 0.98 for SUVmax, SUVmean, MTV, and TLG, respectively. The adjusted R2 values were larger than 0.98 in all linear regression models, suggesting excellent goodness of fit.
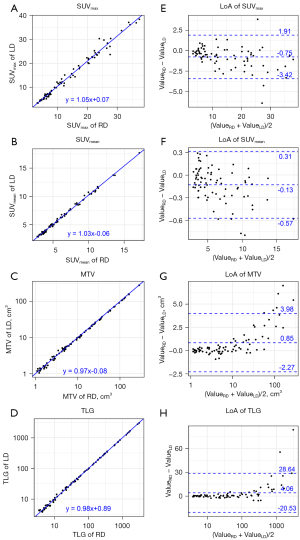
In the LoA analysis, the mean differences between the RD and LD groups were –0.88 (95% CI: –3.47 to 1.71) for SUVmax, –0.17 (95% CI: –0.69 to 0.35) for SUVmean, 0.69 (95% CI: –2.40 to 3.77) for MTV, and 2.74 (95% CI: –15.92 to 21.40) for TLG. The percentages of the mean differences between the RD and LD groups were –5.32% (95% CI: –22.31% to 11.67%) for SUVmax, –1.83% (95% CI: –9.17% to 5.50%) for SUVmean, 1.98% (95% CI: –13.46% to 17.41%) for MTV, and 0.24% (95% CI: –15.44% to 15.92%) for TLG (Figure S2). The Bland-Altman plots for lesions’ SUVmax, SUVmean, MTV, and TLG are shown in Figure 4E-4H). In Table 3, the ICC and LoA showed excellent reliability for lesion measurements between the two readers.
Table 3
Lesion measurement | Reader 1 | Reader 2 | ICC | LoA |
---|---|---|---|---|
SUVmax | 2.09±0.34 | 1.96±0.30 | 1 | −0.01 [−0.25, 0.23] |
SUVmean | 1.65±0.28 | 1.63±0.28 | 1 | −0.00 [−0.07, 0.07] |
MTV (cm3) | 0.20±0.08 | 0.15±0.03 | 1 | −0.01 [−0.39, 0.38] |
TLG (g/mL·cm3) | 12.03±4.93 | 9.21±2.02 | 1 | −0.03 [−1.20, 1.15] |
The data of SUV, MTV, and TLG between reader 1 and reader 2 are the mean ± SD. The values for the LoA are the mean difference and 95% confidence intervals between the two readers. ICC, intraclass correlation coefficient; LoA, limits of agreement; SUVmax, maximum standardized uptake value; SUVmean, mean standardized uptake value; MTV, metabolic tumor volume; TLG, tumor lesion glycolysis; SD, standard deviation.
The median, mean, and first and third quartiles of SSIM were 0.9975, 0.9972, 0.9961, and 0.9986, respectively, for LD images when RD was used as the reference. The median, mean, and first and third quartiles of PSNR were 53.74, 54.76, 51.98, and 57.97, respectively, for LD images when RD was used as the reference. Using the DL denoised standard-of-care PET images as the reference, the median, mean, and first and third quartiles of SSIM were 0.9984 vs. 0.9989, 0.9983 vs. 0.9989, 0.9976 vs. 0.9986, and 0.9993 vs. 0.9994, respectively, for LD and RD images; meanwhile, the median, mean, and first and third quartiles of PSNR were 55.88 vs. 58.36, 57.43 vs. 0.58.76, 53.82 vs. 56.46, and 61.02 vs. 60.47, respectively, for LD and RD images. Figure S3 displays the comparison of SSIM and PSNR between the RD and LD images.
Discussion
This study demonstrated the capability of the DL denoising technique in LD [18F]FDG PET in patients with lymphoma. Our results showed that with a 50% reduction of [18F]FDG dose, the visual image noise score and quantitative image noise index in the liver and mediastinum were improved with DL denoising compared with those of routine [18F]FDG dose PET with OSEM reconstruction. Furthermore, the SUVs in the liver, mediastinum, and lesions and the MTV and TLG in the lesions were highly correlated and had good LoA between the RD and LD groups. All correlation coefficients were higher than 0.86. The mean difference between the RD and LD groups was less than 0.22 in the SUVmax of the liver and mediastinum, 0.02 in the SUVmean of the liver and mediastinum, –0.75 (–5.32%) in the SUVmax of the lesions, –0.13 (–1.83%) in the SUVmean of the lesions, 0.85 (1.98%) in the MTV of the lesions, and 4.06 (0.24%) in the TLG of the lesions. The DSs were mostly consistent between the RD and LD groups, and the classifications of negative (DS 1–3) and positive (Deauville 4–5) results were unchanged, suggesting patient management was unaffected. In summary, applying DL denoising neural networks in PET with a 50% reduction of [18F]FDG dose improved and maintained the image quality, tumor quantification accuracy, and DS in patients with lymphoma. Therefore, the DL denoising technique can reduce the radiation burden of PET studies in patients with lymphoma without sacrificing diagnostic image quality or efficacy.
Reducing radiation dose in accordance with the ALARA principle while maintaining diagnostic image quality of PET/CT is important provide benefit to patients (12,13) but also aid in the work of PET technologists (14). With the advance of PET techniques, the [18F]FDG dose can be further reduced (15-19). Among these techniques, DL denoising neural network is a promising approach that can reduce the [18F]FDG dose by 25–87.5% in oncology patients (9,20-23). Xing et al. (9) reported that a U-Net-based convolutional neural network, such as the one used in our study, improved signal-to-noise ratio and contrast-to-noise ratio with a 25–50% reduction of the counts of coincidence events in oncology patients. Weyts et al. (21) showed that, with a 2.5D encoder-decoder U-Net neural network, the image quality of half-duration PET could be recovered and satisfy clinical requirements in oncology patients. Similarly, Theruvath et al. (22) showed that the assessment of treatment response was correct using PET-magnetic resonance (MR) with a 50% reduction in [18F]FDG dose in children and young patients with lymphoma. Furthermore, Sanaat et al (23) demonstrated that a cycle-consistent generative adversarial network model could generate PET images with one-eighth of injected activity while maintaining the performance of image quality, lesion detection, and quantification accuracy in whole-body PET tumor imaging. In line with these studies, our results suggested that the qualitative and quantitative image quality of 50% dose of [18F]FDG PET with a U-Net-based denoising neural network can satisfy the clinical requirements for tumor quantification, staging, and treatment assessment in patients with lymphoma.
The percentages of [18F]FDG dose reduction depend on the reference dose level, PET performance, and DL algorithms. In this study, the reference dose standard was an injection of 0.11 mCi/kg [18F]FDG multiplied by an emission time of 2 min per bed, which is concordant with the recommendation of the minimal injected dose and scan time product; that is, 7 MBq/kg/min per bed, according to a procedure guideline for [18F]FDG PET tumor imaging published in 2015 (2). This weight-based injection dose was shown to be efficient in minimizing the injected dose and improve the image quality [17]. The state-of-the-art digital PET/CT with higher TOF resolution may allow further reduction of [18F]FDG dose or of acquisition time in lymphoma and tumor PET imaging (16,21). These studies suggest that the reference level of the injected dose is an evolving target with the advance of PET technologies, and this is the reason we referred to the standard-of-care PET protocol as the “routine” dose instead of the “full” dose in this study. A previous study using the same DL technique as used in our study reported a 25–50% reduction with a reference protocol of 3 min per bed and a 259-MBq [18F]FDG dose in PET tumor imaging. Our results supported a 50% reduction in patients with lymphoma with a reference of 2 min per bed and a similar injected dosage. The difference in the percentage of dose reduction may be a result of the PET system performance and fine-tuning of the neural network to adapt to different PET scanner models. Moreover, another study (23) reported the superior performance of the generative adversarial network on dose reduction in [18F]FDG PET tumor imaging compared with the residual neural network; a 87.5% reduction was found to be feasible, which is higher than reported in our and others’ studies (9,20,21). We speculate that the superior performance may be attributed to the generative adversarial network, as suggested by other research (24,25). Another possible explanation for this is that other studies use the training and testing data from the same PET/CT scanner (23-25), while the DL model in our study was a commercial product that was developed with training data from other centers and other PET systems (9). Therefore, the performance is less optimized to a specific PET model but more generalized to multiple centers and/or scanners, as has been demonstrated in a multicenter and multivendor study (26). Nevertheless, more multicenter clinical evaluations are needed to validate the appropriate [18F]FDG dose reduction for PET tumor imaging with DL denoising techniques and to establish new reference dose levels.
Additionally, other cross-center studies should be conducted to address the challenge of ensuring the reliable performance of DL in real-world applications (27). In our research, we used a DL denoising neural network technique to reduce image noise in PET images. The effectiveness of the neural network was independently validated on a third-party dataset, which suggests the DL denoising technique can be potentially used in real-world clinical practice. One plausible explanation for this success could be that the neural network was trained to distinguish between noise and the actual signal in the PET images. The training targets of the network were normal-dose PET images, while the training inputs were LD PET images. To make the process practical, we simulated LD PET images by uniformly downsampling the list-mode data of normal-dose PET during the image reconstruction phase. This approach allowed us to create almost-ideal paired datasets for training and enabled the neural network to learn the noise characteristics of LD PET. An added advantage of this method was the reduced need for human intervention, thereby avoiding potential previously described uncertainties (27). However, this approach does face challenges related to generalization. Since the training datasets exclusively consisted of [18F]FDG PET images from a single vendor’s scanner, the algorithm’s performance on other PET tracers may be limited. Adapting the algorithm for different noise characteristics of other scanners would require fine-tuning. Moreover, the simulation method for LD PET used in our study relies on list-mode PET raw-data for additional image reconstruction, demanding extra data storage and management effort. These concerns and limitations should be further investigated in future studies.
Our results showed that the DL denoising technique can maintain or improve the diagnostic image quality of low [18F]FDG PET in patients with lymphoma. These results were consistent with the finding of previous studies (21,28). Furthermore, the quantitative consistency of SUV between LD PET with DL denoising and RD PET was demonstrated in a larger population. Although our data showed a good overall agreement in MTV and TLG between the RD and LD group, it is interesting to see how absolute differences increased with larger MTV and TLG, while the relative differences did not (Figure 4 and Figure S1). This may be due to the lesion segmentation method. A lesion segmented with a fixed threshold of 2.5 could include more voxels in the images with higher noise such as in the RD group compared with the DL-processed LD group. This phenomenon becomes more notable on the lesions with a large MTV or TLG, for example, >50 or >500, respectively. Other segmentation methods, such as adaptive threshold, statistical methods, or majority vote schemes, should be studied in the future. In line with a previous study that used the TOF technique (16), we found the DS was concordant between the RD group and DL processed LD group. In summary, our results support the use of low [18F]FDG dose PET imaging with DL denoising techniques in patients with lymphoma that can enhance safety for patients and potentially save the cost of tracer use in PET exams (19).
Our study has limitations. We investigated a low [18F]FDG dose with simulation. A true low-injected-dose study should be performed to validate our results. Furthermore, a 50% reduction of the injected dose was chosen in this study based on our preliminary study (Figures S4,S5) and the results of previous studies (9,21). However, the limits of dose reduction should be investigated further. The participants with a DS of 2–4 accounted for 17.7% of the study cohort. A study with a larger cohort and balanced inclusion regarding DS can provide higher statistical power to investigate the concordance of the DS.
Conclusions
The DL denoising technique may enable a lower dose of [18F]FDG in PET imaging for patients with lymphoma. Our data showed that the SUV quantification, tumor burden evaluation, and DS assessment were correct for staging and treatment response assessment in patients with lymphoma subjected to a 50% dose of [18F]FDG in PET with the DL denoising neural network. This result can be used to support the use of DL denoising techniques in LD PET tumor imaging to minimize the risk associated with the radiation and reduce the medical cost of using PET tracers.
Acknowledgments
We would like to thank Dr. Mengshi Yan for the preparation and uploading of the Python code to Github.
Funding: None.
Footnote
Conflicts of Interest: All authors have completed the ICMJE uniform disclosure form (available at https://qims.amegroups.com/article/view/10.21037/qims-23-817/coif). R.W. is an employee of the Beijing United Imaging Research Institute of Intelligent Imaging. The other authors have no conflicts of interest to declare.
Ethical Statement: The authors are accountable for all aspects of the work in ensuring that questions related to the accuracy or integrity of any part of the work are appropriately investigated and resolved. The study was conducted in accordance with the Declaration of Helsinki (as revised in 2013).
Open Access Statement: This is an Open Access article distributed in accordance with the Creative Commons Attribution-NonCommercial-NoDerivs 4.0 International License (CC BY-NC-ND 4.0), which permits the non-commercial replication and distribution of the article with the strict proviso that no changes or edits are made and the original work is properly cited (including links to both the formal publication through the relevant DOI and the license). See: https://creativecommons.org/licenses/by-nc-nd/4.0/.
References
- Arabi H. AkhavanAllaf A, Sanaat A, Shiri I, Zaidi H. The promise of artificial intelligence and deep learning in PET and SPECT imaging. Phys Med 2021;83:122-37. [Crossref] [PubMed]
- Boellaard R, Delgado-Bolton R, Oyen WJ, Giammarile F, Tatsch K, Eschner W, et al. FDG PET/CT: EANM procedure guidelines for tumour imaging: version 2.0. Eur J Nucl Med Mol Imaging 2015;42:328-54. [Crossref] [PubMed]
- Vali R, Alessio A, Balza R, Borgwardt L, Bar-Sever Z, Czachowski M, Jehanno N, Kurch L, Pandit-Taskar N, Parisi M, Piccardo A, Seghers V, Shulkin BL, Zucchetta P, Lim R. SNMMI Procedure Standard/EANM Practice Guideline on Pediatric (18)F-FDG PET/CT for Oncology 1.0. J Nucl Med 2021;62:99-110. [Crossref] [PubMed]
- Graham MM, Wahl RL, Hoffman JM, Yap JT, Sunderland JJ, Boellaard R, Perlman ES, Kinahan PE, Christian PE, Hoekstra OS, Dorfman GS. Summary of the UPICT Protocol for 18F-FDG PET/CT Imaging in Oncology Clinical Trials. J Nucl Med 2015;56:955-61. [Crossref] [PubMed]
- Catana C. The Dawn of a New Era in Low-Dose PET Imaging. Radiology 2019;290:657-8. [Crossref] [PubMed]
- Apostolopoulos ID, Papathanasiou ND, Apostolopoulos DJ, Panayiotakis GS. Applications of Generative Adversarial Networks (GANs) in Positron Emission Tomography (PET) imaging: A review. Eur J Nucl Med Mol Imaging 2022;49:3717-39. [Crossref] [PubMed]
- Illimoottil M, Ginat D. Recent Advances in Deep Learning and Medical Imaging for Head and Neck Cancer Treatment: MRI, CT, and PET Scans. Cancers (Basel) 2023;15:3267. [Crossref] [PubMed]
- Xiao H, Teng X, Liu C, Li T, Ren G, Yang R, Shen D, Cai J. A review of deep learning-based three-dimensional medical image registration methods. Quant Imaging Med Surg 2021;11:4895-916. [Crossref] [PubMed]
- Xing Y, Qiao W, Wang T, Wang Y, Li C, Lv Y, Xi C, Liao S, Qian Z, Zhao J. Deep learning-assisted PET imaging achieves fast scan/low-dose examination. EJNMMI Phys 2022;9:7. [Crossref] [PubMed]
- Pain CD, Egan GF, Chen Z. Deep learning-based image reconstruction and post-processing methods in positron emission tomography for low-dose imaging and resolution enhancement. Eur J Nucl Med Mol Imaging 2022;49:3098-118. [Crossref] [PubMed]
- Pati S, Thakur SP, Hamamcı İE, Baid U, Baheti B, Bhalerao M, et al. GaNDLF: the generally nuanced deep learning framework for scalable end-to-end clinical workflows. Commun Eng 2023;2:23.
- Huang B, Law MW, Khong PL. Whole-body PET/CT scanning: estimation of radiation dose and cancer risk. Radiology 2009;251:166-74. [Crossref] [PubMed]
- Chawla SC, Federman N, Zhang D, Nagata K, Nuthakki S, McNitt-Gray M, Boechat MI. Estimated cumulative radiation dose from PET/CT in children with malignancies: a 5-year retrospective review. Pediatr Radiol 2010;40:681-6. [Crossref] [PubMed]
- Roberts FO, Gunawardana DH, Pathmaraj K, Wallace A. U PL, Mi T, Berlangieri SU, O'Keefe GJ, Rowe CC, Scott AM. Radiation dose to PET technologists and strategies to lower occupational exposure. J Nucl Med Technol 2005;33:44-7.
- Sun H, Jiang Y, Yuan J, Wang H, Liang D, Fan W, Hu Z, Zhang N. High-quality PET image synthesis from ultra-low-dose PET/MRI using bi-task deep learning. Quant Imaging Med Surg 2022;12:5326-42. [Crossref] [PubMed]
- Weber M, Jentzen W, Hofferber R, Herrmann K, Fendler WP, Rischpler C, Umutlu L, Conti M, Costa PF, Sraieb M, Kersting D. Evaluation of (18)F-FDG PET/CT images acquired with a reduced scan time duration in lymphoma patients using the digital biograph vision. BMC Cancer 2021;21:62. [Crossref] [PubMed]
- Alves VPV, Brady S, Ata NA, Li Y, MacLean J, Zhang B, Sharp SE, Trout AT. Simulated Reduced-Count Whole-Body FDG PET: Evaluation in Children and Young Adults Imaged on a Digital PET Scanner. AJR Am J Roentgenol 2022;219:952-61. [Crossref] [PubMed]
- Sagara H, Inoue K, Yaku H, Ohsawa A, Someya T, Yanagisawa K, Ohashi S, Ishigaki R, Wakabayashi M, Muramatsu Y, Fujii H. Optimization of injection dose in (18)F-FDG PET/CT based on the 2020 national diagnostic reference levels for nuclear medicine in Japan. Ann Nucl Med 2021;35:1177-86. [Crossref] [PubMed]
- Ng CKC. Artificial Intelligence for Radiation Dose Optimization in Pediatric Radiology: A Systematic Review. Children (Basel) 2022;9:1044. [Crossref] [PubMed]
- Katsari K, Penna D, Arena V, Polverari G, Ianniello A, Italiano D, Milani R, Roncacci A, Illing RO, Pelosi E. Artificial intelligence for reduced dose 18F-FDG PET examinations: a real-world deployment through a standardized framework and business case assessment. EJNMMI Phys 2021;8:25. [Crossref] [PubMed]
- Weyts K, Lasnon C, Ciappuccini R, Lequesne J, Corroyer-Dulmont A, Quak E, Clarisse B, Roussel L, Bardet S, Jaudet C. Artificial intelligence-based PET denoising could allow a two-fold reduction in [18F]FDG PET acquisition time in digital PET/CT. Eur J Nucl Med Mol Imaging 2022;49:3750-60.
- Theruvath AJ, Siedek F, Yerneni K, Muehe AM, Spunt SL, Pribnow A, Moseley M, Lu Y, Zhao Q, Gulaka P, Chaudhari A, Daldrup-Link HE. Validation of Deep Learning-based Augmentation for Reduced (18)F-FDG Dose for PET/MRI in Children and Young Adults with Lymphoma. Radiol Artif Intell 2021;3:e200232. [Crossref] [PubMed]
- Sanaat A, Shiri I, Arabi H, Mainta I, Nkoulou R, Zaidi H. Deep learning-assisted ultra-fast/low-dose whole-body PET/CT imaging. Eur J Nucl Med Mol Imaging 2021;48:2405-15. [Crossref] [PubMed]
- Lei Y, Dong X, Wang T, Higgins K, Liu T, Curran WJ, Mao H, Nye JA, Yang X. Whole-body PET estimation from low count statistics using cycle-consistent generative adversarial networks. Phys Med Biol 2019;64:215017. [Crossref] [PubMed]
- Zhou L, Schaefferkoetter JD, Tham IWK, Huang G, Yan J. Supervised learning with cyclegan for low-dose FDG PET image denoising. Med Image Anal 2020;65:101770. [Crossref] [PubMed]
- Chaudhari AS, Mittra E, Davidzon GA, Gulaka P, Gandhi H, Brown A, Zhang T, Srinivas S, Gong E, Zaharchuk G, Jadvar H. Low-count whole-body PET with deep learning in a multicenter and externally validated study. NPJ Digit Med 2021;4:127. [Crossref] [PubMed]
- Bhowmik A, Eskreis-Winkler S. Deep learning in breast imaging. BJR Open 2022;4:20210060. [Crossref] [PubMed]
- Yamagiwa K, Tsuchiya J, Yokoyama K, Watanabe R, Kimura K, Kishino M, Tateishi U. Enhancement of 18F-Fluorodeoxyglucose PET Image Quality by Deep-Learning-Based Image Reconstruction Using Advanced Intelligent Clear-IQ Engine in Semiconductor-Based PET/CT Scanners. Diagnostics (Basel) 2022;12:2500. [Crossref] [PubMed]