The association of global vessel width with cognitive decline and cerebral small vessel disease burden in the KaiLuan study
Introduction
Cognitive decline is becoming more prevalent as the world’s population ages, with serious health and societal consequences. Some people may reach the threshold for mild cognitive impairment (MCI), which can lead to dementia in up to 50% of cases over the course of five years (1).
Cerebral small vessel disease (CSVD) is a major cause of cognitive impairment in the elderly, accounting for around 50% of dementia (2). White matter hyperintensities (WMHs), lacunes, enlarged perivascular spaces (EPVS), and cerebral microbleeds (CMBs) are typical magnetic resonance imaging (MRI) features of the disease (3). The severity of CSVD may be more accurately reflected by the CSVD burden score, which incorporates the imaging indicators into a single value (4). Among the various mechanisms involved in vascular cognitive impairment (VCI), CSVD is perhaps the most common, contributing to cognitive impairment regardless of stroke (5).
Similar embryologic, anatomical, and physiological traits exist in retinal vessels and the cerebral circulation. Numerous studies on retinal imaging have shown that the retina can be used to diagnose, predict, and assess the risk of cognitive decline (6,7). Additionally, a number of population-based investigations have verified the association between retinal biomarkers and CSVD (8,9). As a result, we assume that there might be an association between them. However, the relationship between retinal biomarkers and both CSVD burden and cognitive performance has not been investigated simultaneously. All these findings make it questionable if CSVD burden can be considered as a mediating element in the association between retinal microvascular parameters (RMPs) and cognitive function. In addition, a number of investigations have used semi-automated retinal vascular measurement software, which is time-consuming and vulnerable to error (10,11). Deep-learning algorithms have been effectively used on retinal images to assess diabetic retinopathy and other aspects over the past few years (12,13). In our study, retinal vascular characteristics were automatically measured using a deep-learning system—NFN+.
In this research, we aimed to examine the relationship between RMPs and cognitive function as well as the effect of CSVD burden. We present this article in accordance with the STROBE reporting checklist (available at https://qims.amegroups.com/article/view/10.21037/qims-23-927/rc).
Methods
Participants
The KaiLuan study is an ongoing population-based study executing in the Kailuan community of Tangshan in Northern China (14). From December 2020 to October 2021, a total of 910 patients were included. Inclusion criteria were as follows: (I) aged ≥18 years old; (II) without contra-indications of retinal fundus photograph; (III) with completed brain MRI to evaluate CSVD markers; (IV) completed cognitive function assessment. The study excluded those with: (I) acute stroke, hematologic disorders, myocardial infarction, malignant tumors, autoimmune diseases; (II) tumors of the brain or other systems, surgery, or severe trauma; (III) pregnant and lactating women; (IV) cognitive decline caused by other conditions, such as hyperthyroidism, severe anxiety, depression, carbon monoxide poisoning or schizophrenia; (V) MRI artifacts; (VI) incomplete fundus photography.
Ultimately, a total of 905 eligible subjects enrolled in the research (Figure 1). This study was conducted according to the Declaration of Helsinki (as revised in 2013). This study was approved by the Medical Ethics Committee of Kailuan General Hospital (IRB number: 2021002). The informed consent was taken from all the patients.
Clinical assessment
The following clinical variables were obtained: age, sex, education level, height, weight, systolic and diastolic blood pressure, current smoking or drinking, history of hypertension, diabetes, coronary heart disease, and medication use. Body mass index (BMI) was calculated as weight (kg) divided by square of height (m2). Laboratory biomarkers included fasting blood glucose (FBG), total cholesterol (TC).
Montreal Cognitive Assessment (MoCA)
The MoCA was utilized to measure cognitive function (15). The test was administered by an experienced examiner (10 years of experience). The MoCA total score range is 0–30, with lower scores (<26 points) suggesting poorer cognitive functioning.
Brain MRI acquisition
The brain MRI was obtained using a 3.0 T scanner (GE 750W; General Electric Medical Systems, Milwaukee, Wisconsin, USA)and an eight-channel phased-array coil. The MRI consisted of at least five sequences that followed predetermined standardized protocols: T1-weighted imaging (T1WI), T2-weighted imaging (T2WI), fluid-attenuated inversion recovery (FLAIR), diffusion-weighted imaging (DWI), and susceptibility weighted imaging (SWI). In our previous study, we recorded the exact parameter settings for the five sequences (14). Based on the Neuroimaging Standards for Research into Small Vessel Disease (STRIVE) recommendation there are four main kinds of imaging markers: WMHs, lacunar, CMBs, and EPVS (16). Two experienced neuroradiologists (reader 1: 8 years of experience; reader 2: 4 years of experience) independently reviewed the brain imaging, and a third neuroradiologist with more than ten years of expertise who was blind to the individuals’ clinical information resolved with any discrepancies.
The Fazekas rating scale was used to assess the severity of periventricular WMH (pWMH) (range, 0–3) and deep WMH (dWMH) (range, 0–3) (17). On SWI sequences, CMBs were identified as rounded hypointense lesions with a diameter of 2 to 10 mm (18). Lacune was defined as a 3–15 mm subcortical lesion with cerebrospinal fluid (CSF) intensity on T1WI and FLAIR (16). EPVS is the basal ganglia or centrum semiovale’s circular, ovoid, or linear space with a CSF signal on all sequences that is less than 3 mm in diameter. The severity of EPVS was assessed using a semi-quantitative scale (grade 0–4). An ordinal scale from 0 to 4 was used to rate the total CSVD burden score. Each of the following gained a point: (I) severe pWMH (score =3) or moderate to severe dWMH (score ≥2); (II) at least one lacunes; (III) any deep CMB; (IV) moderate to extensive EPVS. The total MRI burden of CSVD scores was then categorized into three groups based on the CSVD score: mild burden (0–1 point), moderate burden (2 points), severe burden (3–4 points) (19,20).
RMPs
A fundus camera (Topcon CX-1, Topcon Corporation, Tokyo, Japan) was used to record the bilateral fundus photography images. The following were the quality assurance standards: (I) no significant artifacts or blurring; (II) brightness that was not either too dark or too light; and (III) the image field had to include the full optic disc and macula. Images of poor quality were discarded. If an image could not be correctly measured, the images of the other eye were employed. If images of both eyes were of poor image quality, the patient was finally excluded from this investigation.
The study achieved autonomous extraction of retinal vessels using NFN+, a recently published deep learning-based technique (12). In terms of cascaded design and inter-network skip connections, the proposed NFN+ model is innovative. A front network and a following network, two cascaded identical backbones, are connected via inter-network skip connections. The front network outputs prime vessel probability maps using picture patches as input. The subsequent network produces vessel segmentation results using the prime vessel probability maps created by the front network as its input (21). Following the deep learning model’s vascular segmentation mask, two skilled retinal specialists classified the arteries and veins manually. Subsequently we took measurements for a vessel geometric class known as retinal vascular fractal dimension (FD). Each segmented mask was divided into a series of squares with varying side lengths along the centerline tracings of retinal vessels using the box-counting approach. Retinal vascular FD was consequently defined as the gradient of logarithms of the number of boxes and their sizes. In addition to the vascular geometric class, we examined the diameters of all arterioles and venules passing through a 0.50 to 0.75 disc diameter area surrounding the optic disc. The average retinal arteriolar and venular caliber, identified as central retinal arterial equivalent (CRAE) and central retinal venular equivalent (CRVE), were calculated using the Knudtson- Hubbard formula. The arteriole-venular ratio (AVR) is the CRAE/CRVE ratio. The global vessel width (global arteriolar and venular width) is the average width of all the artery or vein branches from the specific subjects’ eye.
Statistical analysis
The data were analyzed using SPSS version 20.0 software (SPSS Inc., Chicago, IL, USA). The demographic characteristics of the subjects were presented as means (standard deviation) or frequencies (percentage), when appropriate. The differences between the cognitive decline group and the non- cognitive decline group were compared using Student’s t-test or Mann-Whitney’s U-test for continuous variables and χ2-test for categorical variables. One-way analysis of variance (ANOVA), Chi-squared test or rank-sum test was used to compare the differences between the CSVD groups (CSVD 0, 1, 2). We applied ordinal logistic regression models to evaluate the associations of RMPs with cognitive function and the severity of CSVD burden. Trend tests in ORs across RMPs tertiles were performed with the median within each tertile as the variable. Three logistic regression models were conducted in this study. Model 1: age, sex, and education; Model 2: additionally adjusted for FBG, BMI, smoking, alcohol use, TC; Model 3: additionally adjusted for systolic blood pressure, heart rate, and use of antihypertensive and/or lipid-modifying medication. A P<0.05 was considered statistically significant (two-sided). The PROCESS macro for SPSS was used to investigate the mediation effect of CSVD burden in the relationship between RMPs and MoCA. The bootstrap method was used to test the mediation effect.
Results
Participants’ baseline characteristics
This study included 905 participants. Mean age of the subjects was 55.42±12.02 years, and 54.5% (n=493) were female. Based on MoCA, subjects were divided into cognitive decline group (n=488) and no cognitive decline group (n=417). According to CSVD burden, participants were divided into mild group (n=567), moderate group (n=189) and severe group (n=149). Features of the subjects in different groups are shown in Tables 1,2.
Table 1
Clinical variables | All (n=905) | Cognitive decline group (n=488) | No cognitive decline group (n=417) | P value |
---|---|---|---|---|
Age, year, mean ± SD | 55.42±12.02 | 59.61±10.67 | 50.52±11.65 | <0.001 |
Sex (female), n (%) | 493 (54.5) | 295 (60.5) | 198 (47.5) | <0.001 |
Education, secondary level and above, n (%) | 670 (74.0) | 293 (60.0) | 377 (90.4) | <0.001 |
Body mass index, kg/m2, mean ± SD | 25.21±3.40 | 25.37±3.25 | 25.01±3.56 | 0.113 |
Smoking, n (%) | 221 (24.4) | 130 (26.6) | 91 (21.8) | 0.104 |
Alcohol, no-drinker n (%) | 679 (75.0) | 362 (74.2) | 317 (76.0) | 0.490 |
Hypertension, n (%) | 433 (47.8) | 265 (54.3) | 168 (40.3) | <0.001 |
Diabetes, n (%) | 100 (11.0) | 58 (11.9) | 42 (10.1) | 0.457 |
Coronary heart disease, n (%) | 2 (0.2) | 2 (0.4) | 0 | 0.503 |
Fasting blood glucose, mmol/L, median [IQR] | 5.13 [4.73, 5.66] | 5.26 [4.82, 5.79] | 5.04 [4.68, 5.47] | <0.001 |
SBP, mmHg, median [IQR] | 133 [120, 148] | 136 [122, 151] | 129 [115, 143] | <0.001 |
DBP, mmHg, median [IQR] | 79.33 [70.67, 87.67] | 78.84 [70.33, 87.33] | 79.33 [71, 88] | 0.541 |
TC, mmol/L, median [IQR] | 5.09 [4.48, 5.83] | 5.11 [4.46, 5.77] | 5.07 [4.50, 5.85] | 0.622 |
CSVD, n (%) | <0.001 | |||
0 | 296 (32.7) | 101 (20.7) | 195 (46.8) | |
1 | 271 (29.9) | 147 (30.1) | 124 (29.7) | |
2 | 189 (20.9) | 125 (25.6) | 64 (15.3) | |
3 | 90 (9.9) | 65 (13.3) | 25 (6.0) | |
4 | 59 (6.5) | 50 (10.2) | 9 (2.2) | |
AVR, mean ± SD | 0.723±0.128 | 0.715±0.142 | 0.733±0.108 | 0.030 |
CRAE, μm, mean ± SD | 155.118±28.443 | 151.918±29.351 | 158.863±26.864 | <0.001 |
CRVE, μm, mean ± SD | 217.139±34.388 | 214.807±36.912 | 219.868±30.954 | 0.025 |
FD, mean ± SD | 1.305±0.084 | 1.290±0.089 | 1.324±0.074 | <0.001 |
Global artery width, μm, mean ± SD | 79.273±12.850 | 78.838±13.791 | 79.782±11.632 | 0.265 |
Global vein width, μm, mean ± SD | 127.652±28.860 | 132.885±33.257 | 121.529±21.063 | <0.001 |
MoCA, median [IQR] | 25 [22, 27] | 23 [20, 24] | 28 [27, 29] | <0.001 |
SD, standard deviation; IQR, interquartile range; SBP, systolic blood pressure; DBP, diastolic blood pressure; TC, total cholesterol; CSVD, cerebral small vessel disease; AVR, retinal arteriole/venular ratio; CRAE, central retinal arteriolar equivalent; CRVE, central retinal venular equivalent; FD, fractal dimension; MoCA, Montreal Cognitive Assessment.
Table 2
Clinical variables | All (n=905) | CSVD0 (n=567) | CSVD1 (n=189) | CSVD2 (n=149) | P value |
---|---|---|---|---|---|
Age, year, mean ± SD | 55.42±12.02 | 50.47±11.09 | 61.89±8.34 | 66.03±7.89 | <0.001 |
Sex (female), n (%) | 493 (54.5) | 306 (54.0) | 77 (40.7) | 31 (20.8) | <0.001 |
education, secondary level and above, n (%) | 670 (74.0) | 483 (85.2) | 112 (59.3) | 75 (50.3) | <0.001 |
Body mass index, kg/m2, mean ± SD | 25.21±3.40 | 25.07±3.48 | 25.35±3.43 | 25.54±3.05 | 0.269 |
smoking, n (%) | 221 (24.4) | 178 (31.4) | 87 (46.0) | 95 (63.8) | <0.001 |
Alcohol, no-drinker n (%) | 679 (75.0) | 334 (58.9) | 87 (46.0) | 64 (43.0) | <0.001 |
Hypertension, n (%) | 433 (47.8) | 212 (37.4) | 104 (55.0) | 115 (77.2) | <0.001 |
Diabetes, n (%) | 100 (11.0) | 39 (6.9) | 30 (15.9) | 31 (20.8) | <0.001 |
Coronary heart disease, n (%) | 2 (0.2) | 0 | 0 | 2 (1.3) | 0.007 |
Fasting blood glucose, mmol/L, median [IQR] | 5.13 [4.73, 5.66] | 5.09 [4.71, 5.54] | 5.14 [4.76, 5.78] | 5.40 [4.89, 6.28] | <0.001 |
SBP, mmHg, median [IQR] | 133 [120, 148] | 128 [116, 143] | 137 [125, 150] | 146 [134, 159] | <0.001 |
DBP, mmHg, median [IQR] | 79.33 [70.67, 87.67] | 78.33 [70, 87.33] | 79.67 [71.67, 87.33] | 82.33 [72.33, 89] | 0.090 |
TC, mmol/L, median [IQR] | 5.09 [4.48, 5.83] | 5.14 [4.54, 5.85] | 5.05 [4.36, 5.87] | 4.98 [4.38, 5.67] | 0.235 |
AVR, mean ± SD | 0.723±0.128 | 0.773±0.106 | 0.720±0.109 | 0.686±0.197 | <0.001 |
CRAE, μm, mean ± SD | 155.118±28.443 | 159.653±27.082 | 151.206±25.169 | 142.822±32.967 | <0.001 |
CRVE, μm, mean ± SD | 217.139±34.388 | 220.544±31.284 | 213.373±33.212 | 208.960±44.241 | <0.001 |
FD, mean ± SD | 1.305±0.084 | 1.323±0.077 | 1.286±0.083 | 1.259±0.089 | <0.001 |
Global artery width, μm, mean ± SD | 79.273±12.850 | 79.701±11.501 | 80.750±14.355 | 75.771±15.044 | 0.001 |
Global vein width, μm, mean ± SD | 127.652±28.860 | 122.543±23.415 | 130.541±34.792 | 143.433±33.123 | <0.001 |
MoCA, median [IQR] | 25 [22, 27] | 26 [24, 28] | 24 [21, 26] | 22 [19, 25] | <0.001 |
CSVD0, mild group; CSVD1, moderate group; CSVD2, severe group. CSVD, cerebral small vessel disease; SD, standard deviation; IQR, interquartile range; SBP, systolic blood pressure; DBP, diastolic blood pressure; TC, total cholesterol; AVR, retinal arteriole/venular ratio; CRAE, central retinal arteriolar equivalent; CRVE, central retinal venular equivalent; FD, fractal dimension; MoCA, Montreal Cognitive Assessment.
Between no cognitive decline and cognitive decline groups, statistically significant differences were found in age, sex, education level, hypertension, FBG, systolic blood pressure, CSVD burden (all above P<0.001) and RMPs (PAVR=0.030, PCRAE<0.001, PCRVE=0.025, PFD<0.001, Pglobal artery width=0.265, Pglobal vein width<0.001). Between different groups of CSVD burden, statistically significant differences were found in age, sex, education level, current drinking, current smoking, history of hypertension, diabetes or coronary heart disease, FBG, systolic blood pressure, diastolic blood pressure and RMPs (P<0.05).
Association of RMPs with cognitive decline
Ordinal logistic regression model result after adjusting for all the potential confounders in Model 3 showed that FD [odds ratio (OR): 0.098, 95% confidence interval (CI): 0.015–0.639; P=0.015] and global vein width (OR: 1.010, 95% CI: 1.005–1.015; P<0.001) were significantly associated with the risk of cognitive decline (Table 3). Wider global vein width and less complicated FD were associated with a significantly higher risk of cognitive decline when adjusting for Model 1 (global vein width: OR for tertile 2: 1.144, 95% CI: 0.815–1.605, P for trend <0.001; OR for tertile 3: 1.351, 95% CI: 0.953–1.915, P for trend <0.001; FD: OR for tertile 2: 0.969, 95% CI: 0.686–1.370, P for trend <0.001; OR for tertile 3: 0.829, 95% CI: 0.565–1.219, P for trend <0.001) and after further adjustment for Model 3 (global vein width: OR for tertile 2: 1.117, 95% CI: 0.793–1.574, P for trend <0.001; OR for tertile 3: 1.290, 95% CI: 0.901–1.847, P for trend <0.001; FD: OR for tertile 2: 0.964, 95% CI: 0.678–1.369, P for trend <0.001; OR for tertile 3: 0.849, 95% CI: 0.574–1.257, P for trend <0.001) in the highest tertile of RMPs index levels compared with the lowest (Table 4).
Table 3
Retinal vascular parameters | Model | Cognitive function | |
---|---|---|---|
β (95% CI) | P value | ||
AVR | 1 | 0.291 (0.087–0.975) | 0.045 |
2 | 0.313 (0.091–1.074) | 0.065 | |
3 | 0.336 (0.097–1.164) | 0.085 | |
CRAE | 1 | 0.996 (0.991–1.001) | 0.097 |
2 | 0.996 (0.991–1.001) | 0.120 | |
3 | 0.996 (0.991–1.002) | 0.170 | |
CRVE | 1 | 1.000 (0.996–1.004) | 0.855 |
2 | 1.000 (0.995–1.004) | 0.825 | |
3 | 1.000 (0.996–1.004) | 0.871 | |
FD | 1 | 0.095 (0.015–0.594) | 0.012 |
2 | 0.096 (0.015–0.620) | 0.014 | |
3 | 0.098 (0.015–0.639) | 0.015 | |
Global artery width | 1 | 0.990 (0.980–1.001) | 0.072 |
2 | 0.991 (0.980–1.001) | 0.091 | |
3 | 0.992 (0.981–1.003) | 0.164 | |
Global vein width | 1 | 1.010 (1.005–1.015) | <0.001 |
2 | 1.010 (1.005–1.015) | <0.001 | |
3 | 1.010 (1.005–1.015) | <0.001 |
Model 1: adjusted for age, sex, and education. Model 2: model 1 + FBG, BMI, smoking, alcohol use, TC. Model 3: Model 2 + SBP, HR, and use of antihypertensive and/or lipid-modifying medication. CI, confidence interval; AVR, retinal arteriole/venular ratio; CRAE, central retinal arteriolar equivalent; CRVE, central retinal venular equivalent; FD, fractal dimension; FBG, fasting blood glucose; BMI, body mass index; TC, total cholesterol; SBP, systolic blood pressure; HR, heart rate.
Table 4
Variables | Cognitive decline | ||
---|---|---|---|
Model 1 | Model 2 | Model 3 | |
FD | |||
Tertile 1 (<1.300) | 1.0 (reference) | 1.0 (reference) | 1.0 (reference) |
Tertile 2 (1.300–1.354) | 0.969 (0.686–1.370) | 0.964 (0.680–1.366) | 0.964 (0.678–1.369) |
Tertile 3 (>1.354) | 0.829 (0.565–1.219) | 0.842 (0.570–1.244) | 0.849 (0.574–1.257) |
P value for trend | <0.001 | ||
Global vein width | |||
Tertile1 (<114.474) | 1.0 (reference) | 1.0 (reference) | 1.0 (reference) |
Tertile2 (114.474–130.282) | 1.144 (0.815–1.605) | 1.145 (0.851–1.610) | 1.117 (0.793–1.574) |
Tertile3 (>130.282) | 1.351 (0.953–1.915) | 1.337 (0.940–1.902) | 1.290 (0.901–1.847) |
P value for trend | <0.001 |
Model 1: adjusted for age, sex, and education. Model 2: Model 1 + FBG, BMI, smoking, alcohol use, TC. Model 3: Model 2 + SBP, HR, and use of antihypertensive and/or lipid-modifying medication. OR, odds ratio; CI, confidence interval; RMPs, retinal microvascular parameters; FD, fractal dimension; FBG, fasting blood glucose; BMI, body mass index; TC, total cholesterol; SBP, systolic blood pressure; HR, heart rate.
Association of RMPs with CSVD burden
After adjusting for Model 3, the global artery width was an independent risk factor of severe CSVD burden (OR: 0.985, 95% CI: 0.974–0.997; P=0.013) (Table 5). The OR of severe CSVD burden of the highest tertile of global vein width compared with the lowest was 1.546 (95% CI: 1.004–2.290, P for trend <0.001) after adjusting for Model 3. After adjustment of potential confounders in Model 3, the OR of severe CSVD burden in the highest tertile of global artery width in comparison to the lowest was 0.550 (95% CI: 0.373–0.811, P for trend =0.002) (Table S1).
Table 5
Retinal vascular parameters | Model | CSVD burden | |
---|---|---|---|
β (95% CI) | P value | ||
AVR | 1 | 0.401 (0.113–1.427) | 0.158 |
2 | 0.462 (0.126–1.691) | 0.244 | |
3 | 0.513 (0.137–1.923) | 0.322 | |
CRAE | 1 | 0.996 (0.991–1.002) | 0.172 |
2 | 0.997 (0.991–1.002) | 0.269 | |
3 | 0.999 (0.993–1.004) | 0.630 | |
CRVE | 1 | 1.001 (0.996–1.005) | 0.849 |
2 | 1.001 (0.996–1.005) | 0.808 | |
3 | 1.002 (0.997–1.006) | 0.462 | |
FD | 1 | 0.483 (0.072–3.261) | 0.455 |
2 | 0.586 (0.083–4.151) | 0.593 | |
3 | 0.769 (0.103–5.754) | 0.798 | |
Global artery width | 1 | 0.979 (0.968–0.990) | <0.001 |
2 | 0.980 (0.968–0.991) | <0.001 | |
3 | 0.985 (0.974–0.997) | 0.013 | |
Global vein width | 1 | 1.006 (1.001–1.011) | 0.013 |
2 | 1.006 (1.001–1.011) | 0.014 | |
3 | 1.005 (1.000–1.010) | 0.050 |
Model 1: adjusted for age, sex, and education. Model 2: Model 1 + FBG, BMI, smoking, alcohol use, TC. Model 3: Model 2 + SBP, HR, and use of antihypertensive and/or lipid-modifying medication. CSVD, cerebral small vessel disease; CI, confidence interval; AVR, retinal arteriole/venular ratio; CRAE, central retinal arteriolar equivalent; CRVE, central retinal venular equivalent; FD, fractal dimension; FBG, fasting blood glucose; BMI, body mass index; TC, total cholesterol; SBP, systolic blood pressure; HR, heart rate.
Mediation by CSVD burden
The mediation pathway model with coefficients is shown in Figure 2. The indirect effect was −0.0011 (−0.0027, −0.0002), direct effect was −0.0156 (−0.0234, −0.0077), and total effect was −0.0167 (−0.0246, −0.0088). Thus, 6.59% (ab/c) of the total effect between the association of global vein width and cognitive decline was attributable to the presence of CSVD burden.
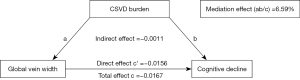
Discussion
In this study, we investigated the associations of RMPs with CSVD burden and cognitive function. The study demonstrated that RMPs (FD, global vein width), measured using a deep learning method on fundus photographs, were independent risk factors of cognitive decline. The global artery width and global vein width were associated with the development of CSVD, even after taking into account potential confounders. Additionally, mediation analyses indicated that CSVD burden was a mediating factor in the association between global vein width and cognitive function.
The embryological origins and physiological properties of the retinal and cerebral microvasculature are similar. Several previous studies have focused on the association between RMPs and cognitive function and reported controversial results (10,22,23). A prospective study involving 491 participants suggested that narrower retinal arteriolar calibre and wider retinal venular calibre were associated with increased risk of cognitive decline (24). In addition, another cross-sectional study of 69 subjects indicated that the retinal microvascular network was altered in cognitive impairment group compared to the controls, and the FD decreased significantly (25). Consistent with these studies, our study found that RMPs (FD, global vein width) were associated with an increased risk of cognitive impairment. Additionally, with the aggravation of cognitive function, global vein width tended to increase, whereas FD tended to express less complexity. Although the exact mechanism is yet unknown, a number of possible candidate methods, such as endothelial dysfunction, blood-brain barrier dysfunction, systemic inflammation, and other vascular risk factors, have been suggested as possible explanations for this association (26,27). However, in contrast to our study, two studies failed to detect any associations between RMPs and cognitive loss (10,28). The underlying reason for this variation could be due to the study’s design, outcome measures, enrolled population’s vascular risk factors, age, or gender.
CSVD is a slowly developing disease with nonspecific symptoms. The development of CSVD is thought to be influenced by endothelial dysfunction, hypoperfusion, inflammation, and oxidative stress (29,30). On cerebral MRI, the following signs of CSVD can be seen: lacunar, white WMHs, EPVS, and CMBs. A total CSVD assessment could help assess the combined impact of neuropathological changes in CSVD because of the simultaneous occurrence and joint effects of various MRI indicators (31). It is believed that the retinal microvasculature acts as a “window” to reflect the health of the cerebral microvasculature (32). Retinal alterations may indicate pathological processes in the central nervous system and can be examined non-invasively using imaging techniques. Moreover, because retinal biomarkers provide information on tissue structure and function at the microscopic level, they may enable for the early detection of CSVD in less severe or asymptomatic phases (33).
There is mounting evidence that retinal biomarkers are related to the emergence of various CSVD imaging features, which is important for the increased risk of cognitive impairment (20,34). It has been reported that the CSVD burden was associated with a wider CRVE, a smaller AVR, and a higher incidence of vessel tortuosity (35). A further study indicated that cerebral WMH might be partially predicted by non-invasive fundus photography via deep learning algorithm (36). Different from the previous study, our research failed to detect any associations between the total CSVD burden score and conventional RMPs (CRAE, CRVE, FD, AVR). A possible explanation for the lack of association may be that other disorders that can have a profound effect on ocular biomarkers (such as hypertension, diabetes and coronary/cardiovascular disease), and may obscure a possible neurodegenerative effect (20,37). Another explanation can be that our study was based on the whole population (aged 23–83 years), while other age-related processes may affect ocular biomarkers (38).
Many recent algorithms show outstanding outcomes on retinal vasculature owing to the blooming development of deep learning. Correlations between blood vessel morphology and diseases can be examined based on the segmentation results. The NFN+ method and vignetting mask strategy were previously created by our team to achieve retinal vessels segmentation, and they were validated on 50 randomly selected fundus images from the collected dataset (12,39). Using this method, we discovered connections between renal function and retinal blood vessels features in those with type 2 diabetes mellitus (21). In this research, more characteristics were included based on deep learning segmentation. Our study indicated that the presence of the global vessel width (global vein width and global artery width) is an independent risk factor for the development of CSVD, after adjusting for potential confounding factors. The global vessel width is a new parameter for assessing the retinal vasculature, calculated by the NFN+ software. It represents the evaluation of the whole retinal vein (artery) using deep learning techniques. We believe that these parameters allow for a more comprehensive assessment of retinal vascular structure and is therefore more sensitive than the previous retinal vascular parameter.
The current study observed that RMPs was closely associated with both CSVD and cognitive decline. CSVD should be taken into account when exploring the relationship between RMPs and cognitive decline, but to our knowledge very few studies do so. Our findings revealed a partial mediating role of severe CSVD burden on the association between global vein width and cognitive decline, supporting the hypothesis that wider global vein width could indicate an exacerbation of CSVD, which, in turn, increased the risk of cognitive impairment. The main strength of our study is that we took CSVD burden into account when analyzing the relationship between RMPs and cognitive decline. Another advantage is that we used a novel deep-learning system to measure retinal vessel. Given its precision and accuracy, this system can lessen the possibility of human mistake in retinal grading and has substantially lower inter- and intra-grader variability. In addition, the system has developed new retinal vascular parameters to better reflect retinal microvascular changes.
There were some limitations to this study. First, because this was a cross-sectional study, a causal association between CSVD and cognitive function could not be established. Second, because the participants in the current research were recruited from a single institution in China, the findings may not be generalizable to other populations. Third, the current study did not distinguish between patients with dementia and those without dementia. To overcome this issue, additional research is required in the future. Fourthly, it is probable that there are residual confounding factors which have not been accounted for, such as pulse cycle variation in an individual’s retinal calibre, that might have affected the associations found (40). Additionally, because our study individuals did not have any biomarker tests, our findings were not disease pathology specific.
Conclusions
In conclusion, our research demonstrated that RMPs are independent risk factors for cognitive decline and the development of CSVD. Furthermore, the association between RMPs and cognitive decline appeared to be partially mediated by the CSVD burden. In the future, prospective studies are needed to determine the causality of this association.
Acknowledgments
Funding: This research was supported by
Footnote
Reporting Checklist: The authors have completed the STROBE reporting checklist. Available at https://qims.amegroups.com/article/view/10.21037/qims-23-927/rc
Conflicts of Interest: All authors have completed the ICMJE uniform disclosure form (available at https://qims.amegroups.com/article/view/10.21037/qims-23-927/coif). X.Z., B.L., Y.N. and G.X., employees of Ping An Healthcare Technology, contributed to the image post-processing, collection and assembly of data without impacting the research results. The other authors have no conflicts of interest to declare.
Ethical Statement: The authors are accountable for all aspects of the work in ensuring that questions related to the accuracy or integrity of any part of the work are appropriately investigated and resolved. The study was conducted in accordance with the Declaration of Helsinki (as revised in 2013). This study was approved by the Medical Ethics Committee of Kailuan General Hospital (IRB number: 2021002). The informed consent was taken from all the patients.
Open Access Statement: This is an Open Access article distributed in accordance with the Creative Commons Attribution-NonCommercial-NoDerivs 4.0 International License (CC BY-NC-ND 4.0), which permits the non-commercial replication and distribution of the article with the strict proviso that no changes or edits are made and the original work is properly cited (including links to both the formal publication through the relevant DOI and the license). See: https://creativecommons.org/licenses/by-nc-nd/4.0/.
References
- Pérez Palmer N, Trejo Ortega B, Joshi P. Cognitive Impairment in Older Adults: Epidemiology, Diagnosis, and Treatment. Psychiatr Clin North Am 2022;45:639-61. [Crossref] [PubMed]
- Hamilton OKL, Backhouse EV, Janssen E, Jochems ACC, Maher C, Ritakari TE, Stevenson AJ, Xia L, Deary IJ, Wardlaw JM. Cognitive impairment in sporadic cerebral small vessel disease: A systematic review and meta-analysis. Alzheimers Dement 2021;17:665-85. [Crossref] [PubMed]
- Litak J, Mazurek M, Kulesza B, Szmygin P, Litak J, Kamieniak P, Grochowski C. Cerebral Small Vessel Disease. Int J Mol Sci 2020;21:9729. [Crossref] [PubMed]
- Chen X, Wang J, Shan Y, Cai W, Liu S, Hu M, Liao S, Huang X, Zhang B, Wang Y, Lu Z. Cerebral small vessel disease: neuroimaging markers and clinical implication. J Neurol 2019;266:2347-62. [Crossref] [PubMed]
- Zanon Zotin MC, Sveikata L, Viswanathan A, Yilmaz P. Cerebral small vessel disease and vascular cognitive impairment: from diagnosis to management. Curr Opin Neurol 2021;34:246-57. [Crossref] [PubMed]
- Ge YJ, Xu W, Ou YN, Qu Y, Ma YH, Huang YY, Shen XN, Chen SD, Tan L, Zhao QH, Yu JT. Retinal biomarkers in Alzheimer's disease and mild cognitive impairment: A systematic review and meta-analysis. Ageing Res Rev 2021;69:101361. [Crossref] [PubMed]
- Alber J, Arthur E, Sinoff S, DeBuc DC, Chew EY, Douquette L, Hatch WV, Hudson C, Kashani A, Lee CS, Montaquila S, Mozdbar S, Cunha LP, Tayyari F, Van Stavern G, Snyder PJ. A recommended "minimum data set" framework for SD-OCT retinal image acquisition and analysis from the Atlas of Retinal Imaging in Alzheimer's Study (ARIAS). Alzheimers Dement (Amst) 2020;12:e12119. [Crossref] [PubMed]
- Xu Z, Dong Y, Wang Y, Song L, Niu S, Wang S, Zhao M, Wang J, Cong L, Han X, Hou T, Tang S, Zhang Q, Du Y, Qiu C. Associations of macular microvascular parameters with cerebral small vessel disease in rural older adults: A population-based OCT angiography study. Front Neurol 2023;14:1133819. [Crossref] [PubMed]
- Gao Y, Kwapong WR, Zhang Y, Yan Y, Jin X, Tao Y, Xu H, Wu B, Zhang M. Retinal microvascular changes in white matter hyperintensities investigated by swept source optical coherence tomography angiography. BMC Ophthalmol 2022;22:77. [Crossref] [PubMed]
- O'Neill RA, Maxwell AP, Paterson EN, Kee F, Young I, Hogg RE, Cruise S, Murphy S, McGuinness B, McKay GJ. Retinal microvascular parameters are not significantly associated with mild cognitive impairment in the Northern Ireland Cohort for the Longitudinal Study of Ageing. BMC Neurol 2021;21:112. [Crossref] [PubMed]
- McGrory S, Taylor AM, Pellegrini E, Ballerini L, Kirin M, Doubal FN, Wardlaw JM, Doney ASF, Dhillon B, Starr JM, Trucco E, Deary IJ, MacGillivray TJ. Towards Standardization of Quantitative Retinal Vascular Parameters: Comparison of SIVA and VAMPIRE Measurements in the Lothian Birth Cohort 1936. Transl Vis Sci Technol 2018;7:12. [Crossref] [PubMed]
- Wu Y, Xia Y, Song Y, Zhang Y, Cai W. NFN+: A novel network followed network for retinal vessel segmentation. Neural Netw 2020;126:153-62. [Crossref] [PubMed]
- Arcadu F, Benmansour F, Maunz A, Willis J, Haskova Z, Prunotto M. Deep learning algorithm predicts diabetic retinopathy progression in individual patients. NPJ Digit Med 2019;2:92. [Crossref] [PubMed]
- Sun J, Hui Y, Li J, Zhao X, Chen Q, Li X, Wu N, Xu M, Liu W, Li R, Zhao P, Wu Y, Xing A, Shi H, Zhang S, Liang X, Wang Y, Lv H, Wu S, Wang Z. Protocol for Multi-modality MEdical imaging sTudy bAsed on KaiLuan Study (META-KLS): rationale, design and database building. BMJ Open 2023;13:e067283. [Crossref] [PubMed]
- Ilardi CR, Menichelli A, Michelutti M, Cattaruzza T, Manganotti P. Optimal MoCA cutoffs for detecting biologically-defined patients with MCI and early dementia. Neurol Sci 2023;44:159-70. [Crossref] [PubMed]
- Wardlaw JM, Smith EE, Biessels GJ, Cordonnier C, Fazekas F, Frayne R, et al. Neuroimaging standards for research into small vessel disease and its contribution to ageing and neurodegeneration. Lancet Neurol 2013;12:822-38. [Crossref] [PubMed]
- Fazekas F, Kleinert R, Offenbacher H, Schmidt R, Kleinert G, Payer F, Radner H, Lechner H. Pathologic correlates of incidental MRI white matter signal hyperintensities. Neurology 1993;43:1683-9. [Crossref] [PubMed]
- Greenberg SM, Vernooij MW, Cordonnier C, Viswanathan A, Al-Shahi Salman R, Warach S, Launer LJ, Van Buchem MA, Breteler MM. Cerebral microbleeds: a guide to detection and interpretation. Lancet Neurol 2009;8:165-74. [Crossref] [PubMed]
- Teng Z, Feng J, Lv P. Subclinical Hypothyroidism is Associated with Cognitive Impairment in Patients with Cerebral Small Vessel Disease. Neuropsychiatr Dis Treat 2023;19:303-10. [Crossref] [PubMed]
- Zhang Y, Zhang Z, Zhang M, Cao Y, Yun W. Correlation Between Retinal Microvascular Abnormalities and Total Magnetic Resonance Imaging Burden of Cerebral Small Vessel Disease in Patients With Type 2 Diabetes. Front Neurosci 2021;15:727998. [Crossref] [PubMed]
- Zhao X, Liu Y, Zhang W, Meng L, Lv B, Lv C, Xie G, Chen Y. Relationships Between Retinal Vascular Characteristics and Renal Function in Patients With Type 2 Diabetes Mellitus. Transl Vis Sci Technol 2021;10:20. [Crossref] [PubMed]
- Czakó C, Kovács T, Ungvari Z, Csiszar A, Yabluchanskiy A, Conley S, Csipo T, Lipecz A, Horváth H, Sándor GL, István L, Logan T, Nagy ZZ, Kovács I. Retinal biomarkers for Alzheimer's disease and vascular cognitive impairment and dementia (VCID): implication for early diagnosis and prognosis. Geroscience 2020;42:1499-525. [Crossref] [PubMed]
- Zhang Y, Wang Y, Shi C, Shen M, Lu F. Advances in retina imaging as potential biomarkers for early diagnosis of Alzheimer's disease. Transl Neurodegener 2021;10:6. [Crossref] [PubMed]
- Cheung CY, Wong WLE, Hilal S, Kan CN, Gyanwali B, Tham YC, Schmetterer L, Xu D, Lee ML, Hsu W, Venketasubramanian N, Tan BY, Wong TY, Chen CPLH. Deep-learning retinal vessel calibre measurements and risk of cognitive decline and dementia. Brain Commun 2022;4:fcac212. [Crossref] [PubMed]
- Cabrera DeBuc D, Somfai GM, Arthur E, Kostic M, Oropesa S, Mendoza Santiesteban C. Investigating Multimodal Diagnostic Eye Biomarkers of Cognitive Impairment by Measuring Vascular and Neurogenic Changes in the Retina. Front Physiol 2018;9:1721. [Crossref] [PubMed]
- Liu M, Lovern C, Lycett K, He M, Wake M, Wong TY, Burgner DP. The association between markers of inflammation and retinal microvascular parameters: A systematic review and meta-analysis. Atherosclerosis 2021;336:12-22. [Crossref] [PubMed]
- van der Heide FCT, van Sloten TT, Willekens N, Stehouwer CDA. Neurovascular coupling unit dysfunction and dementia: Retinal measurements as tools to move towards population-based evidence. Front Endocrinol (Lausanne) 2022;13:1014287. [Crossref] [PubMed]
- van de Kreeke JA, Legdeur N, Badissi M, Nguyen HT, Konijnenberg E, Tomassen J, Ten Kate M, den Braber A, Maier AB, Tan HS, Verbraak FD, Visser PJ. Ocular biomarkers for cognitive impairment in nonagenarians; a prospective cross-sectional study. BMC Geriatr 2020;20:155. [Crossref] [PubMed]
- Quick S, Moss J, Rajani RM, Williams A. A Vessel for Change: Endothelial Dysfunction in Cerebral Small Vessel Disease. Trends Neurosci 2021;44:289-305. [Crossref] [PubMed]
- Low A, Mak E, Rowe JB, Markus HS, O'Brien JT. Inflammation and cerebral small vessel disease: A systematic review. Ageing Res Rev 2019;53:100916. [Crossref] [PubMed]
- Markus HS, de Leeuw FE. Cerebral small vessel disease: Recent advances and future directions. Int J Stroke 2023;18:4-14. [Crossref] [PubMed]
- Marchesi N, Fahmideh F, Boschi F, Pascale A, Barbieri A. Ocular Neurodegenerative Diseases: Interconnection between Retina and Cortical Areas. Cells 2021;10:2394. [Crossref] [PubMed]
- Liao H, Zhu Z, Peng Y. Potential Utility of Retinal Imaging for Alzheimer's Disease: A Review. Front Aging Neurosci 2018;10:188. [Crossref] [PubMed]
- Langner SM, Terheyden JH, Geerling CF, Kindler C, Keil VCW, Turski CA, Turski GN, Behning C, Wintergerst MWM, Petzold GC, Finger RP. Structural retinal changes in cerebral small vessel disease. Sci Rep 2022;12:9315. [Crossref] [PubMed]
- Tang Q, Zhang Y, Yang Z, Li S, Wu M, Guo Y, Zhao W, Yin C. Study on the Interaction between the Characteristics of Retinal Microangiopathy and Risk Factors for Cerebral Small Vessel Disease. Contrast Media Mol Imaging 2022;2022:9505945. [Crossref] [PubMed]
- Cho BJ, Lee M, Han J, Kwon S, Oh MS, Yu KH, Lee BC, Kim JH, Kim C. Prediction of White Matter Hyperintensity in Brain MRI Using Fundus Photographs via Deep Learning. J Clin Med 2022;11:3309. [Crossref] [PubMed]
- Shu L, Liang J, Xun W, Yang H, Lu T. Prediction for the Total MRI Burden of Cerebral Small Vessel Disease With Retinal Microvascular Abnormalities in Ischemic Stroke/TIA Patients. Front Neurol 2020;11:268. [Crossref] [PubMed]
- Tao W, Kwapong WR, Xie J, Wang Z, Guo X, Liu J, Ye C, Wu B, Zhao Y, Liu M. Retinal microvasculature and imaging markers of brain frailty in normal aging adults. Front Aging Neurosci 2022;14:945964. [Crossref] [PubMed]
- Lv B, Li S, Liu Y, Wang W, Li H, Zhang X, Sha Y, Yang X, Yang Y, Wang Y, Zhang C, Wang Y, Lv C, Xie G, Wang K. Development and validation of an explainable artificial intelligence framework for macular disease diagnosis based on optical coherence tomography images. Retina 2022;42:456-64. [Crossref] [PubMed]
- Zhao M, Lam AK, Ying MT, Cheong AM. Hemodynamic and morphological changes of the central retinal artery in myopic eyes. Sci Rep 2022;12:7104. [Crossref] [PubMed]