Quantitative assessment of pneumothorax by using Shannon entropy of lung ultrasound M-mode image and diaphragmatic excursion based on automated measurement
Introduction
Pneumothorax is defined as the presence of excess air in the pleural cavity which caused by unknown reason, other lung diseases, trauma, or medical intervention (1-3). It may hinder lung inflation, deflation and gas exchange due to its high pleural pressure. Its clinical manifestations can express from asymptomatic to life threatening (1-3). For patients with stable pneumothorax, current clinical guidelines recommend the aggressive treatments (e.g., chest drainage) as first-line treatment; however, many studies have reported that conservative observation at the outpatient clinic until syndrome released by themselves has similar therapeutic outcomes as aggressive treatments while being more cost-effective and hospitalization exemption (3,4). In addition, timely detection and treatment can be life-saving for those tension pneumothorax or dyspnea patients who are irrespective of etiology, and even in the prehospital setting (2,3). Although pneumothorax diagnosis based on pleural manometry is intuitive, it has not received widespread clinical acceptance because of invasion nature and high interpatient variability (5-7). Computed tomography (CT) and chest X-ray (CXR) are the gold standard and primary methods, respectively, for confirming pneumothorax (1,2), with several drawbacks such as radiation exposure and incapable to perform serial images or for prehospital or bedside scanning.
Lung ultrasound (LUS) can overcome the limitations of CT and CXR for pneumothorax diagnosis. Because of the lack of radiation and low cost, LUS can be performed repeatedly without causing harm to the patients and clinicians, and budget overload. Moreover, its portability and real-time assessment capability enable clinicians to detect and monitor pneumothorax at any location—from the operating room to the bedside to outside the hospital. The LUS diagnostic criteria for pneumothorax include the presence of lung point and lung pulse, and the absence of lung sliding and A-line, with the absence of lung sliding being the most common criteria (2,8,9). Nevertheless, it is a great challenge to interpret lung sliding compared to identify it since the changes in lung sliding on traditional LUS B-mode images may be subtle from normal to severe pneumothoraces. The M-mode image records the successive positions of a structure over time and captures a still image of this dynamic process, thereby improving visualization and providing more evidence of lung sliding (10,11). On LUS M-mode images, the seashore sign indicates lung sliding and excludes pneumothorax, whereas the stratosphere or barcode sign reflects the absence of lung sliding and indicates the presence of pneumothorax (11-13). However, visual inspection of LUS M-mode images depends on the operator’s skill and experience and is subjective (14,15). To assist novice operators and alleviate the workloads of the experts, objective information obtained from the quantitative analysis of the LUS M-mode image is useful and helpful. Deep learning-based methods are powerful tools for detection of lung sliding and characterization of pneumothorax (16,17). However, requirement of large training data with labels, inconsistent system settings, scanning protocols, and annotations, and most importantly, unclear underlaying mechanisms limited their use in clinical. Methods based on the occurrence probability of pixels with different brightness values are one of the most widely used for ultrasound quantification. They have been successfully applied for monitoring the progression of Duchenne muscular dystrophy (18) and liver ablation (19); detecting cataracts (20) and middle ear effusion (21); characterizing thrombi (22); and evaluating thrombolysis (23) and hepatic steatosis (24,25). Unfortunately, raw data (i.e., radiofrequency or envelope signals) are required for the methods used in these studies, and not every ultrasound system outputs the raw data of M-mode images. Thus, a quantitative method based on the occurrence probability of standard-output LUS M-mode images can enhance the applicability of this objective method for pneumothorax diagnosis.
In information theory, Shannon entropy (ShanEn) is a metric used for measuring uncertainty, and it is proportional to the occurrence probability of message content. ShanEn is the highest when the message is totally unpredictable and decreases with content predictability (26). Pixels with different brightness values result in different visual appearances of images, representing the uncertainty and occurrence probability of messages carried by images. Therefore, ShanEn based on occurrence probability can quantify the visual appearance of an image. In addition, ShanEn estimation can be made using any type of image—raw data, grayscale, or others—because ShanEn only considers the occurrence of pixel brightness, irrespective of the underlying origins (27-29). Accordingly, the ShanEn of LUS M-mode image (ShanEnLM) can help detect and quantify pneumothorax.
The diaphragm is a dome-shaped muscle and is the main source of pressure changes in the pleural cavity during respiration. It contracts and descends during inspiration, decreasing pleural pressure and thus allowing air to flow into the lungs, whereas it relaxes and ascends during expiration, increasing pleural pressure and thus causing air to flow out of the lungs (30,31). The position difference between diaphragmatic contraction and relaxation on diaphragm ultrasound (DUS) M-mode images is known as diaphragmatic excursion (DE) (32,33). DE may be abnormal in cases of diaphragmatic dysfunction, such as during spinal cord injury (34,35), muscular dystrophy (34,36), and diaphragmatic paralysis (34,37). Pneumothorax can also cause diaphragmatic dysfunction because high pleural pressure may push the diaphragm away from its normal position. Thus, similar to the use of ShanEnLM, DE based on automated measurement (DEAM) instead of manual measurement for pneumothorax quantification is worth exploring.
In this study, we implemented ShanEnLM and DEAM as the quantitative methods for the objective diagnosis of rabbit pneumothorax to validate our assumptions. We present this article in accordance with the STARD and ARRIVE reporting checklists (available at https://qims.amegroups.com/article/view/10.21037/qims-23-636/rc).
Methods
Animal preparation
The experimental protocols of this study were approved by the Institutional Animal Care and Use Committee of China Medical University (CMUIACUC-2020-381), in compliance with the Taiwan Council of Agriculture’s Guideline for the Care and Use of Laboratory Animals. The animals, housing and experimental environments were not specific pathogen-free. Twenty-four male New Zealand rabbits weighing 2.7–3.1 kg (age: fifteen weeks) were used according to Hill et al. (38). They were housed in air-conditioned cages with free access to laboratory chow and drinking water, and conventional cleaning. Randomization was not blinded in this study. Animals assigned to lab by animal house staff with no prior knowledge of experiment to be performed. After acclimatization for 1 week, the rabbits were all healthy according to regular eating and toileting, bright eyes, no significant discharge coming from nose, and normal coat without scurf, parasites, and fleas (39).
Experimental protocols
The experiment was conducted in the operation room of China Medical University animal center after environmental sterilization by 75% ethanol. We used the pneumothorax induction methods by Hill et al. (38) and Summers et al. (40) for rabbits, with some modifications. Briefly, following an 8-h fast, each rabbit was anesthetized using 30 mg/kg pentobarbital sodium (Sigma-Aldrich, St. Louis, MO, USA). A sterile 16-gauge needle was inserted into the pleural cavity 1 cm below the right scapular tip and was connected to a 50-mL syringe and the homemade U-tube manometer through a three-way stopcock and flexible polyethylene tubes without respiratory monitoring and support by ventilator. Pneumothorax was induced only on the right side to minimize the interference from the beating heart during LUS scanning and to maximize the DUS acoustic window through the liver rather than through the spleen (31,41). Room air was insufflated into the pleural cavity at 5, 5, 5, 10, and 15 mL/kg sequentially to create excess air volumes of 5, 10, 15, 25, and 40 mL/kg, respectively. Immediately after each insufflation, LUS, DUS, and pleural pressure measurements with the homemade U-tube manometer were performed. Each insufflation only took a few seconds. However, the following scanning and measurements took 10 to 15 minutes.
A point-of-care system (LU700C, Leltek, Taipei) was employed for scanning of LUS and DUS. Before scanning, the upper bodies of the rabbits were shaved, and they were placed in the left lateral position. Transverse LUS scanning along the right midclavicular line was used to visualize the right lung surface. The M-mode interrogation line was adjusted to be perpendicular to the lung surface. We recorded 17-s M-mode images of the second to sixth intercostal spaces. Image depth, gain, dynamic range, central frequency, frame rate, pulse length, mechanical index, and thermal index were 3.2 cm, 50, 40, 3.6 MHz, 15 frames per second, 2.13 mm, 0.6, and 0.42, respectively. The synthetic transmit focusing of the plane waves (i.e., total focusing imaging without focus zone) was used without time-gain compensation. The unfavorable effects of motions on scans were minimized by manual operation of handhold transducer based on operator’s skill and experience. The scan was abandoned if the quality was not good enough according to operator’s judgments. Five qualified scans of each rabbit were saved. Transverse DUS scanning of the right subcostal area between the midsternal and anterior axillary lines was used to visualize the right diaphragmatic dome. The M-mode interrogation line was adjusted to be perpendicular to the diaphragmatic dome. Again, 17-s M-mode images were recorded. The scanning settings of DUS were the same as those of LUS, except for depth, gain, and dynamic range. To visualize the diaphragm at a deeper position, the image depth was increased to 4.7 cm. To simplify the automated measurement of DE by emphasizing the hyperechoic signal of the diaphragm through the elimination of the hypoechoic signals of its reverberations, other tissues, and noises, we set a lower gain and dynamic range: 30 and 20, respectively. One qualified scans of each rabbit were saved. The LUS and DUS M-mode grayscale images after beamforming and focusing by ultrasound system were all output in the .jpg format, with a resolution of 1,328×530. During pleural manometry, because of the oscillations of the fluid column caused by pressure swings during respiration, and open end of the homemade U-tube manometer, only average pleural pressure values relative to 1 atmosphere were obtained. Twenty measurements of each rabbit with each air insufflation volumes were taken. After LUS and DUS scanning, and pleural pressure measurements, all rabbits were immediately euthanized with an overdose of pentobarbital sodium. No rabbits died during the experiment, indicating the acceptable risk of our protocols without ventilator use.
ShanEnLM and DEAM estimations
Programs for estimating ShanEnLM and DEAM were developed using MATLAB R2016a (MathWorks, Natick, MA, USA, RRID:SCR_001622). For each LUS M-mode image, the rectangular region of interest (ROI) was manually selected. The ShanEnLMH can be estimated as follows (26):
where and are the minimal and maximal pixel brightness of ROI, respectively, and represents the occurrence probability of pixel with each brightness. Although the optimal logarithm base of ShanEn remains unclear, we used a base of 2, following the first choice in previous studies (27-29).
For each DUS M-mode image, DEAM was estimated using the following steps (Figure 1):
- A binary DUS M-mode image was binarized using Otsu’s method.
- The upper boundary between black and white regions was detected based on the first brightness transition between 0 and 1 from top to bottom.
- The local extremum of the boundary was obtained. The minimum peak separation was set to 0 (the default value of MATLAB software). Therefore, the window size of local extremum calculation was the length of whole boundary.
- The extrema values above the third quartile were regarded as the positions of the relaxed diaphragms.
- The line connecting the positions of the contracted diaphragms was acquired through the linear fitting of extrema values below the first quartile based on the assumption of the same position of the diaphragmatic contraction in each respiratory cycle.
- DEAM was defined as the average of the differences between the positions of relaxed diaphragms and their corresponding positions on the fitting line.
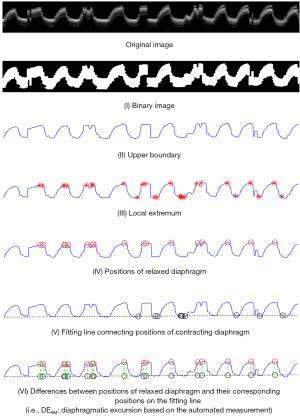
Statistical analysis
All plots and statistical analyses were performed using SigmaPlot software (Version 12.0, Systat Software, Inc., Chicago, IL, USA, RRID:SCR_003210). Because our lab lacked the dedicated pleural manometer as references, the accuracy of the homemade U-tube manometer was estimated using measurements themselves. We treated the average of 20 measurements and each measurement of pleural pressures as true and observation values of each rabbit with each air insufflation volume, respectively. The accuracy of each measurement using the homemade U-tube manometer can be estimated based on relative errors as follows (42,43):
where and are observation (i.e., each measurement) and true (i.e., the average of 20 measurements) values, respectively. The accuracy of each rabbit with each air insufflation volumes was obtained from the average of 20 measurements. The accuracy of each air insufflation volume was obtained from the average of 24 rabbits and expressed as scatter plots with mean ± standard deviation (SD). The pleural pressures of each rabbit with different air insufflation volumes were the average results of 20 measurements and illustrated using line and scatter plots with mean ± SD. ShanEnLM of each rabbit was the average of five LUS M-mode scans. ShanEnLM and DEAM as functions of air insufflation volumes were expressed as scatter plots with mean ± SD and curve fitting. The distributions of ShanEnLM and DEAM with different air insufflation volumes were derived using scatter plots, respectively, and presented as mean ± SD. Because the severity of pneumothorax has not been defined with a consensus (44), pneumothorax with air insufflation volumes of 5, 10 and 15, and 25 and 40 were denoted as mild, moderate, and severe, respectively, according to data distribution. Receiver operating characteristic curves (ROCs) illustrated performances of ShanEnLM and DEAM for differentiating normal and mild pneumothorax, mild and moderate pneumothoraces, and moderate and severe pneumothoraces. The ROC curves were produced by plotting sensitivity [true positive/(true positive + false positive)] on the y-axis against 1-specificity [true negative/(false positive + true negative)] on the x-axis under varying thresholds. Areas under the ROCs (AUROCs) with 95% confidence interval (CI) and ROC indices, including cut off value, positive predictive value [true positive/(true positive + false positive)], negative predictive value [true negative / (true negative + false negative)], and accuracy [(true positive + true negative) / (positive + negative)] were estimated.
Results
Figure 2 shows the accuracies with different air insufflation volumes. They had similar performances. Figure 3 depicts each rabbit’s pleural pressure with different air insufflation volumes. The all increased with air insufflation volumes and exhibited high interindividual variability. Figure 4 presents the representative LUS and DUS M-mode images of different air insufflation volumes. The changes of them between air insufflation volumes may be subtle and undetectable by the naked eye. In LUS M-mode images, as the air insufflation volumes increased from 0 to 15 mL/kg, the visual appearances of the subpleural region changed from the granular pattern to parallel lines, being almost restored to the granular pattern at volumes >25 mL/kg. In DUS M-mode images, DE decreased significantly as the air insufflation volumes increased from 0 to 10 mL/kg and remained stable thereafter. ShanEnLM and DEAM results supported the qualitative findings in Figure 4. Figure 5A,5B illustrate the U-shaped and exponentially decreasing fitting curves of ShanEnLM and DEAM as functions of air insufflation volumes, respectively. The determination coefficients (r2) between them and air insufflation volumes were 0.6205 and 0.8506, respectively. Figure 5C illustrates the distributions of ShanEnLM and DEAM with different air insufflation volumes based on scans of the same 24 rabbits induced by sequential insufflation of room air. ShanEnLM could differentiate between mild (i.e., air insufflation volume of 5) and moderate (i.e., air insufflation volumes of 10 and 15) pneumothoraces, and moderate and severe (i.e., air insufflation volumes of 25 and 40) pneumothoraces. In contrast, DEAM could differentiate between normal and mild pneumothorax, and mild and moderate pneumothoraces. Table 1 summarizes the AUROCs and ROC indices of ShanEnLM and DEAM for differentiating between normal and mild pneumothorax, mild and moderate pneumothoraces, and moderate and severe pneumothoraces. Either ShanEnLM or DEAM had AUROCs of 1.0000 (95% CI: 1.0000–1.0000), 0.9833 (95% CI: 0.9214–1.0000), and 0.9407 (95% CI: 0.8511–1.0000) for differentiating between normal and mild pneumothorax, mild and moderate pneumothoraces, and moderate and severe pneumothoraces, respectively, indicating the effectiveness of combination of ShanEnLM and DEAM for pneumothorax quantification.
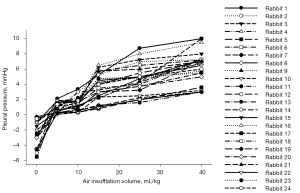
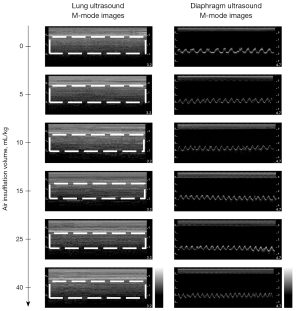
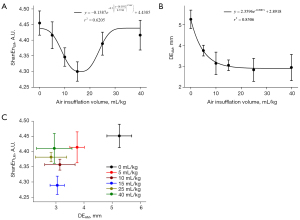
Table 1
Parameters/indices | Cut off value | AUROC (95% CI) | Sensitivity (%) | Specificity (%) | PPV (%) | NPV (%) | Accuracy (%) |
---|---|---|---|---|---|---|---|
ShanEnLM | |||||||
Normal and mild | 4.4220 | 0.7292 (0.4840–0.9735) | 75.0000 | 75.0000 | 66.6667 | 81.8182 | 75.0000 |
Mild and moderate | 4.3514 | 0.9750 (0.9135–1.0000) | 86.6667 | 100.0000 | 100.0000 | 80.0000 | 91.3043 |
Moderate and severe | 4.3514 | 0.9407 (0.8511–1.0000) | 86.6667 | 94.4444 | 92.8571 | 89.4737 | 90.9090 |
DEAM | |||||||
Normal and mild | 4.2183 | 1.0000 (1.000–1.0000) | 100.0000 | 100.0000 | 100.0000 | 100.0000 | 100.0000 |
Mild and moderate | 3.4618 | 0.9833 (0.9214–1.0000) | 90.0000 | 100.0000 | 100.0000 | 85.7143 | 93.7500 |
Moderate and severe | 2.8611 | 0.6000 (0.3278–0.8722) | 66.6667 | 70.0000 | 66.6667 | 70.0000 | 68.4211 |
ShanEnLM, Shannon entropy of lung ultrasound M-mode image; DEAM, diaphragmatic excursion based on automated measurement; AUROC, area under the receiver operating characteristics curve; CI, confidence interval; Sensitivity, [true positive/(true positive + false negative]; Specificity, [true negative/(false positive + true negative]; PPV, positive predictive value [true positive/(true positive + false positive)]; NPV, negative predictive value [true negative/(true negative + false negative)]; Accuracy, [(true positive + true negative)/(positive + negative)].
Discussion
Study significance
This study explored the feasibility of quantifying pneumothorax by using ShanEnLM and DEAM. Pneumothorax was induced in rabbits by air insufflation through a needle inserted into the pleural cavity. The pleural pressure measured using the homemade U-tube manometer was planned to be the reference for indicating pneumothorax severity. Although increasing pleural pressure indicates the successful induction of pneumothorax, high interindividual variability renders it a qualitative description rather than a quantitative reference as the same as it measured in clinical (5-7). Therefore, we replaced pleural pressure with air insufflation volumes because of the positive correlation between them of each rabbit. Our results revealed that ShanEnLM and DEAM exhibited U-shaped curves and exponentially decreasing correlations, respectively, with air insufflation volumes. Furthermore, the AUROCs of either ShanEnLM or DEAM for differentiating between normal and mild pneumothorax (i.e., air insufflation volume of 5), mild and moderate (i.e., air insufflation volumes of 10 and 15) pneumothoraces, and moderate and severe (i.e., air insufflation volumes of 25 and 40) pneumothoraces were 1.0000 (95% CI: 1.0000–1.0000), 0.9833 (95% CI: 0.9214–1.0000), and 0.9407 (95% CI: 0.8511–1.0000), respectively. The U-shaped curve between ShanEnLM and air insufflation volumes had better ability to differentiate between moderate and severe pneumothoraces. In contrast, the exponentially decreasing correlations with air insufflation volumes of DEAM had better ability to differentiate between normal and mild pneumothorax, and mild and moderate pneumothoraces. These findings demonstrate that ShanEnLM and DEAM provide objective information of pneumothorax, in particularly to combine both methods.
Effects of pneumothorax on ShanEnLM
Lung sliding refers to the cyclic to-and-fro movements of the pleural line (i.e., relative motions between the parietal and visceral pleurae) caused by lung inflation and deflation (8-11). Above the pleural line, the soft tissues of the chest wall did not affect by the lung movement and are almost static; they thus generate parallel lines on LUS M-mode images because of reverberations, which appear like waves approaching the beach. Moving pleural line, superficial lung structures and their reverberations change positions over time and create a granular pattern on LUS M-mode images, appearing like sand on beach. These two appearances together constitute the seashore sign of LUS M-mode images, indicating the presence of lung sliding and the absence of pneumothorax (2,12,13). High pleural pressure of pneumothorax separates the two pleurae. The air beneath the parietal pleura almost completely reflects the ultrasound waves, thus preventing the visualization of visceral pleura and superficial lung structures. Consequently, relative motions between two pleurae and resulting lung sliding are loss; the sand-like appearance is replaced by parallel lines, identical to that observed for tissues above the pleural line. This appearance is termed as the stratosphere or barcode sign; this indicates the absence of lung sliding and the presence of pneumothorax (2,12,13). Because the appearances above the pleural line on LUS M-mode images are similar between normal lungs and lungs with pneumothorax, this study paid more attention to the changes in subpleural appearances. For ShanEnLM estimation, the granular pattern has many pixels with different brightness values and corresponding high uncertainty. However, parallel lines only have white (i.e., lines themselves) and black (i.e., background) pixels. Thus, their uncertainty is smaller than that of the granular pattern. That ShanEnLM decreased with increasing pneumothorax severity is reasonable; however, the opposite was observed from moderate to severe pneumothorax. This finding can be explained as follows. First, the high pleural pressure in severe pneumothorax triggers intercostal muscle retraction for maintaining normal respiratory function. In the end, it further causes chest partial motion along the pleural line, which may be mistaken for normal lung sliding on LUS M-mode images (40). Second, our experimental setup may be another reason. We insufflated air into the pleural cavity from the posterior zone. The high air volume of severe pneumothorax may push the lung toward the chest wall and disrupt the air layer that separates the two pleurae during mild and moderate pneumothoraces, leading to restoration of the visualization of the visceral pleura—and lung sliding—in severe pneumothorax. Consequently, the corresponding uncertainty and ShanEnLM of severe pneumothorax may increase.
Effects of pneumothorax on DEAM
The decreasing DEAM with pneumothorax severity in our study was similar to that with pleural effusion in previous studies (45,46). External pressure on the diaphragm, such as that exerted during pneumothorax and pleural effusion, may push it caudally and reduce its length. Due to a contracted diaphragm is harder and more resistant to pressure than a relaxed diaphragm, the downward displacement of the contracted diaphragm becomes smaller than that of the relaxed diaphragm as the pleural cavity pressure increases (from normal to pneumothorax). Additionally, the shortened diaphragm is no longer the optimal length for force generation and has impaired contraction capacity against pressure according to the force-length relationship of muscle (47-49). Thus, the smaller position differences between the contracted and relaxed diaphragms during respiration (i.e., decreased DEAM) are observed.
Limitations, and future challenges and studies
First, despite the promising performance of the combination of ShanEnLM and DEAM for differentiating pneumothorax severity, the correlation between ShanEnLM and air insufflation volumes was U-shaped instead of a monotonic decrease as per the theoretical hypothesis. Future studies should include a technique unaffected by intercostal muscle retraction in severe pneumothorax and our method of air insufflation to validate our results. Second, DUS M-mode images has parameters other than DE, such as cycle and velocity of diaphragmatic contraction (30,34), which we did not evaluate. Future studies should explore their quantifications and performances in pneumothorax diagnosis. Third, although we used animal model rather than clinical data for concept validation of our proposed method in this study, the employment of commercial ultrasound system, widely available image types to ShanEnLM and DEAM, the anatomical, physiological, and biochemical similarities of lung between rabbit and human (50,51) make our proposed method easily translate to human in vivo applications. Recruitment of patients with pneumothorax is the next step of our group. Fourth, although the absence of lung sliding is the most common diagnostic criterion of pneumothorax (2,8,9), the presence of some other lung conditions (e.g., chronic obstructive pulmonary disease and large parenchymal tumor) may also have an absent lung sliding and confuse clinicians (1-3,52). Differentiating pneumothorax from these conditions based on our proposed method may be challenging, fortunately, using the bedside LUS in emergency (BLUE) protocol (53-55), the detection of other parameters, such as the B-line artifact, consolidation, and lung pulse, can help overcome this limitation. The integration of the BLUE protocol, ShanEnLM, and DEAM is the future direction of our group.
Conclusions
In this study, ShanEn of LUS M-mode images (i.e., ShanEnLM) and DE based on the automated measurement of DUS M-mode images (i.e., DEAM) was applied as new quantitative methods for the objective diagnosis of pneumothorax in a rabbit model. Our study provided two key findings: (I) ShanEnLM and DEAM had U-shaped and exponentially decreasing correlations with air insufflation volumes, respectively, and (II) either ShanEnLM or DEAM had AUROCs of 1.0000 (95% CI: 1.0000–1.0000), 0.9833 (95% CI: 0.9214–1.0000), and 0.9407 (95% CI: 0.8511–1.0000) for differentiating between normal and mild pneumothorax (i.e., air insufflation volume of 5), mild and moderate (i.e., air insufflation volumes of 10 and 15) pneumothoraces, and moderate and severe (i.e., air insufflation volumes of 25 and 40) pneumothoraces, respectively. The combination of ShanEnLM and DEAM has great potential for diagnosing and quantifying pneumothorax.
Acknowledgments
This manuscript was edited by Wallace Academic Editing.
Funding: This work was supported by
Footnote
Reporting Checklist: The authors have completed the STARD and ARRIVE reporting checklists. Available at https://qims.amegroups.com/article/view/10.21037/qims-23-636/rc
Conflicts of Interest: All authors have completed the ICMJE uniform disclosure form (available at https://qims.amegroups.com/article/view/10.21037/qims-23-636/coif). All authors report that they received research funding from China Medical University Hospital (grant No. DMR-104-024) and Ministry of Science and Technology of Taiwan (grant No. MOST 109-2221-E-039-001-MY3). The authors have no other conflicts of interest to declare.
Ethical Statement: The authors are accountable for all aspects of the work in ensuring that questions related to the accuracy or integrity of any part of the work are appropriately investigated and resolved. This study was approved by the Institutional Animal Care and Use Committee of China Medical University (CMUIACUC-2020-381), in compliance with the Taiwan Council of Agriculture’s Guideline for the Care and Use of Laboratory Animals.
Open Access Statement: This is an Open Access article distributed in accordance with the Creative Commons Attribution-NonCommercial-NoDerivs 4.0 International License (CC BY-NC-ND 4.0), which permits the non-commercial replication and distribution of the article with the strict proviso that no changes or edits are made and the original work is properly cited (including links to both the formal publication through the relevant DOI and the license). See: https://creativecommons.org/licenses/by-nc-nd/4.0/.
References
- Huan NC, Sidhu C, Thomas R. Pneumothorax: Classification and Etiology. Clin Chest Med 2021;42:711-27. [Crossref] [PubMed]
- Tran J, Haussner W, Shah K. Traumatic Pneumothorax: A Review of Current Diagnostic Practices And Evolving Management. J Emerg Med 2021;61:517-28. [Crossref] [PubMed]
- Welch H, Walker S, Maskell N. Current Management Strategies for Primary Spontaneous Pneumothorax. Curr Pulmonol Rep 2020;9:56-62.
- Brown SGA, Ball EL, Perrin K, Asha SE, Braithwaite I, Egerton-Warburton D, Jones PG, Keijzers G, Kinnear FB, Kwan BCH, Lam KV, Lee YCG, Nowitz M, Read CA, Simpson G, Smith JA, Summers QA, Weatherall M, Beasley R. Conservative versus Interventional Treatment for Spontaneous Pneumothorax. N Engl J Med 2020;382:405-15. [Crossref] [PubMed]
- Lee HJ, Yarmus L, Kidd D, Ortiz R, Akulian J, Gilbert C, Hughes A, Thompson RE, Arias S, Feller-Kopman D. Comparison of pleural pressure measuring instruments. Chest 2014;146:1007-12. [Crossref] [PubMed]
- Zielinska-Krawczyk M, Krenke R, Grabczak EM, Light RW. Pleural manometry-historical background, rationale for use and methods of measurement. Respir Med 2018;136:21-8. [Crossref] [PubMed]
- Hu K, Chopra A, Huggins JT, Nanchal R. Pleural manometry: techniques, applications, and pitfalls. J Thorac Dis 2020;12:2759-70. [Crossref] [PubMed]
- Rose G, Siadecki S, Tansek R, Baranchuk N, Saul T. A novel method of assessing for lung sliding using Doppler imaging. Am J Emerg Med 2017;35:1738-42. [Crossref] [PubMed]
- Xiao R, Shao Q, Zhao N, Liu F, Qian KJ. Quantification analysis of pleural line movement for the diagnosis of pneumothorax. World J Clin Cases 2021;9:5889-99. [Crossref] [PubMed]
- Summers SM, Chin EJ, Long BJ, Grisell RD, Knight JG, Grathwohl KW, Ritter JL, Morgan JD, Salinas J, Blackbourne LH. Computerized Diagnostic Assistant for the Automatic Detection of Pneumothorax on Ultrasound: A Pilot Study. West J Emerg Med 2016;17:209-15. [Crossref] [PubMed]
- Avila J, Smith B, Mead T, Jurma D, Dawson M, Mallin M, Dugan A. Does the Addition of M-Mode to B-Mode Ultrasound Increase the Accuracy of Identification of Lung Sliding in Traumatic Pneumothoraces? J Ultrasound Med 2018;37:2681-7. [Crossref] [PubMed]
- Ding W, Shen Y, Yang J, He X, Zhang M. Diagnosis of pneumothorax by radiography and ultrasonography: a meta-analysis. Chest 2011;140:859-66. [Crossref] [PubMed]
- Husain LF, Hagopian L, Wayman D, Baker WE, Carmody KA. Sonographic diagnosis of pneumothorax. J Emerg Trauma Shock 2012;5:76-81. [Crossref] [PubMed]
- Alrajab S, Youssef AM, Akkus NI, Caldito G. Pleural ultrasonography versus chest radiography for the diagnosis of pneumothorax: review of the literature and meta-analysis. Crit Care 2013;17:R208. [Crossref] [PubMed]
- Blehar DJ, Barton B, Gaspari RJ. Learning curves in emergency ultrasound education. Acad Emerg Med 2015;22:574-82. [Crossref] [PubMed]
- Boice EN, Hernandez Torres SI, Knowlton ZJ, Berard D, Gonzalez JM, Avital G, Snider EJ. Training Ultrasound Image Classification Deep-Learning Algorithms for Pneumothorax Detection Using a Synthetic Tissue Phantom Apparatus. J Imaging 2022;8:249. [Crossref] [PubMed]
- Montgomery S, Li F, Funk C, Peethumangsin E, Morris M, Anderson JT, Hersh AM, Aylward S. Detection of pneumothorax on ultrasound using artificial intelligence. J Trauma Acute Care Surg 2023;94:379-84. [Crossref] [PubMed]
- Hughes MS, Marsh JN, Wallace KD, Donahue TA, Connolly AM, Lanza GM, Wickline SA. Sensitive ultrasonic detection of dystrophic skeletal muscle in patients with duchenne muscular dystrophy using an entropy-based signal receiver. Ultrasound Med Biol 2007;33:1236-43. [Crossref] [PubMed]
- Li S, Tsui PH, Song S, Wu W, Zhou Z, Wu S. Detection of microwave ablation coagulation areas using ultrasound Nakagami imaging based on Gaussian pyramid decomposition: A feasibility study. Ultrasonics 2022;124:106758. [Crossref] [PubMed]
- Caixinha M, Jesus DA, Velte E, Santos MJ, Santos JB. Using ultrasound backscattering signals and Nakagami statistical distribution to assess regional cataract hardness. IEEE Trans Biomed Eng 2014;61:2921-9. [Crossref] [PubMed]
- Chen CK, Wan YL, Hsieh LC, Tsui PH. Transmastoid Ultrasound Detection of Middle Ear Effusion and Its Association with Clinical Audiometric Tests. Life (Basel) 2022.
- Fang J, Chen CK, Peng JY, Hsu CH, Jeng YM, Lee YH, Lin JJ, Tsui PH. Changes in backscattered ultrasonic envelope statistics as a function of thrombus age: an in vitro study. Ultrasound Med Biol 2015;41:498-508. [Crossref] [PubMed]
- Fang J, Tsui PH. Evaluation of thrombolysis by using ultrasonic imaging: an in vitro study. Sci Rep 2015;5:11669. [Crossref] [PubMed]
- Sato Y, Tamura K, Mori S, Tai DI, Tsui PH, Yoshida K, Hirata S, Maruyama H, Yamaguchi T. Fatty liver evaluation with double-Nakagami model under low-resolution conditions. Jpn J Appl Phys 2021;60:SDDE06.
- Wear KA, Han A, Rubin JM, Gao J, Lavarello R, Cloutier G, Bamber J, Tuthill T. US Backscatter for Liver Fat Quantification: An AIUM-RSNA QIBA Pulse-Echo Quantitative Ultrasound Initiative. Radiology 2022;305:526-37. [Crossref] [PubMed]
- Shannon CE. A mathematical theory of communication. Bell Syst Tech J 1948;27:379-423.
- Tsui PH, Wan YL. Effects of fatty infiltration of the liver on the Shannon entropy of ultrasound backscattered signals. Entropy 2016;18:341.
- Zhou Z, Tai DI, Wan YL, Tseng JH, Lin YR, Wu S, Yang KC, Liao YY, Yeh CK, Tsui PH. Hepatic Steatosis Assessment with Ultrasound Small-Window Entropy Imaging. Ultrasound Med Biol 2018;44:1327-40. [Crossref] [PubMed]
- Fang J, Chang NF, Tsui PH. Performance Evaluations on Using Entropy of Ultrasound Log-Compressed Envelope Images for Hepatic Steatosis Assessment: An In Vivo Animal Study. Entropy (Basel) 2018.
- Zambon M, Greco M, Bocchino S, Cabrini L, Beccaria PF, Zangrillo A. Assessment of diaphragmatic dysfunction in the critically ill patient with ultrasound: a systematic review. Intensive Care Med 2017;43:29-38. [Crossref] [PubMed]
- Laghi FA Jr, Saad M, Shaikh H. Ultrasound and non-ultrasound imaging techniques in the assessment of diaphragmatic dysfunction. BMC Pulm Med 2021;21:85. [Crossref] [PubMed]
- Bobbia X, Clément A, Claret PG, Bastide S, Alonso S, Wagner P, Tison T, Muller L, de La Coussaye JE. Diaphragmatic excursion measurement in emergency patients with acute dyspnea: toward a new diagnostic tool? Am J Emerg Med 2016;34:1653-7. [Crossref] [PubMed]
- Palkar A, Narasimhan M, Greenberg H, Singh K, Koenig S, Mayo P, Gottesman E. Diaphragm Excursion-Time Index: A New Parameter Using Ultrasonography to Predict Extubation Outcome. Chest 2018;153:1213-20. [Crossref] [PubMed]
- Dubé BP, Dres M. Diaphragm Dysfunction: Diagnostic Approaches and Management Strategies. J Clin Med 2016;5:113. [Crossref] [PubMed]
- Zhu Z, Li J, Yang D, Gao F, Du L, Yang M. Ultrasonographic evaluation of diaphragm thickness and excursion in patients with cervical spinal cord injury. J Spinal Cord Med 2021;44:742-7. [Crossref] [PubMed]
- van Doorn JLM, Pennati F, Hansen HHG, van Engelen BGM, Aliverti A, Doorduin J. Respiratory muscle imaging by ultrasound and MRI in neuromuscular disorders. Eur Respir J 2021;58:2100137. [Crossref] [PubMed]
- Yajima W, Yoshida T, Kondo T, Uzura M. Respiratory failure due to diaphragm paralysis after brachial plexus injury diagnosed by point-of-care ultrasound. BMJ Case Rep 2022;15:e246923. [Crossref] [PubMed]
- Hill RC, DeCarlo DP Jr, Hill JF, Beamer KC, Hill ML, Timberlake GA. Resolution of experimental pneumothorax in rabbits by oxygen therapy. Ann Thorac Surg 1995;59:825-7; discussion 827-8. [Crossref] [PubMed]
- Moyes S. Caring for rabbits in practice. Vet Nurs J 2014;29:123-5.
- Summers SM, Chin EJ, April MD, Grisell RD, Lospinoso JA, Kheirabadi BS, Salinas J, Blackbourne LH. Diagnostic accuracy of a novel software technology for detecting pneumothorax in a porcine model. Am J Emerg Med 2017;35:1285-90. [Crossref] [PubMed]
- Boussuges A, Gole Y, Blanc P. Diaphragmatic motion studied by m-mode ultrasonography: methods, reproducibility, and normal values. Chest 2009;135:391-400. [Crossref] [PubMed]
- Sanders NR. Measuring forecast accuracy: some practical suggestions. Prod Inventory Manag J 1997;38:43-6.
- Kitchenham BA, Pickard LM, MacDonell SG, Shepperd MJ. What accuracy statistics really measure. IEE proc Softw 2001;148:81-5.
- Feller-Kopman D, Light R. Pleural Disease. N Engl J Med 2018;378:740-51. [Crossref] [PubMed]
- Umbrello M, Mistraletti G, Galimberti A, Piva IR, Cozzi O, Formenti P. Drainage of pleural effusion improves diaphragmatic function in mechanically ventilated patients. Crit Care Resusc 2017;19:64-70.
- Aguilera Garcia Y, Palkar A, Koenig SJ, Narasimhan M, Mayo PH. Assessment of Diaphragm Function and Pleural Pressures During Thoracentesis. Chest 2020;157:205-11. [Crossref] [PubMed]
- Braun NM, Arora NS, Rochester DF. Force-length relationship of the normal human diaphragm. J Appl Physiol Respir Environ Exerc Physiol 1982;53:405-12. [Crossref] [PubMed]
- Fogarty MJ, Sieck GC. Diaphragm Muscle Adaptations in Health and Disease. Drug Discov Today Dis Models 2019;29-30:43-52. [Crossref] [PubMed]
- van der Pijl RJ, Granzier HL, Ottenheijm CAC. Diaphragm contractile weakness due to reduced mechanical loading: role of titin. Am J Physiol Cell Physiol 2019;317:C167-76. [Crossref] [PubMed]
- Matute-Bello G, Frevert CW, Martin TR. Animal models of acute lung injury. Am J Physiol Lung Cell Mol Physiol 2008;295:L379-99. [Crossref] [PubMed]
- Kamaruzaman NA, Kardia E, Kamaldin N. ', Latahir AZ, Yahaya BH. The rabbit as a model for studying lung disease and stem cell therapy. Biomed Res Int 2013;2013:691830. [Crossref] [PubMed]
- Quick JA, Uhlich RM, Ahmad S, Barnes SL, Coughenour JP. In-flight ultrasound identification of pneumothorax. Emerg Radiol 2016;23:3-7. [Crossref] [PubMed]
- Lichtenstein DA, Mezière GA. Relevance of lung ultrasound in the diagnosis of acute respiratory failure: the BLUE protocol. Chest 2008;134:117-25. [Crossref] [PubMed]
- Mayo PH, Copetti R, Feller-Kopman D, Mathis G, Maury E, Mongodi S, Mojoli F, Volpicelli G, Zanobetti M. Thoracic ultrasonography: a narrative review. Intensive Care Med 2019;45:1200-11. [Crossref] [PubMed]
- Peng QY, Liu LX, Zhang Q, Zhu Y, Zhang HM, Yin WH, He W, Shang XL, Chao YG, Lv LW, Wang XT, Zhang LN. Lung ultrasound score based on the BLUE-plus protocol is associated with the outcomes and oxygenation indices of intensive care unit patients. J Clin Ultrasound 2021;49:704-14. [Crossref] [PubMed]