Mono+ algorithm assessment of the diagnostic value of dual-energy CT for high-risk factors for colorectal cancer: a preliminary study
Introduction
Colorectal cancer (CRC) accounts for approximately 10% of diagnosed cancers and cancer-related deaths worldwide each year, has the second highest mortality rate, with a prognosis influenced by tumor node metastasis (TNM) stage and other high-risk factors, and has 5-year survival rates ranging from 10% to 90% (1-3). High-risk factors for CRC include lymph node metastasis, extramural vascular invasion (EMVI), peripheral nerve invasion (PNI), high-grade tumors (including poorly differentiated adenocarcinomas and undifferentiated carcinomas, both less than 50% gland formation) T4 stage, and tumor deposits (4,5). For high-risk patients, the recommended duration of adjuvant therapy is 6 months if FOLFOX is chosen, while for the low-risk group, the recommended duration of adjuvant therapy is 3 months if the CapeOX chemotherapy regimen is chosen (6). In addition, patients with stage-II high-risk colon cancer who undergo routine standard management may have a worse prognosis than patients with stage-III low-risk colon cancer (7). Thus, screening the high-risk factors for CRC is important for the selection and timing of adjuvant chemotherapy regimens.
Histopathology is the gold standard for the diagnosis of high-risk factor CRC. However, biopsy is invasive, and the results can be falsely negative due to the site and depth of the tumor. Thus, an accurate, objective and non-invasive preoperative method for evaluating high-risk factors for CRC is needed.
Computerized tomography (CT) is one of the most important preoperative examinations for CRC. Compared to magnetic resonance imaging (MRI), CT has a faster imaging time and a better ability to detect distant metastases (8), but conventional CT provides only limited information to accurately assess high-risk factors, such as EMVI, lymph node metastasis, and tumor deposits.
Dual-energy computerized tomography (DECT), which uses both high and low energies to achieve material decomposition and material classification, has the ability to assess the biological behavior of tumors by CT (9,10). Current applications of DECT in CRC include determining the nature of the lymph nodes, pathological staging, and histological grading (11,12). A recent study demonstrated the diagnostic value of DECT for the EMVI of rectal cancer (13). These previous studies focused on only one high-risk factor for CRC, but in clinical settings, patients may actually have one or more high-risk factors, and the preoperative predictive value of DECT for these patients remains unknown.
Recently, the quantitative parameters of DECT have been a major advantage in tumor imaging, and the introduction of the Mono+ algorithm has pushed this to new heights. Mono+ is an algorithm that uses frequency division to superimpose the low spatial frequencies of a 40 keV image with the high spatial frequencies of a 70 keV image to improve the image noise at low keV in virtual monoenergetic imaging (VMI) mode, thus improving the contrast-to-noise ratio (CNR) of the image (14). Compared to standard linear reconstruction, VMI based on the Mono+ algorithm (VMI+) reduces image noise while improving iodine attenuation, especially at low kilo-electron volt (keV), which has been reported to improve the reliability and diagnostic accuracy of the DECT quantitative size measurements used to assess colorectal liver metastases (CRLMs) (15). Compared to conventional VMI, VMI+ has proven to be superior in qualitative and quantitative image analysis of cutaneous malignant melanomas, abdominal malignant lymphomas, and CRLMs (16-18). However, the quality of the qualitative and quantitative images based on VMI+ of CRC is unknown.
This study had two main objectives. First, it sought to assess the optimal energy level for the qualitative and quantitative analysis of CRC based on the Mono+ algorithm. Second, it sought to analyze the tumor quantitative DECT parameters based on the optimal image and constructed a regression model with clinical factors to evaluate the diagnostic value for high-risk CRC. We present this article in accordance with the STROBE reporting checklist (available at https://qims.amegroups.com/article/view/10.21037/qims-23-291/rc).
Methods
Patients
This prospective study was conducted in accordance with the Declaration of Helsinki (as revised in 2013). The Ethics Committee of the Second Affiliated Hospital of Chongqing Medical University approved the study and waived the requirement for informed consent because the study did not adversely affect the rights and health of the subjects.
Between April 2021 to January 2023, the clinical and imaging data of 265 patients diagnosed with CRC were prospectively analyzed at The Second Affiliated Hospital of Chongqing Medical University. Sample size calculations were based on a small sample pre-experiment [venous phase normalized iodine concentration (VP-NIC) of 40.25±7.29 vs. 46.23±7.47 for the high- and low-risk groups] with 90% power at a two-sided alpha level of 0.05. Based on these results, about 34 cases were needed in each of the high- and low-risk groups. Ultimately, 74 patients (a total of 75 tumors) were included in this study, including 41 in the high-risk group and 33 in the low-risk group (Figure 1).
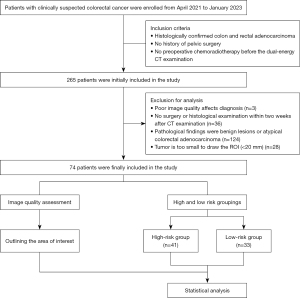
Based on the pathology of biopsy samples or postoperative lesion samples, the patients in this study were allocated to the high-risk group if they had ≥1 of the following risk factors: lymph node metastasis, EMVI, PNI, high-grade tumors, stage T4, stage M1, and tumor deposits. While the patients were allocated to the low-risk group if they had no risk factors.
To be eligible for inclusion in this study, the patients had to meet the following inclusion criteria: (I) have histologically confirmed colon and rectal adenocarcinoma; (II) have no history of pelvic surgery; and (III) not have undergone preoperative chemoradiotherapy before the dual-energy CT examination. Patients were excluded from the study if they met any of the following exclusion criteria: (I) had poor quality images that affected diagnosis; (II) had not undergone surgery or a histological examination within two weeks of the CT examination; (III) had benign lesions or atypical colorectal adenocarcinoma based on the pathological findings; and/or (IV) had tumors that were too small (i.e., <20 mm) to draw regions of interest (ROIs).
DECT acquisition
Patients were asked not to take drugs containing heavy metals, such as barium, for a week before the CT scan, and were asked to fast 12 hours before the CT examination and drink 600–1,000 mL of water 10 minutes before the examination. All the examinations were performed on a dual-energy CT system (Drive, Siemens Healthcare, Germany). Two contrast-enhanced CT scans were performed from the apex of the diaphragm to the inferior margin of the pubic symphysis in dual-energy spectral CT imaging mode. The scan parameters were as follows: tube voltages: 100 and 140 kV; tube current: 350 mA; pitch: 1; and gantry rotation time: 0.5 s. The contrast agent (Ultravist, 370 mg/mL, Schering, Berlin, Germany; dose: 1.5 mL/kg, flow rate: 3 mL/s) was injected through an elbow vein using a double-head power injector. The scan was threshold triggered, with the arterial phase (AP) automatically starting after a delay of 15 s when the abdominal aortic threshold reached 120 HU, and with the VP automatically starting after a delay of 17 s.
DECT image reconstruction
All the DECT data sets were postprocessed on a commercially available three-dimensional (3D) multimodality workstation (syngo.via, version VA30A, Siemens) using a dedicated soft tissue convolution kernel (Qr40, Siemens) and an iterative reconstruction technique (ADMIRE, Siemens; strength level, 3). Standard linear mixed images were automatically reconstructed using a mixing factor of 0.6 (M0.6, containing 60% 100 keV low tube voltage, and 40% 140 keV high tube voltage) (19). Given the low attenuation of iodine concentration (IC) at energy levels above 100 keV in previous studies, for the image quality evaluation part of this study, the energy-level range was set at 40–100 keV in 10 keV increments (20).
Quantitative image analysis
The quantitative image analysis was performed by a radiologist with three years of experience in abdominal radiodiagnosis on a Siemens syngo MMWP VE36A workstation. The location of the tumor was confirmed according to the histopathological results. Three circular ROIs with areas of 20–30 mm2 were placed in the largest axial image of the tumor, avoiding necrotic foci and larger vessels, and the final value was the average of these three ROIs. Defining fat standard deviation (SD) as image noise, three circular ROIs with an area of 100 mm2 were placed in the subcutaneous fat on the same slice of the outlined tumor, and the final value was averaged over the three ROIs. Based on previous studies, the signal-to-noise ratio (SNR) and CNR were calculated as follows (16):
Qualitative image analysis
The qualitative image analysis was performed by two radiologists with three and four years of experience in abdominal radiology, respectively. The observers were only aware of the patients’ clinical information and were not aware of the image reconstruction algorithm. The standard linear hybrid images (M0.6) and noise-optimized VMI+ reconstructed images were rated randomly, and only one random energy level was assessed during each round of evaluation. Based on a five-point Likert scale, the following quality criteria were assessed: overall image quality (where 1 = poor overall image quality and 5 = excellent overall image quality), lesion delineation (where 1 = unable to exclude lesions and 5 = excellent lesion margins), and image noise (where 1 = very pronounced image noise and 5 = barely detectable image noise).
Imaging analysis
All the images were individually analyzed by two radiologists with more than 3 years of experience each in abdominal radiology who outlined the ROIs based on the histological examination as a reference. Duplex-enhanced images with a slice thickness of 1 mm were imported into the workstation and opened in dual-energy mode to automatically obtain virtual single-energy images, iodine-based material decomposition images, and effective atomic number images in the range of 40–150 keV. The localization of the lesions and outlining of the ROIs were performed with reference to 60 keV VMI+ on a 40 keV VMI+ single-energy image. Three ROIs of 20–30 mm2 were placed in the largest axis image of the tumor, avoiding large vessels and necrotic lesions, and selecting areas of uniform and distinct enhancement. The final value was the average of these three ROIs. The previously outlined ROIs were then copied to the iodine-based material breakdown image and the effective atomic number image. The final IC, NIC, effective atomic number, electron cloud density, dual-energy index (DEI), and slope of the energy spectrum in the AP and VP were recorded; the NIC of the tumor was obtained by normalizing the IC of the tumor to the IC of the aorta or iliac artery at the same level. The differential iodine concentration (DIC) in the arteriovenous phase was calculated using the following formula: DIC = (AP – VP) IC. The dual-energy curve slope value (λHU) was calculated from the CT values of the 50 and 140 keV virtual monochrome images {λHU = [CT50 value (50 keV) – CT140 value (140 keV)]/[140–50]}.
Statistical analysis
The statistical analysis was performed using MedCalc Version 19.2 (Ostende, Belgium) and GraphPad Prism Version 9.4.1 (San Diego, California, USA). The intraclass correlation coefficient (ICC) was used to evaluate the interobserver agreement of the measured parameters (ICC <0.40 = poor agreement, ICC 0.40–0.59 = fair agreement, ICC 0.60–0.74 = good agreement, and ICC 0.75–1.0 = excellent agreement) (18).
The quantitative variables are expressed as the mean ± SD, and the ordinal variables are expressed as median values. The Kolmogorov-Smirnov test was used to test the normality of the data distribution. For multiple comparisons, an analysis of variance was used for data conforming to a Gaussian distribution. For comparisons between two data sets, the independent samples t-test was used for data conforming to a Gaussian distribution; otherwise, the Mann-Whitney U test was used. In this study, all P values were two-sided in the test, and a P value <0.05 was considered statistically significant. A single-factor logistic regression analysis was used for variable screening, and variables with P<0.1 were included in the multifactor regression analysis to construct regression models for predicting risk factors for CRC. Receiver operating characteristic (ROC) curves were plotted, and the areas under the curve (AUCs) were calculated to evaluate the diagnostic effect of the DECT parameters and regression models.
Results
Clinical-pathological characteristics
Ultimately, 74 patients were enrolled in the study, of whom 41 were allocated to the high-risk group (41 tumors) and 33 were allocated to the low-risk group (34 tumors). Each specific high-risk factor included T4 stage (n=14), N1 (excluding N1c) (n=17), N2 (n=8), tumor deposition (n=13), M1 (n=5), high-grade tumors (n=6), EMVI-positive (n=9), and PNI-positive (n=14); TNM staging was based on the 8th edition of the American Joint Committee on Cancer Staging Systems. The proportion of people with carcinoembryonic antigen (CEA) concentrations above 5 ng/mL was significantly higher in the high-risk group than the low-risk group (31.71 vs. 6.06; P=0.008). In relation to histological grade, the proportion of high-grade tumors in the high-risk group was also significantly higher than that of the low-risk group (P=0.030). There were no statistically significant differences in the other baseline characteristics between the two groups (Table 1).
Table 1
Clinicopathological characteristics | High-risk group (n=41) | Low-risk group (n=33) | P value |
---|---|---|---|
Age (years), mean ± SD | 63.63±9.58 | 62.71±9.78 | 0.861† |
Sex, n (%) | 0.858§ | ||
Male | 24 (58.54) | 20 (60.61) | |
Female | 17 (41.46) | 13 (39.39) | |
Area (mm2), mean ± SD | 7.80±5.50 | 6.44±3.68 | 0.275‡ |
Tumor location, n (%) | 0.218§ | ||
Right hemicolon | 4 (9.76) | 6 (18.18) | |
Left hemicolon | 9 (21.95) | 11 (33.33) | |
Rectal | 28 (68.29) | 16 (48.48) | |
CEA level, n (%) | 0.008¶ | ||
<5 ng/mL | 28 (68.29) | 31 (93.94) | |
≥5 ng/mL | 13 (31.71) | 2 (6.06) | |
Histological grade, n (%) | 0.030¶ | ||
High | 6 (14.63) | 0 (0) | |
Lowe | 35 (85.37) | 33 (100.00) | |
T4 stage, n (%) | 14 (34.15) | ||
N1 (excluding N1c), n (%) | 17 (41.46) | ||
N2, n (%) | 8 (19.51) | ||
Tumor deposits, n (%) | 13 (31.71) | ||
M1, n (%) | 5 (12.20) | ||
EMVI, n (%) | 9 (21.95) | ||
PNI, n (%) | 14 (34.15) |
†, independent samples t-test; ‡, Mann-Whitney U test; §, chi-square test; ¶, Fisher’s exact test. CRC, colorectal cancer; SD, standard deviation; CEA, carcinoembryonic antigen; EMVI, extramural vascular invasion; PNI, peripheral nerve invasion.
Quantitative image analysis
The results of all the quantitative analyses are shown in Table 2 and Figure 2. The mean signal attenuation of the CRC lesions was highest at 40 keV VMI+ (251.01±33.70 HU) and 89.36±11.59 HU for the standard linearly mixed M0.6 series. Compared to the standard linear mixed M0.6 sequence (SNR, 5.86±0.64; CNR, 11.98±1.09), there was a significant increase in both the SNR and CNR at 40 keV VMI+ (SNR, 8.79±1.27; CNR, 14.89±1.77).
Table 2
Parameters | M0.6 | 40 keV VMI+ | 50 keV VMI+ | 60 keV VMI+ | 70 keV VMI+ | 80 keV VMI+ | 90 keV VMI+ | 100 keV VMI+ |
---|---|---|---|---|---|---|---|---|
Attenuation average | 89.36±11.59 | 251.01±33.70 | 176.77±22.52 | 132.38±15.91 | 103.80±12.09 | 85.44±9.95 | 73.01±8.72 | 64.54±8.20 |
SNR average | 5.86±0.64 | 8.79±1.27 | 8.01±1.11 | 7.33±1.06 | 6.83±1.25 | 6.22±0.99 | 5.77±0.97 | 5.32±0.93 |
CNR average | 11.98±1.09 | 14.89±1.77 | 14.25±1.71 | 13.73±1.82 | 13.36±1.93 | 13.01±2.02 | 12.66±2.08 | 12.17±2.05 |
Data are shown as the mean ± standard deviation. HU, Hounsfield unit; SNR, signal-to-noise; CNR, contrast-to-noise ratio; VMI+, virtual monoenergetic imaging based on Mono+ algorithm.
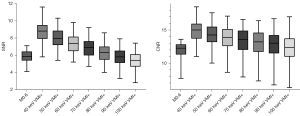
Qualitative parameter analysis
The mean values of the qualitative image analysis are summarized in Table 3. In relation to overall image quality, the 60 keV VMI+ series images had the highest subjective image quality scores [median: 5; ICC =0.84; 95% confidence interval (CI): 0.75–0.89], which were significantly higher than those of the other energy-level series and standard linear mixed M0.6 sequence images (median: 3; ICC =0.80; 95% CI: 0.71–0.87; P<0.001). In relation to lesion delineation, the 40 keV VMI+ series images had the highest lesion delineation definition scores (median: 5; ICC =0.72; 95% CI: 0.59–0.82), which were significantly higher than those of the other energy series and the standard linear mixed M0.6 sequence images (median: 3; ICC =0.70; 95% CI: 0.56–0.80; P<0.001). In relation to image noise, the 40 keV VMI+ series images had the highest image noise scores (median: 5; ICC =0.78; 95% CI: 0.67–0.86), which were significantly higher than those of the other energy series and the standard linear mixed M0.6 sequence images (median: 4; ICC =0.83; 95% CI: 0.73–0.89; P<0.001). A comparison of the images across the different sequences is shown in Figure 3.
Table 3
Qualitative parameters | M0.6 | 40 keV VMI+ | 50 keV VMI+ | 60 keV VMI+ | 70 keV VMI+ | 80 keV VMI+ | 90 keV VMI+ | 100 keV VMI+ |
---|---|---|---|---|---|---|---|---|
Overall image quality | 3 [2–5] | 4 [3–5] | 4 [3–5] | 5 [4–5] | 4 [3–5] | 3 [2–5] | 3 [2–4] | 3 [1–4] |
Lesion delineation | 3 [2–4] | 5 [4–5] | 4 [3–5] | 4 [2–5] | 4 [2–5] | 3 [2–4] | 3 [1–4] | 2 [1–4] |
Image noise | 4 [2–5] | 3 [2–5] | 4 [3–5] | 4 [3–5] | 4 [3–5] | 4 [3–5] | 4 [3–5] | 5 [4–5] |
Data are shown as the median [range]. VMI+, virtual monoenergetic imaging based on Mono+ algorithm.
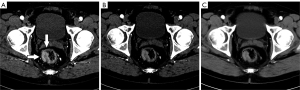
DECT characteristics of high- and low-risk factors for CRC
There was good agreement between the two observers on the measured parameters of DECT in CRC patients (ICC >0.6), and all the measurements are summarized in Table 4. The final values of the DECT parameters of the high- and low-risk groups in the AP and VP are shown in Table 5. Patients in the high-risk group had significantly higher AP-IC (3.09±0.17 vs. 2.80±0.14 mg/mL, P<0.001), AP-NIC (22.30±4.77 vs. 19.65±2.84, P=0.005), AP-DEI [(21.72±1.75)×10–3vs. (19.85±2.05)×10–3, P<0.001], AP-λHU (1.49±0.19 vs. 1.39±0.19, P=0.029), DIC (0.75±0.24 vs. 0.35±0.22, P<0.001), and VP-NIC (41.03±7.09 vs. 47.13±7.19, P<0.001) than those in the low-risk group, while the differences in the other DECT parameters were not statistically significant (Table 5). Figures 4,5 show the images of low- and high-risk CRC.
Table 4
Parameters | IC | NIC | Z | Rho | DEI | λHU |
---|---|---|---|---|---|---|
Arterial phase | ||||||
ICC | 0.82 | 0.66 | 0.92 | 0.84 | 0.88 | 0.88 |
95% confidence interval | 0.73–0.88 | 0.51–0.77 | 0.87–0.95 | 0.75–0.89 | 0.82–0.93 | 0.81–0.92 |
Venous phase | ||||||
ICC | 0.73 | 0.71 | 0.91 | 0.75 | 0.84 | 0.87 |
95% confidence interval | 0.60–0.82 | 0.58–0.81 | 0.86–0.94 | 0.63–0.83 | 0.76–0.90 | 0.80–0.91 |
IC, iodine concentration; NIC, normalized iodine concentration; Z, effective atom number; Rho, electron density; DEI, dual–energy index; λHU, dual-energy curve slope value; ICC, intragroup correlation coefficient.
Table 5
DECT parameters | High-risk group (n=41) | Low-risk group (n=34) | t/Z value | P value |
---|---|---|---|---|
Arterial phase | ||||
IC (mg/mL) | 3.09±0.17 | 2.80±0.14 | 6.77 (Z) | <0.001 |
NIC (%) | 22.30±4.77 | 19.65±2.84 | 2.79 (Z) | 0.005 |
Z | 8.86±0.16 | 8.80±0.16 | −1.59 (t) | 0.116 |
Rho | 41.20±6.89 | 41.35±6.21 | 0.28 (Z) | 0.782 |
DEI (×10–3) | 21.72±1.75 | 19.85±2.05 | 3.77 (Z) | <0.001 |
λHU | 1.49±0.19 | 1.39±0.19 | −2.23 (t) | 0.029 |
Venous phase | ||||
IC (mg/mL) | 2.35±0.27 | 2.45±0.24 | 1.72 (t) | 0.090 |
NIC (%) | 41.03±7.09 | 47.13±7.19 | −3.71 (Z) | <0.001 |
Z | 8.64±0.18 | 8.67±0.17 | 0.71 (t) | 0.480 |
Rho | 40.75±5.43 | 39.09±5.20 | 0.97 (Z) | 0.330 |
DEI (×10–3) | 16.85±2.65 | 17.28±2.42 | 0.72 (t) | 0.470 |
λHU | 1.24±0.20 | 1.23±0.19 | −0.17 (t) | 0.867 |
DIC (mg/mL) | 0.75±0.24 | 0.35±0.22 | −7.35 (t) | <0.001 |
Data are shown as the mean ± SD. CRC, colorectal cancer; DECT, dual-energy computed tomography; IC, iodine concentration; NIC, normalized iodine concentration; Z, effective atom number; Rho, electron density; DEI, dual-energy index; λHU, dual-energy curve slope value; DIC, differential iodine concentration in the arteriovenous phase.
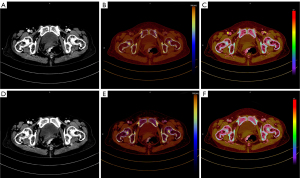
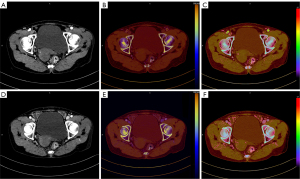
In descending order, the areas under the curve (AUCs) for each parameter were AP-IC: 0.96, DIC: 0.89, AP-DEI: 0.75, VP-NIC: 0.75, AP-NIC: 0.69, and AP-λHU: 0.64 (Table 6; Figure 6). Parameters with P values <0.1 in the one-way binary logistic regression analysis were included in the multifactor binary logistic regression, and the final regression model obtained was as follows: logit (P) = 139.64 – 42.36 × AP-IC – 0.83 × AP-NIC – 4.26 × DIC – 560.57 × AP – DEI – 0.21 × VP-NIC – 2.03 × AP-λHU – 0.58 × CEA – 23.42 × histological grade. The AUC, sensitivity, specificity, and predictive accuracy of the model were 0.99, 100.00%, 92.68%, and 94.67%, respectively, and the AUC had a 95% CI of 0.93–1.00.
Table 6
Parameters | AP-IC | AP-NIC (%) | AP-DEI | AP-λHU | DIC | VP-NIC (%) |
---|---|---|---|---|---|---|
AUC | 0.96 | 0.69 | 0.75 | 0.64 | 0.89 | 0.75 |
95% CI for AUC | 0.88–0.99 | 0.57–0.79 | 0.64–0.85 | 0.52–0.75 | 0.80–0.95 | 0.64–0.84 |
Cut-off point | 2.94 | 21.52 | 19.50 | 1.35 | 0.48 | 41.50 |
Sensitivity (%) | 97.06 | 85.29 | 52.90 | 52.94 | 85.29 | 76.47 |
Specificity (%) | 87.80 | 60.98 | 85.37 | 78.05 | 82.93 | 68.29 |
DECT, dual-energy computed tomography; AP-IC, concentrations of iodine in the arterial phase within the region of interest; AP-NIC, concentrations of normalized iodine in the arterial phase within the region of interest; AP-DEI, dual-energy index in the arterial phase within the region of interest; AP-λHU, dual-energy curve slope value in the arterial phase within the region of interest; DIC, differential iodine concentration in the arteriovenous phase; VP-NIC, concentrations of normalized iodine in the venous phase within the region of interest; AUC, area under the curve; CI, confidence interval.
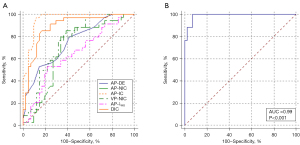
Discussion
The preoperative prediction of risk factors for CRC is important because it not only influences the survival time of patients but also informs individual treatment plans. In this study, we first evaluated the optimal DECT image of CRC, and we then investigated whether the quantitative parameters of DECT could help in the preoperative selection of CRC patients with high-risk factors and constructed a comprehensive diagnostic model.
Previous studies have shown that noise-optimized low-keV VMI+ series reconstructed images significantly reduce image noise while improving tumor saliency compared to conventional VMI series reconstructed images and standard linear hybrid reconstructed series images (16,21,22). The accuracy of DECT for tumor diagnosis has been directly enhanced by the improved contrast and lesion delineation with VMI+. One study found 40 keV VMI+ had significantly higher sensitivity and diagnostic accuracy for detecting CRC liver metastases than standard linear mixed reconstruction (90.6% vs. 80.6%, and 89.1% vs. 81.3%, respectively) (15). Lee et al. (23) found that compared to standard 40 keV VMI, deep learning-based 40 keV VMI+ had better image quality and a higher detection rate of hypoenhancing hepatic metastasis, which shows that the strengths of VMI+ series reconstructed images could be replicated in the field of deep learning.
In our quantitative image analysis, we also found that the 40 keV VMI+ series exhibited the highest tumor attenuation and higher SNR and CNR than the standard linear mixed M0.6 image series, and the results were consistent with those of previous studies (17). However, the overall image quality analysis in our study showed that the 60 keV VMI+ series scored the highest, indicating that tumor attenuation and noise balanced out at 60 keV VMI+, which is generally consistent with the findings of Lenga et al. (17). Thus, we further compared the 40 and 60 keV VMI+ CRC images. Notably, the blood supply vessels inside or beside the tumors were easier to identify in the 40 keV VMI+ images than the 60 keV VMI+ images; this finding enabled us to place the ROIs’ more accurately in the tumor, avoiding the interference of larger blood vessels. Thus, considering the advantages of both the 40 and 60 keV VMI+ images, we recommend using the 60 keV VMI+ series images as a lesion reference and then outlining the ROI on the 40 keV VMI+ series image to provide a more accurate delineation of the lesion for DECT quantitative analysis.
To the best of our knowledge, the risk factors for CRC include lymph node metastasis, EMVI, PNI, high-grade tumors, and T4 stage. In an analysis of the prognosis of T2N0M0 CRC patients, lymphovascular permeation, perineural invasion, and poor differentiation were found to be risk factors for a poor prognosis (24). Another prognostic analysis of lymph node-negative CRC found that perineural infiltration, EMVI, and T4 staging were independent prognostic factors, and these patients would benefit from adjuvant or more aggressive treatment (25). Notably, a recent study demonstrated that high preoperative CEA levels and the presence of vascular cancer emboli were risk factors for lymph node metastasis, which suggests that the risk factors for CRC may be interrelated and coexist (26). Thus, in our study, we allocated patients with one or more risk factors to the high-risk group to provide a more objective and comprehensive preoperative predictive analysis of risk factors for CRC and evaluated the association between DECT quantitative parameters and high-risk factors in CRC tumors.
According to the results, AP-IC, AP-NIC, AP-DEI, AP-λHU, and DEI were significantly higher in the high-risk group than the low-risk group. During tumor progression, tumor cells secrete vascular endothelial factors that increase the permeability of tumor blood vessels, leading to a higher intratissue microvascular density (MVD) and microvascular permeability in high-risk patients. IC, which is positively correlated with blood volume and permeability, directly reflects the angiogenesis and blood supply of the tumor tissue (27,28). Thus, it was not surprising that the AP-IC and AP-NIC values were significantly higher in the high-risk group than the low-risk group, and the results support those of Luo et al. (29).
Additionally, due to the higher X-ray attenuation capacity of iodine compared to soft tissues, the higher the tumor tissue uptake of iodine intake, the greater the attenuation to X-rays and the higher the DEI and AP-λHU value, so the AP-DEI and AP-λHU were also significantly higher in the high-risk group than the low-risk group. However, as we noted that the VP-NIC in the high-risk group was lower than that in the low-risk group (41.59±7.30 vs. 46.56±7.75), we also analyzed the DIC between the two groups in the arteriovenous phase, and the results showed that the DIC was significantly higher in the high-risk group than the low-risk group (0.73±0.25 vs. 0.36±0.22), which may be due to the higher MVD and vascular endothelial growth factor (VEGF) expression in the high-risk group.
The high expression of VEGF could increase the local vascular permeability and cause a higher clearance rate of the contrast agent (30,31). Thus, it may be that more contrast agents were retained in the tumor blood vessels in the low-risk group in the VP, resulting in the higher VP-NIC in the low-risk group than in the high-risk group. Nevertheless, there were no significant differences between the two groups in terms of electron density and effective atomic number, and the results support those of Qiu et al. (32). However, Zhang et al. (33) found that the normalized effective atomic number value of metastatic sentinel lymph nodes in breast cancer patients was significantly larger than that of non-metastatic lymph nodes. This may be related to the different ways of outlining the ROI and the differences in the settings of the DECT scanning parameters, as well as the different tumor types. Thus, there is a need for a standardized dual-energy scanning protocol for different type of tumors.
Of all the DECT parameters, AP-IC had the highest AUC. This is probably because IC is a direct reflection of the vascular enhancement of the tumor tissue and was influenced by other factors, such as NIC. This result supports that of Zou et al. (34). Further, we combined all the DECT parameters with significant differences and CEA and histological grade to establish a clinical DECT model and found that the AUC, sensitivity, specificity, and prediction accuracy of the regression model were 0.99, 100.00%, 92.68%, and 94.67%, respectively. The predictive accuracy of the clinical DECT model was significantly improved compared to the DECT parameter alone, indicating that the combined model may have greater value in high-risk factor predictions. The results were in line with some artificial intelligence (AI) CRC studies (35,36).
Li et al. constructed a machine learning-based CT model that combined radiomics and clinical features that could predict early the presence of metachronous liver metastases in patients by measuring the primary lesions of CRC (35). The model had and AUC of 0.79±0.08 (35). Zhao et al. confirmed the excellent predictive efficacy of deep learning-based imagingomics models for lymph node metastasis in CRC (36). The models constructed in these studies have good predictive efficacy for subgroups with high-risk factors for CRC; however, they were based on conventional CT images of the arteriovenous phase. We speculated that the iodograms and effective atomic number maps derived from DECT may provide potential information, and the AI models based on these maps may perform well in studies related to high-risk prediction and the prognosis of CRC patients.
Liver metastasis is one of the most important factors in the survival and prognosis of CRC patients. The early diagnosis of CRLMs implies a smaller lesion size, which is an indication for minimally invasive surgery (37). Compared to traditional open surgery, robotic-assisted minimally invasive surgery not only allows for the simultaneous resection of CRLMs and primary CRC, but also reduces intraoperative bleeding, postoperative complication rates, and postoperative hospital stays, while shortening the learning curve due to enhanced 3D full-high definition (HD) vision and wristed instruments (38,39). One study showed that laparoscopic ultrasound has better sensitivity than MRI for CRLMs, especially for lesions located in the liver dome (40), which may be due to the abnormal MRI signal in the liver dome. As far as we know, relatively few studies of DECT on CRLM have been conducted compared to studies of MRI. We believe that DECT will play an important role in the development of surgical protocols for CRLM and in the assessment of efficacy following chemotherapy as the benefits of its quantitative and qualitative analysis become better understood.
This study had several limitations. First, it was limited to adenocarcinoma CRC, and special histologic types, such as mucinous adenocarcinoma, were not included. In addition, while one of the principles of ROI outlining is to match the biopsy results, ROI outlining is also based on the maximum cross-sectional area of the tumor, so the ROI outlining and biopsy results may not completely match. Finally, the sample size in this study was relatively small, and no further subgroup analysis was performed based on risk stratification. It will be interesting for us to explore the predictive performance of DECT for the risk stratification of CRC in the future.
Conclusions
In conclusion, our study demonstrated that 40 VMI+ images were optimal for CRC DECT quantitative evaluation. Additionally, the AP-IC, AP-NIC, AP-DEI, AP-λHU, and DEI DECT parameters reflected the biological behavior of high-risk CRC tumors; thus, these DECT parameters can serve as additional tools for the potential prediction of high-risk factors in CRC. The diagnostic performance of the composite model constructed by combining DECT parameters with CEA and histological grading appears to be at a clinically acceptable level, allowing a more accurate prediction of high-risk factors in each patient and assisting in clinical decision-making.
Acknowledgments
Funding: This work was supported by a grant from the
Footnote
Reporting Checklist: The authors have completed the STROBE reporting checklist. Available at https://qims.amegroups.com/article/view/10.21037/qims-23-291/rc
Conflicts of Interest: All authors have completed the ICMJE uniform disclosure form (available at https://qims.amegroups.com/article/view/10.21037/qims-23-291/coif). All authors report that this work was supported by a grant from the Joint Project of Chongqing Health Commission and Science and Technology Bureau (No. 2021MSXM220). The authors have no other conflicts of interest to declare.
Ethical Statement: The authors are accountable for all aspects of the work in ensuring that questions related to the accuracy or integrity of any part of the work are appropriately investigated and resolved. This prospective study was conducted in accordance with the Declaration of Helsinki (as revised in 2013). The Ethics Committee of the Second Affiliated Hospital of Chongqing Medical University approved the prospective study and waived the requirement for informed consent because the study did not adversely affect the rights and health of the subjects.
Open Access Statement: This is an Open Access article distributed in accordance with the Creative Commons Attribution-NonCommercial-NoDerivs 4.0 International License (CC BY-NC-ND 4.0), which permits the non-commercial replication and distribution of the article with the strict proviso that no changes or edits are made and the original work is properly cited (including links to both the formal publication through the relevant DOI and the license). See: https://creativecommons.org/licenses/by-nc-nd/4.0/.
References
- Chen K, Collins G, Wang H, Toh JWT. Pathological Features and Prognostication in Colorectal Cancer. Curr Oncol 2021;28:5356-83. [Crossref] [PubMed]
- Dekker E, Tanis PJ, Vleugels JLA, Kasi PM, Wallace MB. Colorectal cancer. Lancet 2019;394:1467-80. [Crossref] [PubMed]
- Sawicki T, Ruszkowska M, Danielewicz A, Niedźwiedzka E, Arłukowicz T, Przybyłowicz KE. A Review of Colorectal Cancer in Terms of Epidemiology, Risk Factors, Development, Symptoms and Diagnosis. Cancers (Basel) 2021;13:2025. [Crossref] [PubMed]
- Nozawa H, Kawai K, Hata K, Tanaka T, Nishikawa T, Otani K, Sasaki K, Kaneko M, Emoto S, Murono K. High-risk Stage II Colorectal Cancers Carry an Equivalent Risk of Peritoneal Recurrence to Stage III. In Vivo 2018;32:1235-40. [Crossref] [PubMed]
- Nagtegaal ID, Knijn N, Hugen N, Marshall HC, Sugihara K, Tot T, Ueno H, Quirke P. Tumor Deposits in Colorectal Cancer: Improving the Value of Modern Staging-A Systematic Review and Meta-Analysis. J Clin Oncol 2017;35:1119-27. [Crossref] [PubMed]
- Benson AB, Venook AP, Al-Hawary MM, Cederquist L, Chen YJ, Ciombor KK, et al. NCCN Guidelines Insights: Colon Cancer, Version 2.2018. J Natl Compr Canc Netw 2018;16:359-69. [Crossref] [PubMed]
- Parent P, Cohen R, Rassy E, Svrcek M, Taieb J, André T, Turpin A. A comprehensive overview of promising biomarkers in stage II colorectal cancer. Cancer Treat Rev 2020;88:102059. [Crossref] [PubMed]
- Heo SH, Kim JW, Shin SS, Jeong YY, Kang HK. Multimodal imaging evaluation in staging of rectal cancer. World J Gastroenterol 2014;20:4244-55. [Crossref] [PubMed]
- Schmidt B, Flohr T. Principles and applications of dual source CT. Phys Med 2020;79:36-46. [Crossref] [PubMed]
- So A, Nicolaou S. Spectral Computed Tomography: Fundamental Principles and Recent Developments. Korean J Radiol 2021;22:86-96. [Crossref] [PubMed]
- Yang Z, Zhang X, Fang M, Li G, Duan X, Mao J, Shen J. Preoperative Diagnosis of Regional Lymph Node Metastasis of Colorectal Cancer With Quantitative Parameters From Dual-Energy CT. AJR Am J Roentgenol 2019;213:W17-25. [Crossref] [PubMed]
- Chen W, Ye Y, Zhang D, Mao L, Guo L, Zhang H, Du X, Deng W, Liu B, Liu X. Utility of dual-layer spectral-detector CT imaging for predicting pathological tumor stages and histologic grades of colorectal adenocarcinoma. Front Oncol 2022;12:1002592. [Crossref] [PubMed]
- Gao W, Zhang Y, Dou Y, Zhao L, Wu H, Yang Z, Liu A, Zhu L, Hao F. Association between extramural vascular invasion and iodine quantification using dual-energy computed tomography of rectal cancer: a preliminary study. Eur J Radiol 2023;158:110618. [Crossref] [PubMed]
- Grant KL, Flohr TG, Krauss B, Sedlmair M, Thomas C, Schmidt B. Assessment of an advanced image-based technique to calculate virtual monoenergetic computed tomographic images from a dual-energy examination to improve contrast-to-noise ratio in examinations using iodinated contrast media. Invest Radiol 2014;49:586-92. [Crossref] [PubMed]
- Lenga L, Lange M, Arendt CT, Booz C, Yel I, Bodelle B, D'Angelo T, Hammerstingl RM, Huizinga NA, Vogl TJ, Martin SS, Albrecht MH. Measurement Reliability and Diagnostic Accuracy of Virtual Monoenergetic Dual-Energy CT in Patients with Colorectal Liver Metastases. Acad Radiol 2020;27:e168-75. [Crossref] [PubMed]
- Martin SS, Wichmann JL, Weyer H, Albrecht MH, D'Angelo T, Leithner D, Lenga L, Booz C, Scholtz JE, Bodelle B, Vogl TJ, Hammerstingl R. Dual-energy computed tomography in patients with cutaneous malignant melanoma: Comparison of noise-optimized and traditional virtual monoenergetic imaging. Eur J Radiol 2017;95:1-8. [Crossref] [PubMed]
- Lenga L, Czwikla R, Wichmann JL, Leithner D, Albrecht MH, D'Angelo T, Arendt CT, Booz C, Hammerstingl R, Vogl TJ, Martin SS. Dual-energy CT in patients with abdominal malignant lymphoma: impact of noise-optimised virtual monoenergetic imaging on objective and subjective image quality. Clin Radiol 2018;73:833.e19-27. [Crossref] [PubMed]
- Lenga L, Czwikla R, Wichmann JL, Leithner D, Albrecht MH, Booz C, Arendt CT, Yel I, D'Angelo T, Vogl TJ, Martin SS. Dual-energy CT in patients with colorectal cancer: Improved assessment of hypoattenuating liver metastases using noise-optimized virtual monoenergetic imaging. Eur J Radiol 2018;106:184-91. [Crossref] [PubMed]
- Albrecht MH, Scholtz JE, Hüsers K, Beeres M, Bucher AM, Kaup M, Martin SS, Fischer S, Bodelle B, Bauer RW, Lehnert T, Vogl TJ, Wichmann JL. Advanced image-based virtual monoenergetic dual-energy CT angiography of the abdomen: optimization of kiloelectron volt settings to improve image contrast. Eur Radiol 2016;26:1863-70. [Crossref] [PubMed]
- Sudarski S, Apfaltrer P, Nance JW Jr, Schneider D, Meyer M, Schoenberg SO, Fink C, Henzler T. Optimization of keV-settings in abdominal and lower extremity dual-source dual-energy CT angiography determined with virtual monoenergetic imaging. Eur J Radiol 2013;82:e574-81. [Crossref] [PubMed]
- Liang H, Zhou Y, Zheng Q, Yan G, Liao H, Du S, Zhang X, Lv F, Zhang Z, Li YM. Dual-energy CT with virtual monoenergetic images and iodine maps improves tumor conspicuity in patients with pancreatic ductal adenocarcinoma. Insights Imaging 2022;13:153. [Crossref] [PubMed]
- Martin SS, Pfeifer S, Wichmann JL, Albrecht MH, Leithner D, Lenga L, Scholtz JE, Vogl TJ, Bodelle B. Noise-optimized virtual monoenergetic dual-energy computed tomography: optimization of kiloelectron volt settings in patients with gastrointestinal stromal tumors. Abdom Radiol (NY) 2017;42:718-26. [Crossref] [PubMed]
- Lee T, Lee JM, Yoon JH, Joo I, Bae JS, Yoo J, Kim JH, Ahn C, Kim JH. Deep learning-based image reconstruction of 40-keV virtual monoenergetic images of dual-energy CT for the assessment of hypoenhancing hepatic metastasis. Eur Radiol 2022;32:6407-17. [Crossref] [PubMed]
- Xu B, Yu L, Zhao LZ, Ma DW. Prognostic factors in the patients with T2N0M0 colorectal cancer. World J Surg Oncol 2016;14:76. [Crossref] [PubMed]
- Desolneux G, Burtin P, Lermite E, Bergamaschi R, Hamy A, Arnaud JP. Prognostic factors in node-negative colorectal cancer: a retrospective study from a prospective database. Int J Colorectal Dis 2010;25:829-34. [Crossref] [PubMed]
- Hu S, Li S, Teng D, Yan Y, Lin H, Liu B, Gao Z, Zhu S, Wang Y, Du X. Analysis of risk factors and prognosis of 253 lymph node metastasis in colorectal cancer patients. BMC Surg 2021;21:280. [Crossref] [PubMed]
- Kang HJ, Kim SH, Bae JS, Jeon SK, Han JK. Can quantitative iodine parameters on DECT replace perfusion CT parameters in colorectal cancers? Eur Radiol 2018;28:4775-82. [Crossref] [PubMed]
- Moding EJ, Clark DP, Qi Y, Li Y, Ma Y, Ghaghada K, Johnson GA, Kirsch DG, Badea CT. Dual-energy micro-computed tomography imaging of radiation-induced vascular changes in primary mouse sarcomas. Int J Radiat Oncol Biol Phys 2013;85:1353-9. [Crossref] [PubMed]
- Luo YH, Mei XL, Liu QR, Jiang B, Zhang S, Zhang K, Wu X, Luo YM, Li YJ. Diagnosing cervical lymph node metastasis in oral squamous cell carcinoma based on third-generation dual-source, dual-energy computed tomography. Eur Radiol 2023;33:162-71. [Crossref] [PubMed]
- Zheng S, Han MY, Xiao ZX, Peng JP, Dong Q. Clinical significance of vascular endothelial growth factor expression and neovascularization in colorectal carcinoma. World J Gastroenterol 2003;9:1227-30. [Crossref] [PubMed]
- Wang YD, Wu P, Mao JD, Huang H, Zhang F. Relationship between vascular invasion and microvessel density and micrometastasis. World J Gastroenterol 2007;13:6269-73. [Crossref] [PubMed]
- Qiu L, Hu J, Weng Z, Liu S, Jiang G, Cai X. A prospective study of dual-energy computed tomography for differentiating metastatic and non-metastatic lymph nodes of colorectal cancer. Quant Imaging Med Surg 2021;11:3448-59. [Crossref] [PubMed]
- Zhang X, Zheng C, Yang Z, Cheng Z, Deng H, Chen M, Duan X, Mao J, Shen J. Axillary Sentinel Lymph Nodes in Breast Cancer: Quantitative Evaluation at Dual-Energy CT. Radiology 2018;289:337-46. [Crossref] [PubMed]
- Zou Y, Zheng M, Qi Z, Guo Y, Ji X, Huang L, Gong Y, Lu X, Ma G, Xia S. Dual-energy computed tomography could reliably differentiate metastatic from non-metastatic lymph nodes of less than 0.5 cm in patients with papillary thyroid carcinoma. Quant Imaging Med Surg 2021;11:1354-67. [Crossref] [PubMed]
- Li Y, Gong J, Shen X, Li M, Zhang H, Feng F, Tong T. Assessment of Primary Colorectal Cancer CT Radiomics to Predict Metachronous Liver Metastasis. Front Oncol 2022;12:861892. [Crossref] [PubMed]
- Zhao J, Wang H, Zhang Y, Wang R, Liu Q, Li J, Li X, Huang H, Zhang J, Zeng Z, Zhang J, Yi Z, Zeng F. Deep learning radiomics model related with genomics phenotypes for lymph node metastasis prediction in colorectal cancer. Radiother Oncol 2022;167:195-202. [Crossref] [PubMed]
- Calise F, Giuliani A, Sodano L, Crolla E, Bianco P, Rocca A, Ceriello A. Segmentectomy: is minimally invasive surgery going to change a liver dogma? Updates Surg 2015;67:111-5. [Crossref] [PubMed]
- Ceccarelli G, Andolfi E, Fontani A, Calise F, Rocca A, Giuliani A. Robot-assisted liver surgery in a general surgery unit with a "Referral Centre Hub&Spoke Learning Program". Early outcomes after our first 70 consecutive patients. Minerva Chir 2018;73:460-8. [Crossref] [PubMed]
- Rocca A, Cipriani F, Belli G, Berti S, Boggi U, Bottino V, et al. The Italian Consensus on minimally invasive simultaneous resections for synchronous liver metastasis and primary colorectal cancer: A Delphi methodology. Updates Surg 2021;73:1247-65. [Crossref] [PubMed]
- Russolillo N, Borello A, Langella S, Casella M, Lo Tesoriere R, Ferrero A. Comparison of laparoscopic ultrasound and liver-specific magnetic resonance imaging for staging colorectal liver metastases. Surg Endosc 2021;35:3547-53. [Crossref] [PubMed]