The role of large language models in medical image processing: a narrative review
Introduction
Medical image processing technology has rapidly evolved over the past few decades. The relentless advancement and upgrading of imaging modalities, including X-rays, computed tomography (CT), magnetic resonance imaging (MRI), and positron emission tomography (PET), have furnished increasingly precise image data for clinical diagnoses (1). The global medical image analysis software market size was valued at USD 2.80 billion in 2021 and is expected to grow at a compound annual growth rate of 7.8% during the forecast period (from 2023 to 2030) (2). However, with the exponential surge in medical image data, traditional manual image recognition methods grapple with significant challenges, including diagnostic accuracy, reporting velocity, and manual image analysis’s labor-intensive, inconsistent quality. The integration of artificial intelligence (AI) holds the potential to mitigate some of these issues effectively. For instance, specific AI-assisted software now available for X-ray interpretation can autonomously detect and highlight abnormalities, thus aiding physicians in rapidly locating problem areas and expediting diagnosis (3). As AI medical imaging extends its applicability across varied modalities, diseases, and scenarios, it substantially alters conventional image diagnostic analysis procedures. The image analysis paradigm is shifting from qualitative to quantitative, curbing the influence of doctors’ subjective judgments on diagnoses and lessening the workload of imaging departments.
Nonetheless, several impediments exist to applying current machine learning methods in radiological clinical diagnosis and treatment, including the uniqueness of data sources, the interpretability of models, and insufficient interaction with radiologists. Furthermore, existing models in clinical diagnosis lack broad applicability and necessitate vast quantities of high-quality labeled datasets for diverse diseases, the acquisition of which is often costly and challenging (4,5).
Conversely, large language models (LLMs), typified by the Generative Pre-trained Transformer (GPT) series models, have significantly progressed in interactivity, universality, and data diversity. These models exhibit powerful representational learning capabilities, enabling them to comprehend, generate, and process various text and image data types (6). Despite their limited utilization in medical image recognition, they have considerable application potential.
This review focuses on the significant role of LLMs in enhancing transfer learning efficiency, integrating multimodal data, improving clinical interactivity, and reducing costs in medical image processing. Firstly, it explains the principles underlying LLMs and their advantages over previous models in medical image processing, including Transformer architecture and pre-training. Secondly, it explores LLMs’ potential advancements, including improved transfer learning efficacy, multimodal data integration, and enhanced clinical interactivity through presented clinical cases. Lastly, it examines challenges facing LLMs in medical image processing, such as data privacy, model generalization, and clinician communication. We show in Figure 1 a potential flow chart for the clinical application of LLMs. Through this article, we aim to impart to researchers and practitioners a comprehensive understanding of LLMs’ application in medical image processing from a clinical standpoint, thereby contributing to the further progression of this field. We present this article in accordance with the Narrative Review reporting checklist (available at https://qims.amegroups.com/article/view/10.21037/qims-23-892/rc).
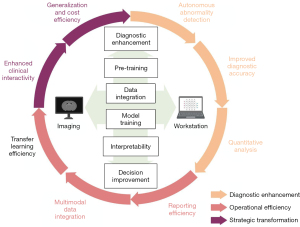
Methods
We conducted a literature search on the Web of Science and PubMed electronic databases for articles published from 2013 to 2023 to include updated data on the transformations of LLMs in Medical Imaging Processing. The search included combinations of the keywords “transformer”, “GPT”, “LLMs”, “medical image processing”, “segmentation”, “AI”, “neural network”, “machine learning”, “multimodal”, “transfer learning”, and “interpretability”. Recent publications on the arXiv database were also reviewed. There were no exclusions on article type, and abstracts, review articles, letters and editorials were also considered. The publication language was restricted to English to facilitate further literature content analysis. The summary of search strategy is illustrated in Table 1 and the detailed search strategy is shown in Table 2.
Table 1
Items | Specification |
---|---|
Date of search | Mar. 27th, 2023 |
Databases and other sources searched | Web of Science, PubMed, arXiv |
Search terms used | “Transformer”, “GPT”, “LLMs”, “medical image processing”, “segmentation”, “AI”, “neural network”, “machine learning”, “multimodal”, “transfer learning”, “interpretability” |
Timeframe | From 2013 to 2023 |
Inclusion and exclusion criteria | No study type exclusion, restricted to English articles |
Selection process | Two authors, Dianzhe Tian and Shitao Jiang, independently review for thematic relevance. In case of disagreement, a third author, Yiyao Xu, serves as an arbitrator, and the decision for inclusion is made only when all three authors are in an agreement |
GPT, Generative Pre-trained Transformer; LLMs, large language models; AI, artificial intelligence.
Table 2
Set | Search strategy of our study |
---|---|
#1 | TS = (“segmentation” OR “AI” OR “neural network” OR “machine learning” OR “multimodal” OR “transfer learning” OR “interpretability”) |
#2 | TS = (“GPT” OR “LLMs” OR “transformer”) |
#3 | TS = (“medical image processing” OR “segmentation”) |
#4 | #1 AND #2 AND #3 |
TS, topic; AI, artificial intelligence; GPT, Generative Pre-trained Transformer; LLMs, large language models.
Transformer architecture, the principle of LLMs
Prevalent deep learning models in medical imaging encompass Convolutional Neural Networks (CNNs), Recurrent Neural Networks (RNNs), and U-Net. Despite significantly enhancing diagnostic efficiency compared to manual interpretation, these models still bear certain limitations in medical imaging processing, as delineated in Table 3 (7-10). These previous models rely on local and sequential operations that limit their ability to model long-range dependencies and global context. Nevertheless, the Transformer architecture can capture global and long-range dependencies among pixels or patches without using recurrence or convolutions through self-attention mechanisms, which allows model versatility (11,12).
Table 3
Architecture | First applied in medical image processing | Features | Difficulties |
---|---|---|---|
CNN | 1998 | 1. Suitable for image classification, segmentation, and detection | 1. Extract local detailed information from images |
2. Extract image features through multiple layers of convolutional and pooling operations | 2. Sensitive to changes in the input image size | ||
3. Pretrained models for transfer learning | |||
RNN | 2001 | 1. Handle text and speech | 1. Long sequential data |
2. Model input data through the calculation of recurrent neurons | 2. Tackle noise or outliers in the input data | ||
3. Able to process current information while retaining historical information | 3. Training process is prone to the vanishing or exploding gradient problem | ||
U-Net | 2015 | 1. Specialized for medical image segmentation | Extract complex shapes and texture information from images |
2. Effectively compress image information while preserving high-resolution features | |||
3. Fewer parameters and trained faster | |||
Transformer | 2021 | 1. Handle text, audio, and images | 1. A lot of computing resources and time |
2. Self-attention to learn global dependencies | 2. Long-term dependency problems when processing long sequential data | ||
3. Parallelizes the processing of multiple sequence elements | 3. Extract local information in input data | ||
4. Pretrained models for transfer learning |
CNN, Convolutional Neural Network; RNN, Recurrent Neural Network.
Initially designed for natural language processing (NLP) tasks like machine translation and text summarization, the Transformer architecture comprises an encoder and a decoder, featuring multiple layers of self-attention and feed-forward sublayers. Self-attention enables the model to understand dependencies and relationships within input or output sequences without recurrent or convolutional operations (13). The feed-forward sublayers consist of fully connected layers with nonlinear activation functions. This architecture offers advantages over previous NLP models, including enhanced parallelization, reduced latency, and improved long-term dependency modeling (13). LLMs, a subset of NLP models, undergo training on extensive text corpora to grasp statistical patterns and semantic representations of natural language. They can be fine-tuned on task-specific data or employed as feature extractors. LLMs predominantly rely on the Transformer architecture due to their superior performance and adaptability. Utilizing the self-attention mechanism inherent to the Transformer, LLMs capture contextual and syntactic information in natural language at various levels of detail, enabling the generation of coherent and fluent text based on learned representations (14). The advancements in medical image recognition attributed to LLMs derive from the enhancement of NLP capabilities, the adoption of the Transformer architecture, and the value added through pre-training and fine-tuning (15).
In the clinical process, medical image reports usually contain much textual information, including imaging findings, diagnoses, and medical treatment. Leveraging NLP techniques such as entity recognition, relation extraction, and text classification to analyze and mine these reports allows for the automatic extraction of essential information, thus alleviating physicians’ manual extraction workload. Furthermore, integrating NLP capabilities with image segmentation techniques enables the utilization of clinical data, such as patient history and examination outcomes, to aid image analysis, thereby augmenting the accuracy of image diagnoses (16).
Current applications of transformer architecture in medical image processing
Despite the original design of the Transformer architecture to address NLP challenges, researchers have efficaciously extended its application to medical image processing tasks in recent years. Within medical image processing, the Transformer architecture can deconstruct images into a succession of local features and apprehend the interrelations among these features via self-attention mechanisms. This capability allows the model to comprehend and process image data more effectively, enhancing image recognition and analysis accuracy. Furthermore, the amalgamation of image features with textual information permits the Transformer architecture to integrate and merge multimodal data, thereby providing a more comprehensive basis for clinical diagnosis (17).
Within the domain of medical imaging, several models predicated on the Transformer architecture, like Vision Transformer (ViT), Data-efficient Image Transformer (DeiT), Transformer U-Net (TransUNet) and Radiology Transformer (RadFormer), have found applications in image analysis and natural language generation, as exemplified in Table 4 (7,17-20). In practical deployments, the task’s characteristics and requirements should be thoroughly contemplated to select an appropriate neural network architecture. Simultaneously, careful parameter tuning and optimization should be conducted to achieve superior performance.
Table 4
Models | Year | Provided by | Functions |
---|---|---|---|
ViT | 2020 | 1. Converting the pixel data in medical images into a set of feature vectors, and then using Transformer to classify or predict these feature vectors | |
2. Better performance than traditional CNNs in many computer vision tasks | |||
3. Strong scalability | |||
DeiT | 2021 | 1. Apply Transformer to learn features from medical images and then uses them for classification tasks | |
2. Comparable performance to traditional CNNs with less training data | |||
TransUNet | 2021 | Max Planck Society of Germany | 1. Perform semantic segmentation tasks in medical images while generating natural language descriptions |
2. Use Transformer in the encoder to combine medical image feature extraction and semantic segmentation processes | |||
RadFormer | 2021 | University of Toronto & University of North Carolina in Canada | 1. Segment different parts of medical images and generate corresponding natural language descriptions |
2. Applied to tasks such as segmentation, registration, and classification in medical imaging |
ViT, Vision Transformer; CNN, Convolutional Neural Network; DeiT, Data-efficient Image Transformer; TransUNet, Transformer U-Net; RadFormer, Radiology Transformer.
Pre-training and fine-tuning stand as principal factors underpinning the success of LLMs. The model can assimilate extensive language knowledge and generalized representations by pre-training on a vast corpus. Then, by fine-tuning specific tasks, the model can apply these general representations to concrete problems, thus achieving efficient transfer learning (15). In medical image processing, pre-training and fine-tuning also hold substantial potential value. Primarily, through pre-training, LLMs can glean comprehensive medical knowledge from a considerable quantity of medical text, encompassing pathological features, clinical manifestations, and treatment methodologies of diseases. Then, by fine-tuning specific medical image processing tasks, the model can apply this knowledge to problems, improving diagnostic accuracy and efficiency. In addition, pre-training and fine-tuning can also reduce the cost of data annotation in medical image processing. The annotation of medical image data usually requires the participation of professional doctors, making data annotation a time-consuming and costly process (21).
Nevertheless, the model can leverage actual annotated data for transfer learning through pre-training and fine-tuning, accomplishing commendable performance even with a limited quantity of annotated data. This provision presents a more cost-effective and efficient solution for research and applications within medical image processing. Finally, pre-training and fine-tuning also help speed up model training. Since LLMs have already been trained on large-scale corpora, the training process on specific tasks can be relatively short (15). This enables researchers and developers to quickly develop and optimize medical image processing systems and better meet clinical and research needs.
In conclusion, the enhancement of NLP capabilities, the deployment of the Transformer architecture for processing image data, and the efficient transfer learning realized through pre-training and fine-tuning lay the theoretical groundwork for the progressively important role of LLMs in medical image processing.
Potential advantages of LLMs
Leveraging the potent NLP capabilities and Transformer architecture inherent in LLMs such as GPT-4, these models demonstrate numerous unique advantages over preceding deep learning models. These benefits encompass, but are not confined to, the integration of multimodal data, pre-training and transfer learning, greatest generality, and enhanced interactivity (22). Collectively, these benefits lay a robust foundation for their further utilization in medical imaging.
Multimodal data integration
Multimodal data integration involves amalgamating data from disparate sensors, mediums, or formats to create a more comprehensive and enriched view of the data. These data may include various forms, such as images, videos, audio, and texts, which can describe the same event, scene, or object from multiple perspectives. By integrating such data, we can enhance the accuracy, reliability, and usability of the data, thus supporting an expanded array of applications and analyses. In medical imaging, multimodal data takes the form of medical imaging data combined with other types of data, such as medical records and laboratory results. LLMs enable multimodal data integration aided by the Transformer architecture. This principle involves employing the Transformer model to encode data from various modalities. For instance, in medical image processing, the model can use the Transformer model to encode medical images and use the encoded features for downstream tasks such as classification, segmentation, and registration. Additionally, the model can use the Transformer model to encode natural language text and combine the encoded features with the encoded image features to achieve joint processing of images and text. This multimodal encoding approach can efficiently harness the correlations between different data modalities, enhancing the model’s performance and generalization ability (23,24).
From a clinical standpoint, integrating medical text data with medical imaging data empowers the model to furnish more comprehensive diagnostic evidence, thereby bolstering clinical decision-making. Different data modalities can yield diverse information; for instance, the fusion of MRI and PET images could enhance the accuracy of tumor detection, or the amalgamation of MRI and electroencephalogram (EEG) data could improve diagnostic precision in brain diseases (3). When paired with the contextual memory capability of LLMs, the system can address issues caused by inadequate or missing data through multiple information sources. Moreover, when sufficient data are available, it can synthesize diagnostic information from various sources, thereby mimicking a clinician’s diagnostic and therapeutic processes and objectively augmenting the application’s usability, accuracy, and robustness.
Multiple research teams are currently developing Interactive Computer-Aided Diagnosis systems that utilize multimodal features and LLMs. One such system is Chat Computer-aided Diagnosis (ChatCAD) (25). This research has proposed an initial method for incorporating LLMs into medical image Computer-Aided Diagnosis (CAD) networks. The technique involves using LLMs to enhance the output of various CAD networks by combining and reformatting information in natural language text format. With this approach, the system can take an X-ray image, generate an analysis report, and facilitate multiple rounds of dialogue and question-answering about the disease. This study has successfully established a strong correlation between image and text data. To convert medical images into text content for input to the LLM, the researchers used a trained CAD model to produce natural language output. The LLM was then employed to summarize the results and generate the final summary. Finally, using these results and a language model pre-trained on medical knowledge, the system engaged in conversations about symptoms, diagnosis, and treatment (25). It is noteworthy that this system replicated a clinician’s diagnostic and therapeutic process, even without chief complaints serving as prompts due to the lack of suitable datasets. This underscores the immense potential of multimodal data integration for assisted diagnosis in medical imaging.
The effectiveness of transfer learning
Before executing their tasks, numerous contemporary deep learning models necessitate vast amounts of labeled training data to yield effective outcomes. This limitation hampers the effectiveness of models in applications where acquiring expert annotation is challenging and leads to prolonged model training cycles. However, leveraging the advantages of the Transformer architecture, the pre-training and fine-tuning strategies employed by LLMs facilitate transfer learning, thereby expediting model training and reducing annotation costs (15). This shortens the training process on specific tasks, enabling researchers and developers to develop and optimize medical image processing systems more economically and efficiently.
Although the effectiveness of transfer learning and semi-supervised self-training has yet to be maturely evaluated in the medical imaging field, the studies conducted by Shang Gao and J. Blair Christian’s team exploring the efficacy of early LLM Bidirectional Encoder Representations from Transformers (BERT) transfer learning and semi-supervised self-training in Named Entity Recognition (NER) could be a reference point (26). They enhanced the performance of the NER model within a biomedical environment with minimal labeled data. Initially, they pre-trained BiLSTM-Conditional Random Field (CRF) and BERT models on extensive general biomedical NER corpora such as MedMentions or Semantic Medline. They then fine-tuned these models on more specific target NER tasks with limited training data. They ultimately utilized semi-supervised self-training with unlabeled data to further enhance model performance. The experimental results showed that combining transfer learning with self-training can enable NER models to perform the same as models trained on 3 to 8 times more labeled data in NER tasks focusing on common biomedical entity types. This underscores the effectiveness of transfer learning in LLMs within the NER realm. Analogous assertions can be made in the field of medical image recognition. Recently, attempts have been made in medical image segmentation. A new medical image segmentation model called MedSAM was introduced. Segment Anything Model (SAM) is a foundation model for natural image segmentation trained on more than 1 billion masks and has strong capabilities for generating accurate object masks based on prompts or in a fully automatic manner (27).
Nevertheless, the team developed a simple fine-tuning method to adapt the SAM for general medical image segmentation. Experimental results show that MedSAM achieves an average Dice similarity coefficient of 22.5% and 17.6% in 3D and 2D segmentation tasks, respectively, outperforming the default SAM (27,28). This research outcome demonstrates the tremendous potential of fine-tuning LLMs in medical imaging.
In addition, by using LLMs for transfer learning, researchers and developers can quickly transfer knowledge between different medical image processing tasks, further improving model performance and universality (29). A groundbreaking initiative, the cross-lingual biomedical entity linking project XL-BEL, has been introduced, accompanied by a comprehensive ten-language XL-BEL benchmark. This novel approach leverages external, domain-specific knowledge to enhance the proficiency of pre-trained language models in managing complex professional tasks, spanning multiple languages. Advancing the scope of knowledge transfer capabilities across diverse medical imaging disciplines is a critical area of inquiry in the sphere of LLM transfer learning.
Enhanced clinical interactivity
LLMs, like ChatGPT, derive their interactivity from powerful NLP abilities, enabling them to engage in natural language dialogues with users. These models can receive user text inputs, interpret their intent through prompts, and generate corresponding text responses. Such interactivity is accomplished by training these models on language processing tasks like understanding language rules, grammar, and vocabulary, thereby simulating human-like response patterns in natural language dialogues. This level of interactivity signifies an advancement in computer-aided diagnosis in the medical field. Current advanced AI interactive applications in clinical medical imaging, including DeepLesion, Med3D, CIRRUS AI, and EnvoyAI, incorporate tools for 2D and 3D tumor localization and annotation, 3D segmentation and reconstruction, visualization, and visual analysis (30-32). Although these interactions can assist doctors in their work, they are limited to providing all image information to doctors without selection, more convenient tools, and more precise visual effects. However, these systems cannot optimize the diagnosis and treatment plan or selectively provide information according to the doctor’s specific needs. The robust interactivity of LLMs makes all this possible. Doctors can select imaging data from distinct areas, structures, and tissues using specific prompts for closer examination. With current 3D segmentation and reconstruction techniques, the diagnosis and treatment process can be more targeted. Of course, achieving this vision requires further improvement in image segmentation technology. However, the advanced segmentation capabilities of models like SAM have begun to reveal the potential of future versatile segmentation models (27,28).
Furthermore, LLMs boost their transparency during the interaction. While the decision-making process of LLMs, akin to other deep learning algorithms, is only partially comprehensible for humans, these models can offer in-depth text explanations and reasoning processes for diagnostic results through natural language generation (14). This enables clinicians to better grasp the basis of the model’s decision-making process. Additionally, by utilizing visualization techniques and attention mechanism analysis, researchers can probe into the specific regions and features the model prioritizes when processing medical images, thus gaining a deeper understanding of the model’s performance.
Practical applications and potential scenarios of LLMs
A series of LLMs in biomedical science have been developed based on Google’s BERT model and OpenAI’s GPT model. These can serve as foundational resources for future applications in Medical Image Analysis. Initial LLMs based on BERT (ClinicalBERT, BioBERT) were smaller in scope, focusing primarily on biomedical text-mining tasks. However, contemporary models built on GPT (BioMedLM, GeneGPT) display more versatility in their functionality (33-35), as illustrated in Table 5.
Table 5
Current LLMs | ClinicalBERT | BioBERT | ChatGPT | BioMedLM | GeneGPT |
---|---|---|---|---|---|
Use of clinical notes | √ | √ | √ | √ | √ |
Biomedical text mining | N/A | √ | √ | √ | √ |
Biomedical Q&A | N/A | N/A | √ | √ | √ |
Multimodal integration | × | × | √ | N/A | √ |
Interactivity | × | × | √ | √ | √ |
Domain-specific | √ | √ | × | √ | √ |
√, have the function; ×, do not have the function. LLMs, large language models; GPT, Generative Pre-trained Transformer; BERT, Bidirectional Encoder Representations from Transformers; Q&A, Questions and Answers; N/A, not applicable.
The size of the LLM plays a crucial role in determining the final processing performance of the model. Compared to BERT-based models from two years ago, GPT-based models have shown significant performance improvements. Specifically, ChatGPT, built on the GPT-4 model, can achieve high-quality processing and question-answering of relevant medical texts, even without specialized training in the biomedical field. This suggests that LLMs are poised to drive significant changes in the field of medical imaging in the future.
Although some clinical practitioners have given objective and positive feedback on the potential applications of LLMs (36,37), a comprehensive evaluation of the model’s performance and accuracy across all aspects remains to be carried out. As such, it remains crucial to rigorously evaluate the performance and effectiveness of LLMs in medical image processing.
Nevertheless, new trials in the field of medical image analysis have been emerging recently at a surprisingly fast rate. MedSAM, as mentioned above, is a successful attempt (28). The authors present the design and implementation of MedSAM, as well as its performance in 3D segmentation tasks and 2D segmentation tasks. As an extension of SAM, MedSAM demonstrates the power of fine-tuning adaptation on LLMs. Since the experimental results show its superiority over the default SAM model,
they further perform a decomposition analysis of SAM and evaluate its potential utility in medical image segmentation tasks. MedSAM shows us how to bridge the gap between general LLMs and professional fields like medical image processing, aimed at creating a universal tool for segmenting various medical objects.
Another method, UniverSeg, addresses new medical image segmentation tasks without requiring additional training (38). UniverSeg employs a novel CrossBlock mechanism to generate accurate segmentation maps. To achieve task generalization, the authors gathered and standardized 53 open-access medical segmentation datasets with over 22,000 scans. They used this dataset to train the UniverSeg model for generalization across various anatomies and imaging modalities. The primary advantage of the UniverSeg method lies in its ability to handle new medical image segmentation tasks by learning task-agnostic models and applying them to medical image segmentation, thereby eliminating the need for additional training. When provided with a query image and a set of image-label pairs defining the new segmentation task, UniverSeg uses the novel CrossBlock mechanism to generate accurate segmentation maps, eliminating additional training. The researchers demonstrated that UniverSeg significantly outperforms various related methods on unseen tasks. Inspired by LLMs’ approach, other medical image segmentation models like STU-Net also emerged (39). This model has parameter sizes ranging from 14 million to 1.4 billion. Upon training the scalable and transferable STU-Net model on large-scale datasets, the authors found that an increase in model size resulted in substantial performance improvements. They assessed the transferability of their model across 14 downstream datasets, observing a favorable performance in direct inference and fine-tuning scenarios. All these emerging models aim to enhance Medical Artificial General Intelligence in medical image processing.
The MedSAM and UniverSeg mentioned above and similar LLMs have promising applications on the horizon. Take MedSAM for example, here are some potential clinical application prospects. Firstly, in the domain of Medical Image Segmentation, it assumes a pivotal role by precisely segmenting organs within CT or MRI images. This proficiency not only aids doctors in gaining a more comprehensive understanding of patients’ conditions but also assists in the formulation of treatment strategies and surgical preparations. Moreover, MedSAM facilitates Natural Language Interaction, fostering seamless dialogues between healthcare professionals and patients. It promptly offers real-time explanations and addresses inquiries, thereby enhancing communication and rendering medical information more comprehensible. Beyond this, in the realm of Personalized Healthcare, LLMs come to the forefront. Leveraging patients’ medical histories and clinical data, they provide tailored medical counsel and treatment regimens, thereby amplifying treatment effectiveness and curbing unnecessary interventions. Furthermore, as an asset for Clinical Decision Support, MedSAM empowers healthcare providers with access to the latest medical research and guidelines. This empowers doctors to make well-informed choices regarding treatment strategies (28). Lastly, in terms of Medical Knowledge Management, LLMs serve as valuable tools for healthcare professionals, facilitating the organization and updating of medical knowledge. This ensures that healthcare practitioners remain well-versed in the latest developments and practices in the medical field. These applications have the potential to transform healthcare by improving diagnosis, treatment, and patient-doctor interactions while keeping medical professionals up-to-date with the latest research and guidelines.
Discussion
As the application of LLMs in medical image processing continues to expand, the present challenges are anticipated to be significantly mitigated in the foreseeable future. Furthermore, it is expected that the principles of digital medicine will be further implemented with the support of LLMs. By combining patients’ information like genetic data, medical history, and chief complaints, the models can assist doctors in providing more accurate and personalized diagnoses for each patient. However, LLMs still have many challenges to overcome to achieve personalized medicine.
High-quality annotated datasets represent a significant investment and are a crucial resource in machine learning. However, many factors in practical applications may affect data quality and annotation accuracy. Beyond the inherent differences in equipment between hospitals, managing large-scale hospital imaging data presents numerous challenges. The adoption rate of Picture Archiving and Communication System (PACS) is currently only 50–60% (40), suggesting that effective sharing of hospital data across different institutions remains a challenge. Meanwhile, the cost of storing and operating hospital data is exceptionally high. Without a robust digital medical infrastructure, the potential of advanced tools such as AI cannot be fully realized.
Consequently, future developments should focus on providing more enterprise-level medical imaging services to replace traditional PACS, thus bridging the gap between disparate medical imaging modalities. Ideally, clinicians should be able to access images and reports from anywhere, not confined to specific workstations. With advancements in digital medical infrastructure, acquiring high-quality datasets and performing highly accurate analyses can be significantly facilitated.
Furthermore, there are areas in which current AI technology could see enhancement.
(I) Interpretability of LLMs
Given the direct impact of clinical applications on patient safety, the interpretability of AI models becomes a critical requirement. While LLMs can assist users in interpreting diagnostic results through language interaction, enhancing model interpretability remains challenging. These models’ “black box” nature needs further elucidation, particularly in medicine (17). New techniques should be explored to improve the interpretability of LLMs in medical image processing. This includes using attention and gradient visualization to reveal how the model focuses on essential features. Additionally, adversarial testing and natural language explanations can help detect model weaknesses and provide more precise, confidence-based results.
(II) The quality of hardware infrastructure
The hardware infrastructure supporting digital medical facilities is still under development. Factors such as noise, blur, and artifacts can affect the quality of images in medical image processing. Therefore, future research must improve the model’s anti-interference ability and generalization performance to ensure stability and reliability in actual applications (41). Collaboration among engineers is crucial for developing imaging systems and software that yield high-quality and artifact-free medical images. Research efforts should create robust machine learning algorithms to enhance the model’s anti-interference ability and generalization performance. This requires training models on diverse datasets encompassing various image qualities and addressing noisy or imperfect input data issues. Techniques like data augmentation and transfer learning can also help improve model stability and reliability in real-world applications (42).
(III) The real-time performance of LLMs
Suppose LLMs will play a more significant role in real-time diagnosis and treatment in the future. In that case, this places higher demands on the more effective integration and utilization of multimodal data, the model’s computational efficiency, and real-time performance (17). It is crucial to develop new data fusion technologies, establish multimodal data standardization methods, utilize advanced deep learning algorithms for feature extraction and representation learning, and devise optimization strategies to enhance the model’s operating speed. To provide healthcare professionals with a complete understanding of patient data, it is imperative to seamlessly merge information from various sources, such as medical images, patient records, and real-time monitoring data. This is where Multimodal Data Standardization comes into play. By defining data formats, metadata, and protocols, healthcare institutions, data scientists, and regulatory bodies can ensure compatibility and consistency across different sources through collaboration. Advanced deep learning algorithms require feature extraction and representation learning to extract significant information from complex multimodal data. Research and development should focus on algorithms that can effectively handle various data types, including images, text, and numerical data (42). Optimization strategies are necessary to enhance the model’s operating speed, including optimizing the model architecture, implementing efficient algorithms for inference, and utilizing hardware acceleration techniques like Graphics Processing Units (GPUs) (14). For real-time capabilities, continuous performance monitoring and fine-tuning are critical. By implementing these strategies, healthcare professionals can gain comprehensive insights into patient data, enabling them to make informed decisions that improve patient outcomes.
(IV) Ethical considerations
Lastly, applying LLMs involves addressing a range of ethical considerations. Concerns like data privacy protection, algorithmic bias, and model accountability necessitate significant attention. Medical imaging data comprise sensitive personal health information; if mishandled or misused, it could compromise patient privacy and rights. Concurrently, potential bias in training data sources for AI algorithms can propagate bias into the resulting models. In medical imaging, some algorithms may be more responsive to specific populations’ data, potentially leading to accidental neglect or prejudice against the health conditions of other groups (43,44).
Nevertheless, determining responsibility for AI-assisted medical care is one of the most pressing challenges. Although AI algorithms may achieve a high level of medical imaging diagnosis, they still cannot avoid mistakes. It becomes tricky when medical negligence happens. Therefore, before the large-scale application of AI in medical imaging, it is vital to establish transparent systems for model responsibility and risk management to safeguard patients’ rights (43,44). To address the ethical concerns associated with applying LLMs in medical imaging, robust data privacy measures should be implemented to protect sensitive medical imaging data. This involves encryption, strict access controls, and compliance with privacy regulations to protect patient privacy and rights. Careful data selection and preprocessing during model training are crucial to mitigate algorithmic bias. Monitoring and auditing model outputs are essential to identify and rectify biases, ensuring fair and unbiased medical decisions. Ensuring model accountability involves making the model’s decision-making processes transparent and interpretable. When a model provides a diagnosis or recommendation, it should explain its decision, promoting trust and accountability. Diverse and representative datasets should be employed to prevent potential biases in training data. Models should be rigorously evaluated across various demographic groups to identify and rectify potential biases, preventing neglect or discrimination against specific health conditions.
In conclusion, promoting LLMs in medical imaging requires developers, regulatory agencies, and medical institutions to collaborate, ensuring that technology applications adhere to legal and ethical principles.
We acknowledge that this study has certain limitations. Firstly, our inclusion criteria were confined to articles and reviews authored in English and indexed mainly within the Web of Science, and PubMed databases. While this approach may have unintentionally omitted some valuable studies, it is noteworthy that the Web of Science and PubMed databases stand as the most employed databases. As such, we anticipate any potential impact on overall trends to be relatively minimal. Secondly, since the development of LLMs is quite rapid, it is important to recognize that the delay in citation volume might have resulted in most recent high-quality studies not receiving the immediate recognition they merit. Acknowledging this, we anticipate the need to update our findings accordingly in subsequent research endeavors. Thirdly, to better showcase the cutting-edge advancements of LLMs in the field of Medical Image Processing, we have referenced some of the latest research from the preprint website arXiv database. While these applications in arXiv effectively highlight the most recent explorations, it is important to note that the reliability of preprint studies still requires further validation in subsequent research. Nevertheless, this study stands as a significant contribution with substantial benefits for pertinent researchers. It offers valuable and cutting-edge insights into LLMs’ potential advancements in Medical Imaging Processing. Furthermore, it aids in pinpointing areas where additional research remains imperative.
Conclusions
In this paper, we aim to delve into the potential applications of LLMs within medical image processing. Starting with the foundational principles of LLMs, we highlight their merits in this field, such as the capacity to integrate multimodal data, the efficacy of transfer learning, and robust interactivity. Using practical examples, we illustrate the specific impacts of LLM applications in this domain. Concurrently, we delve into their future potential and the challenges ahead. These prospects and challenges provide valuable references for researchers and developers to promote technological innovation and the application of LLMs in medical image processing. Through an extensive literature review, we found that numerous teams are already employing LLMs, such as ChatGPT, in the biomedical field, with some garnering recognition within the medical community.
Nonetheless, applying LLMs in medical image processing still has significant room for growth and development. Future research should aim to develop more efficient, accurate, and reliable medical image processing techniques by continuously optimizing model performance, improving data quality, enhancing model interpretability, and addressing ethical challenges. Simultaneously, we aspire to incorporate more medical literature, experimental data, and professional treatments into future medical LLMs. This would allow professionals to participate in the model training process and provide feedback on the results. This will generate considerable medical value, which makes AI diagnosis and treatment recommendations based on patients’ multimodal information. We are confident that LLMs, such as ChatGPT, will evolve into powerful assistants in the medical field in the foreseeable future, potentially driving revolutionary changes in medical imaging. Hence, we advocate for intensified interdisciplinary collaboration and research endeavors that unite experts from NLP, computer vision, and medical domains. This collaborative effort is imperative to propel the development and assessment of LLMs in medical image processing. Hopefully, this article will serve as a valuable reference and source of inspiration for researchers and practitioners interested in this emerging and promising field.
Acknowledgments
We would like to express our heartfelt gratitude to Zhou Fang for her invaluable assistance in language editing and refinement of this manuscript. Their meticulous work significantly contributed to the clarity and coherence of the text.
Funding: This article was partially sponsored by
Footnote
Reporting Checklist: The authors have completed the Narrative Review reporting checklist. Available at https://qims.amegroups.com/article/view/10.21037/qims-23-892/rc
Conflicts of Interest: All authors have completed the ICMJE uniform disclosure form (available at https://qims.amegroups.com/article/view/10.21037/qims-23-892/coif). The authors have no conflicts of interest to declare.
Ethical Statement: The authors are accountable for all aspects of the work in ensuring that questions related to the accuracy or integrity of any part of the work are appropriately investigated and resolved.
Open Access Statement: This is an Open Access article distributed in accordance with the Creative Commons Attribution-NonCommercial-NoDerivs 4.0 International License (CC BY-NC-ND 4.0), which permits the non-commercial replication and distribution of the article with the strict proviso that no changes or edits are made and the original work is properly cited (including links to both the formal publication through the relevant DOI and the license). See: https://creativecommons.org/licenses/by-nc-nd/4.0/.
References
- Beaton L, Bandula S, Gaze MN, Sharma RA. How rapid advances in imaging are defining the future of precision radiation oncology. Br J Cancer 2019;120:779-90. [Crossref] [PubMed]
- Global Medical Image Analysis Software Market Report, 2030 [Internet]. [cited 2023 Oct 7]. Available online: https://www.grandviewresearch.com/industry-analysis/medical-image-analysis-software-market
- Polikar R, Tilley C, Hillis B, Clark CM. Multimodal EEG, MRI and PET data fusion for Alzheimer's disease diagnosis. Annu Int Conf IEEE Eng Med Biol Soc 2010;2010:6058-61. [Crossref] [PubMed]
- Varoquaux G, Cheplygina V. Machine learning for medical imaging: methodological failures and recommendations for the future. NPJ Digit Med 2022;5:48. [Crossref] [PubMed]
- Rajpurkar P, Lungren MP. The Current and Future State of AI Interpretation of Medical Images. N Engl J Med 2023;388:1981-90. [Crossref] [PubMed]
- Liu Y, Han T, Ma S, Zhang J, Yang Y, Tian J, He H, Li A, He M, Liu Z, Wu Z, Zhao L, Zhu D, Li X, Qiang N, Shen D, Liu T, Ge B. Summary of ChatGPT/GPT-4 Research and Perspective Towards the Future of Large Language Models [Internet]. arXiv; 2023 [cited 2023 May 16]. Available online: http://arxiv.org/abs/2304.01852
- Chen J, Lu Y, Yu Q, Luo X, Adeli E, Wang Y, Lu L, Yuille AL, Zhou Y. TransUNet: Transformers Make Strong Encoders for Medical Image Segmentation [Internet]. arXiv; 2021 [cited 2023 May 18]. Available online: http://arxiv.org/abs/2102.04306
- Lecun Y, Bottou L, Bengio Y, Haffner P. Gradient-based learning applied to document recognition. Proceedings of the IEEE 1998;86:2278-324.
- Ronneberger O, Fischer P, Brox T. U-Net: Convolutional Networks for Biomedical Image Segmentation [Internet]. arXiv; 2015 [cited 2023 Apr 20]. Available online: http://arxiv.org/abs/1505.04597
- Zhang Y, Brady M, Smith S. Segmentation of brain MR images through a hidden Markov random field model and the expectation-maximization algorithm. IEEE Trans Med Imaging 2001;20:45-57. [Crossref] [PubMed]
- Hatamizadeh A, Yin H, Heinrich G, Kautz J, Molchanov P. Global Context Vision Transformers [Internet]. arXiv; 2023 [cited 2023 May 17]. Available online: http://arxiv.org/abs/2206.09959
- Wu H, Xiao B, Codella N, Liu M, Dai X, Yuan L, Zhang L. CvT: Introducing Convolutions to Vision Transformers [Internet]. arXiv; 2021 [cited 2023 May 17]. Available online: http://arxiv.org/abs/2103.15808
- Vaswani A, Shazeer N, Parmar N, Uszkoreit J, Jones L, Gomez AN, Kaiser L, Polosukhin I. Attention Is All You Need [Internet]. arXiv; 2017 [cited 2023 Apr 20]. Available online: http://arxiv.org/abs/1706.03762
- Improving language understanding with unsupervised learning [Internet]. [cited 2023 Oct 7]. Available online: https://openai.com/research/language-unsupervised
- Gupta N. A Pre-Trained Vs Fine-Tuning Methodology in Transfer Learning. J Phys: Conf Ser 2021;1947:012028.
- Wang S, Li C, Wang R, Liu Z, Wang M, Tan H, Wu Y, Liu X, Sun H, Yang R, Liu X, Chen J, Zhou H, Ben Ayed I, Zheng H. Annotation-efficient deep learning for automatic medical image segmentation. Nat Commun 2021;12:5915. [Crossref] [PubMed]
- Shamshad F, Khan S, Zamir SW, Khan MH, Hayat M, Khan FS, Fu H. Transformers in Medical Imaging: A Survey [Internet]. arXiv; 2022 [cited 2023 May 16]. Available online: http://arxiv.org/abs/2201.09873
- Dosovitskiy A, Beyer L, Kolesnikov A, Weissenborn D, Zhai X, Unterthiner T, Dehghani M, Minderer M, Heigold G, Gelly S, Uszkoreit J, Houlsby N. An Image is Worth 16x16 Words: Transformers for Image Recognition at Scale [Internet]. arXiv; 2021 [cited 2023 May 18]. Available online: http://arxiv.org/abs/2010.11929
- Touvron H, Cord M, Douze M, Massa F, Sablayrolles A, Jégou H. Training data-efficient image transformers & distillation through attention [Internet]. arXiv; 2021 [cited 2023 May 18]. Available online: http://arxiv.org/abs/2012.12877
- Basu S, Gupta M, Rana P, Gupta P, Arora C. RadFormer: Transformers with Global-Local Attention for Interpretable and Accurate Gallbladder Cancer Detection [Internet]. arXiv; 2022 [cited 2023 May 18]. Available online: http://arxiv.org/abs/2211.04793
- Tajbakhsh N, Shin JY, Gurudu SR, Hurst RT, Kendall CB, Gotway MB, Liang Jianming. Convolutional Neural Networks for Medical Image Analysis: Full Training or Fine Tuning? IEEE Trans Med Imaging 2016;35:1299-312. [Crossref] [PubMed]
- Nori H, King N, McKinney SM, Carignan D, Horvitz E. Capabilities of GPT-4 on Medical Challenge Problems [Internet]. arXiv; 2023 [cited 2023 May 17]. Available online: http://arxiv.org/abs/2303.13375
- Boehm KM, Khosravi P, Vanguri R, Gao J, Shah SP. Harnessing multimodal data integration to advance precision oncology. Nat Rev Cancer 2022;22:114-26. [Crossref] [PubMed]
- Osorio D. Interpretable multi-modal data integration. Nat Comput Sci 2022;2:8-9.
- Wang S, Zhao Z, Ouyang X, Wang Q, Shen D. ChatCAD: Interactive Computer-Aided Diagnosis on Medical Image using Large Language Models [Internet]. arXiv; 2023 [cited 2023 Apr 12]. Available online: http://arxiv.org/abs/2302.07257
- Gao S, Kotevska O, Sorokine A, Christian JB. A pre-training and self-training approach for biomedical named entity recognition. PLoS One 2021;16:e0246310. [Crossref] [PubMed]
- Kirillov A, Mintun E, Ravi N, Mao H, Rolland C, Gustafson L, Xiao T, Whitehead S, Berg AC, Lo WY, Dollár P, Girshick R. Segment Anything. [Internet]. arXiv; 2023 [cited 2023 May 17]. Available online: https://arxiv.org/abs/2304.02643
- Ma J, He Y, Li F, Han L, You C, Wang B. Segment Anything in Medical Images [Internet]. arXiv; 2023 [cited 2023 May 17]. Available online: http://arxiv.org/abs/2304.12306
- Liu F, Vulić I, Korhonen A, Collier N. Learning Domain-Specialised Representations for Cross-Lingual Biomedical Entity Linking [Internet]. arXiv; 2021 [cited 2023 Apr 23]. Available online: http://arxiv.org/abs/2105.14398
- Yan K, Wang X, Lu L, Summers RM. DeepLesion: automated mining of large-scale lesion annotations and universal lesion detection with deep learning. J Med Imaging (Bellingham) 2018;5:036501. [Crossref] [PubMed]
- Chen S, Ma K, Zheng Y. Med3D: Transfer Learning for 3D Medical Image Analysis [Internet]. arXiv; 2019 [cited 2023 May 17]. Available online: http://arxiv.org/abs/1904.00625
- Yang J, He Y, Kuang K, Lin Z, Pfister H, Ni B. Asymmetric 3D Context Fusion for Universal Lesion Detection. 2021:571-80. [cited 2023 May 17]. Available online: http://arxiv.org/abs/2109.08684
- Huang K, Altosaar J, Ranganath R. ClinicalBERT: Modeling Clinical Notes and Predicting Hospital Readmission [Internet]. arXiv; 2020 [cited 2023 Apr 21]. Available online: http://arxiv.org/abs/1904.05342
- Lee J, Yoon W, Kim S, Kim D, Kim S, So CH, Kang J. BioBERT: a pre-trained biomedical language representation model for biomedical text mining. Bioinformatics 2020;36:1234-40. [Crossref] [PubMed]
- Jin Q, Yang Y, Chen Q, Lu Z. GeneGPT: Augmenting Large Language Models with Domain Tools for Improved Access to Biomedical Information [Internet]. arXiv; 2023 [cited 2023 May 18]. Available online: http://arxiv.org/abs/2304.09667
- Lee P, Bubeck S, Petro J. Benefits, Limits, and Risks of GPT-4 as an AI Chatbot for Medicine. N Engl J Med 2023;388:1233-9. [Crossref] [PubMed]
- Haug CJ, Drazen JM. Artificial Intelligence and Machine Learning in Clinical Medicine, 2023. N Engl J Med 2023;388:1201-8. [Crossref] [PubMed]
- Butoi VI, Ortiz JJG, Ma T, Sabuncu MR, Guttag J, Dalca AV. UniverSeg: Universal Medical Image Segmentation [Internet]. arXiv; 2023 [cited 2023 May 5]. Available online: http://arxiv.org/abs/2304.06131
- Huang Z, Wang H, Deng Z, Ye J, Su Y, Sun H, Junjun He J, Gu Y, Gu L, Zhang S, Qiao Y. STU-Net: Scalable and Transferable Medical Image Segmentation Models Empowered by Large-Scale Supervised Pre-training [Internet]. arXiv; 2023 [cited 2023 May 5]. Available online: http://arxiv.org/abs/2304.06716
- Tzeng WS, Kuo KM, Lin HW, Chen TY. A socio-technical assessment of the success of Picture Archiving and Communication Systems: the radiology technologist's perspective. BMC Med Inform Decis Mak 2013;13:109. [Crossref] [PubMed]
-
Johnson D Goodman R Patrinely J Stone C Zimmerman E Donald R Assessing the Accuracy and Reliability of AI-Generated Medical Responses: An Evaluation of the Chat-GPT Model. Res Sq 2023 .[Epub ahead of print] . doi: . - Fernando T, Gammulle H, Denman S, Sridharan S, Fookes C. Deep Learning for Medical Anomaly Detection -- A Survey [Internet]. arXiv; 2021 [cited 2023 Oct 7]. Available online: http://arxiv.org/abs/2012.02364
- Brown TB, Mann B, Ryder N, Subbiah M, Kaplan J, Dhariwal P, et al. Language Models are Few-Shot Learners [Internet]. arXiv; 2020 [cited 2023 Oct 7]. Available online: http://arxiv.org/abs/2005.14165
- Brundage M, Avin S, Clark J, Toner H, Eckersley P, Garfinkel B, et al. The Malicious Use of Artificial Intelligence: Forecasting, Prevention, and Mitigation [Internet]. arXiv; 2018 [cited 2023 Oct 7]. Available online: http://arxiv.org/abs/1802.07228