Association of aberrant structural-functional network coupling with cognitive decline in patients with non-dialysis-dependent stage 5 chronic kidney disease
Introduction
A progressive loss of kidney function, increased urinary albumin excretion, or decreased glomerular filtration rate characterizes chronic kidney disease (CKD) (1). Currently, the global incidence of CKD is 8–16% and rising. Therefore, CKD has become a global public health challenge (2,3). Patients with CKD often experience altered cognitive function (4), including confusion, encephalopathy, and dementia, with the prevalence of cognitive impairment being 10–40% (5). These symptoms are particularly severe in those with stage 5 CKD, which is defined as a glomerular filtration rate <15 mL/min/1.73 m2 (6). Furthermore, after initiation of hemodialysis, hemodialysis-induced hemodynamic disturbances may lead to a significantly increased risk of a variety of adverse outcomes in patients with stage 5 CKD (7), including mortality within 1 year and various clinical neurological and psychological complications (such as stroke, rapid cognitive decline, and depression) (8-10). Therefore, imaging and biological markers related to cognitive impairment should be screened, and the potential neuropathological mechanisms in patients with non-dialysis-dependent stage 5 CKD (CKD 5ND) should be evaluated.
In recent years, with the rapid development of neuroimaging, resting-state functional magnetic resonance imaging (rs-fMRI), and diffusion tensor imaging (DTI) have been widely used to explore the pathophysiological mechanisms of cognitive dysfunction in patients with CKD treated with dialysis. The studies showed that patients with CKD treated with maintenance hemodialysis have abnormal spontaneous brain activity in multiple regions associated with the default mode network (11-14). Moreover, several DTI-based studies have demonstrated that patients with end-stage renal disease exhibit widespread decreases in fractional anisotropy (FA) and increases in radial diffusivity (RD) and mean diffusivity (MD) values in several white matter regions in the brain, with the changes in some indicators correlating with dialysis time and cognitive dysfunction, especially executive function (15-19). Furthermore, compared with healthy controls (HCs), patients with end-stage renal disease in brain network studies show altered graphical properties of structural and functional networks, such as small-world and nodal properties. A significant correlation has also been observed between these properties and cognitive function scale scores (20-23).
With the emergence of multimodal functional magnetic resonance, structural connectivity (SC) and functional connectivity (FC) coupling, an integrated measurement method combining functional and structural networks has been proposed. SC-FC coupling has been widely used to study the changes in SC-FC network relationships in various diseases, such as tinnitus, bipolar disorder, and subcortical stroke (24-27). For example, Wang et al. reported the deterioration of SC-FC coupling in patients with diabetic nephropathy (24). However, to the best of our knowledge, no study has explored the changes in SC-FC coupling in patients with CKD 5ND and a decreased level of cognition. Therefore, this study aimed to examine the altered properties of structural and functional networks in patients with CKD 5ND based on a graph-theoretic approach and assessed the following hypotheses: (I) there is structural and functional network disruption in patients with CKD 5ND; (II) SC-FC coupling is significantly altered in patients with CKD 5ND; and (III) the properties of the brain networks in patients with CKD 5ND correlate with the Montreal Cognitive Assessment (MoCA) score.
Methods
Participants and clinical data
A total of 51 patients with CKD 5ND from the Nephrology Department of Beijing Friendship Hospital, Capital Medical University, and 53 healthy volunteers from local communities were enrolled in this study. This study was conducted in accordance with the Declaration of Helsinki (as revised in 2013) and approved by the Medical Ethics Committee of Beijing Friendship Hospital, Capital Medical University. Written informed consent was obtained from each participant before enrollment in the study. All the participants were right-handed, and none of the HCs had a history of kidney disease. The exclusion criteria were as follows: (I) history of dialysis treatment; (II) history of psychiatric disorders; (III) neurological disorders, including stroke, brain tumor, and brain trauma; (IV) other systemic diseases; (V) contraindications to MRI; and (VI) significant head movement during MRI examination. In addition, 11 patients with CKD 5ND and 6 HCs were excluded due to claustrophobia (n=5), chronic infarction (n=2), and excessive head movement (≥3 mm/3°) (n=4). Finally, 40 patients with CKD 5ND and 47 HCs were included in the analysis. Details of the study population are shown in Figure 1. Cognitive assessments and biochemical blood examinations were performed for all patients with CKD 5ND before scanning. The MoCA was used to assess the cognitive level of the patients with CKD 5ND.
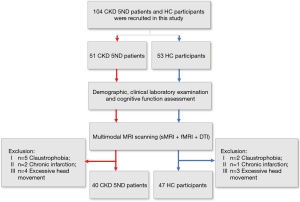
MRI data acquisition
All images were obtained on a 3.0 T magnetic resonance scanner (Discovery MR750w, GE HealthCare, Anaheim, USA) with an 8-channel phased front coil. During the scan, all participants were instructed to lie supine, keep their heads still, close their eyes, and stay awake but try to avoid thinking. We also provided earplugs to reduce noise and comfortable foam pads to reduce head movement. DTI was performed using a single-excitation spin echo plane imaging sequence under the following parameters: repetition time (TR) =8,000 ms, echo time (TE) =98 ms, matrix =128 × 128, acquisition voxel size 2×2×2 mm3, field of view (FOV) =224 mm × 224 mm, nonzero b value =1,000 s/mm2, gradient directions =64, slice thickness =2 mm, and bandwidth =2,500 Hz/Px. For high-resolution T1-weighted images, we used a 3D brain volume imaging (3D BRAVO) sequence under the following parameters: 96 slices with a slice thickness of 1 mm and no gap, TR =8.492 ms, TE =3.276 ms, inversion time (TI) =450 ms, flip angle (FA) =15°, acquisition voxel size 1×1×1 mm3, FOV =224 mm × 224 mm, and matrix =256 × 256. The resting-state functional data were obtained under the following parameters: 200 time points, 28 slices with a slice thickness of 5 mm (1-mm gap), TR=2000 ms, TE =35 ms, acquisition voxel size 1×1×1 mm3, FOV =224 mm × 224 mm, FA =90°, matrix =64×64, and acquisition time =368 s.
Data preprocessing
Preprocessing of DTI data
The DTI data were preprocessed using the PANDA software package (28) based on the FSL software package (https://fsl.fmrib.ox.ac.uk/fsl/fslwiki/). First, we converted the Digital Imaging and Communications in Medicine (DICOM) files to neuroimaging informatics technology initiative (NIfTI) images. We then used the b0 image to estimate the brain mask and cropped the raw image based on the obtained mask. Subsequently, we corrected the head movement and eddy distortion and performed whole-brain fiber tracking. Finally, we averaged multiple directions and calculated the diffusion tensor metrics. In this process, a deterministic fiber tracking method was adopted for whole-brain fiber tracking, and the criteria for terminating tracking were set as FA <0.15° or a deflection angle > 45° (29).
Preprocessing of fMRI data
The rs-fMRI data were preprocessed using the GRETNA package (https://www.nitrc.org/projects/gretna/) (30) installed in MATLAB 2018b (MathWorks). First, we converted the DICOM files to NIfTI images, removed the first 5 volumes of each time series, kept 195 volumes, and corrected slice-timing for the remaining 195 volumes. Next, we performed realignment and excluded participants whose maximum head movements were >3 mm or 3° at the horizontal and rotational levels. Diffeomorphic anatomical registration through exponentiated lie algebra (DARTEL) registration was used to spatially normalize the corrected images to the standard Montreal Neurological Institute template (resampled voxel size =3×3×3 mm3). Following this, detrending was implemented to remove the linear and nonlinear drift. Next, the white matter signal, Friston-24 head motion parameters, and cerebrospinal fluid signal were regressed off as covariates, and 0.01–0.08 Hz was applied for temporal filtering. Finally, we controlled the head movements again through scrubbing with the following parameters: frame-wise displacements (FD) threshold =0.5, previous time point number =1, and subsequent time point number =2.
Construction of brain networks
In this study, structural networks of all participants were constructed with DTI, and functional networks were acquired via rs-fMRI. Through use of the PANDA software package and the GRETNA package, respectively, the structural and functional networks were automatically divided into 246 anatomical regions of interest based on the Brainnetome Atlas, excluding the cerebellum (31). The FA was selected to construct the structural network, and the average FA of the connecting fibers between the weights of the edge nodes of the structural network was extracted.
Regarding the functional network, the average time series of each node was acquired by averaging the fMRI time courses on each node. We then used the correlation coefficients between each node as the weights of the edges in the functional network (Figure 2).
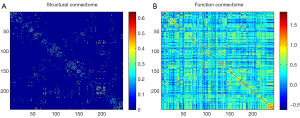
Graph theory analysis
The GRETNA software package (https://www.nitrc.org/projects/gretna/) (30) was applied to calculate the properties of the structural and functional networks. A threshold of connection sparsity (0.05–0.5, step size: 0.01) was applied to the functional network, and the values of the matrix elements (threshold set to 0) were applied to the structural network. We characterized the properties of the structural and functional networks with the following parameters: characteristic path length (Lp), clustering coefficient (Cp), normalized clustering coefficient (Gamma), normalized characteristic path length (Lambda), small-worldness (Sigma), global efficiency (Eglob), and local efficiency (Eloc). For functional network intergroup comparisons, we further calculated the area under the curve (AUC) for the sparsity range, which provided a generalized scale for the brain network features independent of a single threshold selection.
SC-FC coupling analysis
The method used for SC-FC coupling analysis in this study was consistent with that used in previous studies (20,22,32,33). The nonzero network edges of the SC matrix were extracted to produce a vector of structural connectivity values and to return them to a Gaussian distribution. Meanwhile, the corresponding FC matrix network edges were extracted to form a vector of FC values. Finally, the Pearson correlation coefficient between the two vectors was calculated, which were the network coupling values.
Statistical analysis
Patients’ demographic characteristics, clinical features, and MoCA scores were statistically analyzed using SPSS25.0 software (IBM Corp.). The chi-squared test was used for qualitative variables. The Kolmogorov-Smirnov test confirmed that the small-world attributes and network efficiency values were normally distributed. The statistical significance threshold was set at P<0.05. The chi-squared test was used to compare sex between the two groups. Group differences in small-world attributes, network efficiency, and SC-FC coupling were evaluated by 2-sample t-tests with age as the covariate. Pearson correlation analysis detected correlations between small-world attributes, network efficiency, and MoCA scores with age and estimated glomerular filtration rate (eGFR) as covariates. For multiple comparisons, tests were corrected using the Bonferroni method (P<0.05/7=0.007).
Results
Demographic and clinical characteristics
The demographic and clinical characteristics of the patients with CKD 5ND and HCs are shown in Table 1. No statistical differences were observed between the patients with CKD 5ND and the HCs regarding sex, age, or education. The biochemical blood test results of the patients with CKD 5ND are shown in Table 1.
Table 1
Characteristic | Patients with CKD 5ND (n=40) | HCs (n=47) | P value |
---|---|---|---|
Age (years) | 51.28±12.7 [20–73] | 46.23±11.7 [23–63] | 0.056a |
Education | 12.4±3.7 | 13.0±4.5 | 0.058a |
Sex (male/female) | 27/13 | 27/20 | 0.336b |
Albumin (g/L) | 35.97±5.00 | NA | NA |
Urea (mmol/L) | 30.19±8.21 | NA | NA |
Creatinine (μmol/L) | 760.8±241.6 | NA | NA |
Phosphate (mmol/L) | 2.0±0.50 | NA | NA |
Ca (mmol/L) | 2.08±0.23 | NA | NA |
Parathyroid hormone (pg/mL) | 266.4±266.7 | NA | NA |
Hemoglobin (g/L) | 96.4±16.1 | NA | NA |
Ferritin (ng/mL) | 131.7±193.6 | NA | NA |
Urinary albumin (g/24 h) | 2.4±2.1 | NA | NA |
eGFR (mL/min) | 6.8±3.3 | NA | NA |
Serum iron (μmol/L) | 12.4±7.8 | NA | NA |
MoCA scores | 24.1±3.5 [14–30] | NA | NA |
Data are presented as the mean ± standard deviation [range]. a, two-sample t test. b, chi-squared test. CKD 5ND, non-dialysis-dependent stage 5 chronic kidney disease; HC, healthy control; Ca, calcium; NA, not applicable; eGFR, estimated glomerular filtration rate; MoCA, Montreal Cognitive Assessment.
Structural and functional network analysis
Alterations in small-world properties and network efficiency
Within the set threshold range, all participants showed small-world attributes (Sigma >1, Gamma >1, Lambda ≈1) in the structural and functional networks (Figure 3). In the structural network, patients with CKD 5ND displayed significantly lower Sigma (P=0.009) and Cp (P=0.01) than did the HCs. In contrast, the Lp (P<0.001) and Lambda (P<0.001) values of patients with CKD 5ND were significantly higher than those of the HCs. No significant difference was observed in the Gamma values between the two groups. Regarding network efficiency, Eglob (P<0.001) and Eloc (P=0.01) were lower in patients with CKD 5ND than in HCs (Figure 4 and Table 2).
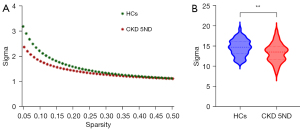
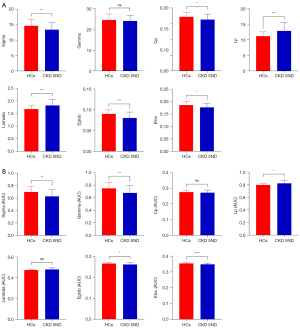
Table 2
Graph measure | Patients with CKD 5ND (n=40) | HCs (n=47) | t value | P value |
---|---|---|---|---|
Structural network | ||||
Sigma | 13.38±2.30 | 14.62±2.00 | 2.66 | 0.009 |
Gamma | 23.94±2.56 | 24.36±2.91 | 0.75 | 0.48 |
Cp | 0.16±0.02 | 0.17±0.02 | 2.65 | 0.01 |
Lp | 12.83±2.67 | 11.03±1.42 | 4.01 | <0.001 |
Lambda | 1.82±0.23 | 1.67±0.14 | 3.62 | <0.001 |
Eglob | 0.08±0.01 | 0.09±0.01 | 4.21 | <0.001 |
Eloc | 0.18±0.02 | 0.19±0.01 | 2.67 | 0.01 |
Functional network (AUC) | ||||
Sigma | 0.63±0.10 | 0.70±0.09 | 3.59 | 0.008 |
Gamma | 0.68±0.11 | 0.75±0.10 | 3.38 | 0.009 |
Cp | 0.27±0.02 | 0.27±0.01 | 1.18 | 0.24 |
Lp | 0.82±0.05 | 0.80±0.02 | 2.91 | 0.02 |
Lambda | 0.48±0.02 | 0.47±0.00 | 2.36 | 0.17 |
Eglob | 0.26±0.01 | 0.27±0.01 | 2.54 | 0.04 |
Eloc | 0.35±0.01 | 0.35±0.00 | 4.35 | <0.0001 |
Coupling | −0.16±0.08 | −0.22±0.06 | 3.71 | <0.001 |
Graphical measurement of structural and functional network. Data are presented as the mean ± standard deviation. Covariance analysis adjusted for age was conducted for the intergroup comparisons. CKD 5ND, non-dialysis-dependent stage 5 chronic kidney disease; HC, healthy control; Sigma, small-worldness; Gamma, normalized clustering coefficient; Cp, clustering coefficient; Lp, characteristic path length; Lambda, normalized characteristic path length; Eglob, global efficiency; Eloc, local efficiency. AUC, area under the curve.
Regarding the functional network, patients with CKD 5ND showed significantly lower Sigma (P=0.008) and Gamma (P=0.009) values than did the HCs. In contrast, the Lp values (P=0.02) of patients with CKD 5ND were significantly higher than those of the HCs. However, no significant difference was observed in the Cp and Lambda values between the two groups. Regarding network efficiency, Eglob (P=0.04) and Eloc (P<0.0001) were lower in patients with CKD 5ND than in HCs (Figure 4 and Table 2).
SC-FC coupling analysis
Patients with CKD 5ND (−0.16±0.08) demonstrated significantly higher SC-FC coupling values than did HCs (−0.22±0.06; P<0.001) (Figure 5 and Table 2).
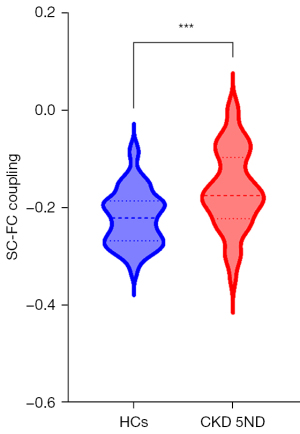
Relationships between graphical properties and MoCA scores
Regarding the structural network, in patients with CKD 5ND, we found significant positive correlations between Cp and MoCA scores (r=0.374; P=0.003), Eglob and MoCA scores (r=0.452; P=0.004), and Eloc and MoCA scores (r=0.455; P=0.004). However, a significant negative correlation was observed between Lp and MoCA scores (r=−0.452; P=0.004), and Lambda and MoCA scores (r=−0.437; P=0.004). No significant correlation was observed between Gamma and MoCA scores (r=−0.016; P=0.92) or between Sigma and MoCA scores (r=0.244; P=0.12) (Figure 6).
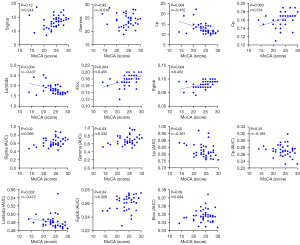
Regarding the functional network, Sigma and MoCA scores (r=0.366; P=0.02), Gamma and MoCA scores (r=0.333; P=0.03), and Eglob and MoCA scores (r=0.326; P=0.04) exhibited positive correlations; meanwhile, Lp and MoCA scores (r=−0.361; P=0.02) and Lambda and MoCA scores (r=−0.472; P=0.002) exhibited negative correlations.
Regarding the SC-FC coupling, no significant correlation was observed between SC-FC coupling and MoCA scores (r=−0.070; P=0.67).
Relationships between functional graphical properties and structural graphical properties
In patients with CKD 5ND, no significant correlation was observed between Sigma AUC and Sigma (r=−0.096; P=0.55), Lambda AUC and Lambda (r=0.014; P=0.93), Cp AUC and Cp (r=0.130; P=0.42), Lp AUC and Lp (r=−0.005, P=0.97), Gamma AUC and Gamma (r=−0.013; P=0.93), Eglob AUC and Eglob (r=0.014; P=0.92), or between Eloc AUC and Eloc (r=0.152; P=0.35) (Figure S1).
Discussion
This study examined the brain network characteristics of patients with CKD 5ND using multimodal MRI techniques. We found that patients with CKD 5ND showed disruptions in functional and structural network features compared with HCs. These network features were correlated with the patients’ MoCA scores. In addition, the SC-FC coupling of patients with CKD 5ND was significantly different from that of the HCs. This is the first study to explore the underlying mechanisms of cognitive impairment in patients with CKD 5ND from a functional-structural perspective through a combination of DTI and rs-fMRI. Our results may provide new insights into the role of brain SC-FC network coupling in the generation of cognitive impairment in CKD 5ND and may aid in discovering indicators for the early diagnosis and treatment of cognitive impairment in patients with CKD 5ND.
Changes in graphical properties of structural and functional networks
Consistent with graph theory findings from previous studies related to CKD, patients with CKD 5ND and HCs exhibited small-world properties of structural and functional networks (20,22,32). The small-world network is ideal, as it provides a structural basis for local and global interactions and allows for effective information separation and integration (34,35). However, in this study, we found some differences in the properties of the structural networks of the two groups. For example, we discovered that Sigma and Cp were lower while Lp was higher in the patients with CKD 5ND than in the HCs. CP and Lp are important brain network parameters, and the human brain is a small-world network with high Cp and low Lp (36-38).
Moreover, Lp reflects the brain’s capacity for information integration and transmission (39). Therefore, the reduced Sigma observed in our study indicated that CKD 5ND can lead to global changes in structural brain networks, and the increased Lp indicated a reduced ability to integrate and rapidly transmit information between distant brain regions effectively (34). In addition, Eloc and Eglob were lower in patients with CKD 5ND than in HCs. Eglob, defined as the inverse of Lp, is the best measure of information integration. The lower Eglob of the patients with CKD 5ND indicated that information integration efficiency and information transmission ability were decreased in these patients. Eloc mainly mediates the modular information transfer and fault tolerance of the network (40). Therefore, the low Eloc represented a shorter range of SC and reduced local information processing capacity in patients with CKD 5ND.
Regarding the functional network properties, patients with CKD 5ND showed higher Lp and lower Sigma, Eloc, and Eglob values than HCs. We also found that patients with CKD 5ND exhibited lower Gamma values in the functional brain network than did the HCs; however, no difference in Cp was observed between the two groups. Cp represents the transition process of the network from a small-world network to a regular or random network, while Gamma is the normalized form of Cp. These results suggest that patients with CKD 5ND have reduced global and local information processing capacity of the functional brain networks.
Regarding SC-FC coupling, previous studies have shown that SC and FC interact with one another. SC provides scaffolding for FC, and lower levels of local properties of the structural network may constrain and predict the strength and spatial patterns of FC, while FC shapes SC through plasticity mechanisms (41-43). Moreover, the SC-FC coupling strength is significantly altered in neuropsychiatric disorders that may reflect the psychological state of an individual (44). Reduced SC-FC coupling strength may suggest a loss of consistency in SC and FC (26). Moreover, disordered SC-FC coupling has been reported in various diseases, such as tinnitus, stroke, bipolar disorder, epilepsy, and Alzheimer disease, and some studies have reported an association between SC-FC coupling strength and clinical symptoms (26,27,29,45). Consistent with previous studies, we found that the SC-FC coupling strength was significantly higher in patients with CKD 5ND than in HCs. Chen et al. (27) showed that increased SC-FC coupling in patients with tinnitus might indicate that these patients experience a more abnormal state of overcompensation through increased structural and functional brain connectivity. We hypothesize that both functional and structural brain connections are impaired in patients with CKD 5ND, resulting in a compensatory enhancement of SC-FC coupling. Furthermore, we found that the mean coupling values were negative for both groups, which we speculate may be because SC-FC coupling is the strongest in visual and subcortical regions and the weakest in limbic and default network regions (46). Therefore, the SC-FC coupling showed negative values at the whole-brain level.
Relationships between graphical properties and MoCA scores
Notably, several studies have investigated the relationship between changes in functional and structural brain characteristics and clinical scores in patients with stage 5 CKD (12,47,48). Zheng et al. found microstructural and functional brain changes in patients with stage 5 CKD on dialysis, with significant correlations between these changes and MoCA scores (47). Similarly, Gu et al. observed atrophy in the subcortical structures of patients with stage 5 CKD on dialysis, which correlated significantly with clinical presentation (48). Consistent with previous research, we found that structural network parameters (Cp, Eloc, Eglob) and functional network parameters (Sigma, Gamma, Eglob) in patients with CKD 5ND were positively correlated with MoCA; however, Lp was negatively correlated with MoCA. These findings suggest that the whole brain has a reduced ability to integrate and transmit information globally and locally and may mediate cognitive impairment in patients with CKD 5ND; however, the underlying neural mechanisms require further investigation.
Limitations
Our study has several limitations. First, as this was a cross-sectional study of patients with CKD 5ND, in the future we will conduct separate studies of patients with early-stage CKD (CKD1-4) with follow-up to further analyze the differences in cognitive deficits in patients with CKD at different disease stages. Furthermore, the present investigation focused on whole-brain level SC-FC coupling, and additional inter- and intranetwork SC-FC coupling studies in patients with CKD 5ND are warranted, which we aim to conduct in future. Moreover, this study was based on the Brainnetome Atlas and excluded the cerebellum; in future studies, we will select nodes based on different brain anatomy or functional templates and use other atlases containing the cerebellum to study the possible role of the cerebellum in diseases. Finally, this study explored whole-brain SC-FC coupling in patients with CKD 5ND based on rs-fMRI and DTI. Recently, a study combining arterial spin labeling (ASL) and quantitative susceptibility mapping (QSM) found that cerebral blow flow-susceptibility coupling was reduced in patients with CKD and related to cognition (49). In the future, we will integrate additional modalities, such as rs-fMRI, ASL, and QSM, to explore the potential neural mechanisms of cognitive reduction in patients with CKD.
Conclusions
Patients with CKD 5ND in this study exhibited altered graph-theoretical properties of both the structural and functional brain networks. In addition, these changes in structural network properties were closely related to mild cognitive impairment. Furthermore, SC-FC coupling values were increased in patients with CKD 5ND compared with those in HCs. Our findings may contribute to a better understanding of the anatomical-functional interaction mechanisms underlying the cognitive impairment in patients with CKD 5ND. SC-FC coupling and MoCA scores were not correlated; however, whole-brain SC-FC coupling may provide new insights into the search for potential imaging markers to assess cognitive decline in CKD 5ND, and thus the exact biological mechanisms responsible for the altered structure-function coupling in CKD 5ND need to be further investigated.
Acknowledgments
Funding: This work was supported by
Footnote
Conflicts of Interest: All authors have completed the ICMJE uniform disclosure form (available at https://qims.amegroups.com/article/view/10.21037/qims-23-295/coif). B.X. is a current employee of MR Research, GE HealthCare, Beijing, China. The other authors have no conflicts of interest to declare.
Ethical Statement: The authors are accountable for all aspects of the work in ensuring that questions related to the accuracy or integrity of any part of the work are appropriately investigated and resolved. This study was conducted in accordance with the Declaration of Helsinki (as revised in 2013) and was approved by the Medical Ethics Committee of Beijing Friendship Hospital, Capital Medical University. Written informed consent was obtained from each participant before enrollment in the study.
Open Access Statement: This is an Open Access article distributed in accordance with the Creative Commons Attribution-NonCommercial-NoDerivs 4.0 International License (CC BY-NC-ND 4.0), which permits the non-commercial replication and distribution of the article with the strict proviso that no changes or edits are made and the original work is properly cited (including links to both the formal publication through the relevant DOI and the license). See: https://creativecommons.org/licenses/by-nc-nd/4.0/.
References
- Miglinas M, Cesniene U, Janusaite MM, Vinikovas A. Cerebrovascular Disease and Cognition in Chronic Kidney Disease Patients. Front Cardiovasc Med 2020;7:96. [Crossref] [PubMed]
- Jha V, Garcia-Garcia G, Iseki K, Li Z, Naicker S, Plattner B, Saran R, Wang AY, Yang CW. Chronic kidney disease: global dimension and perspectives. Lancet 2013;382:260-72. [Crossref] [PubMed]
- Yang C, Gao B, Zhao X, Su Z, Sun X, Wang HY, et al. Executive summary for China Kidney Disease Network (CK-NET) 2016 Annual Data Report. Kidney Int 2020;98:1419-23. [Crossref] [PubMed]
- Lau WL, Fisher M. New insights into cognitive decline in chronic kidney disease. Nat Rev Nephrol 2023;19:214-5. [Crossref] [PubMed]
- Drew DA, Weiner DE, Sarnak MJ. Cognitive Impairment in CKD: Pathophysiology, Management, and Prevention. Am J Kidney Dis 2019;74:782-90. [Crossref] [PubMed]
- Kurella Tamura M, Yaffe K. Dementia and cognitive impairment in ESRD: diagnostic and therapeutic strategies. Kidney Int 2011;79:14-22. [Crossref] [PubMed]
- Wolfgram DF. Intradialytic Cerebral Hypoperfusion as Mechanism for Cognitive Impairment in Patients on Hemodialysis. J Am Soc Nephrol 2019;30:2052-8. [Crossref] [PubMed]
- Kalirao P, Pederson S, Foley RN, Kolste A, Tupper D, Zaun D, Buot V, Murray AM. Cognitive impairment in peritoneal dialysis patients. Am J Kidney Dis 2011;57:612-20. [Crossref] [PubMed]
- Bugnicourt JM, Godefroy O, Chillon JM, Choukroun G, Massy ZA. Cognitive disorders and dementia in CKD: the neglected kidney-brain axis. J Am Soc Nephrol 2013;24:353-63. [Crossref] [PubMed]
- Natale P, Palmer SC, Ruospo M, Saglimbene VM, Rabindranath KS, Strippoli GF. Psychosocial interventions for preventing and treating depression in dialysis patients. Cochrane Database Syst Rev 2019;12:CD004542. [Crossref] [PubMed]
- Chen HJ, Qiu J, Fu Q, Chen F. Alterations of Spontaneous Brain Activity in Hemodialysis Patients. Front Hum Neurosci 2020;14:278. [Crossref] [PubMed]
- Gu Z, Lu H, Zhou H, Zhang J, Xing W. The relationship between abnormal cortical activity in the anterior cingulate gyrus and cognitive dysfunction in patients with end- stage renal disease: a fMRI study on the amplitude of low- frequency fluctuations. Ann Palliat Med 2020;9:4187-93. [Crossref] [PubMed]
- Su H, Fu S, Liu M, Yin Y, Hua K, Meng S, Jiang G, Quan X. Altered Spontaneous Brain Activity and Functional Integration in Hemodialysis Patients With End-Stage Renal Disease. Front Neurol 2021;12:801336. [Crossref] [PubMed]
- Chen HJ, Qi R, Kong X, Wen J, Liang X, Zhang Z, Li X, Lu GM, Zhang LJ. The impact of hemodialysis on cognitive dysfunction in patients with end-stage renal disease: a resting-state functional MRI study. Metab Brain Dis 2015;30:1247-56. [Crossref] [PubMed]
- Mu J, Ma L, Ma S, Ding D, Li P, Ma X, Zhang M, Liu J. Neurological effects of hemodialysis on white matter microstructure in end-stage renal disease. Neuroimage Clin 2021;31:102743. [Crossref] [PubMed]
- Zhang R, Liu K, Yang L, Zhou T, Qian S, Li B, Peng Z, Li M, Sang S, Jiang Q, Sun G. Reduced white matter integrity and cognitive deficits in maintenance hemodialysis ESRD patients: a diffusion-tensor study. Eur Radiol 2015;25:661-8. [Crossref] [PubMed]
- Guo H, Liu W, Li H, Yang J. Structural and Functional Brain Changes in Hemodialysis Patients with End-Stage Renal Disease: DTI Analysis Results and ALFF Analysis Results. Int J Nephrol Renovasc Dis 2021;14:77-86. [Crossref] [PubMed]
- Chou MC, Hsieh TJ, Lin YL, Hsieh YT, Li WZ, Chang JM, Ko CH, Kao EF, Jaw TS, Liu GC. Widespread white matter alterations in patients with end-stage renal disease: a voxelwise diffusion tensor imaging study. AJNR Am J Neuroradiol 2013;34:1945-51. [Crossref] [PubMed]
- Zhang C, Yu H, Cai Y, Wu N, Liang S, Zhang C, Duan Z, Zhang Z, Cai G. Diffusion tensor imaging of the brain white matter microstructure in patients with chronic kidney disease and its correlation with cognition. Front Neurol 2022;13:1086772. [Crossref] [PubMed]
- Jin M, Wang L, Wang H, Han X, Diao Z, Guo W, Yang Z, Ding H, Wang Z, Zhang P, Zhao P, Lv H, Liu W, Wang Z. Altered resting-state functional networks in patients with hemodialysis: a graph-theoretical based study. Brain Imaging Behav 2021;15:833-45. [Crossref] [PubMed]
- Zhang D, Chen Y, Wu H, Lin L, Xie Q, Chen C, Jing L, Wu J. Associations of the Disrupted Functional Brain Network and Cognitive Function in End-Stage Renal Disease Patients on Maintenance Hemodialysis: A Graph Theory-Based Study of Resting-State Functional Magnetic Resonance Imaging. Front Hum Neurosci 2021;15:716719. [Crossref] [PubMed]
- Ma S, Zhang M, Liu Y, Ding D, Li P, Ma X, Liu H, Mu J. Abnormal rich club organization in end-stage renal disease patients before dialysis initiation and undergoing maintenance hemodialysis. BMC Nephrol 2020;21:515. [Crossref] [PubMed]
- Chou MC, Ko CH, Chang JM, Hsieh TJ. Disruptions of brain structural network in end-stage renal disease patients with long-term hemodialysis and normal-appearing brain tissues. J Neuroradiol 2019;46:256-62. [Crossref] [PubMed]
- Wang YF, Gu P, Zhang J, Qi R, de Veer M, Zheng G, Xu Q, Liu Y, Lu GM, Zhang LJ. Deteriorated functional and structural brain networks and normally appearing functional-structural coupling in diabetic kidney disease: a graph theory-based magnetic resonance imaging study. Eur Radiol 2019;29:5577-89. [Crossref] [PubMed]
- Ma J, Liu F, Yang B, Xue K, Wang P, Zhou J, Wang Y, Niu Y, Zhang J. Selective Aberrant Functional-Structural Coupling of Multiscale Brain Networks in Subcortical Vascular Mild Cognitive Impairment. Neurosci Bull 2021;37:287-97. [Crossref] [PubMed]
- Zhang R, Shao R, Xu G, Lu W, Zheng W, Miao Q, Chen K, Gao Y, Bi Y, Guan L, McIntyre RS, Deng Y, Huang X, So KF, Lin K. Aberrant brain structural-functional connectivity coupling in euthymic bipolar disorder. Hum Brain Mapp 2019;40:3452-63. [Crossref] [PubMed]
- Chen Q, Lv H, Wang Z, Wei X, Liu J, Liu F, Zhao P, Yang Z, Gong S, Wang Z. Distinct brain structural-functional network topological coupling explains different outcomes in tinnitus patients treated with sound therapy. Hum Brain Mapp 2022;43:3245-56. [Crossref] [PubMed]
- Cui Z, Zhong S, Xu P, He Y, Gong G. PANDA: a pipeline toolbox for analyzing brain diffusion images. Front Hum Neurosci 2013;7:42. [Crossref] [PubMed]
- Chen H, Geng W, Shang S, Shi M, Zhou L, Jiang L, Wang P, Yin X, Chen YC. Alterations of brain network topology and structural connectivity-functional connectivity coupling in capsular versus pontine stroke. Eur J Neurol 2021;28:1967-76. [Crossref] [PubMed]
- Wang J, Wang X, Xia M, Liao X, Evans A, He Y. GRETNA: a graph theoretical network analysis toolbox for imaging connectomics. Front Hum Neurosci 2015;9:386. [PubMed]
- Fan L, Li H, Zhuo J, Zhang Y, Wang J, Chen L, Yang Z, Chu C, Xie S, Laird AR, Fox PT, Eickhoff SB, Yu C, Jiang T. The Human Brainnetome Atlas: A New Brain Atlas Based on Connectional Architecture. Cereb Cortex 2016;26:3508-26. [Crossref] [PubMed]
- Park BS, Seong M, Ko J, Park SH, Kim YW, Hwan Kim I, Park JH, Lee YJ, Park S, Park KM. Differences of connectivity between ESRD patients with PD and HD. Brain Behav 2020;10:e01708. [Crossref] [PubMed]
- Zhang Z, Liao W, Chen H, Mantini D, Ding JR, Xu Q, Wang Z, Yuan C, Chen G, Jiao Q, Lu G. Altered functional-structural coupling of large-scale brain networks in idiopathic generalized epilepsy. Brain 2011;134:2912-28. [Crossref] [PubMed]
- Bullmore E, Sporns O. Complex brain networks: graph theoretical analysis of structural and functional systems. Nat Rev Neurosci 2009;10:186-98. [Crossref] [PubMed]
- Rubinov M, Sporns O. Complex network measures of brain connectivity: uses and interpretations. Neuroimage 2010;52:1059-69. [Crossref] [PubMed]
- Bassett DS, Bullmore E. Small-world brain networks. Neuroscientist 2006;12:512-23. [Crossref] [PubMed]
- Sporns O. The human connectome: a complex network. Ann N Y Acad Sci 2011;1224:109-25. [Crossref] [PubMed]
- Sporns O, Honey CJ. Small worlds inside big brains. Proc Natl Acad Sci U S A 2006;103:19219-20. [Crossref] [PubMed]
- Watts DJ, Strogatz SH. Collective dynamics of 'small-world' networks. Nature 1998;393:440-2. [Crossref] [PubMed]
- Latora V, Marchiori M. Efficient behavior of small-world networks. Phys Rev Lett 2001;87:198701. [Crossref] [PubMed]
- Huang H, Ding M. Linking Functional Connectivity and Structural Connectivity Quantitatively: A Comparison of Methods. Brain Connect 2016;6:99-108. [Crossref] [PubMed]
- Honey CJ, Sporns O, Cammoun L, Gigandet X, Thiran JP, Meuli R, Hagmann P. Predicting human resting-state functional connectivity from structural connectivity. Proc Natl Acad Sci U S A 2009;106:2035-40. [Crossref] [PubMed]
- Dacosta-Aguayo R, Graña M, Savio A, Fernández-Andújar M, Millán M, López-Cancio E, Cáceres C, Bargalló N, Garrido C, Barrios M, Clemente IC, Hernández M, Munuera J, Dávalos A, Auer T, Mataró M. Prognostic value of changes in resting-state functional connectivity patterns in cognitive recovery after stroke: A 3T fMRI pilot study. Hum Brain Mapp 2014;35:3819-31. [Crossref] [PubMed]
- Wang Z, Dai Z, Gong G, Zhou C, He Y. Understanding structural-functional relationships in the human brain: a large-scale network perspective. Neuroscientist 2015;21:290-305. [Crossref] [PubMed]
- Cao R, Wang X, Gao Y, Li T, Zhang H, Hussain W, Xie Y, Wang J, Wang B, Xiang J. Abnormal Anatomical Rich-Club Organization and Structural-Functional Coupling in Mild Cognitive Impairment and Alzheimer's Disease. Front Neurol 2020;11:53. [Crossref] [PubMed]
- Gu Z, Jamison KW, Sabuncu MR, Kuceyeski A. Heritability and interindividual variability of regional structure-function coupling. Nat Commun 2021;12:4894. [Crossref] [PubMed]
- Zheng J, Sun Q, Wu X, Dou W, Pan J, Jiao Z, Liu T, Shi H. Brain Micro-Structural and Functional Alterations for Cognitive Function Prediction in the End-Stage Renal Disease Patients Undergoing Maintenance Hemodialysis. Acad Radiol 2023;30:1047-55. [Crossref] [PubMed]
- Gu W, He R, Su H, Ren Z, Zhang L, Yuan H, Zhang M, Ma S. Changes in the Shape and Volume of Subcortical Structures in Patients With End-Stage Renal Disease. Front Hum Neurosci 2021;15:778807. [Crossref] [PubMed]
- Wang H, Liu X, Song L, Yang W, Li M, Chen Q, Lv H, Zhao P, Yang Z, Liu W, Wang ZC. Dysfunctional Coupling of Cerebral Blood Flow and Susceptibility Value in the Bilateral Hippocampus is Associated with Cognitive Decline in Nondialysis Patients with CKD. J Am Soc Nephrol 2023;34:1574-88. [Crossref] [PubMed]