Histogram analysis of quantitative susceptibility mapping and apparent diffusion coefficient for identifying isocitrate dehydrogenase genotypes and tumor subtypes of adult-type diffuse gliomas
Introduction
Diffuse gliomas are the most common malignant brain tumors in adults (1,2). Reliable preoperative classification is crucial for the proper treatment and prognosis evaluation of patients with glioma. Since 2016, the isocitrate dehydrogenase (IDH) genotype, which is strongly linked to the internal heterogeneity and biological behavior of glioma, has been considered one of the most important molecular markers in the classification of gliomas (3). Compared with those with IDH-wildtype gliomas, patients with IDH-mutant gliomas usually have a much better prognosis (4). Recently, the 2021 World Health Organization Classification of Tumors of the Central Nervous System (CNS WHO classification 2021) further advanced the role of molecular diagnostics in the classification of CNS tumors (5). Hence, a non-invasive assessment of IDH genotypes and tumor subtypes is essential before choosing a proper treatment strategy.
Magnetic resonance imaging (MRI) is a non-invasive technique widely used for evaluating glioma (6,7). Diffusion, perfusion, and metabolic imaging have been extensively utilized in glioma grading and genotyping research. However, perfusion-weighted imaging requires the injection of a contrast agent, whereas magnetic resonance spectroscopy (MRS) has limitations such as the need for individualized positioning, complex operation, weak acquisition signal, susceptibility to interference, and time-consuming nature (8-10). Susceptibility-weighted imaging (SWI) is a non-quantitative technique that employs gradient (recalled) echo (GRE) phase images to enhance the smallest susceptibility variations on the corresponding magnitude images (11). SWI can detect intratumoral hemorrhage, neovascularity, and calcification in glioma (12). However, SWI has limitations in accurately quantifying these features due to non-quantitative and blooming artifacts. Recently, a novel post-processing technique was introduced that produces quantitative maps of tissue magnetic susceptibility using GRE phase data (13,14). This technique, called quantitative susceptibility mapping (QSM), has been offered accurate quantitative measurements of magnetic susceptibility distributions in the brain (15,16). QSM also has a higher contrast-to-noise ratio (CNR) than SWI for lesion depiction and can eliminate the negative effects observed at SWI in the process of reconstruction (17). QSM has been used to estimate the levels of various substances in the brain, such as iron, hemosiderin, deoxyhemoglobin, and calcification (17-19). Therefore, QSM can be used to identify microhemorrhages, microvascularity, and calcification in glioma, which may facilitate the diagnosis and classification of gliomas. A recently published research paper found that tumor parenchyma magnetic susceptibility had limited value in grading gliomas and identifying IDH mutation status, whereas the relatively low magnetic susceptibility of the tumor parenchyma helped to identify oligodendrogliomas in IDH mutated gliomas (20). However, as the sample size of this study was small, and it is still controversial whether QSM can be applied to identify IDH genotypes, data and research from other centers are needed to support the findings.
Diffusion-weighted imaging (DWI) and apparent diffusion coefficient (ADC) are applied routinely for gliomas, as it provides the valuable information of cellularity and extracellular spaces within tumors (21). The ADC is negatively correlated with cell density and certain proliferation indices (22). Furthermore, the ADC is significantly different between low-grade and high-grade gliomas (23) and IDH-mutant and IDH-wildtype gliomas (24). A recent study found that PET using 18F-fluoromisonidazole (18F-FMISO) and ADC might provide a valuable tool for differentiating IDH mutation status of 2021 WHO classification grade 3 and 4 adult-type diffuse glioma (25). However, the association of ADC with the tumor subtypes of glioma based on CNS WHO classification 2021 has not been thoroughly investigated.
As two quantitative imaging techniques, QSM can reflect microhemorrhages and microvascularity of the tumor, whereas ADC can quantify cellularity and extracellular spaces within a tumor. Therefore, we hypothesized that combining QSM and ADC would better evaluate the histopathological microstructures of gliomas. We aimed to determine the utility of these two techniques in non-invasive assessment of the IDH genotypes and tumor subtypes of adult-type diffuse gliomas by histogram analysis. We present this article in accordance with the STARD reporting checklist (available at https://qims.amegroups.com/article/view/10.21037/qims-23-832/rc).
Methods
Patients and clinical information
Patients with suspected gliomas were prospectively and randomly enrolled in this study between March 2019 and January 2022. The study was conducted in accordance with the Declaration of Helsinki (as revised in 2013). The study was approved by the ethics committee board of Fujian Medical University Union Hospital, and informed consent was provided by all participants.
The following exclusion criteria were applied: (I) incomplete imaging data or poor image quality, namely, obvious artifacts or head motion; (II) without surgery or biopsy; (III) with histologically confirmed non-glioma lesions; (IV) failure of genetic detection due to insufficient tissue.
The screening process for included glioma patients is shown in Figure 1. The clinical information including age, gender, and recurrence rate were recorded.
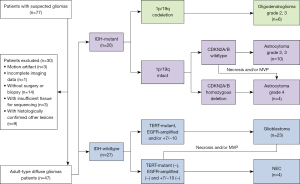
MRI data acquisition
All participants underwent structural MRI, QSM, and DWI imaging on a 3T MR scanner (MAGNETOM Prisma; Siemens Healthineers, Erlangen, Germany) with a 64-channel head coil. The structural MRI protocols included sagittal T1-weighted magnetization-prepared rapid gradient echo sequence (T1-MPRAGE), axial T2-weighted (T2W) fast spin-echo (FSE) images, axial fluid-attenuated inversion recovery (FLAIR) T2W images, and contrast-enhanced axial/sagittal/coronal T1W (CE-T1W) images.
QSM maps were created based on a 3-dimensional (3D) flow-compensated multi-echo GRE sequence in the axial plane [repetition time (TR) =35 ms; first echo time (TE) =6.67 ms, uniform echo spacing =6.24 ms, last TE =25.39 ms; number of echoes =4; fractional anisotropy (FA) =15°; field of view (FOV) =280×320 mm2; voxel size =0.72×0.72×2 mm3].
A single-shot echo planer imaging sequence was used for the DWI imaging with the following parameters: TR/TE =3,800 ms/74 ms; slice thickness =5 mm, gap =1 mm; FOV =23 cm × 23 cm; acquisition matrix =128×128, reconstruction matrix =256×256; and pixel bandwidth =2,055 Hz/pixel. The sequence was performed with two different b values (0 and 1,000 s/mm2).
Data processing and analysis
Calculation of QSM and ADC maps
QSM maps were produced using the susceptibility tensor imaging (STI) Suite V3.0 software package (https://chunleiliulab.github.io/software.html) as follows: (I) Phase unwrapping: the raw phase was unwrapped using Laplacian-based phase unwrapping, and the normalized phase was calculated (26,27); (II) V_SHARP: the normalized background phase was removed using spherical-mean-value filtering (V_SHARP) (28); and (III) STAR-QSM: tissue susceptibility was calculated using the STAR-QSM method (streaking artifact reduction for QSM) (29,30). Wu et al. (31) also used this QSM processing method. ADC maps were calculated by fitting the b0 images and DWI images into the mono-exponential equation: Sb/S0 = exp (–b × ADC), where Sb is the diffusion-weighted signal intensity for the b value, and S0 is the signal intensity obtained with the b0 value.
T1-MPRAGE, T2W, FLAIR, and CE-T1W images were co-registered to the QSM and ADC images by rigid-body registering to the first echo magnitude image from the GRE pulse sequence and b0 images respectively, using the SPM12 software (www.fil.ion.ucl.ac.uk/spm/software/spm12).
Region of interest (ROI) placement and measurement
All MR images were independently reviewed by two radiologists (R.J. and Y.S., with 15 and 12 years of experience, respectively) who were unaware of the clinical information and histopathological results. They then performed semi-automatic delineation of ROIs over the tumor parenchyma slice by slice using ImageJ (version 1.49o, National Institutes of Health [NIH], https://imagej.nih.gov/ij/). Then, multiple 2-dimensional (2D) plane ROIs were converted into 3D ROIs. The ROIs over the solid enhancing tumor were delineated on transverse CE-T1W, and the ROIs over the non-enhancing tumor were delineated on the transverse FLAIR or T2W. Cystic components, necrosis, hemorrhage, and calcification in the solid region of the tumor were avoided. ROIs delineation over the solid region of the tumor and representative cases of different tumor subtypes are shown in Figure 2.
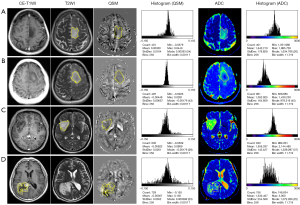
Subsequently, the ROIs were spatially transferred to the QSM and ADC maps to obtain the quantitative histogram characteristics using the pyradiomics software (version 3.0.1, https://github.com/AIM-Harvard/pyradiomics). The following histogram characteristics were extracted: 10th percentile (P10), 90th percentile (P90), interquartile range (IQR), kurtosis, maximum, mean absolute deviation (MAD), mean, median, minimum, root mean squared (RMS), skewness, and variance.
Glioma classification based on pathological and genetic detection
Pathological tests were performed to determine the tumor type. Immunohistochemical staining was performed using the EnVision (Dako, Hamburg, Germany) method. The Ki-67 labeling index (Ki-67 LI) was measured, which was defined as the percentage of nuclear staining-positive cells with any intensity in the high-density staining area in the total cells.
Multiplexed polymerase chain reaction (PCR) followed by next-generation sequencing (NGS) was used to obtain necessary molecular features. Single nucleotide variants (SNV) detection was used to detect the gene mutation of IDH1 codon 132, IDH2 codon 172, telomerase reverse transcriptase (TERT) codon C228T, and TERT codon C250T. Copy number variation (CNV) detection was used to detect the heterozygous deletion on chromosome 1p/19q, loss of chromosome 10, and gain of chromosome 7. Real-time quantitative PCR (qRT-PCR) was used to detect epidermal growth factor receptor variant III (EGFRvIII) amplification and cyclin-dependent kinase inhibitor 2A/B (CDKN2A/B). A mutation in any one of IDH1 codon 132 and IDH2 codon 172 was diagnosed as IDH mutation; otherwise, it was diagnosed as IDH wildtype.
Patients were first grouped according to IDH genotypes (i.e., IDH-wildtype and IDH-mutant). Subsequently, according to the current CNS WHO classification 2021 criteria (5), patients were further divided into astrocytoma, IDH-mutant (including grade 2, 3, and 4); oligodendroglioma, IDH-mutant and 1p/19q-codeleted (including grade 2 and 3), and glioblastoma (GBM), IDH-wildtype.
Statistical analysis
Statistical analysis was performed using the software SPSS 26.0 (IBM Corp., Armonk, NY, USA). The inter-observer variability of measurements in glioma patients was evaluated using the intra-class correlation coefficient. The Kruskal-Wallis test was used to compare the difference of the age and Ki-67 LI, and Fisher’s exact test was used to compare the difference of gender and recurrence rate among groups. The Mann-Whitney U test was used to compare the differences in the histogram features between different IDH genotypes and tumor subtypes, and corrected for multiple comparisons using the Benjamini-Hochberg method. Binary logistic regression analysis was used to combine significant histogram features of QSM and ADC to create regression equations and calculate the corresponding prediction probability for identifying the IDH genotypes and tumor subtypes. Receiver operating characteristic (ROC) curve and the corresponding area under the curve (AUC) were constructed to assess the corresponding diagnostic performance of each histogram feature and the prediction probability. Delong test was further used to compare the differences of AUCs. The Spearman correlation analysis was used to evaluate the correlation between Ki-67 LI and each histogram feature. A default alpha level of 0.05 was used for all tests, and all the tests were 2-tailed.
Results
Demographic, clinical, and pathological characteristics of participants
The demographic, clinical, and pathological characteristics of the included glioma patients are summarized in Table 1. A total of 47 patients with pathologically confirmed adult-type diffuse gliomas were included in this study. Among them, 27 were with IDH-wildtype glioma, including 23 with GBM and 4 classified as not elsewhere classified (NEC) due to the insufficient molecular status analysis, and the other 20 were with IDH-mutant glioma, including 14 with astrocytoma and 6 with oligodendroglioma. There were 37 primary and 10 recurrent gliomas. The gender and recurrence rate showed no significance across three groups of gliomas. A significant difference was found in age and Ki-67 LI among three groups of gliomas (P=0.002 and 0.001), and both age and Ki-67 LI were significantly higher for glioma with IDH-wildtype.
Table 1
Characteristic | IDH-wildtype (n=27) | IDH-mutant | P value | |
---|---|---|---|---|
1p/19q-non-codeleted (n=14) | 1p/19q-codeleted (n=6) | |||
Age (years) | 53 [46–62] | 37 [32–50] | 46 [34–47] | 0.002* |
Gender (male/female) | 15/12 | 10/4 | 2/4 | 0.282 |
Recurrent glioma | 5 | 4 | 1 | 0.738 |
Subtypes | ||||
Glioblastoma | 23 | NA | NA | NA |
Astrocytoma | ||||
Grade 2 | NA | 9 | NA | NA |
Grade 3 | NA | 1 | NA | NA |
Grade 4 | NA | 4 | NA | NA |
Oligodendroglioma | ||||
Grade 2 | NA | NA | 5 | NA |
Grade 3 | NA | NA | 1 | NA |
Ki-67 LI (%) | 40 [12.5–55] | 8 [5–30] | 5 [4–5] | 0.001* |
Values are presented as median [inter-quartile range]. *, represents a statistical difference (P<0.05). IDH, isocitrate dehydrogenase; 1p/19q-codeleted, synchronous deletion of the short arm of chromosome 1 and long arm of chromosome 19; Ki-67 LI, Ki-67 labeling index; NA, not applicable.
Repeatability of measurements between two radiologists
The average sizes of the ROIs over the tumor parenchyma were 40.54±30.84 cm3 on QSM and 40.44±30.92 cm3 on ADC for the neuroradiologist R.J. and 37.49±29.35 cm3 on QSM and 37.42±29.48 cm3 on ADC for the neuroradiologist Y.S.. The intraclass correlation coefficients of averages of QSM and ADC were between 0.830 and 0.984 and between 0.521 and 0.977, respectively. Due to R.J.’s seniority and extensive clinical experience, the measurement data from R.J. were used for subsequent statistical analysis.
Comparisons of QSM and ADC histogram features in identifying IDH genotypes and glioma subtypes
The results of the differences in QSM and ADC histogram features between different IDH genotypes are shown in Tables S1,S2, and the corresponding box and whisker plots are shown in Figure 3A,3B. The average susceptibility of QSM was lower (P=0.121), whereas the average ADC was significantly higher (P=0.020) in IDH-mutant gliomas than that in IDH-wildtype gliomas. For histogram features, the P10 of QSM was significantly higher (P=0.016), whereas the P90, IQR, maximum, MAD, RMS, and variance of QSM were significantly lower (P=0.007, 0.001, 0.037, 0.003, 0.005, and 0.006, respectively) in IDH-mutant gliomas than those in IDH-wildtype gliomas. The skewness of ADC was significantly lower (P=0.017), whereas the P10, median, and RMS of ADC were significantly higher (P=0.004, 0.022, and 0.026, respectively) in IDH-mutant gliomas than those in IDH-wildtype gliomas.
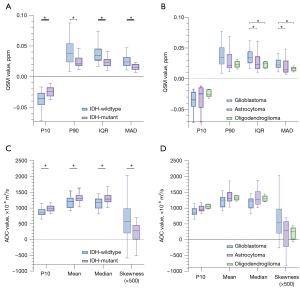
The differences in QSM and ADC histogram features among tumor subtypes are shown in Tables S1,S2, and the corresponding box and whisker plots are shown in Figure 3C,3D. The average susceptibility of QSM was higher, whereas the average ADC was lower in GBM than those in astrocytoma and oligodendroglioma (P>0.05). For histogram features, the IQR and MAD of QSM were found to be significantly lower in both astrocytoma (P=0.020 and 0.036, respectively) and oligodendroglioma (P=0.030 and 0.045, respectively) than those in GBM after the correction using the Benjamini-Hochberg method. The RMS of QSM was also significantly lower in oligodendroglioma than that in GBM (P=0.047). In contrast, no statistical difference was found for the other QSM histogram features and all the ADC histogram features in separating tumor subtypes.
Evaluation of the diagnostic performance of QSM and ADC histogram features
The best diagnostic performance of QSM and ADC histogram features in identifying IDH genotypes are shown in Table 2, and the ROC curves with the top three AUCs are shown in Figure 4A. The IQR of QSM demonstrated the highest diagnostic performance among QSM histogram features, with an AUC of 0.774, a sensitivity of 70.0%, and a specificity of 81.5%. Similarly, the P10 of ADC demonstrated a higher diagnostic value among ADC histogram features, with an AUC of 0.757, a sensitivity of 89.5%, and a specificity of 58.3%. The other QSM and ADC histogram features exhibited relatively lower diagnostic performance.
Table 2
Parameters | AUC | 95% CI | Threshold value | Sensitivity (%) | Specificity (%) |
---|---|---|---|---|---|
IDH-wildtype vs. IDH-mutant | |||||
QSM_IQR* | 0.774 | 0.635–0.913 | 2.42×10−2 | 70.0 | 81.5 |
ADC_P10* | 0.757 | 0.613–0.901 | 8.72×102 | 89.5 | 58.3 |
GBM vs. astrocytoma | |||||
QSM_IQR* | 0.745 | 0.566–0.924 | 2.35×10−2 | 71.4 | 82.6 |
ADC_P10 | 0.685 | 0.503–0.866 | 8.43×102 | 92.3 | 50.0 |
GBM vs. oligodendroglioma | |||||
QSM_IQR* | 0.848 | 0.706–0.989 | 2.88×10−2 | 73.9 | 100.0 |
ADC_P10 | 0.825 | 0.667–0.983 | 9.54×102 | 100.0 | 70.0 |
Astrocytoma vs. oligodendroglioma | |||||
QSM_IQR | 0.548 | 0.273–0.823 | 2.37×10−2 | 66.7 | 71.4 |
ADC_P10 | 0.744 | 0.522–0.965 | 9.69×102 | 100.0 | 61.5 |
*, represents a statistical difference (P<0.05). AUC, area under the curve; CI, confidence interval; IDH-wildtype; QSM, quantitative susceptibility mapping; IQR, interquartile range; ADC, apparent diffusion coefficient; P10, 10th percentile; GBM, glioblastoma.
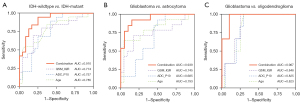
The diagnostic performance of QSM and ADC histogram features in separating tumor subtypes are shown in Table 2, and the ROC curves with the top three AUCs are shown in Figure 4B,4C. For GBM versus astrocytoma, the IQR of QSM demonstrated the highest diagnostic performance among QSM histogram features, with an AUC of 0.745, a sensitivity of 71.4%, and a specificity of 82.6%. Similarly, for GBM versus oligodendroglioma, the IQR of QSM also showed a higher diagnostic value among QSM histogram features, with an AUC of 0.848, a sensitivity of 73.9%, and a specificity of 100.0%. The other histogram features showed weak to moderate diagnostic performance.
Combination of features to improve the diagnostic performance
In this study, age showed a good diagnostic performance in identifying IDH genotypes and glioma subtypes, with AUCs of 0.785 for IDH-wildtype versus IDH-mutant, 0.793 for GBM versus astrocytoma, and 0.822 for GBM versus oligodendroglioma. Therefore, the histogram features with the best diagnostic performance of QSM and ADC (i.e., IQR of QSM and P10 of ADC) and age were combined to create regression equations, as shown in Appendix 1.
For separating IDH genotypes, the diagnostic performance of the regression equation improved compared with those of IQR of QSM (P=0.090) and P10 of ADC (P=0.021), with an AUC of 0.910, a sensitivity of 94.7%, and a specificity of 75.0%, as shown in Figure 4A.
For separating tumor subtypes, the diagnostic performance of the regression equation also improved accordingly. The AUC was 0.939 for GBM versus astrocytoma, which was higher than those of IQR of QSM (P=0.071) and P10 of ADC (P=0.009). Similarly, the AUC was 0.967 for GBM versus oligodendroglioma, which was also higher than those of IQR of QSM (P=0.075) and P10 of ADC (P=0.053), as shown in Figure 4B,4C.
Correlation of Ki-67 LI with histogram features of QSM and ADC
Positive correlations between the Ki-67 LI and the following QSM histogram features were identified: P90, IQR, maximum, MAD, RMS, skewness, and variance (rho ranged from 0.407 to 0.531, P<0.01 for all). Negative correlations with Ki-67 LI were found for P10 of QSM (rho=–0.452, P= 0.001) and P10 of ADC (rho=–0.554, P<0.001), as shown in Table S3 and Figure 5.
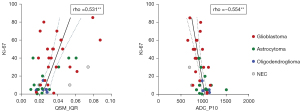
Discussion
Preoperative classification of gliomas is clinically important. The present study showed that the average susceptibility of QSM was higher, whereas the average ADC was lower in IDH-wildtype gliomas than in IDH-mutant gliomas, and in GBM than in non-GBM, but these differences were not significant. We found that histogram features of QSM and ADC were significantly associated with the IDH genotypes, tumor subtypes, and cellular proliferation of glioma; a recent study also found that compared to conventional MRI modalities, DL-assisted QSM shows great advantages in distinguishing other grade gliomas (OGG) from GBM, and predicting IDH1 subtypes (32). Our findings in identifying the IDH genotypes using average susceptibility of QSM are similar to those in a previous study (20), but unlike that study, average susceptibility of QSM did not help in identifying oligodendroglioma. The ADC histogram features are largely consistent with those in some previous studies (24,33-35).
On the one hand, IDH-wildtype glioma and GBMs are more aggressive, and immature new microvessels proliferated in the tumor are more likely to rupture and bleed. In this sense, the difference of the above QSM histogram features is reasonable, and there seemed to be a clear trend of increased average susceptibility associated with more aggressive tumors, suggesting that QSM can be used as an independent measure of tumor aggression and heterogeneity in brain tumors. On the other hand, IDH-mutant gliomas including astrocytoma and oligodendroglioma tend to have fewer diffusion barriers and a lower degree of tissue complexity such as less invasion in anatomic structures, decreased cellularity, and a more homogeneous tumor population, indicating that diffusion of the water molecule is not highly restricted in the tissue. In contrast, GBM is a mitotically active tumor characterized by microstructural changes with increased cellularity, cellular heterogeneity, hemorrhage, necrosis, and microvascular proliferation, which can obviously restrict the movement of water molecules (36). These histopathological differences could well explain the differences found in ADC histogram features between different IDH genotypes and tumor subtypes of gliomas.
In terms of analysis, compared with the average value, a usual measurement of the ROI, histogram analysis provides more abundant information on tumor characteristics, especially when the intensity distribution is inhomogeneous (37). The changes of histogram shape, asymmetry, and variation can also better reflect the change of tumor structure, physiology, molecules, and metabolism (38). This method showed potential for predicting tumor grade and differential diagnosis (39,40). This study found 7 of 12 QSM histogram features and 5 of 12 ADC histogram features exhibiting statistical differences between different IDH genotypes, and 5 of 12 QSM histogram features and 1 of 12 ADC histogram features exhibiting significant differences among tumor subtypes. QSM had more histogram features with significant differences than did ADC. The AUCs of QSM histogram features were also higher, particularly for those that describe the degree of numerical dispersion. This suggests that QSM histogram features can better capture the heterogeneity of tumors, and thus better discriminate IDH genotypes and tumor subtypes of adult-type diffuse gliomas based on CNS WHO classification 2021. Interestingly, no significant difference was found for the average susceptibility of QSM between different IDH genotypes, or for the average susceptibility of QSM and average ADC between different tumor subtypes. However, by using the histogram analysis, more abundant information or useful features on tumor characteristics were extracted (6,38), which enhanced the diagnostic performance of QSM and ADC in classifying gliomas. Another important finding was that the combination of age and the best histogram features of QSM and ADC achieved a great improvement in the diagnostic performance, suggesting that those combined indexes may serve as more effective imaging markers in identifying IDH genotypes and tumor subtypes of gliomas.
There are some limitations in this study. First, this is a single center study, and the sample size is relatively small, especially for the patients with oligodendroglioma. The ability of QSM and ADC histogram features for differentiating between the 3 tumor subtypes should be further validated in a larger patient cohort. Second, the ROI-based measurement might have caused some bias, especially in gliomas with unclear boundary.
Conclusions
QSM and ADC histogram features may serve as potential imaging markers for noninvasively assessing IDH genotypes and tumor subtypes of adult-type diffuse gliomas based on the CNS WHO classification 2021 by capturing more intrinsic microstructural and physiological information of tumors. Combining significant features may substantially improve the diagnostic performance.
Acknowledgments
Funding: This work was supported by grants from Guidance Project of Fujian Science and Technology Program (No.2022Y0024), the Science and Technology Plan Project of Fujian Health Commission (2022GGA013), the Natural Science Foundation of Fujian Province (No. 2022J01252), Startup Fund for Scientific Research, Fujian Medical University (No. 2021QH1025), Fujian Health Research Project (No. 2021QNA013), and the Scientific Research Project of Fujian Education Department (No. JAT210108).
Funding: This work was supported by
Footnote
Reporting Checklist: The authors have completed the STARD reporting checklist. Available at https://qims.amegroups.com/article/view/10.21037/qims-23-832/rc
Conflicts of Interest: All authors have completed the ICMJE uniform disclosure form (available at https://qims.amegroups.com/article/view/10.21037/qims-23-832/coif). Yang Song is from a commercial company, Siemens Healthineers Ltd., was an MR collaboration scientist conducting technical support in this study under Siemens collaboration regulation without any payment and personal interest pertaining to this study. The other authors have no conflicts of interest to declare.
Ethical Statement: The authors are accountable for all aspects of the work in ensuring that questions related to the accuracy or integrity of any part of the work are appropriately investigated and resolved. The study was conducted in accordance with the Declaration of Helsinki (as revised in 2013). The study was approved by the ethics committee board of Fujian Medical University Union Hospital, and informed consent was provided by all the patients.
Open Access Statement: This is an Open Access article distributed in accordance with the Creative Commons Attribution-NonCommercial-NoDerivs 4.0 International License (CC BY-NC-ND 4.0), which permits the non-commercial replication and distribution of the article with the strict proviso that no changes or edits are made and the original work is properly cited (including links to both the formal publication through the relevant DOI and the license). See: https://creativecommons.org/licenses/by-nc-nd/4.0/.
References
- van der Meulen M, Ramos RC, Mason WP, Von Deimling A, Maas SLN. Opinion & Special Article: Glioma Classification: How to Interpret Molecular Markers in a Diffuse Glioma Pathology Report. Neurology 2022. [Epub ahead of print]. pii:
10.1212/WNL.0000000000201262 . doi:10.1212/WNL.0000000000201262 .10.1212/WNL.0000000000201262 - Ostrom QT, Price M, Neff C, Cioffi G, Waite KA, Kruchko C, Barnholtz-Sloan JS. CBTRUS Statistical Report: Primary Brain and Other Central Nervous System Tumors Diagnosed in the United States in 2015-2019. Neuro Oncol 2022;24:v1-v95. [Crossref] [PubMed]
- Louis DN, Perry A, Reifenberger G, von Deimling A, Figarella-Branger D, Cavenee WK, Ohgaki H, Wiestler OD, Kleihues P, Ellison DW. The 2016 World Health Organization Classification of Tumors of the Central Nervous System: a summary. Acta Neuropathol 2016;131:803-20. [Crossref] [PubMed]
- Śledzińska P, Bebyn MG, Furtak J, Kowalewski J, Lewandowska MA. Prognostic and Predictive Biomarkers in Gliomas. Int J Mol Sci 2021;22:10373. [Crossref] [PubMed]
- Louis DN, Perry A, Wesseling P, Brat DJ, Cree IA, Figarella-Branger D, Hawkins C, Ng HK, Pfister SM, Reifenberger G, Soffietti R, von Deimling A, Ellison DW. The 2021 WHO Classification of Tumors of the Central Nervous System: a summary. Neuro Oncol 2021;23:1231-51. [Crossref] [PubMed]
- Gao A, Zhang H, Yan X, Wang S, Chen Q, Gao E, Qi J, Bai J, Zhang Y, Cheng J. Whole-Tumor Histogram Analysis of Multiple Diffusion Metrics for Glioma Genotyping. Radiology 2022;302:652-61. [Crossref] [PubMed]
- Li G, Li L, Li Y, Qian Z, Wu F, He Y, Jiang H, Li R, Wang D, Zhai Y, Wang Z, Jiang T, Zhang J, Zhang W. An MRI radiomics approach to predict survival and tumour-infiltrating macrophages in gliomas. Brain 2022;145:1151-61. [Crossref] [PubMed]
- Xu J, Ren Y, Zhao X, Wang X, Yu X, Yao Z, Zhou Y, Feng X, Zhou XJ, Wang H. Incorporating multiple magnetic resonance diffusion models to differentiate low- and high-grade adult gliomas: a machine learning approach. Quant Imaging Med Surg 2022;12:5171-83. [Crossref] [PubMed]
- Zhang HW, Lyu GW, He WJ, Lei Y, Lin F, Wang MZ, Zhang H, Liang LH, Feng YN, Yang JH. DSC and DCE Histogram Analyses of Glioma Biomarkers, Including IDH, MGMT, and TERT, on Differentiation and Survival. Acad Radiol 2020;27:e263-71. [Crossref] [PubMed]
- Ozturk-Isik E, Cengiz S, Ozcan A, Yakicier C, Ersen Danyeli A, Pamir MN, Özduman K, Dincer A. Identification of IDH and TERTp mutation status using (1) H-MRS in 112 hemispheric diffuse gliomas. J Magn Reson Imaging 2020;51:1799-809. [Crossref] [PubMed]
- Deistung A, Schweser F, Wiestler B, Abello M, Roethke M, Sahm F, Wick W, Nagel AM, Heiland S, Schlemmer HP, Bendszus M, Reichenbach JR, Radbruch A. Quantitative susceptibility mapping differentiates between blood depositions and calcifications in patients with glioblastoma. PLoS One 2013;8:e57924. [Crossref] [PubMed]
- Hangel G, Schmitz-Abecassis B, Sollmann N, Pinto J, Arzanforoosh F, Barkhof F, et al. Advanced MR Techniques for Preoperative Glioma Characterization: Part 2. J Magn Reson Imaging 2023;57:1676-95. [Crossref] [PubMed]
- Shmueli K, de Zwart JA, van Gelderen P, Li TQ, Dodd SJ, Duyn JH. Magnetic susceptibility mapping of brain tissue in vivo using MRI phase data. Magn Reson Med 2009;62:1510-22. [Crossref] [PubMed]
- Wang Y, Liu T. Quantitative susceptibility mapping (QSM): Decoding MRI data for a tissue magnetic biomarker. Magn Reson Med 2015;73:82-101. [Crossref] [PubMed]
- Li W, Wu B, Liu C. Quantitative susceptibility mapping of human brain reflects spatial variation in tissue composition. Neuroimage 2011;55:1645-56. [Crossref] [PubMed]
- de Rochefort L, Liu T, Kressler B, Liu J, Spincemaille P, Lebon V, Wu J, Wang Y. Quantitative susceptibility map reconstruction from MR phase data using bayesian regularization: validation and application to brain imaging. Magn Reson Med 2010;63:194-206. [Crossref] [PubMed]
- Harada T, Kudo K, Fujima N, Yoshikawa M, Ikebe Y, Sato R, Shirai T, Bito Y, Uwano I, Miyata M. Quantitative Susceptibility Mapping: Basic Methods and Clinical Applications. Radiographics 2022;42:1161-76. [Crossref] [PubMed]
- García Saborit M, Jara A, Muñoz N, Milovic C, Tepper A, Alliende LM, Mena C, Iruretagoyena B, Ramirez-Mahaluf JP, Diaz C, Nachar R, Castañeda CP, González A, Undurraga J, Crossley N, Tejos C. Quantitative Susceptibility Mapping MRI in Deep-Brain Nuclei in First-Episode Psychosis. Schizophr Bull 2023;49:1355-63. [Crossref] [PubMed]
- Cogswell PM, Wiste HJ, Senjem ML, Gunter JL, Weigand SD, Schwarz CG, Arani A, Therneau TM, Lowe VJ, Knopman DS, Botha H, Graff-Radford J, Jones DT, Kantarci K, Vemuri P, Boeve BF, Mielke MM, Petersen RC, Jack CR Jr. Associations of quantitative susceptibility mapping with Alzheimer’s disease clinical and imaging markers. Neuroimage 2021;224:117433. [Crossref] [PubMed]
- Zeng S, Ma H, Xie D, Huang Y, Wang M, Zeng W, Zhu N, Ma Z, Yang Z, Chu J, Zhao J. Quantitative susceptibility mapping evaluation of glioma. Eur Radiol 2023;33:6636-47. [Crossref] [PubMed]
- Guo AC, Cummings TJ, Dash RC, Provenzale JM. Lymphomas and high-grade astrocytomas: comparison of water diffusibility and histologic characteristics. Radiology 2002;224:177-83. [Crossref] [PubMed]
- Higano S, Yun X, Kumabe T, Watanabe M, Mugikura S, Umetsu A, Sato A, Yamada T, Takahashi S. Malignant astrocytic tumors: clinical importance of apparent diffusion coefficient in prediction of grade and prognosis. Radiology 2006;241:839-46. [Crossref] [PubMed]
- Bulakbasi N, Guvenc I, Onguru O, Erdogan E, Tayfun C, Ucoz T. The added value of the apparent diffusion coefficient calculation to magnetic resonance imaging in the differentiation and grading of malignant brain tumors. J Comput Assist Tomogr 2004;28:735-46. [Crossref] [PubMed]
- Liu T, Cheng G, Kang X, Xi Y, Zhu Y, Wang K, Sun C, Ye J, Li P, Yin H. Noninvasively evaluating the grading and IDH1 mutation status of diffuse gliomas by three-dimensional pseudo-continuous arterial spin labeling and diffusion-weighted imaging. Neuroradiology 2018;60:693-702. [Crossref] [PubMed]
- Wang Y, Fushimi Y, Arakawa Y, Shimizu Y, Sano K, Sakata A, Nakajima S, Okuchi S, Hinoda T, Oshima S, Otani S, Ishimori T, Tanji M, Mineharu Y, Yoshida K, Nakamoto Y. Evaluation of isocitrate dehydrogenase mutation in 2021 world health organization classification grade 3 and 4 glioma adult-type diffuse gliomas with 18F-fluoromisonidazole PET. Jpn J Radiol 2023;41:1255-64. [Crossref] [PubMed]
- Li W, Avram AV, Wu B, Xiao X, Liu C. Integrated Laplacian-based phase unwrapping and background phase removal for quantitative susceptibility mapping. NMR Biomed 2014;27:219-27. [Crossref] [PubMed]
- Li W, Wang N, Yu F, Han H, Cao W, Romero R, Tantiwongkosi B, Duong TQ, Liu C. A method for estimating and removing streaking artifacts in quantitative susceptibility mapping. Neuroimage 2015;108:111-22. [Crossref] [PubMed]
- Wu B, Li W, Guidon A, Liu C. Whole brain susceptibility mapping using compressed sensing. Magn Reson Med 2012;67:137-47. [Crossref] [PubMed]
- Wei H, Dibb R, Zhou Y, Sun Y, Xu J, Wang N, Liu C. Streaking artifact reduction for quantitative susceptibility mapping of sources with large dynamic range. NMR Biomed 2015;28:1294-303. [Crossref] [PubMed]
- Wei H, Zhang Y, Gibbs E, Chen NK, Wang N, Liu C. Joint 2D and 3D phase processing for quantitative susceptibility mapping: application to 2D echo-planar imaging. NMR Biomed 2017; [Crossref] [PubMed]
- Wu J, Guo T, Zhou C, Bai X, Liu X, Gu L, Xuan M, Gu Q, Huang P, Song Z, Zhang B, Xu X, Zhang M, Guan X. Genetic impacts on nigral iron deposition in Parkinson’s disease: A preliminary quantitative susceptibility mapping study. CNS Neurosci Ther 2023;29:1776-84. [Crossref] [PubMed]
- Rui W, Zhang S, Shi H, Sheng Y, Zhu F, Yao Y, Chen X, Cheng H, Zhang Y, Aili A, Yao Z, Zhang XY, Ren Y. Deep Learning-Assisted Quantitative Susceptibility Mapping as a Tool for Grading and Molecular Subtyping of Gliomas. Phenomics 2023;3:243-54. [Crossref] [PubMed]
- Xing Z, Zhang H, She D, Lin Y, Zhou X, Zeng Z, Cao D. IDH genotypes differentiation in glioblastomas using DWI and DSC-PWI in the enhancing and peri-enhancing region. Acta Radiol 2019;60:1663-72. [Crossref] [PubMed]
- Aliotta E, Dutta SW, Feng X, Tustison NJ, Batchala PP, Schiff D, Lopes MB, Jain R, Druzgal TJ, Mukherjee S, Patel SH. Automated apparent diffusion coefficient analysis for genotype prediction in lower grade glioma: association with the T2-FLAIR mismatch sign. J Neurooncol 2020;149:325-35. [Crossref] [PubMed]
- Cindil E, Sendur HN, Cerit MN, Erdogan N, Celebi F, Dag N, Celtikci E, Inan A, Oner Y, Tali T. Prediction of IDH Mutation Status in High-grade Gliomas Using DWI and High T1-weight DSC-MRI. Acad Radiol 2022;29:S52-62. [Crossref] [PubMed]
- Le Rhun E, Preusser M, Roth P, Reardon DA, van den Bent M, Wen P, Reifenberger G, Weller M. Molecular targeted therapy of glioblastoma. Cancer Treat Rev 2019;80:101896. [Crossref] [PubMed]
- Liu HS, Chiang SW, Chung HW, Tsai PH, Hsu FT, Cho NY, Wang CY, Chou MC, Chen CY. Histogram analysis of T2*-based pharmacokinetic imaging in cerebral glioma grading. Comput Methods Programs Biomed 2018;155:19-27. [Crossref] [PubMed]
- Just N. Improving tumour heterogeneity MRI assessment with histograms. Br J Cancer 2014;111:2205-13. [Crossref] [PubMed]
- Li D, Cui Y, Hou L, Bian Z, Yang Z, Xu R, Jia Y, Wu Z, Yang X. Diffusion kurtosis imaging-derived histogram metrics for prediction of resistance to neoadjuvant chemoradiotherapy in rectal adenocarcinoma: Preliminary findings. Eur J Radiol 2021;144:109963. [Crossref] [PubMed]
- Xie T, Zhao Q, Fu C, Grimm R, Gu Y, Peng W. Improved value of whole-lesion histogram analysis on DCE parametric maps for diagnosing small breast cancer (≤ 1 cm). Eur Radiol 2022;32:1634-43. [Crossref] [PubMed]