Quality assessment of radiomics models in carotid plaque: a systematic review
Introduction
Stroke is one of the most serious diseases threatening human health and is the second leading cause of death around the world after ischemic heart disease (1). In China, it is the most common cause of death and disability, with a standardized prevalence ranging from 7.2% to 4.25% (2,3); meanwhile, in the United States, the mortality rate among Americana 35 to 64 years of age has increased over the past two decades (4,5). Stroke is thus a massive health and economic burden for global society. Carotid atherosclerosis (CAS) is a chronic, progressive disease characterized by focal fibrosis, lipid accumulation, and plaque formation. It causes about 7–18% of ischemic strokes (6) and affects about one-quarter of the population (7). Unfortunately, therapy options for individuals with severe CAS remain unsatisfactory (8-10); hence, risk stratification and individualized treatment of these patients are critical. For high-risk patients, combining strict best medical therapy (BMT) with aggressive revascularization therapy can prevent the occurrence of ischemic events. In contrast, BMT alone may be a preferable option for low-risk patients, as the danger of perioperative stroke and death can also be avoided. As a result, the 2017 European Society of Vascular Surgery Clinical Practice Guidelines stated that there is a need for the development of clinical and imaging algorithms to identify patients with high-risk CAS who require revascularization therapy (11).
Radiomics is an emerging multidisciplinary intersectional research field that integrates digital image information, statistics, artificial intelligence, machine learning, and deep learning methods to transform traditional radiological image information into comprehensive features for quantitative research. It has exhibited exciting potential for oncological diagnosis, differential diagnosis, and prognostic prediction (12) and has opened new possibilities in atherosclerosis (AS) research (13,14). An increasing number of studies have implemented radiomics algorithms preoperatively for AS risk stratification and prognostic assessment, indicating that radiomics-based features have greater potential and lower heterogeneity than do traditional radiological methods (15), especially in coronary artery diseases (16,17). Since radiomics models are predictive, focusing solely on statistical metrics such as the area under the receiver operating characteristic curve (AUC) when evaluating a model seems arbitrary. That is to say, the risk of bias in radiomics models should be assessed before applying the promising results of these earlier studies into actual clinical practice. A model, even if it has a high AUC, is not reliable if it has a high risk of bias in the development and validation processes. A rigorous and transparent study process accompanied by standardized reporting can improve the reproducibility and reliability of radiomics models. Several recent studies have attempted to evaluate the prediction power and the methodological quality of radiomics models based on the radiomics quality score (RQS) tool (18,19), assessing the risk of bias using the Prediction Model Risk of Bias Assessment Tool (PROBAST) (20) and investigating the report quality of the TRIPOD (Transparent Reporting of a multivariable prediction model for Individual Prognosis Diagnosis) checklist (21). In our previous study, radiomics models for CAS had low RQS scores (19); however, there are no published results concerning the use of PROBAST or TRIPOD for CAS. Therefore, in this review, we aimed to analyze the current status of radiomics research related to diagnosing and/or predicting CAS by combining these three above-mentioned tools (TRIPOD, PROBAST, and RQS) to evaluate their scientific reporting quality, risk of bias, and radiomics methodological quality. We present this article in accordance with the Preferred Reporting Items for Systematic Reviews and Meta-Analyses (PRISMA) reporting checklist (available at https://qims.amegroups.com/article/view/10.21037/qims-23-712/rc).
Methods
Search strategy
We systematically searched PubMed, Web of Science, and the Cochrane Library for articles published in English up to March 2023. We searched the titles, abstracts, and keywords of literature using the following search phrases: “radiomics/radiomic/texture/textural”, “carotid arteries”, and “atherosclerosis/atherosclerotic plaque/carotid stenosis”. Keywords in different groups were linked together by “AND”, whereas those in the same group were combined using “OR”. Table S1 provides the search details for each database. The protocol of this systematic review has been registered in PROSPERO (International Prospective Register of Systematic Reviews; registration No. CRD42023407441).
Study selection
Literature was screened according to the inclusion and exclusion criteria of this review (Table 1). Two reviewers (S.L. and S.Z.) independently screened the titles and abstracts for initial selection and then performed a full-text review of each paper to identify the studies eligible for final analysis. Any disagreements were resolved via mediation by a senior reviewer (L.P.L.). Reference lists of the eligible studies and pre-existing systematic reviews/meta-analyses were also searched manually to identify any potentially eligible studies.
Table 1
Items | Inclusion criteria | Exclusion criteria |
---|---|---|
Population | Human participants (adults, age ≥18 years) with carotid plaque or carotid atherosclerosis | Nonhuman participants (animals or modeling data generated algorithmically) |
Intervention | Assess application of radiomics or texture analysis to patient data; develop a diagnostic or prognostic model by using radiomics features with or without other features | Deep-learning research without any texture feature in the model; assess the predictive value of a single feature without any prediction model |
Comparison | Human clinicians or previously validated models | NA |
Outcome | Model performance | NA |
Study design | Published peer-reviewed scientific reports (prospective or retrospective) published in English until the search date | Letters, reviews, case reports, abstracts, editorial, or other informal publication types |
PICO, patient/population, intervention, comparison and outcomes; NA, not applicable.
Data extraction
For each study, information including first author, year of publication, country, study type, population size, mean age, history, imaging modality, number of candidate and final predictors, modeling method, predictive performance, and validation methods was extracted from the full text. If there were multiple prediction models in a study, the one that performed best in the test or training group was selected.
TRIPOD, consisting of 22 main criteria with 37 items, is a guideline specifically devised for reporting studies that developed or validated multivariate predictive models and was used in our review to assess the reporting quality of the included studies (22). Although both the Quality Assessment of Diagnostic Accuracy Studies-2 (QUADAS-2) and PROBAST assess the risk of bias and applicability, the former tends to focus on diagnostic performance rather than predictive value, whereas the latter focuses on primary studies that developed and/or validated multivariable prediction models for diagnosis or prognosis. Since radiomics models have predictive values, we used PROBAST (23) to assess the concerns regarding the applicability and risk of bias of these included studies. Finally, the methodological quality of the included studies was evaluated with the RQS tool, which comprises 16 different criteria within six categories. Each item has a score range from −5 to 7, giving a total score of 36 points (100%) (24). Extraction and assessment were completed by two reviewers (S.L. and S.Z.), and any discrepancies were resolved via discussion. The details and description of these 3 evaluation methods are table available at https://cdn.amegroups.cn/static/public/qims-23-712-1.xls.
Statistical analysis
The extracted information of each study was entered into Microsoft Excel and computed with internal Excel tools. Descriptive statistics were calculated as the mean and SD for continuous variables and as frequencies and rates for categorical data. The overall TRIPOD adherence rate was statistically and numerically explained (adherence rate ≤33.3%, poor; 33.3%< adherence rate ≤66.7%, moderate; adherence rate >66.7%, good) (20). We did not undertake meta-analysis due to the high heterogeneity of the multiple variables and classifiers used in the final modeling. SPSS 25 (IBM Corp.) was used to perform statistical analyses.
Results
Literature selection
After implementation of the search strategy, 406 studies were initially identified. Following removal of 229 duplicates, 174 studies were screened for titles and abstracts; of these, 24 inappropriate types of publication and 89 studies that were irrelevant to the purpose of this review were excluded through more detailed assessment. Finally, a full-text screening identified 19 studies that met the inclusion criteria for this review (25-43) (Figure 1).
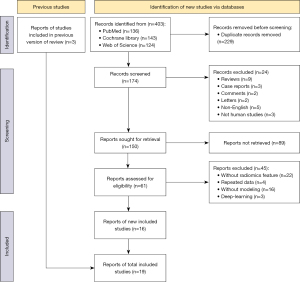
General characteristics
Almost all studies (18/19, 94.7%) were single-center research published between July 2014 and January 2023, of which 84.2% (16/19) were published within the past 3 years. Only 1 study was prospectively designed (32). The majority of study participants were Chinese (12/19, 63.1%), followed by Italian (3/19, 15.8%) and British (2/19, 10.5%). Overall, 2,738 patients (1,706 males) were enrolled, with population sizes ranging from 21 to 548 (mean 144), and the mean age was 66.02±9.52 years. Among the included studies, 7 (36.8%) had fewer than 100 participants. Computed tomography angiography (CTA) was the most prevalent imaging modality (n=7, 36.8%), followed by ultrasonography (US) (n=6, 31.5%); computed tomography (CT), high resolution magnetic resonance imaging (HRMRI), 3D HRMRI, MRI, 3D US, and positron emission tomography CT (PET/CT) were the other modalities used (n=1, 5%). Three kinds of features, including radiomics, conventional radiologic, and clinical variables, were used as candidate predictors for further selection and modeling, with the number of candidate features ranging from 2 to 4,198 (mean 768). Least absolute shrinkage and selection operator regression, logistic regression, and support vector machine were common classifiers for the final model. For predictive power, the mean AUC of the final models in training group was 0.876±0.09, ranging from 0.741 to 0.989. Internal validation was performed in 14 studies (25-27,29,32-38,40,41,43), and 1 study employed external validation (42), but only 12 studies (25,26,29,32-34,36-38,40,41,43) reported the AUCs of the validation groups, ranging from 0.73 to 0.986. Model calibration was investigated in 7 studies (36.8%) and demonstrated good calibration performance (25,30,32,37,40,42,43).
Through radiomics or texture analysis, 10 studies tended to classify unstable or risk plaques (25,27,29,32,33,39-43). Moreover, 5 studies focused on predicting unfavorable cerebral and cardiovascular events (28,35-38), 2 on evaluating stenosis or in-stent restenosis (26,30), 1 on assessing the robustness of radiomics features (34), and 1 on evaluating the relationship between glycated hemoglobin and ultrasound plaque textures (31). The details are shown in Table 2.
Table 2
Study | Country | Study design | No. of patients | Age, mean ± SD, years | Males | Participant type | Modality | Candidate features (RF/TRF/CF) | Modeling method | No. of features in the final model | AUC in training set (95% CI) | Validation and method | Validation performance (95% CI) |
---|---|---|---|---|---|---|---|---|---|---|---|---|---|
Chen et al., 2022 (24) | China | R | 115 | 51.38±13.32 | 91 | ≥30% stenosis | HRMRI | 1,130 (1,121/2/7) | LASSO, LR | 8 | 0.93 (0.88–0.98) | Internal, 10-FCV | 0.91 (0.81–1.00) |
Cheng et al., 2022 (25) | China | R | 221 | 66.89±8.07 | 186 | Carotid endarterectomy | CTA | 2,134 (2,107/10/17) | Cox, LASSO | 6 | 0.88 (0.82–0.95) | Internal, 5-FCV | 0.83 (0.74–0.91) |
Cilla et al., 2022 (26) | Italy | R | 30 | 72.96 | 19 | >70% stenosis | CTA | 230 (230/0/0) | LR, SVM, CART | 2 | 0.99 (NA) | Internal, 5-FCV | NA |
Colombi et al., 2021 (27) | Italy | R | 172 | 77 | 112 | Underwent carotid artery stenting | CTA | 20 (2/9/9) | LR | 3 | 0.79 (0.73–0.85) | NA | NA |
Dong et al., 2022 (28) | China | R | 120 | 66.68±7.75 | 100 | ≥50% stenosis | CTA | 2,129 (2,107/8/14) | LR, SVM, SGBOOST | 20 | 0.86 (0.78–0.93) | Internal, 5-FCV | 0.85 (NA) |
Ebrahimian et al., 2022 (29) | USA | R | 85 | 73±10 | 56 | Suspected or known stenosis | US | 78 (74/0/4) | LR | 10 | 0.94 (NA) | NA | NA |
Huang et al., 2016 (30) | China | R | 136 | 68.6±8.86 | NA | Carotid plaques | US | 318 (300/2/16) | Linear regression | 12 | 0.83 (NA) | NA | NA |
Huang et al., 2022 (31) | China | P | 548 | 62±10 | 373 | Carotid plaque | US | 124 (107/17/10) | LASSO, LR | 4 | 0.93 (0.90–0.96) | Internal, randomly | 0.912 (0.87–0.96) |
Kafouris et al., 2021 (32) | France | R | 21 | 70.43±7 | 18 | High-grade stenosis | 18F-FDG PET | 67 (67/0/0) | LR | 1 | 0.97 (NA) | Internal, 200 bootstrap | 0.87 (NA) |
Le et al., 2021 (33) | UK | R | 41 | 63.47±8.89 | 32 | Carotid artery-related stroke or TIA | CTA | 96 (93/3/0) | LASSO, SVW, decision tree, random forest | 3 | NA | Internal, 5-FCV | 0.73±0.09 |
Lo et al., 2022 (34) | China | R | 177 | 61.5 | 89 | Stroke | CCD | 49 (49/0/0) | LR, SVW, | 11 | 0.94 (NA) | Internal, LOOCV | NA |
van Engelen et al., 2014 (35) | Netherlands | R | 298 | 70.45 | 110 | Plaque area between 40–600 mm2 | 3D US | 402 (376/3/23) | Cox | 8 | 0.78 (NA) | Internal, 10-FCV | 0.94 (0.86–1.00) |
Wang et al., 2022 (36) | China | Cohort | 105 | 63.4 | 73 | CAD | US | 883 (851/11/21) | LASSO, LR | 11 | 0.741 (0.65–0.84) | Internal, 10-FCV | 0.94 (0.86–1.00) |
Xia et al., 2023 (37) | China | R | 179 | 65.4 | 119 | 30–50% stenosis | CTA | 142 (129/0/13) | XGBoost, KNN, SVM, LR | 11 | 0.98 (0.98–0.99) | Internal, 5-FCV | 0.88 (0.79–0.98) |
Zaccagna et al., 2021 (38) | UK | R | 24 | 63±10 | 14 | Carotid atherosclerosis | CT | 25 (6/6/13) | NA | 6 | 0.81 (NA) | NA | NA |
Zhang et al., 2022 (39) | China | Case-control | 150 | 61.7±10 | 120 | Atherosclerotic plaque | US | 331 (303/4/24) | LASSO, LR | 8 | 0.88 (NA) | Internal, 10-FCV | 0.87 |
Zhang et al., 2021 (40) | China | R | 162 | 66.8±7.35 | 148 | >30% stenosis | MRI | 802 (788/8/6) | LASSO, LR | 33 | 0.99 (NA) | Internal, 1000 Bootstrap | 0.99 |
Zhang et al., 2022 (41) | China | R | 64 | 60.9±10.3 | 46 | Carotid atherosclerosis | CTA | 1,414 (1,409/4/11) | LASSO, LR | 9 | 0.743 (0.65–0.84) | External, 5-FCV | 0.81 (0.66–0.96) |
Zhang et al., 2023 (42) | China | R | 90 | / | NA | 50% stenosis | 3D-HRMRI | 4,198 (4,170/4/24) | LASSO, LR | 17 | 0.96 (0.92–0.99) | Internal, 5-FCV | 0.86 (0.72–1.00) |
RF/TRF/CF, radiomics feature/traditional radiological feature/clinical feature; AUC, area under the curve; CI, confidence interval; R, retrospective study; HRMRI, high-resolution magnetic resonance; LASSO, least absolute shrinkage and selection operator; LR, logistic regression; FCV, folds cross-validation; CTA, computed tomography angiography; SVM, support vector machine; CART, classification and regression trees; US, ultrasonography; NA, not available; P, prospective study; TIA, transient ischemic attack; 18F-FDG PET, fluorine-18 fluorodeoxyglucose positron emission tomography; UK, United Kingdom; CCD, carotid color doppler; LOOCV, leave-one-out cross-validation; KNN, k-nearest neighbor; CAD, coronary artery disease.
Quality of reporting
The quality of reporting for included studies from the TRIPOD checklist is shown in Figure 2. The mean overall TRIPOD adherence rate was 66.1% (SD 12.8%), with a range of 45% to 87%. Of the 19 studies, 18 were model development studies, and only 1 aimed to develop and externally validate the same model (42). Of the 31 TRIPOD items, 8 (3a, 3b, 4a, 6a, 7a, 14a, 18, 19b) were completely described in all studies, 11 (4b, 5b, 5c, 8, 10a, 10b, 11, 14, 20, 21 22) were specified in more than 60% of the studies, the remaining 9 (5a, 6b, 7b, 9, 10d, 13a, 15a, 15b, 16) were described in fewer than 50% of the studies. Notably, 3 items (1, 2, 13b) were poorly represented in all studies.
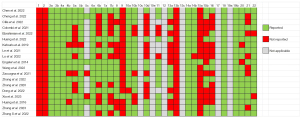
For domain “Title and abstract”, there was no study that reported the type of study (development or validation of a model) in item 1 or the information regarding the data sources, study settings, and model calibration results in item 2. Moreover, 9 studies reported blind outcome assessments, whereas blind predictors assessment (item 7b) was presented in another 9 studies. Only 1 study described the details for managing data (item 9) (38). No study reported the number of participants with missing data for predictors (item 13b). For model performance, 18 studies calculated the concordance index or AUC and other performance parameters such as sensitivity and specificity. Only 1 study described the full prediction model (36), and 8 studies used a nomogram to explain how the models generated personalized predictions (item 15b). Finally, 2 studies failed to discuss the implications for further research (33,36), and appendix information was not available in 7 studies (26,27,30,31,35,37,39).
Risk of bias
Generally, the overall risk of bias was high for all studies, especially in “Analysis” category (Figure 3A). The major source of bias stemmed from signaling question 4.1, with no study having reasonable number of participants. All studies exhibited a low events per variable (EPV) value due to the large amount of candidate features and the limited carotid plaque samples. Regarding missing data, 12 studies conducted complete-case analysis and manually eliminated individuals without satisfactory images or clinical data or those who were lost to follow-up (25,26,28-30,32,36-41). In contrast, 7 studies did not describe how missing data were handled or the analytical procedures used to assess missing data (27,31,33-35,42,43), 4 studies lacked validation (28,30,31,39), and 2 studies had a high risk of bias in domain 1 for the stated inappropriate inclusion or exclusion criteria (33,39). The time interval between predictor assessment and outcome determination was unclear in 6 studies (25,30,31,35,40,42). Of note, the overall risk of applicability was low: only 2 studies (33,39) had a high risk in the participant domain, and 6 studies (25,27,30,31,35,42) had an unclear risk in the outcome domain (Figure 3B).
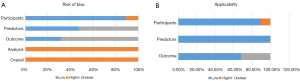
RQS results
The lowest RQS score was −2 points while the highest score was 22 points, with an average RQS score of 9.89 (±5.70), which is equal to 27.4% of the total possible points achievable. Approximately 63.1% (12/19) of studies received 10 to 20 points, accounting for 27.8% to 55.6% of the maximum score. Among the 16 RQS components, 4 were underutilized in all studies: phantom study, imaging at multiple time points, cost-effectiveness analysis, and open science and data. Image protocols in all studies were well-documented without any public sources, and discrimination statistics were well reported with no resample method; therefore, each study was scored 1 point in item 1, and 9. A proportion of 68.4% (13/19) of the studies mentioned 2 physicians who segmented the regions of interest to evaluate the stability and repeatability of texture features. Two studies did not take any measurements to reduce the number of retrieved features (28,39), while another two studies only used radiomics features in their candidate predictors (27,35). A biological correlate was analyzed in 1 study (33), and 5 studies calculated cutoff values to delineate risk groups (30,32,33,39,41). Seven studies reported calibration statistics using calibration plots or the Hosmer-Lemeshow test (17,30,32,37,40,42,43), and 73.7% (14/19) of the studies had internal validation, with only one study using external validation (42). Two studies did not include a comparison with a gold standard (30,34). Five studies conducted decision curve analysis to determine the current and potential application of the models (32,37,42,43,44). The details of RQS results are shown in Table 3.
Table 3
Study | I | II | III | IV | V | VI | VII | VIII | IX | X | XI | XII | XIII | XIV | XV | XVI | Total points (N=36), n (%) |
---|---|---|---|---|---|---|---|---|---|---|---|---|---|---|---|---|---|
Chen et al., 2022 (24) | 1 | 1 | 0 | 0 | 3 | 1 | 0 | 0 | 1 | 1 | 0 | 2 | 2 | 2 | 0 | 0 | 14 (38.89) |
Cheng et al., 2022 (25) | 1 | 1 | 0 | 0 | 3 | 1 | 0 | 0 | 1 | 0 | 0 | 2 | 2 | 0 | 0 | 0 | 11 (30.56) |
Cilla et al., 2022 (26) | 1 | 1 | 0 | 0 | 3 | 0 | 0 | 0 | 1 | 0 | 0 | 2 | 2 | 0 | 0 | 0 | 10 (27.78) |
Colombi et al., 2021 (27) | 1 | 1 | 0 | 0 | −3 | 1 | 0 | 0 | 1 | 0 | 0 | −5 | 2 | 0 | 0 | 0 | −2 (−5.56) |
Dong et al., 2022 (28) | 1 | 1 | 0 | 0 | 3 | 1 | 0 | 1 | 1 | 1 | 0 | −5 | 0 | 0 | 0 | 0 | 4 (11.11) |
Ebrahimian et al., 2022 (29) | 1 | 1 | 0 | 0 | 3 | 1 | 0 | 1 | 1 | 1 | 7 | 2 | 2 | 2 | 0 | 0 | 22 (61.11) |
Huang et al., 2016 (30) | 1 | 0 | 0 | 0 | 3 | 0 | 0 | 0 | 1 | 0 | 0 | 2 | 2 | 0 | 0 | 0 | 9 (25.00) |
Huang et al., 2022 (31) | 1 | 1 | 0 | 0 | 3 | 1 | 0 | 0 | 1 | 0 | 0 | 2 | 0 | 0 | 0 | 0 | 9 (25.00) |
Kafouris et al., 2021 (32) | 1 | 0 | 0 | 0 | 3 | 1 | 0 | 0 | 1 | 0 | 0 | 2 | 2 | 0 | 0 | 0 | 10 (27.78) |
Le et al., 2021 (33) | 1 | 0 | 0 | 0 | 3 | 1 | 0 | 0 | 1 | 1 | 0 | 2 | 2 | 2 | 0 | 0 | 13 (36.11) |
Lo et al., 2022 (34) | 1 | 0 | 0 | 0 | −3 | 1 | 0 | 1 | 1 | 0 | 0 | −5 | 2 | 0 | 0 | 0 | −2 (−5.56) |
Engelen et al., 2014 (35) | 1 | 0 | 0 | 0 | 3 | 1 | 1 | 1 | 1 | 0 | 0 | 2 | 2 | 0 | 0 | 0 | 12 (33.33) |
Wang et al., 2022 (36) | 1 | 1 | 0 | 0 | 3 | 1 | 0 | 0 | 1 | 1 | 0 | 2 | 2 | 0 | 0 | 0 | 12 (33.33) |
Xia et al., 2023 (37) | 1 | 1 | 0 | 0 | 3 | 1 | 0 | 1 | 1 | 0 | 0 | 2 | 2 | 0 | 0 | 0 | 12 (33.33) |
Zaccagna et al., 2021 (38) | 1 | 1 | 0 | 0 | 3 | 1 | 0 | 0 | 1 | 0 | 0 | 2 | 2 | 0 | 0 | 0 | 11 (30.56) |
Zhang et al., 2022 (39) | 1 | 1 | 0 | 0 | 3 | 1 | 0 | 0 | 1 | 0 | 0 | −5 | 2 | 0 | 0 | 0 | 4 (11.11) |
Zhang et al., 2021(40) | 1 | 0 | 0 | 0 | 3 | 1 | 0 | 0 | 1 | 0 | 0 | 2 | 2 | 0 | 0 | 0 | 10 (27.78) |
Zhang et al., 2022 (41) | 1 | 1 | 0 | 0 | 3 | 1 | 0 | 0 | 1 | 1 | 0 | 3 | 2 | 2 | 0 | 0 | 15 (41.67) |
Zhang et al., 2023 (42) | 1 | 1 | 0 | 0 | 3 | 1 | 0 | 0 | 1 | 1 | 0 | 2 | 2 | 2 | 0 | 0 | 14 (38.89) |
I, image protocol quality; II, multiple segmentation; III, phantom study; IV, imaging at multiple time points; V, feature reduction; VI, multivariable analysis with non-radiomics features; VII, biological correlates; VIII, cutoff analysis; IX, discrimination statistics; X, calibration statistics; XI, prospective study; XII, validation; XIII, comparison to gold standard; XIV, potential clinical utility; XV, cost-effectiveness analysis; XVI, open science and data.
Discussion
Even with the growing number of radiomics studies, there have been relatively few cases of radiomics models being successfully translated into clinically useful tools or receiving Food and Drug Administration approval. This can partially be attributed to the limited repeatability and reproducibility of the developed models. Therefore, reliable radiomics model with a standardized modeling process, high-quality reporting, and a lower risk of bias are necessary. In this systematic review, TRIPOD, PROBAST, and RQS were used to assess various aspects of radiomics models for carotid plaques. These models had adequate AUCs to show their predictive or diagnostic power for CAS, and adherence to TRIPOD was generally relatively good throughout these studies; however, some key aspects of the assessment methods were lacking, and studies with low RQS values and a high risk of bias were also common. These findings illustrate that current radiomics models may not yet be applicable for carotid atherosclerotic plaque evaluation in actual clinical practice and there is still room for the improvement of radiomics methods.
TRIPOD is a guideline specifically designed to assess the development or validation of multivariate prediction models for diagnostic or prognostic purposes and enables the scientific or medical community to objectively examine the strengths and shortcomings of prediction model-based research. RQS is a tool for standardizing and improving the methodological quality of radiomics reports. Finally, PROBAST functions to assess the risk of bias and applicability of diagnostic and prognostic prediction model studies. Study design, predictor selection, and model performance are three intersecting items within these checklists. First, for study design, both TRIPOD and PROBAST encourage authors to enroll acceptable data sources with suitable inclusion and exclusion criteria; however, in our review, this was poorly reported in 68.4% (13/19) of the included articles. Notably, a prospective study earned 7 points in the RQS tool, although this was the only prospective trial, accounting for only 5.2% (1/19) of the included literature. Overall, radiomics studies with prospective designs are lacking, both in the oncologic and cardiovascular fields (45,46). Prospective studies have many desirable properties, but they can be time-consuming and costly, and a prospective-retrospective hybrid study with a well-designed protocol may serve as a suitable compromise (47). Second, predictors play key role in model development. Region of interest segmentation and feature selection are the two prerequisite steps before final model implementation. TRIPOD requires authors to report predictors included in the final model in the abstract and results sections, and predictor definition is needed in TRIPOD and PROBAST. The approach employed for feature reduction and selection to limit the risk of overfitting is necessary in RQS and TRIPOD; fortunately, most of included studies in our review satisfied these requirements. Additionally, radiomics models included multivariable analysis with non-radiomics features in modeling, and detecting the correlation between radiomics features and biological factors is expected to deepen the understanding of radiomics and biology but appears to have better prospects in the oncologic field (48,49) than in the cardiovascular field (45). However, according to the consensus of the European Society of Radiology, biological relevance can be established after clinical validation. Third, model performance is typically evaluated in terms of discrimination and calibration: the former was achieved in all included studies, but the latter in less than half of them. In addition to ROC curve and AUC or concordance index, sensitivity, specificity, predictive values, and decision curve analysis are also excellent indicators of model power. Using appropriate model validation techniques to show the sufficiently robust ability of a developed model to predict an end point of interest is required before the model can be translated into clinical use (47). An externally validated model is considered to be more credible than is an internally validated one, as the former obtains more individualized data (e.g., varying temporally or geographically), which reinforces the validation and decreases model overfitting risk (50). In a comprehensive review of the internal validation approach, data from simulation studies showed that inadequate internal validation methods can still result in AUC estimates of 0.7–0.8 even when variables are not relevant to the outcome (51). Even though the external validation based on 3 or more datasets scored 5 points in RQS, the study by Zhang et al. was the only study in our review that applied a single external data source for validation (42). External validation was better performed in oncology studies; for instance, in Park et al.’s systematic review, which enrolled 77 radiomics studies (including 70 in the field of oncology), 18.2% (14/77) of the studies were externally validated (21).
Other questionable items with TRIPOD and PROBAST were related to participants, handling of missing data, and blinding of the assessment of predictors and outcomes. The average population size in the 19 included studies was 144, with a mean number of candidate features of 768. Almost all studies had missing data, but only that by Xia et al. imputed mean values with missing values (38). Moreover, 52.6% of studies did not mention any information concerning blindness assessment. In theory, the larger the sample size is, the smaller the standard error and the narrower the confidence intervals are, leading to more accurate results and less risk of overfitting and underfitting. Currently, an EPV value of at least 10 is the baseline criterion for radiomics models; for validation models, an EPV larger than 100 is preferable (52). Although different opinions on these criteria are inevitable (53-55), a well-planned prospective longitudinal cohort study, in practice, will allow researchers to predetermine sample size based on statistical grounds and generate plausible records. Missing data are unavoidable in research, and most studies eliminate patient data from analysis if an outcome or predictor is lacking to perform complete-case analysis. Not only is it inefficient to include only participants with complete data, but the remaining participants without missing data are not representative of the entire original study sample (i.e., they are selective participants). Missing data are consistently inadequately reported and managed, as shown by systematic reviews of methodological practices and research reports on predictive model development and evaluation (56-58). There are already several imputation approaches that may be used to estimate missing cases from multiple imputation datasets in statistical packages (such as Stata, R, SAS, and Python) (59). Blinded assessment of predictor and outcome is important not only in prognostic trails but also in diagnostic model studies to reduce the risk of bias (60,61). Knowledge of predictor results may affect how outcomes are determined; in other words, lacking blinding of predictor accessors to outcome information increases their association and inflates model performance estimates (62,63).
For RQS, no study in our review reported on the cost-effectiveness or open science and data. Given the status of radiomics in methodological and clinical validation, the evaluation of cost-effectiveness may be perceived to be less urgent (18,45). However, cost-effectiveness analysis can assess the value of radiomics predictive models in health economics when applied clinically (19,64). New models with comparable prediction power should not be more expensive than pre-existing ones. In addition, adopting open access to scientific data ensures better clinical applicability and academic transparency, as researchers will be able to use the database for external validation, reproduction, or replication if there is available publication of code and partial disclosure of raw data (24,65). It is worth mentioning that “phantom study” and “test-retest analysis” were two more items in which the reviewed studies showed poor performance, and these were intended to detect any uncertainties associated with organ movement or contraction, and a phantom study in particular can recognize potential differences in characteristics between suppliers. The reliability of radiomics features depends on the choice of feature calculation platforms and software version, and failure to harmonize calculation settings results in poor reliability, even on platforms compliant with the Image Biomarker Standardization Initiative (66).
This review has some inherent limitations. First, we did not search literature through Embase or Scopus, which might have led to some key data being missed. Second, direct comparisons of AUCs between individual studies suggested the potential of radiomics in assessing carotid plaque, but we were unable to evaluate the pooled predictive power via meta-analysis due to different research objectives, high heterogeneity, and wide variations in predictor variables. Furthermore, future prospective studies designed with a reasonable EPV and external validation are recommended, as there was a lack of sufficient evidence from prospective studies and independent external validation cohorts. Finally, the results of RQS, PROBAST, and TRIPOD were not always consistent. RQS can help assess the methodological quality of radiomics studies but does not evaluate sources of bias; moreover, obtaining a perfect RQS score is extremely difficult due to complex computation and subtractive factors (45). The PROBAST primarily deals with regression-based clinical prognostic models instead of radiomics models, whereas TRIPOD is a benchmark for traditional predictive model development studies and is less suitable for radiomics research of image analysis and large feature libraries. Thus, comprehensive checklists for studies based on artificial intelligence, such as TRIPOD-AI and PROBAST-AI, will be more viable in the future (67).
Conclusions
Our review suggests that radiomics models have potential value in assessing carotid plaque, but the overall methodology and reporting quality of radiomics studies on carotid plaque remain inadequate, and many obstacles must be overcome before these models can be translated into clinical practice. We anticipate that reliable and reproducible imaging predictions will be achieved under rigorously designed prospective study with sufficiently large sample sizes and external validation. Greater attention should be paid to the correlations among radiomics features and gene expressions, the handling of missing data, analysis methods, and open-access code and data.
Acknowledgments
Funding: This study has received
Footnote
Reporting Checklist: The authors have completed the PRISMA reporting checklist. Available at https://qims.amegroups.com/article/view/10.21037/qims-23-712/rc
Conflicts of Interest: All authors have completed the ICMJE uniform disclosure form (available at https://qims.amegroups.com/article/view/10.21037/qims-23-712/coif). The authors have no conflicts of interest to declare.
Ethical Statement: The authors are accountable for all aspects of the work in ensuring that questions related to the accuracy or integrity of any part of the work are appropriately investigated and resolved.
Open Access Statement: This is an Open Access article distributed in accordance with the Creative Commons Attribution-NonCommercial-NoDerivs 4.0 International License (CC BY-NC-ND 4.0), which permits the non-commercial replication and distribution of the article with the strict proviso that no changes or edits are made and the original work is properly cited (including links to both the formal publication through the relevant DOI and the license). See: https://creativecommons.org/licenses/by-nc-nd/4.0/.
References
- Burkart KG, Brauer M, Aravkin AY, Godwin WW, Hay SI, He J, Iannucci VC, Larson SL, Lim SS, Liu J, Murray CJL, Zheng P, Zhou M, Stanaway JD. Estimating the cause-specific relative risks of non-optimal temperature on daily mortality: a two-part modelling approach applied to the Global Burden of Disease Study. Lancet 2021;398:685-97. [Crossref] [PubMed]
- Ma LY, Wang XD, Liu S, Gan J, Hu W, Chen Z, Han J, Du X, Zhu H, Shi Z, Ji Y. Prevalence and risk factors of stroke in a rural area of northern China: a 10-year comparative study. Aging Clin Exp Res 2022;34:1055-63. [Crossref] [PubMed]
- Zhang FL, Guo ZN, Wu YH, Liu HY, Luo Y, Sun MS, Xing YQ, Yang Y. Prevalence of stroke and associated risk factors: a population based cross sectional study from northeast China. BMJ Open 2017;7:e015758. [Crossref] [PubMed]
- Benjamin EJ, Muntner P, Alonso A, Bittencourt MS, Callaway CW, Carson AP, et al. Heart Disease and Stroke Statistics-2019 Update: A Report From the American Heart Association. Circulation 2019;139:e56-e528. [Crossref] [PubMed]
- Tsao CW, Aday AW, Almarzooq ZI, Alonso A, Beaton AZ, Bittencourt MS, et al. Heart Disease and Stroke Statistics-2022 Update: A Report From the American Heart Association. Circulation 2022;145:e153-639. [Crossref] [PubMed]
- Barrett KM, Brott TG. Stroke Caused by Extracranial Disease. Circ Res 2017;120:496-501. [Crossref] [PubMed]
- Global, regional, and national burden of stroke and its risk factors, 1990-2019: a systematic analysis for the Global Burden of Disease Study 2019. Lancet Neurol 2021;20:795-820. [Crossref] [PubMed]
- Goyal M, Singh N, Ospel J. Clinical trials in stroke in 2022: new answers and questions. Lancet Neurol 2023;22:9-10. [Crossref] [PubMed]
- Naylor R, Rantner B, Ancetti S, de Borst GJ, De Carlo M, Halliday A, et al. Editor's Choice - European Society for Vascular Surgery (ESVS) 2023 Clinical Practice Guidelines on the Management of Atherosclerotic Carotid and Vertebral Artery Disease. Eur J Vasc Endovasc Surg 2023;65:7-111. [Crossref] [PubMed]
- Gasior SA, O'Donnell JPM, Davey M, Clarke J, Jalali A, Ryan É, Aherne TM, Walsh SR. Optimal Management of Asymptomatic Carotid Artery Stenosis: A Systematic Review and Network Meta-Analysis. Eur J Vasc Endovasc Surg 2023;65:690-9. [Crossref] [PubMed]
- Aboyans V, Ricco JB, Bartelink MEL, Björck M, Brodmann M, Cohnert T, et al. 2017 ESC Guidelines on the Diagnosis and Treatment of Peripheral Arterial Diseases, in collaboration with the European Society for Vascular Surgery (ESVS): Document covering atherosclerotic disease of extracranial carotid and vertebral, mesenteric, renal, upper and lower extremity arteriesEndorsed by: the European Stroke Organization (ESO)The Task Force for the Diagnosis and Treatment of Peripheral Arterial Diseases of the European Society of Cardiology (ESC) and of the European Society for Vascular Surgery (ESVS). Eur Heart J 2018;39:763-816. [Crossref] [PubMed]
- Lohmann P, Franceschi E, Vollmuth P, Dhermain F, Weller M, Preusser M, Smits M, Galldiks N. Radiomics in neuro-oncological clinical trials. Lancet Digit Health 2022;4:e841-9. [Crossref] [PubMed]
- Schoepf UJ, Emrich T. A Brave New World: Toward Precision Phenotyping and Understanding of Coronary Artery Disease Using Radiomics Plaque Analysis. Radiology 2021;299:107-8. [Crossref] [PubMed]
- De Cecco CN, van Assen M. Can Radiomics Help in the Identification of Vulnerable Coronary Plaque? Radiology 2023;307:e223342. [Crossref] [PubMed]
- Fu F, Shan Y, Yang G, Zheng C, Zhang M, Rong D, Wang X, Lu J. Deep Learning for Head and Neck CT Angiography: Stenosis and Plaque Classification. Radiology 2023;307:e220996. [Crossref] [PubMed]
- Lin A, Manral N, McElhinney P, Killekar A, Matsumoto H, Kwiecinski J, et al. Deep learning-enabled coronary CT angiography for plaque and stenosis quantification and cardiac risk prediction: an international multicentre study. Lancet Digit Health 2022;4:e256-65. [Crossref] [PubMed]
- Chen Q, Pan T, Wang YN, Schoepf UJ, Bidwell SL, Qiao H, Feng Y, Xu C, Xu H, Xie G, Gao X, Tao XW, Lu M, Xu PP, Zhong J, Wei Y, Yin X, Zhang J, Zhang LJ. A Coronary CT Angiography Radiomics Model to Identify Vulnerable Plaque and Predict Cardiovascular Events. Radiology 2023;307:e221693. [Crossref] [PubMed]
- Wang Q, Li C, Zhang J, Hu X, Fan Y, Ma K, Sparrelid E, Brismar TB. Radiomics Models for Predicting Microvascular Invasion in Hepatocellular Carcinoma: A Systematic Review and Radiomics Quality Score Assessment. Cancers (Basel) 2021.
- Hou C, Liu XY, Du Y, Cheng LG, Liu LP, Nie F, Zhang W, He W. Radiomics in Carotid Plaque: A Systematic Review and Radiomics Quality Score Assessment. Ultrasound Med Biol 2023;49:2437-45. [Crossref] [PubMed]
- Yuan E, Chen Y, Song B. Quality of radiomics for predicting microvascular invasion in hepatocellular carcinoma: a systematic review. Eur Radiol 2023;33:3467-77. [Crossref] [PubMed]
- Park JE, Kim D, Kim HS, Park SY, Kim JY, Cho SJ, Shin JH, Kim JH. Quality of science and reporting of radiomics in oncologic studies: room for improvement according to radiomics quality score and TRIPOD statement. Eur Radiol 2020;30:523-36. [Crossref] [PubMed]
- Collins GS, Reitsma JB, Altman DG, Moons KG. Transparent reporting of a multivariable prediction model for individual prognosis or diagnosis (TRIPOD): the TRIPOD statement. The TRIPOD Group. Circulation 2015;131:211-9.
- Wolff RF, Moons KGM, Riley RD, Whiting PF, Westwood M, Collins GS, Reitsma JB, Kleijnen J, Mallett S. PROBAST: A Tool to Assess the Risk of Bias and Applicability of Prediction Model Studies. Ann Intern Med 2019;170:51-8. [Crossref] [PubMed]
- Lambin P, Leijenaar RTH, Deist TM, Peerlings J, de Jong EEC, van Timmeren J, Sanduleanu S, Larue RTHM, Even AJG, Jochems A, van Wijk Y, Woodruff H, van Soest J, Lustberg T, Roelofs E, van Elmpt W, Dekker A, Mottaghy FM, Wildberger JE, Walsh S. Radiomics: the bridge between medical imaging and personalized medicine. Nat Rev Clin Oncol 2017;14:749-62. [Crossref] [PubMed]
- Chen S, Liu C, Chen X, Liu WV, Ma L, Zha Y. A Radiomics Approach to Assess High Risk Carotid Plaques: A Non-invasive Imaging Biomarker, Retrospective Study. Front Neurol 2022;13:788652. [Crossref] [PubMed]
- Cheng X, Dong Z, Liu J, Li H, Zhou C, Zhang F, Wang C, Zhang Z, Lu G. Prediction of Carotid In-Stent Restenosis by Computed Tomography Angiography Carotid Plaque-Based Radiomics. J Clin Med 2022;11:3234. [Crossref] [PubMed]
- Cilla S, Macchia G, Lenkowicz J, Tran EH, Pierro A, Petrella L, Fanelli M, Sardu C, Re A, Boldrini L, Indovina L, De Filippo CM, Caradonna E, Deodato F, Massetti M, Valentini V, Modugno P. CT angiography-based radiomics as a tool for carotid plaque characterization: a pilot study. Radiol Med 2022;127:743-53. [Crossref] [PubMed]
- Colombi D, Bodini FC, Rossi B, Bossalini M, Risoli C, Morelli N, Petrini M, Sverzellati N, Michieletti E. Computed Tomography Texture Analysis of Carotid Plaque as Predictor of Unfavorable Outcome after Carotid Artery Stenting: A Preliminary Study. Diagnostics (Basel) 2021;11:2214. [Crossref] [PubMed]
- Dong Z, Zhou C, Li H, Shi J, Liu J, Liu Q, Su X, Zhang F, Cheng X, Lu G. Radiomics versus Conventional Assessment to Identify Symptomatic Participants at Carotid Computed Tomography Angiography. Cerebrovasc Dis 2022;51:647-54. [Crossref] [PubMed]
- Ebrahimian S, Homayounieh F, Singh R, Primak A, Kalra MK, Romero JM. Spectral segmentation and radiomic features predict carotid stenosis and ipsilateral ischemic burden from DECT angiography. Diagn Interv Radiol 2022;28:264-74. [Crossref] [PubMed]
- Huang XW, Zhang YL, Meng L, Qian M, Zhou W, Zheng RQ, Zheng HR, Niu LL. The relationship between HbA1c and ultrasound plaque textures in atherosclerotic patients. Cardiovasc Diabetol 2016;15:98. [Crossref] [PubMed]
- Huang Z, Cheng XQ, Liu HY, Bi XJ, Liu YN, Lv WZ, Xiong L, Deng YB. Relation of Carotid Plaque Features Detected with Ultrasonography-Based Radiomics to Clinical Symptoms. Transl Stroke Res 2022;13:970-82. [Crossref] [PubMed]
- Kafouris PP, Koutagiar IP, Georgakopoulos AT, Spyrou GM, Visvikis D, Anagnostopoulos CD. Fluorine-18 fluorodeoxyglucose positron emission tomography-based textural features for prediction of event prone carotid atherosclerotic plaques. J Nucl Cardiol 2021;28:1861-71. [Crossref] [PubMed]
- Le EPV, Rundo L, Tarkin JM, Evans NR, Chowdhury MM, Coughlin PA, Pavey H, Wall C, Zaccagna F, Gallagher FA, Huang Y, Sriranjan R, Le A, Weir-McCall JR, Roberts M, Gilbert FJ, Warburton EA, Schönlieb CB, Sala E, Rudd JHF. Assessing robustness of carotid artery CT angiography radiomics in the identification of culprit lesions in cerebrovascular events. Sci Rep 2021;11:3499. [Crossref] [PubMed]
- Lo CM, Hung PH. Computer-aided diagnosis of ischemic stroke using multi-dimensional image features in carotid color Doppler. Comput Biol Med 2022;147:105779. [Crossref] [PubMed]
- van Engelen A, Wannarong T, Parraga G, Niessen WJ, Fenster A, Spence JD, de Bruijne M. Three-dimensional carotid ultrasound plaque texture predicts vascular events. Stroke 2014;45:2695-701. [Crossref] [PubMed]
- Wang X, Luo P, Du H, Li S, Wang Y, Guo X, Wan L, Zhao B, Ren J. Ultrasound Radiomics Nomogram Integrating Three-Dimensional Features Based on Carotid Plaques to Evaluate Coronary Artery Disease. Diagnostics (Basel) 2022;12:256. [Crossref] [PubMed]
- Xia H, Yuan L, Zhao W, Zhang C, Zhao L, Hou J, Luan Y, Bi Y, Feng Y. Predicting transient ischemic attack risk in patients with mild carotid stenosis using machine learning and CT radiomics. Front Neurol 2023;14:1105616. [Crossref] [PubMed]
- Zaccagna F, Ganeshan B, Arca M, Rengo M, Napoli A, Rundo L, Groves AM, Laghi A, Carbone I, Menezes LJ. CT texture-based radiomics analysis of carotid arteries identifies vulnerable patients: a preliminary outcome study. Neuroradiology 2021;63:1043-52. [Crossref] [PubMed]
- Zhang L, Lyu Q, Ding Y, Hu C, Hui P. Texture Analysis Based on Vascular Ultrasound to Identify the Vulnerable Carotid Plaques. Front Neurosci 2022;16:885209. [Crossref] [PubMed]
- Zhang R, Zhang Q, Ji A, Lv P, Zhang J, Fu C, Lin J. Identification of high-risk carotid plaque with MRI-based radiomics and machine learning. Eur Radiol 2021;31:3116-26. [Crossref] [PubMed]
- Zhang S, Gao L, Kang B, Yu X, Zhang R, Wang X. Radiomics assessment of carotid intraplaque hemorrhage: detecting the vulnerable patients. Insights Imaging 2022;13:200. [Crossref] [PubMed]
- Zhang X, Hua Z, Chen R, Jiao Z, Shan J, Li C, Li Z. Identifying vulnerable plaques: A 3D carotid plaque radiomics model based on HRMRI. Front Neurol 2023;14:1050899. [Crossref] [PubMed]
- Chen Q, Zhang L, Mo X, You J, Chen L, Fang J, Wang F, Jin Z, Zhang B, Zhang S. Current status and quality of radiomic studies for predicting immunotherapy response and outcome in patients with non-small cell lung cancer: a systematic review and meta-analysis. Eur J Nucl Med Mol Imaging 2021;49:345-60. [Crossref] [PubMed]
- Sohn B, Won SY. Quality assessment of stroke radiomics studies: Promoting clinical application. Eur J Radiol 2023;161:110752. [Crossref] [PubMed]
- Spadarella G, Calareso G, Garanzini E, Ugga L, Cuocolo A, Cuocolo R. MRI based radiomics in nasopharyngeal cancer: Systematic review and perspectives using radiomic quality score (RQS) assessment. Eur J Radiol 2021;140:109744. [Crossref] [PubMed]
- Huang EP, O'Connor JPB, McShane LM, Giger ML, Lambin P, Kinahan PE, Siegel EL, Shankar LK. Criteria for the translation of radiomics into clinically useful tests. Nat Rev Clin Oncol 2023;20:69-82. [Crossref] [PubMed]
- Zhang X, Ruan S, Xiao W, Shao J, Tian W, Liu W, Zhang Z, Wan D, Huang J, Huang Q, Yang Y, Yang H, Ding Y, Liang W, Bai X, Liang T. Contrast-enhanced CT radiomics for preoperative evaluation of microvascular invasion in hepatocellular carcinoma: A two-center study. Clin Transl Med 2020;10:e111. [Crossref] [PubMed]
- Nebbia G, Zhang Q, Arefan D, Zhao X, Wu S. Pre-operative Microvascular Invasion Prediction Using Multi-parametric Liver MRI Radiomics. J Digit Imaging 2020;33:1376-86. [Crossref] [PubMed]
- Fournier L, Costaridou L, Bidaut L, Michoux N, Lecouvet FE, de Geus-Oei LF, et al. Incorporating radiomics into clinical trials: expert consensus endorsed by the European Society of Radiology on considerations for data-driven compared to biologically driven quantitative biomarkers. Eur Radiol 2021;31:6001-12. [Crossref] [PubMed]
- Sachs MC, McShane LM. Issues in developing multivariable molecular signatures for guiding clinical care decisions. J Biopharm Stat 2016;26:1098-110. [Crossref] [PubMed]
- Vergouwe Y, Steyerberg EW, Eijkemans MJ, Habbema JD. Substantial effective sample sizes were required for external validation studies of predictive logistic regression models. J Clin Epidemiol 2005;58:475-83. [Crossref] [PubMed]
- van Smeden M, de Groot JA, Moons KG, Collins GS, Altman DG, Eijkemans MJ, Reitsma JB. No rationale for 1 variable per 10 events criterion for binary logistic regression analysis. BMC Med Res Methodol 2016;16:163. [Crossref] [PubMed]
- Steyerberg EW, Eijkemans MJ, Harrell FE Jr, Habbema JD. Prognostic modeling with logistic regression analysis: in search of a sensible strategy in small data sets. Med Decis Making 2001;21:45-56. [Crossref] [PubMed]
- Vittinghoff E, McCulloch CE. Relaxing the rule of ten events per variable in logistic and Cox regression. Am J Epidemiol 2007;165:710-8. [Crossref] [PubMed]
- Nijman S, Leeuwenberg AM, Beekers I, Verkouter I, Jacobs J, Bots ML, Asselbergs FW, Moons K, Debray T. Missing data is poorly handled and reported in prediction model studies using machine learning: a literature review. J Clin Epidemiol 2022;142:218-29. [Crossref] [PubMed]
- Bouwmeester W, Zuithoff NP, Mallett S, Geerlings MI, Vergouwe Y, Steyerberg EW, Altman DG, Moons KG. Reporting and methods in clinical prediction research: a systematic review. PLoS Med 2012;9:1-12. [Crossref] [PubMed]
- Collins GS, Omar O, Shanyinde M, Yu LM. A systematic review finds prediction models for chronic kidney disease were poorly reported and often developed using inappropriate methods. J Clin Epidemiol 2013;66:268-77. [Crossref] [PubMed]
- Arnaud E, Elbattah M, Ammirati C, Dequen G, Ghazali DA. Predictive models in emergency medicine and their missing data strategies: a systematic review. NPJ Digit Med 2023;6:28. [Crossref] [PubMed]
- Moons KGM, Wolff RF, Riley RD, Whiting PF, Westwood M, Collins GS, Reitsma JB, Kleijnen J, Mallett S. PROBAST: A Tool to Assess Risk of Bias and Applicability of Prediction Model Studies: Explanation and Elaboration. Ann Intern Med 2019;170:W1-33. [Crossref] [PubMed]
- Moons KG, Altman DG, Reitsma JB, Ioannidis JP, Macaskill P, Steyerberg EW, Vickers AJ, Ransohoff DF, Collins GS. Transparent Reporting of a multivariable prediction model for Individual Prognosis or Diagnosis (TRIPOD): explanation and elaboration. Ann Intern Med 2015;162:W1-73. [Crossref] [PubMed]
- Whiting PF, Rutjes AW, Westwood ME, Mallett SQUADAS-2 Steering Group. A systematic review classifies sources of bias and variation in diagnostic test accuracy studies. J Clin Epidemiol 2013;66:1093-104. [Crossref] [PubMed]
- Moons KG, Royston P, Vergouwe Y, Grobbee DE, Altman DG. Prognosis and prognostic research: what, why, and how? BMJ 2009;338:b375. [Crossref] [PubMed]
- Wu B, Yang Z, Tobe RG, Wang Y. Medical therapy for preventing recurrent endometriosis after conservative surgery: a cost-effectiveness analysis. BJOG 2018;125:469-77. [Crossref] [PubMed]
- Wakabayashi T, Ouhmich F, Gonzalez-Cabrera C, Felli E, Saviano A, Agnus V, Savadjiev P, Baumert TF, Pessaux P, Marescaux J, Gallix B. Radiomics in hepatocellular carcinoma: a quantitative review. Hepatol Int 2019;13:546-59. [Crossref] [PubMed]
- Fornacon-Wood I, Mistry H, Ackermann CJ, Blackhall F, McPartlin A, Faivre-Finn C, Price GJ, O'Connor JPB. Reliability and prognostic value of radiomic features are highly dependent on choice of feature extraction platform. Eur Radiol 2020;30:6241-50. [Crossref] [PubMed]
- Collins GS, Dhiman P, Andaur Navarro CL, Ma J, Hooft L, Reitsma JB, Logullo P, Beam AL, Peng L, Van Calster B, van Smeden M, Riley RD, Moons KG. Protocol for development of a reporting guideline (TRIPOD-AI) and risk of bias tool (PROBAST-AI) for diagnostic and prognostic prediction model studies based on artificial intelligence. BMJ Open 2021;11:e048008. [Crossref] [PubMed]