Characteristics of whole-body dynamic 18F-FDG PET/CT Patlak multi-parametric imaging in lung cancer and the influence of different delineation methods on quantitative parameters
Introduction
Lung cancer is the most common cancer type and the leading cause of cancer-related death worldwide (1). Positron emission tomography/computed tomography (PET/CT) using flourine-18 fluorodeoxyglucose (18F-FDG) plays an important role in the diagnosis and staging of lung cancer (2). Whole-body dynamic multi-parameter PET imaging is a recently developed technique in nuclear medicine, which allows for tracking the positron tracer’s activity distribution inside the body in vivo over 3-dimensional (3D) space and time, namely over a 4-dimensional (4D) spatiotemporal space, to provide valuable clinically relevant information beyond the metrics that can be deduced from the standardized uptake value (SUV) metric alone (3-5). Researchers have explored the scanning mode of whole-body dynamic 18F-FDG PET and recommended continuous dynamic acquisition from the start of the imaging agent injection to 75 min, and new parameter images can be obtained through the Patlak reconstruction (6): an image representing the metabolic rate of FDG into the tissue (MRFDG) and another representing the distribution volume (DV) of free FDG in the tissue (7).
Although dynamic whole-body PET has been in clinical use for fewer than 5 years, the clinical workflow has been optimized to reduce the scanning time (8). Dias et al. (9) recommended the use of a population-based input function (PBIF) to transform Patlak imaging into a 20-min procedure]. We have previously reported on the feasibility of shorter acquisition times using two short dynamic scans for a multiparametric PET study and the influence of quantitative performance in shortened dynamic PET (10). Several reports have demonstrated the use of dynamic whole-body PET/CT to describe parameters in normal tissues and different types of tumors, such as non-small cell lung cancer, breast cancer, nasopharyngeal carcinoma, and so on (11-13). The role of dynamic 18F-FDG PET/CT Patlak multi-parametric imaging in tumor diagnosis, staging, and efficacy evaluation has also been preliminary explored (14-16). Dynamic PET imaging has enabled the continuous assessment of tissue metabolism. DV images reflect free FDG, whereas MRFDG images reflect FDG metabolism, and SUV images are equivalent to the “sum” of MRFDG and DV images (17). Thus, the combination of the three parameters improves the specificity and accuracy of lesion diagnosis. However, to our knowledge, the characteristics and the delineation method of whole-body dynamic 18F-FDG PET/CT Patlak multi-parametric imaging of lung tumor need further exploration. The aim of this study was to assess the characteristics of whole-body dynamic 18F-FDG PET/CT Patlak multi-parametric imaging in lung cancer and the influence of different delineation methods on quantitative parameters.
Methods
Patient clinical data collection
The study was approved by the Clinical Medical Research Ethics Committee of The First Affiliated Hospital of Anhui Medical University (PJ2019-15-04) and informed consent was provided by all individual participants. This study was conducted in accordance with the Declaration of Helsinki (as revised in 2013).
Lung cancer diagnosis and treatment rely heavily on accurate imaging techniques, with 18F-FDG PET/CT playing a crucial role. The Patlak multi-parametric analysis method offers insights into tumor behavior and heterogeneity. In this study, we explored different ways of outlining to enhance the reliability and accuracy of lung cancer assessment using whole-body dynamic 18F-FDG PET/CT Patlak multi-parametric imaging.
The inclusion criteria were as follows (Figure 1): (I) adult lung cancer patients who underwent dynamic whole-body 18F-FDG PET/CT before treatment, (II) adequate image quality for image interpretation, and (III) the lesion pathology type was obtained by histopathological staining and immunohistochemical analysis. The exclusion criteria were poor image quality for analysis or incomplete pathological information.
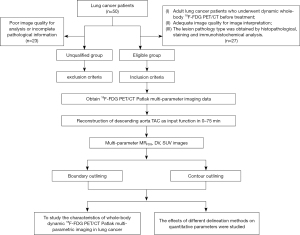
From August 2020 to August 2022, 27 patients with pathologically confirmed lung cancer, who underwent dynamic whole-body 18F-FDG PET imaging were randomly included. Parametric images of MRFDG and Patlak intercept (DV) (DV = V0 + Vp = K1K2/(K2 + K3)2 + Vp) were generated using the nested directed Patlak reconstruction method on Siemens Biograph Vision workstation (Siemens Healthineers, Erlangen, Germany). The maximum, mean, and peak values of primary lung cancer lesions were investigated using contour delineation and boundary delineation. Statistical analysis was performed to analyze the characteristics of multi-parametric images of lung cancer and the relationship with clinicopathological features, and to compare the effects of contour delineation and boundary delineation on quantitative parameters.
Whole-body dynamic 18F-FDG PET/CT imaging
A Siemens Biograph Vision PET/CT scanner (Siemens) was used. 18F-FDG was purchased from Nanjing Jiangyuan Andike Positron Research and Development Co. (Wuxi, China). Patients were required to fast for at least 6 hours before the scan, and their blood glucose levels were controlled below 8.1 mmol/L after 15 min of quiet rest. Once the purpose and advantages of the examination process had been explained to the patients and their family members and informed consent had been provided, the participants underwent dynamic whole-body PET/CT. PET data were acquired starting simultaneously with the injection of a weight-based dose of 18F-FDG (3.71±1.05 MBq/kg). The whole scanning process took approximately 75 min and consisted of the following steps (Figure 2): (I) low-dose whole-body CT (1.0 mm section thickness, 100 kV tube voltage, 43 mA/s tube current) for attenuation correction; (II) 18F-FDG injection that started at the same time as a 6-min dynamic single-bed PET scan centered on the cardiac region, which was used to acquire the input function (12 frames × 5 s, 6 frames × 10 s, 8 frames × 30 s); and (III) a subsequent set of 16 whole-body PET scans in continuous bed motion to capture the late dynamics of the tracer in both the blood plasma and the tissues (5 frames × 2 min, 11 frames × 5 min).
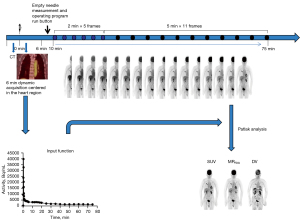
Reconstruction method
After the scan was completed, the image-derived input function (IDIF) was automatically generated from the delineation of the proximal descending aorta using step (II). Patlak reconstruction (to perform the Patlak transformation) to form the MRFDG, and DV parametric images (Figure 3) used all frames of the PET series and the IDIF. The patient’s blood glucose was obtained to calculate the MRFDG using the following equation: the tracer uptake rate (Ki) = K1K3/(K2 + K3). K1: plasma to non-phosphorylated compartment, K2: non-phosphorylated to plasma compartment, K3: non-phosphorylated to phosphorylated compartment.
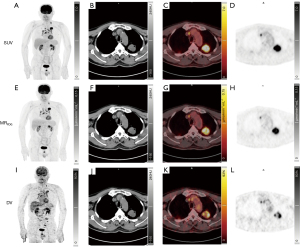
MRFDG = Ki × blood glucose. The last three dynamic PET frames (60 to 75 min) were used to reconstruct the routine static clinical PET images for SUV calculation [SUV = radiation concentration in the lesion (kBq/mL)/injection volume (MBq)/weight (kg)]. The reconstruction parameters were as follows: ordered subset expectation maximization (OSEM) True X + TOF, four iterations, five subsets, and 220 matrices, with relative scatter correction and no Gaussian post filtering.
Image analysis
Double-blind visual comparison of SUV, MRFDG, and DV images was performed on the workstation (syngo.via, Siemens Healthineers) by two nuclear medicine physicians (attending physicians with more than 10 years of experience in PET reading). When conducting quantitative analysis of SUV, MRFDG, and DV images, we needed to consider how to delineate the volume of interest (VOI) in the three images, especially the delineation of lesions. We used two different outlining methods in this study. The first implemented the contour outlining (i.e., threshold outlining) method to outline the lesion in the SUV images and replicate the contour (threshold outlining) in the MRFDG and DV images, where the threshold outlining method automatically draws the VOI on the primary lung cancer lesion using 41% of the maximum pixel value as the segmentation threshold outline boundary (18). The second method used the boundary outline (i.e., shape outline, a spherical VOI of the largest diameter of the primary lesion) method in the SUV images to outline the lesion and replicate the shape in the MRFDG and DV images (VOI shape consistent). The max, peak, and mean values of SUV, MRFDG, and DV parameters of the primary lesion were obtained. In essence, ‘peak’ emphasizes the momentary highest value at a specific time or location, whereas the ‘maximum value’ considers the highest average or cumulative value over the entire time or spatial range. The background area was manually outlined in the adjacent tissue to obtain the mean value of the background (BKGmean) and the standard deviation of the background (BKGSD).
We used the target-to-background ratio (TBR) and the contrast-to-noise ratio (CNR) as two objective indicators to quantitatively assess the image quality in terms of the “lesion detectability”. The mean and max values of the TBR were given as: TBRmax = tumor VOImax/background VOImean; TBRmean = tumor VOImean/background VOImean. These were measured on SUV images TBRmax-SUV and TBRmean-SUV, and on parametric MRFDG images TBRmax-MRFDG and TBRmean-MRFDG.
CNR is the lesion contrast (target tumor-background) divided by the noise (SD in the background). The CNR for the mean and max was given as: CNRmean = (tumor VOImean-background VOImean)/background ROISD; CNRmax = (tumor VOImax-background VOImean)/background ROISD. These were measured on SUV images CNRmean-SUV and CNRmax-SUV, and on parametric MRFDG images CNRmean-MRFDG and CNRmax-MRFDG.
Statistical analysis
Statistical analysis was performed using SPSS software (version 23.0; IBM Corp., Armonk, NY, USA) and GraphPad Prism 8.0.2 (GraphPad Software, San Diego, CA, USA). All data were presented as mean ± SD or range as appropriate. Paired t-tests or unpaired t-tests were performed to compare two sets of data, and P values less than 0.05 were considered statistically significant.
Results
Patient characteristics and quantitative and semi quantitative FDG uptake in lung cancer lesions
In this study, 27 patients (15 males and 12 females; mean age: 64.67±10.55 years; tumor diameter: 2.94±1.11 cm; 13 adenocarcinomas, 12 squamous carcinomas, and two small cell lung cancer) were enrolled (Table 1) and Patlak reconstruction methods were used to generate good-quality multi-parametric images of the SUV, MRFDG, and DV. The quantitative parameter values of SUVmax (13.16±5.45), SUVpeak (10.36±4.83), SUVmean (7.56±3.52), MRFDGmax (0.26±0.15) µmol·min−1·mL−1, MRFDG-peak (0.19±0.12) µmol·min−1·mL−1, MRFDG-mean (0.14±0.08) µmol·min−1·mL−1, DVmax (165.56%±99.89%), DVpeak (117.66%±72.24%), and DVmean (62.16%±33.65%) were obtained for the lung primary lesions (Table 2 and Figure 4).
Table 1
Patient characteristics | Values |
---|---|
Age (years), mean ± SD | 64.67±10.55 |
The long diameter of the tumor lesion (cm), mean ± SD | 2.94±1.11 |
Sex, n (%) | |
Male | 15 (55.6) |
Female | 12 (44.4) |
Pathological type, n (%) | |
Adenocarcinoma | 13 (48.1) |
Squamous cell carcinoma | 12 (44.4) |
Small cell lung cancer | 2 (7.4) |
SD, standard deviation.
Table 2
Parameters | Median [min–max] | Mean ± SD |
---|---|---|
SUVmax | 12.54 [2.98–20.61] | 13.16±5.45 |
SUVpeak | 10.58 [2.32–18.38] | 10.36±4.83 |
SUVmean | 7.67 [1.68–14.97] | 7.56±3.52 |
MRFDG-max (μmol·min−1·mL−1) | 0.24 [0.07–0.64] | 0.26±0.15 |
MRFDG-peak (μmol·min−1·mL−1) | 0.17 [0.05–0.47] | 0.19±0.12 |
MRFDG-mean (μmol·min−1·mL−1) | 0.12 [0.03–0.34] | 0.14±0.08 |
DVmax (%) | 129.69 [18.92–369.45] | 165.56±99.89 |
DVpeak (%) | 82.89 [8.06–260.75] | 117.66±72.24 |
DVmean (%) | 54.87 [6.60–146.43] | 62.16±33.65 |
SUV, standardized uptake value; MRFDG, metabolic rate of FDG; FDG, fluorodeoxyglucose; DV, Patlak intercept; SD, standard deviation; SUVmax, maximum SUV of target lesion; SUVpeak, peak SUV of target lesion; SUVmean, average SUV of target lesion; MRFDG-max, maximum MRFDG of target lesion; MRFDG-peak, peak MRFDG of target lesion; MRFDG-mean, average MRFDG of target lesion; DVmax, maximum DV of target lesion; DVpeak, peak DV of target lesion; DVmean, average DV of target lesion.
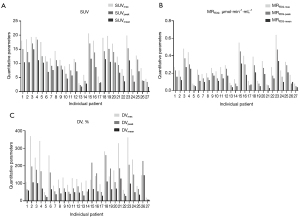
Comparison of dynamic and static PET images of lesions
The maximum and mean values of the objective indicators TBR and CNR were statistically analyzed for 27 primary lesions in MRFDG and SUV images (Table 3). As shown in Figure 5, the maximum and mean values of TBR and CNR of the primary lesion in MRFDG images were significantly higher than SUV images, and the differences were statistically significant (all P<0.05).
Table 3
Objective indicators | Median [min–max] | Mean ± SD |
---|---|---|
TBRmax-SUV | 19.40 [5.14–47.21] | 20.16±9.77 |
TBRmax-MRFDG | 22.00 [8.97–53.33] | 24.36±11.50 |
TBRmean-SUV | 9.47 [3.12–26.26] | 11.32±5.86 |
TBRmean-MRFDG | 12.22 [4.44–28.33] | 12.99±6.29 |
CNRmax-SUV | 61.73 [24.00–142.64] | 64.67±23.44 |
CNRmax-MRFDG | 79.75 [40.50–177.67] | 85.97±29.03 |
CNRmean-SUV | 32.75 [12.30–53.07] | 34.70±14.53 |
CNRmean-MRFDG | 43.33 [15.50–97.67] | 43.78±16.87 |
TBR, target-to-background ratio;TBRmax, maximum value of TBR; TBRmean, average value of TBR; SUV, standardized uptake value; MRFDG, metabolic rate of FDG; FDG, fluorodeoxyglucose; CNR, contrast-to-noise ratio; CNRmean, average value of CNR; CNRmax, maximum value of CNR; SD, standard deviation.
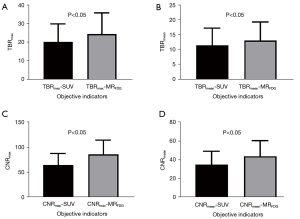
The relationship between MRFDG and clinicopathological characteristics of lung cancer
The relationship between the maximum, peak, and mean values of MRFDG images and the clinicopathological characteristics of lung cancer patients was statistically analyzed (Figure 6). As shown in Table 4, the differences of MRFDG-max, MRFDG-peak, and MRFDG-mean, in different subgroups of lung tumor lesions were statistically significant (all P<0.05) with different lengths and pathological types, and there were higher in the subgroups of tumor lesions ≥3 cm and squamous cell carcinoma of the lung.
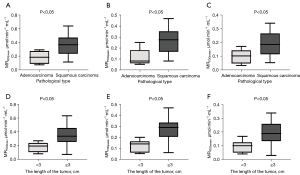
Table 4
Clinicopathologic feature | Classification | n | MRFDG-max (μmol·min−1·mL−1) | MRFDG-peak (μmol·min−1·mL−1) | MRFDG-mean (μmol·min−1·mL−1) | |||||
---|---|---|---|---|---|---|---|---|---|---|
Mean ± SD | P value | Mean ± SD | P value | Mean ± SD | P value | |||||
The diameter of tumor (cm) | <3 | 14 | 0.18±0.07† | 0.0015 | 0.12±0.05† | 0.0003 | 0.10±0.04† | 0.0018 | ||
≥3 | 13 | 0.35±0.16† | 0.27±0.12† | 0.19±0.09† | ||||||
Pathological type | Adenocarcinoma | 13 | 0.17±0.08‡ | 0.0012 | 0.12±0.07‡ | 0.0012 | 0.09±0.05‡ | 0.0021 | ||
Squamous carcinoma | 12 | 0.33±0.17‡ | 0.25±0.13‡ | 0.18±0.09‡ | ||||||
Small cell lung cancer | 2 | 0.28±0.08 | – | 0.22±0.11 | – | 0.16±0.07 | – |
†, P<0.05 (the diameter of tumor <3 vs. ≥3 cm); ‡, P<0.05 (adenocarcinoma vs. squamous carcinoma). MRFDG, metabolic rate of FDG; FDG, fluorodeoxyglucose; MRFDG-max, maximum MRFDG of target lesion; SD, standard deviation; MRFDG-peak, peak MRFDG of target lesion; MRFDG-mean, average MRFDG of target lesion.
Influence of VOI delineation method on quantitative data
We obtained quantitative parameters of the primary lesions in 27 lung cancer patients by different outlining methods (Table 5), the means and ratios of which are shown in Figure 7. Statistical analysis showed that the differences of the maximum and peak values of MRFDG and DV were not statistically significant in the different outlining method subgroups (all P>0.05). However, the difference of the mean values of MRFDG and DV were statistically significant in the different outline method groupings (all P<0.05).
Table 5
Parameters | Method | Median [min–max] | Mean ± SD | P value |
---|---|---|---|---|
MRFDG-max (μmol·min−1·mL−1) | Boundary delineation | 0.24 [0.07–0.64] | 0.26±0.15 | >0.99 |
Contour delineation | 0.24 [0.07–0.64] | 0.26±0.15 | ||
MRFDG-peak (μmol·min−1·mL−1) | Boundary delineation | 0.17 [0.05–0.47] | 0.19±0.12 | >0.99 |
Contour delineation | 0.17 [0.05–0.47] | 0.19±0.12 | ||
MRFDG-mean (μmol·min−1·mL−1) | Boundary delineation | 0.10 [0.02–0.24] | 0.11±0.06* | <0.0001 |
Contour delineation | 0.12 [0.03–0.35] | 0.14±0.08* | ||
DVmax (%) | Boundary delineation | 133.28 [18.92–369.45] | 167.82±100.41 | 0.0852 |
Contour delineation | 129.69 [18.92–369.45] | 165.56±99.89 | ||
DVpeak (%) | Boundary delineation | 82.89 [8.06–260.75] | 116.72±71.67 | 0.1638 |
Contour delineation | 82.89 [8.06–260.75] | 117.66±72.24 | ||
DVmean (%) | Boundary delineation | 42.83 [5.55–125.26] | 49.05±28.10* | <0.0001 |
Contour delineation | 54.87 [6.60–146.43] | 62.16±33.65* | ||
SUVmax | Boundary delineation | 12.54 [2.98–20.61] | 13.16±5.45 | >0.99 |
Contour delineation | 12.54 [2.98–20.61] | 13.16±5.45 | ||
SUVpeak | Boundary delineation | 10.58 [2.32–18.38] | 10.36±4.83 | >0.99 |
Contour delineation | 10.58 [2.32–18.38] | 10.36±4.83 | ||
SUVmean | Boundary delineation | 7.67 [1.68–14.97] | 7.56±3.52* | 0.0007 |
Contour delineation | 9.32 [1.81–26.32] | 9.63±5.30* |
*, P<0.05 (boundary delineation vs. contour delineation). SD, standard deviation; MRFDG-max, maximum MRFDG of target lesion; MRFDG, metabolic rate of FDG; FDG, fluorodeoxyglucose; MRFDG-peak, peak MRFDG of target lesion; MRFDG-mean, average MRFDG of target lesion; DVmax, maximum DV of target lesion; DV, Patlak intercept; DVpeak, peak DV of target lesion; DVmean, average DV of target lesion; SUVmax, maximum SUV of target lesion; SUV, standardized uptake value; SUVpeak, peak SUV of target lesion; SUVmean, average SUV of target lesion.
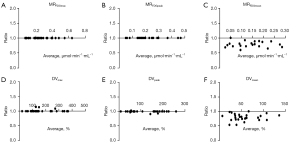
Discussion
In the present study, we used the direct Patlak parametric model to reconstruct multi-parametric images derived from the 0–75-min dynamic scans, which resulted in multi-parametric images that were clearly less noisy than the parametric images produced by the traditional indirect image-based approach. Multi-parameter images of SUV, MRFDG, and DV can be obtained through reconstruction. After that, the lesions can be delineated, so as to obtain the overall max, mean, and peak value of SUV, MRFDG, and DV of lung cancer lesions. The new parametric images in this study complement the static SUV images, which adds more data to support lung cancer diagnoses. Our study contributes to the growing body of literature on quantitative PET imaging in oncology. By specifically investigating the impact of delineation methods on lung cancer assessment, we provide valuable insights into the nuances of utilizing the Patlak multi-parametric analysis method for this purpose.
Whole-body dynamic multi-parameter PET imaging is a recently developed technique in nuclear medicine. In this study, the multi-parametric PET images were of great visual quality. The objective parameters of SUV images and MRFDG images were compared and analyzed by paired t-test. The results indicated that the TBR and CNR of MRFDG images were both higher than those of the SUV images (P<0.05). This feature of MRFDG images is consistent with the previous study (14,17). It has been reported that multi-parameter PET imaging contains more tracer dynamics information and is therefore less susceptible to the limitations associated with static SUV imaging, such as signal differences caused by acquisition time, blood glucose levels, and population heterogeneity (19-21). Higher TBR and CNR indicates better lesion detectability, thus MRFDG images have better lesion detectability than SUV images. Although quantitative parameters image characteristics can theoretically facilitate lesion detectability, it is prudent to acknowledge that the discovery of additional lesions is contingent on multiple factors, beyond just image improvements. Our study echoed this trend, and we appreciate the clarification that this phenomenon is not unique to our investigation.
In this study, the differences of MRFDG-max, MRFDG-peak and MRFDG-mean, in different subgroups of lung tumor lesions were statistically significant with different lengths and pathological types, and there were higher in the subgroups of tumor lesions ≥3 cm and squamous cell carcinoma of the lung. Tan et al. (22) also found that lung cancer lesions with different diameters have different PET metabolic parameters, and SUV values have important value in predicting tumor size. Schuurbiers et al. (23) proposed that lung adenocarcinomas undergo glycolysis under normoxic conditions, whereas squamous cell carcinoma has a very high anaerobic glycolysis rate. A previous study (24) showed that the metabolic rate of lung squamous cell carcinoma is significantly higher than that of lung adenocarcinoma. Glucose transporters (GLUTs) are associated with FDG uptake in lung cancer cells, and GLUT expression in lung squamous cell carcinoma is higher than that in lung adenocarcinoma (25,26).
It is well known that different delineation methods influence the quantitative parameters. The threshold delineation and the shape delineation are commonly used in the clinic. However, each delineation method has its own advantages and limitations (27). The threshold delineation method is widely used in lung cancer and can be performed quickly and consistently, with little variability between delineators, but the volume of the lesion is not fully delineated by ensuring that the threshold used for delineation is higher than the average background value, taking into account background absorption. In fact, the simple method involving selection of a fixed threshold failed to provide an accurate estimation of the tumor volume (28). The shape delineation method can completely delineate the lesion volume, but it often overestimates the lesion volume, including a large proportion of the background area in the delineation. The more the lesion shape deviates from the sphere, the more inaccurate the delineation results, and the greater the variability between different delineators. The large inter-observer variation in the gross tumor volume definition jeopardizes comparisons between clinicians, institutes, and treatments (29,30). The results of this study found that the two types of delineation method had less influence on the max and peak values of the quantitative parameters. Furthermore, in both delineation methods, only in individual DV figures did deviation appear. This is mainly because DV is more sensitive to the influence of movement, and the lesion position may be mismatched (31). During the post-processing phase, manual shifting should be performed to make them match. In the present study, the use of a different delineation method had a great influence on the mean value of the quantitative parameters (P<0.05). The possible cause is that the tumor volume will be overestimated via shape delineation method and underestimated via threshold delineation method. In clinical practice, we need to select the suitable delineation method according to the objective. Both delineation methods are acceptable if only the max and peak values of the quantitative parameters will be studied. Meanwhile, if the mean value of quantitative parameters needs to be considered, we must select the delineation method as required. For example, it is better to use the threshold delineation method in radiation therapy studies, because the aim is to describe the extent of the tumor as accurately as possible to avoid unnecessary irradiation of healthy tissue. Meanwhile, it is better to use the shape delineation method in tumor response assessment and to accept any tendency to overestimate the true volume.
This study had several limitations including the small sample size and single center study. In addition, the dynamic PET scan protocol and reconstruction method deserve to be optimized and further discussed. Moreover, it was only a preliminary exploration of multi-parameter imaging of lung cancer, and further exploration of its value in differential diagnosis and staging is required. We are currently collecting cases for future studies.
Conclusions
This study demonstrated that multi-parametric dynamic whole-body PET scanning helps to obtain quantitative estimates of glucose metabolism in lung cancer lesions with high TBR and CNR. The multi-parameters are various from different pathology types to tumor size. Different delineation methods were found to have a great influence on MRFDG-mean and DVmean. This study provides reference data and guidance on the delineation method for further research on lung cancer in the later stage.
Acknowledgments
We thank the staff members of the Department of Nuclear Medicine, The First Affiliated Hospital of Anhui Medical University for excellent technical support.
Funding: This study was supported by
Footnote
Conflicts of Interest: All authors have completed the ICMJE uniform disclosure form (available at https://qims.amegroups.com/article/view/10.21037/qims-23-862/coif). All authors report that this research was funded by the National Natural Science Foundation of China (No. 81801736). The authors have no other conflicts of interest to declare.
Ethical Statement: The authors are accountable for all aspects of the work in ensuring that questions related to the accuracy or integrity of any part of the work are appropriately investigated and resolved. This study was conducted in accordance with the Declaration of Helsinki (as revised in 2013). The study protocol was approved by the Clinical Medical Research Ethics Committee of The First Affiliated Hospital of Anhui Medical University (PJ2019-15-04) and informed consent was provided by all individual participants.
Open Access Statement: This is an Open Access article distributed in accordance with the Creative Commons Attribution-NonCommercial-NoDerivs 4.0 International License (CC BY-NC-ND 4.0), which permits the non-commercial replication and distribution of the article with the strict proviso that no changes or edits are made and the original work is properly cited (including links to both the formal publication through the relevant DOI and the license). See: https://creativecommons.org/licenses/by-nc-nd/4.0/.
References
- Siegel RL, Miller KD, Fuchs HE, Jemal A. Cancer statistics, 2022. CA Cancer J Clin 2022;72:7-33. [Crossref] [PubMed]
- Kitajima K, Doi H, Kanda T, Yamane T, Tsujikawa T, Kaida H, Tamaki Y, Kuribayashi K. Present and future roles of FDG-PET/CT imaging in the management of lung cancer. Jpn J Radiol 2016;34:387-99. [Crossref] [PubMed]
- Rahmim A, Lodge MA, Karakatsanis NA, Panin VY, Zhou Y, McMillan A, Cho S, Zaidi H, Casey ME, Wahl RL. Dynamic whole-body PET imaging: principles, potentials and applications. Eur J Nucl Med Mol Imaging 2019;46:501-18. [Crossref] [PubMed]
- Zaker N, Kotasidis F, Garibotto V, Zaidi H. Assessment of Lesion Detectability in Dynamic Whole-Body PET Imaging Using Compartmental and Patlak Parametric Mapping. Clin Nucl Med 2020;45:e221-31. [Crossref] [PubMed]
- Li EJ, Spencer BA, Schmall JP, Abdelhafez Y, Badawi RD, Wang G, Cherry SR. Efficient Delay Correction for Total-Body PET Kinetic Modeling Using Pulse Timing Methods. J Nucl Med 2022;63:1266-73. [Crossref] [PubMed]
- Liu G, Xu H, Hu P, Tan H, Zhang Y, Yu H, Li X, Shi H. Kinetic metrics of (18)F-FDG in normal human organs identified by systematic dynamic total-body positron emission tomography. Eur J Nucl Med Mol Imaging 2021;48:2363-72. [Crossref] [PubMed]
- Dias AH, Hansen AK, Munk OL, Gormsen LC. Normal values for (18)F-FDG uptake in organs and tissues measured by dynamic whole body multiparametric FDG PET in 126 patients. EJNMMI Res 2022;12:15. [Crossref] [PubMed]
- Liu G, Yu H, Shi D, Hu P, Hu Y, Tan H, Zhang Y, Yin H, Shi H. Short-time total-body dynamic PET imaging performance in quantifying the kinetic metrics of (18)F-FDG in healthy volunteers. Eur J Nucl Med Mol Imaging 2022;49:2493-503. [Crossref] [PubMed]
- Dias AH, Smith AM, Shah V, Pigg D, Gormsen LC, Munk OL. Clinical validation of a population-based input function for 20-min dynamic whole-body (18)F-FDG multiparametric PET imaging. EJNMMI Phys 2022;9:60. [Crossref] [PubMed]
- Wang H, Miao Y, Yu W, Zhu G, Wu T, Zhao X, Yuan G, Li B, Xu H. Improved Clinical Workflow for Whole-Body Patlak Parametric Imaging Using Two Short Dynamic Acquisitions. Front Oncol 2022;12:822708. [Crossref] [PubMed]
- Wang D, Zhang X, Liu H, Qiu B, Liu S, Zheng C, Fu J, Mo Y, Chen N, Zhou R, Chu C, Liu F, Guo J, Zhou Y, Zhou Y, Fan W, Liu H. Assessing dynamic metabolic heterogeneity in non-small cell lung cancer patients via ultra-high sensitivity total-body [18F]FDG PET/CT imaging: quantitative analysis of [18F]FDG uptake in primary tumors and metastatic lymph nodes. Eur J Nucl Med Mol Imaging 2022;49:4692-704.
- Sundaraiya S, T R, Nangia S, Sirohi B, Patil S. Role of dynamic and parametric whole-body FDG PET/CT imaging in molecular characterization of primary breast cancer: a single institution experience. Nucl Med Commun 2022;43:1015-25. [Crossref] [PubMed]
- Huang X, Zhuang M, Yang S, Wang Y, Liu Q, Xu X, et al. The valuable role of dynamic (18)F FDG PET/CT-derived kinetic parameter K(i) in patients with nasopharyngeal carcinoma prior to radiotherapy: A prospective study. Radiother Oncol 2023;179:109440. [Crossref] [PubMed]
- Fahrni G, Karakatsanis NA, Di Domenicantonio G, Garibotto V, Zaidi H. Does whole-body Patlak (18)F-FDG PET imaging improve lesion detectability in clinical oncology? Eur Radiol 2019;29:4812-21. [Crossref] [PubMed]
- Ralli GP, Carter RD, McGowan DR, Cheng WC, Liu D, Teoh EJ, Patel N, Gleeson F, Harris AL, Lord SR, Buffa FM, Fenwick JD. Radiogenomic analysis of primary breast cancer reveals [18F]-fluorodeoxglucose dynamic flux-constants are positively associated with immune pathways and outperform static uptake measures in associating with glucose metabolism. Breast Cancer Res 2022;24:34.
- Cai K, Zhang Q, Wang H, Yu W, Xue Y, Xu H. Value of whole-body dynamic (18)F-FMISO PET/CT Patlak multi-parameter imaging for evaluating the early radiosensitizing effect of oleanolic acid on C6 rat gliomas. Cancer Chemother Pharmacol 2023;91:133-41. [Crossref] [PubMed]
- Dias AH, Pedersen MF, Danielsen H, Munk OL, Gormsen LC. Clinical feasibility and impact of fully automated multiparametric PET imaging using direct Patlak reconstruction: evaluation of 103 dynamic whole-body (18)F-FDG PET/CT scans. Eur J Nucl Med Mol Imaging 2021;48:837-50. [Crossref] [PubMed]
- Di Stasio GD, Travascio L, Colandrea M, Spaggiari L, Sorbello S, Ferrari ME, Maisonneuve P, Galetta D, Travaini L, Grana CM. Prognostic value of PET parameters in patients with pleomorphic lung cancer: Results from a single institution. Lung Cancer 2021;158:40-6. [Crossref] [PubMed]
- Fukukita H, Suzuki K, Matsumoto K, Terauchi T, Daisaki H, Ikari Y, Shimada N, Senda M. Japanese guideline for the oncology FDG-PET/CT data acquisition protocol: synopsis of Version 2.0. Ann Nucl Med 2014;28:693-705. [Crossref] [PubMed]
- Fayolle H, Jehanno N, Lauwers-Cances V, Castex MP, Orbach D, Mognetti T, Nadège C, Payoux P, Hitzel A. PET metabolic tumor volume as a new prognostic factor in childhood rhabdomyosarcoma. PLoS One 2022;17:e0261565. [Crossref] [PubMed]
- Debus C, Afshar-Oromieh A, Floca R, Ingrisch M, Knoll M, Debus J, Haberkorn U, Abdollahi A. Feasibility and robustness of dynamic (18)F-FET PET based tracer kinetic models applied to patients with recurrent high-grade glioma prior to carbon ion irradiation. Sci Rep 2018;8:14760. [Crossref] [PubMed]
- Tan H, Ma M, Huang J, Zhu W, Hu S, Zheng K, Rong P. Glucose Metabolism Reprogramming of Primary Tumor and the Liver Is Associated With Disease-Free Survival in Patients With Early NSCLC. Front Oncol 2021;11:752036. [Crossref] [PubMed]
- Schuurbiers OC, Meijer TW, Kaanders JH, Looijen-Salamon MG, de Geus-Oei LF, van der Drift MA, van der Heijden EH, Oyen WJ, Visser EP, Span PN, Bussink J. Glucose metabolism in NSCLC is histology-specific and diverges the prognostic potential of 18FDG-PET for adenocarcinoma and squamous cell carcinoma. J Thorac Oncol 2014;9:1485-93. [Crossref] [PubMed]
- Messerli M, Kotasidis F, Burger IA, Ferraro DA, Muehlematter UJ, Weyermann C, Kenkel D, von Schulthess GK, Kaufmann PA, Huellner MW. Impact of different image reconstructions on PET quantification in non-small cell lung cancer: a comparison of adenocarcinoma and squamous cell carcinoma. Br J Radiol 2019;92:20180792. [Crossref] [PubMed]
- Choi WH, Yoo IeR. O JH, Kim TJ, Lee KY, Kim YK. Is the Glut expression related to FDG uptake in PET/CT of non-small cell lung cancer patients? Technol Health Care 2015;23:S311-8. [Crossref] [PubMed]
- Na KJ, Choi H, Oh HR, Kim YH, Lee SB, Jung YJ, Koh J, Park S, Lee HJ, Jeon YK, Chung DH, Paeng JC, Park IK, Kang CH, Cheon GJ, Kang KW, Lee DS, Kim YT. Reciprocal change in Glucose metabolism of Cancer and Immune Cells mediated by different Glucose Transporters predicts Immunotherapy response. Theranostics 2020;10:9579-90. [Crossref] [PubMed]
- Suzawa N, Ito M, Qiao S, Uchida K, Takao M, Yamada T, Takeda K, Murashima S. Assessment of factors influencing FDG uptake in non-small cell lung cancer on PET/CT by investigating histological differences in expression of glucose transporters 1 and 3 and tumour size. Lung Cancer 2011;72:191-8. [Crossref] [PubMed]
- Ceriani L, Milan L, Johnson PWM, Martelli M, Presilla S, Giovanella L, Zucca E. Baseline PET features to predict prognosis in primary mediastinal B cell lymphoma: a comparative analysis of different methods for measuring baseline metabolic tumour volume. Eur J Nucl Med Mol Imaging 2019;46:1334-44. [Crossref] [PubMed]
- Wang XY, Zhao YF, Liu Y, Yang YK, Zhu Z, Wu N. Comparison of different automated lesion delineation methods for metabolic tumor volume of 18F-FDG PET/CT in patients with stage I lung adenocarcinoma. Medicine (Baltimore) 2017;96:e9365. [Crossref] [PubMed]
- Haga A, Takahashi W, Aoki S, Nawa K, Yamashita H, Abe O, Nakagawa K. Classification of early stage non-small cell lung cancers on computed tomographic images into histological types using radiomic features: interobserver delineation variability analysis. Radiol Phys Technol 2018;11:27-35. [Crossref] [PubMed]
- Kaji T, Osanai K, Nakata T, Tamaki N. Dynamic Whole-Body 18F-FDG PET for Minimizing Patient Motion Artifact. Clin Nucl Med 2020;45:880-2. [Crossref] [PubMed]