CT radiomics in the identification of preoperative understaging in patients with clinical stage T1–2N0 esophageal squamous cell carcinoma
Introduction
Esophageal cancer is one of the most common cancers in the digestive tract. It is the seventh most common cancer and the sixth leading cause of cancer-related deaths worldwide (1). Despite great advances in treatment, esophageal cancer still has a poor prognosis, with a 5-year survival rate of less than 25% (2). The importance of preoperative neoadjuvant therapy followed by radical surgical resection has been emphasized in clinical guidelines in recent years (3). It is recommended as the first-line treatment strategy for patients with clinical stage T2–T4aN0/TanyN1–3 esophageal cancer. The benefits of neoadjuvant therapy in clinical stage T3N0 and clinical stage TanyN1–3 patients have been recognized (4,5). Neoadjuvant therapy improves disease-free survival (DFS) and overall survival in these patients (4,5). However, there are still concerns regarding clinical stage T1–2N0 (cT1–2N0) esophageal cancers.
The inaccuracy of clinical staging affects the overall survival of esophageal cancer patients (6). In one study, the pathological findings revealed that 37–54% of the patients with pT1b esophageal cancer had lymph node metastasis (7); however, 33% of these lymph node metastases patients were misdiagnosed as cN0 based on preoperative evaluations. Moreover, the treatment options for clinical stage T2N0 (cT2N0) esophageal cancer remain controversial (6). Several studies have suggested that as cT2N0 is considered an early-stage cancer, radical surgical resection without neoadjuvant therapy is a sufficient treatment (4,8,9). However, other studies have reported that as T2 esophageal cancer infiltrates the submucosa, such patients are at an increased risk of nodal metastasis, which cannot be accurately diagnosed according to current imaging diagnostic criteria (10,11). Thus, some clinicians suggest that a multimodality approach of neoadjuvant therapy followed by surgery is appropriate for cT2N0 patients.
The inaccuracy of clinical staging is the main reason for the controversy over which strategies should be adopted to treat cT2N0 esophageal cancer (12-16). Studies have noted the preoperative understaging of cT1–2N0 patients may be the reason why neoadjuvant therapy has improved the survival of these patients (16-18). Indeed, most cT1–2N0 esophageal cancers are pathologically staged as T3N0 or N1–3 based on postoperative pathological findings (4,5,8-10,12-18). “Preoperative understaging” was defined as a patient with a pathologic stage of T3–4N0 (pT3–4N0) or pTanyN1–3 being misdiagnosed with cT2N0 before surgery. Thus, recognition of understaging in cT1–T2N0 patients is key if clinicians are to select more appropriate therapeutic strategies.
Endoscopic ultrasonography (EUS) is the standard imaging approach for the clinical evaluation of primary tumors in patients with esophageal cancer. However, the accuracy of EUS staging is operator dependent. Additionally, EUS cannot be used for patients with stenotic tumors. Chest computed tomography (CT), which is the most common imaging technique for the evaluation of esophageal cancer, is non-invasive, low cost, easy to perform, and has a short examination time. It can also be used to evaluate lymph nodes and primary tumors (19). A prospective controlled study showed that CT and EUS had similar accuracy in differentiating between T1/T2 and T3/T4 esophageal cancer (20,21).
Radiomics has improved the accuracy of CT in tumor staging in patients with esophageal cancer. Previous studies have shown that CT-based radiomics has satisfying value in diagnosing the overall stage (22), T stage (23), and lymphatic metastasis (24-26). However, previous studies have mainly focused on patients with locally advanced esophageal cancer. To date, no study on the value of CT-based radiomics in identifying preoperative understaging in patients with cT1–2N0 esophageal cancer has been conducted.
The present study sought to develop and validate a CT-based radiomics model to predict the preoperative understaging in patients with cT1–2N0 esophageal squamous cell carcinoma (ESCC). In addition, the study also assessed the prognostic value of the model for DFS in these patients.
Methods
Patients
This retrospective study was approved by the Institutional Review Boards of Peking University Cancer Hospital and Henan Cancer Hospital and was conducted according to the guidelines of the Declaration of Helsinki (as revised in 2013). The requirement of informed consent was waived due to the retrospective nature of the study.
A total of 196 patients with cT1–2N0 ESCC who underwent radical surgery at two hospitals [Peking University Cancer Hospital (Hospital 1) and Henan Cancer Hospital (Hospital 2)] were retrospectively identified. The patients from Hospital 1 (n=134) were diagnosed between January 2015 and December 2017, and the patients from Hospital 2 (n=62) were diagnosed between January 2017 and June 2018. To be eligible for inclusion in the study, the patients had to meet the following inclusion criteria: (I) have biopsy-proven ESCC; (II) have qualified preoperative enhanced CT images and have been clinically staged as cT1–2N0 based on the CT images; (III) have a time interval of less than 30 days between the preoperative CT examination and radical esophagectomy; and (IV) have undergone radical esophagectomy without neoadjuvant therapy. Patients were excluded from the study if they met any of the following exclusion criteria: (I) had esophageal adenocarcinoma, neuroendocrine tumor, etc.; (II) had undergone preoperative chemotherapy or radiochemotherapy; (III) had failed to have over 11 lymph nodes excised by radical surgery (27); (IV) had multiple esophageal primary tumors, or had esophageal primary tumors combined with other malignant tumors; and/or (V) had poor quality enhanced images. Patients from Hospital 1 were used as the training cohort. Patients from Hospital 2 were used as the independent external validation cohort for the developed model.
CT protocols
All the patients underwent enhanced chest CT examinations before surgery. At Hospital 1, the scans were performed with a Lightspeed VCT (GE Healthcare, Milwaukee, WI, USA) or Brilliance iCT (Philips Healthcare, Cleveland, OH, USA). The following imaging parameters were used: tube voltage: 120–140 kVp; tube current: 200–400 mA; rotation time: 0.6 s; detector collimation: 64×0.625 mm; slice thickness: 5 mm; and matrix: 512 mm × 512 mm. At Hospital 2, the scans were performed with Bright speed 16-slice or Light speed Pro 32-slice VCT (GE Healthcare, Milwaukee, WI, USA) or Philips 256 iCT (Philips Healthcare). The following imaging parameters were used: tube voltage: 120 kVp; tube current: 123–344 mA; rotation time: 0.5 s; detector collimation: 64×0.625 mm or 16×1.25 mm, or 32×1.25 mm; slice thickness: 5 mm; and matrix: 512 mm × 512 mm.
CT scans of chest are from neck to renal hilum. The scans were started 55 seconds after the intravenous injection of non-ionic contrast material (1.5 mL/kg body weight; Hospital 1: Omnipaque 300, GE Healthcare; Loversol, Jiangsu Hengrui Medicine, Jiangsu, China; Hospital 2: Ousu, Yangtze River Pharmaceutical, Taizhou, China) at a rate of 3 mL/s via a pump injector. Sagittal and coronal reconstructions were carried out with the contrast-enhanced images.
CT evaluation and follow-ups
ESCC was considered cT1–2N0 when it met the following criteria: there was diffuse or focal esophageal wall thickening (greater than 5 mm) without periesophageal fat infiltration; and there were no intrathoracic and abdominal lymph nodes larger than 1 cm and no supraclavicular and para-recurrent nerve lymph nodes larger than 5 mm in the short-axis diameter (19,20).
The location, thickness, and length of the primary tumors evaluated based on CT images were recorded. All the CT images were reviewed by two radiologists (B.Z. and S.Y.) with 6 and 9 years of experience in chest imaging, respectively. A third radiologist (Y.J.S.) with 15 years of experience was consulted when disagreements arose. The reviewers were aware of the preoperative endoscopic findings but were blinded to patients’ pathological stage.
The primary tumors were located based on the center of the tumor according to the eighth edition of the American Joint Committee on Cancer (AJCC) Staging Manual (28). The thickness of the tumor was measured from the inner surface to the outer surface of the esophageal wall perpendicular to the lumen on the axial images at the slice with the largest diameter of the tumor. The tumor length was calculated as the slice thickness multiplied by the number of images in which the tumor was present, taking the sagittal images as the reference.
After the radical esophagectomy, regular follow-ups were conducted, including interviews at a three-month interval for the first two years, followed by a six-month interval for one more year, and finally a 12-month interval until death. DFS was used as the endpoint for the analysis. DFS was measured from the date of surgery to the date of first local or distant recurrence or disease-specific death. For patients without disease progression, DFS was censored at the last follow-up visit.
CT segmentation
We exported the enhanced CT images from the picture archiving and communication system and then delineated the volumes of interest (VOIs) of the primary tumors on the axial images using open-source software three-dimensional slicer (https://www.slicer.org). The tumor boundaries were determined with reference to the sagittal and coronal images. The VOIs were first manually delineated slice by slice to encompass the tumor, avoiding the esophageal lumen, vessels, periesophageal fat, and artifacts. Next, a thresholding method was set to refine the regions of interest (ROIs) to avoid the part with CT values below −50 Hounsfield unit (HU) or over 200 HU. The ROIs were delineated by a radiologist (Reader 1: B.Z.) with 6 years of experience. Thirty randomly selected patients in the training cohort were delineated for a second time by Reader 1 one month later to assess the repeatability and were delineated by another reader (Reader 2: S.Y.) with nine years of experience to assess the interreader consistency.
Feature selection and model construction
A total of 1,316 features were extracted from VOIs by the Pyradiomics (V3.0.1) package and Python (V3.6.4), including 14 shape features, 18 first-order features, 24 gray-level co-occurrence matrix features, 14 gray-level difference matrix features, 16 gray-level run length matrix features, 16 gray-level size zone features, five neighborhood gray-tone difference matrix features from the original images and 93 features from each of the exponential, gradient, logarithm, square, and square root transform, and 744 features from the wavelet transform. The intraclass correlation coefficient (ICC) between the two readers was calculated for each feature from the 30 patients. Any feature with an ICC smaller than 0.8 was removed. A t-test was used to compare the radiomics features between the positive and negative samples in the training group. The radiomics features with a P value smaller than 0.05 were reserved for further analysis, while other features were removed. Correlations between each pair of the remaining radiomics features were calculated by the Pearson correlation analysis to reduce their redundancy. If the absolute value of the Pearson correlation coefficient was larger than 0.5 for any pair of the remaining radiomics features, then the feature with the smaller T value in the t-test was removed. Logistic regression with least absolute shrinkage and selection operator (LASSO) was performed in the training group. Five-fold cross-validation was used to determine the weight of L1 regularization by maximizing the average accuracy. All the subjects in the training group were trained again to obtain the coefficient of each selected features in their linear combination.
Statistical analysis
The primary aim of the study was to explore the power of CT radiomics in assessing preoperative understaging in cT1–2N0 ESCC patients. “Preoperative understaging” was defined as a patient with a pathologic stage of T3–4N0 (pT3–4N0) or pTanyN1–3 being misdiagnosed as cT2N0 before surgery. A univariate analysis was conducted to identify the preoperative clinical and CT characteristics that were correlated with preoperative understaging, including age, sex, differentiation, and the location, length, thickness, and thickness-to-length ratio (TLR) of the tumor. A multivariate logistic analysis was conducted to construct a model that combined the clinical characteristics and CT radiomics score for preoperative understaging prediction. Next, the receiver operating characteristic (ROC) curves were used to assess the diagnostic performance of the models, and Delong’s method was used to compare the areas under the ROC curve (AUCs) of the models. Further, a calibration curve was drawn by bootstrapping 1,000 samples to evaluate the calibration of the model, and the Hosmer-Lemeshow fit test was also used.
The secondary aim of this study was to investigate the prognostic value of the CT radiomics model for DFS. Univariate and multivariate Cox regression analyses were conducted to analyze the correlations between the clinical characteristics and the nomogram and DFS in the training cohort, and the hazard ratios (HRs) and 95% confidence intervals (CIs) were also calculated. Harrell’s concordance index (C-index) was used to analyze the predictive performance of the radiomics models. Kaplan-Meier curves were generated to assess the relationship between the nomogram and DFS.
The statistical analysis was carried out using SPSS 22.0 (IBM, Armonk, NY, USA) and R 3.5.0 software (https://www.r-project.org/). The categorical variables are presented as numbers (%) and were compared using a two-sided chi-square test. The Kolmogorov-Smirnov test was applied to check the normality of the continuous variables. The normally distributed variables are presented as the mean ± standard deviation, and the skewed data are presented as the median (interquartile range) and were compared using an independent t-test and Mann-Whitney U test, respectively. A P value <0.05 was considered statistically significant.
Results
Demographic characteristics
A total of 134 patients from Hospital 1 with an average age of 63 years were included as the training cohort, and 62 patients from Hospital 2 with an average age of 64 years were included as the external validation cohort. Preoperative understaging was detected in 50.0% (67/134) and 51.6% (32/62) of the patients in the training cohort and external validation cohort, respectively. No significant difference was found in the clinical and pathological characteristics between the cohorts (Table 1).
Table 1
Variables | Training cohort (n=134) | External validation cohort (n=62) | P |
---|---|---|---|
Age, year, median [IQR] | 63.00 [58.00, 68.00] | 64.00 [59.00, 67.00] | 0.917 |
Sex, case (%) | |||
Female | 25 (18.7) | 16 (25.8) | 0.339 |
Male | 109 (81.3) | 46 (74.2) | |
Differentiation, case (%) | |||
Well | 24 (17.9) | 12 (19.4) | 0.732 |
Moderate | 77 (57.5) | 32 (51.6) | |
Poorly | 33 (24.6) | 18 (29.0) | |
Tumor length, mm, median [IQR] | 22.50 [18.00, 31.50] | 21.00 [18.00, 31.12] | 0.589 |
Tumor thickness, mm, median [IQR] | 11.00 [8.00, 14.00] | 11.50 [8.25, 15.00] | 0.356 |
Tumor location, case (%) | |||
Upper | 6 (4.5) | 4 (6.5) | 0.637 |
Middle | 63 (47.0) | 32 (51.6) | |
Lower | 65 (48.5) | 26 (41.9) | |
Ratio of tumor thickness/length, median [IQR] | 0.48 [0.37, 0.59] | 0.53 [0.35, 0.76] | 0.282 |
Pathological T stage, case (%) | |||
pT1 | 35 (26.1) | 12 (19.4) | 0.537 |
pT2 | 57 (42.5) | 27 (43.5) | |
pT3 | 42 (31.3) | 23 (37.1) | |
Pathological N stage, case (%) | |||
pN0 | 91 (67.9) | 44 (71.0) | 0.710 |
pN1 | 35 (26.1) | 16 (25.8) | |
pN2 | 8 (6.0) | 2 (3.2) | |
Preoperative understaging, case (%) | 67 (50.0) | 32 (51.6) | 0.955 |
IQR, interquartile range.
Factors associated with preoperative understaging
The results of the univariate logistic analysis showed that tumor length, tumor thickness, and TLR were correlated with preoperative understaging (Table 2). The results of the multivariate logistic regression analysis revealed that tumor thickness and TLR were independent measurements that were correlated with understaging, with odds ratios of 1.537 (95% CI: 1.162–2.034) and 0.000 (95% CI: 0.000–0.003) (P<0.05), respectively.
Table 2
Factors | Univariate analysis | Multivariate analysis | |||
---|---|---|---|---|---|
OR (95% CI) | P | OR (95% CI) | P | ||
Age | 0.972 (0.928–1.102) | 0.227 | |||
Sex | |||||
Female | Reference | ||||
Male | 2.507 (0.998–6.298) | 0.051 | |||
Differentiation | |||||
Poorly | Reference | ||||
Moderate | 0.390 (0.132–1.151) | 0.088 | |||
Well | 0.633 (0.276–1.451) | 0.280 | |||
Tumor location | |||||
Upper | Reference | ||||
Middle | 0.378 (0.065–2.215) | 0.281 | |||
Lower | 0.605 (0.301–1.217) | 0.159 | |||
Tumor length | 1.121 (1.061–1.184) | <0.001 | 0.908 (0.794–1.039) | 0.162 | |
Tumor thickness | 1.128 (1.031–1.233) | 0.009 | 1.537 (1.162–2.034) | 0.003 | |
Ratio of tumor thickness/length | 0.004 (0.000–0.070) | <0.001 | 0.000 (0.000–0.003) | <0.001 |
IQR, interquartile range; OR, odds ratio; CI, confidence interval.
Radiomics feature selection and model construction
A total of 893 features remained after removing features with ICCs smaller than 0.8. Next, 586 features were removed by the t-test, and 298 features were removed due to redundancy. The remaining nine features were input into the LASSO logistic regression model. The optimal weight of L1 regularization was chosen as 0.01 by five-fold cross-validation. Finally, seven features with non-zero coefficients were selected. The radiomics score was given as the linear combination of the features in the following equation:
Construction of the combined model and nomogram
Based on the logistic regression analysis, we established a clinical radiomics combined model for preoperative understaging prediction that included the TLR and radiomics score. The AUCs of the combined model for preoperative understaging prediction were 0.874 (95% CI: 0.815–0.933) in the training cohort and 0.812 (95% CI: 0.703–0.912) in the external validation cohort (Table 3). The results of the DeLong test revealed that the combined model and the radiomics model outperformed the clinical model in the training cohort (P=0.024 and 0.001, respectively) and in the external validation cohort (P=0.038 and 0.201, respectively). A nomogram was developed to show the clinical utility of the combined model (Figure 1A). The calibration curve of the nomogram showed good consistency between the predicted risks and the actual risks for understaging in the training and external validation cohorts (Figure 1B,1C). The Hosmer-Lemeshow goodness-of-fit tests results for the combined model were P=0.77 in the training cohort and P=0.95 in the external validation cohort.
Table 3
Variables | AUC (95% CI) | Sensitivity | Specificity | PPV | NPV | Accuracy |
---|---|---|---|---|---|---|
Training cohort | ||||||
Clinical model | 0.795 (0.718–0.871) | 0.78 | 0.73 | 0.74 | 0.77 | 0.75 |
Radiomics model | 0.864 (0.803–0.925) | 0.79 | 0.81 | 0.80 | 0.79 | 0.80 |
Clinico-radiomics combined model | 0.874 (0.815–0.933) | 0.81 | 0.87 | 0.86 | 0.82 | 0.84 |
External validation cohort | ||||||
Clinical model | 0.727 (0.592–0.861) | 0.78 | 0.73 | 0.76 | 0.76 | 0.76 |
Radiomics model | 0.796 (0.683–0.910) | 0.88 | 0.70 | 0.76 | 0.84 | 0.79 |
Clinico-radiomics combined model | 0.812 (0.703–0.912) | 0.84 | 0.73 | 0.77 | 0.81 | 0.79 |
AUC, area under the curve; CI, confidence interval; PPV, positive predictive value; NPV, negative predictive value.
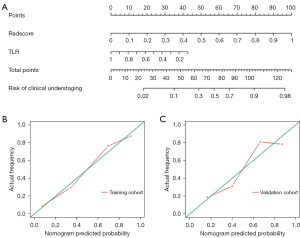
The Brier score for the combined model was 0.175, a figure lower than that of the model that included the radiomics features alone (0.182) or the clinical model (0.200), indicating the higher accuracy of the combined model. Brier scores for the three models were obtained using the same external validation cohort.
Predictive value for DFS
The median follow-up duration in both cohorts was 36.7 months (range, 3–63.6 months). At the last follow-up, recurrence was confirmed in 28 (20.9%) and 11 (17.7%) patients in the training and validation cohorts, respectively (P=0.607). The results of the Cox analysis for DFS are listed in Table 4. Tumor thickness, the TLR, radiomics score, pathological T stage, and Node stage were covariables that exhibited statistical significance in the univariate Cox analysis for DSF (P<0.05). The multivariate analysis based only on preoperative factors showed that only the total score of the nomogram independently predicted DFS (HR: 13.074, 95% CI: 2.075–82.376; P=0.006). The C-index of the nomogram for DFS was 0.722 (95% CI: 0.648–0.796) in the training cohort and 0.689 (95% CI: 0.531–0.847) in the validation cohort. The patients in the training and validation cohorts were classified into high- and low-risk groups based on the corresponding nomogram threshold value. The prognosis of the high-risk group was significantly worse than that of the low-risk group in the training (Figure 2A) and validation cohorts (Figure 2B).
Table 4
Variables | Univariate analysis | Multivariate analysis | |||
---|---|---|---|---|---|
HR (95% CI) | P | HR (95% CI) | P | ||
Age | 0.984 (0.918–1.055) | 0.652 | |||
Sex | |||||
Female | Reference | ||||
Male | 1.986 (0.599–6.577) | 0.220 | |||
Tumor location | |||||
Upper | Reference | ||||
Middle | 1.160 (0.150–8.941) | 0.887 | |||
Lower | 1.309 (0.631–2.797) | 0.487 | |||
Differentiation | |||||
Well | Reference | ||||
Moderate | 0.760 (0.276–2.091) | 0.595 | |||
Poorly | 0.668 (0.204–2.192) | 0.506 | |||
Tumor length | 1.036 (0.992–1.082) | 0.114 | |||
Tumor thickness | 1.067 (0.983–1.158) | 0.121 | |||
Ratio of tumor thickness/length | 0.022 (0.001–0.432) | 0.012 | 0.952 (0.021–43.479) | 0.980 | |
Risks stratified by nomogram | |||||
Low-risk group | Reference | ||||
High-risk group | 13.280 (3.327–53.000) | <0.001 | 13.074 (2.075–82.376) | 0.006 | |
Pathological T stage | |||||
pT1–2 | Reference | ||||
pT3 | 2.181 (1.038–4.585) | 0.040 | |||
Pathological N stage | |||||
pN0 | Reference | ||||
pN1–3 | 2.327 (1.109–4.883) | 0.003 | |||
Pathological TN stage | |||||
T1–2N0 | Reference | ||||
T3N0/TanyN1–3 | 17.68 (3.034–103.034) | 0.001 |
HR, hazard ratio; CI, confidence interval.
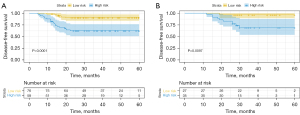
Instructions for clinical use
The clinical use of the nomogram consisted of the following steps: (I) select patients with CT-based clinical stage cT1–2N0 ESCC; (II) calculate the TLR and the radiomics score based on the CT images; (III) calculate the scores for the TLR and the radiomics score according to the nomogram (the corresponding score of each factor is listed on the top line of the nomogram); (IV) sum the scores for each factor; and (V) calculate the corresponding risk of the total score according to the line at the bottom of the nomogram. Figure 3 shows examples of the clinical use of the nomogram.
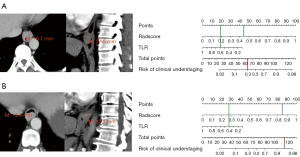
Discussion
In the present study, we developed and validated a clinico-radiomics model combining radiomics features and the TLR of tumors for the identification of preoperative understaging in patients with cT1–2N0 ESCC. The nomogram based on the combined model showed satisfying diagnostic ability in both the training and external validation cohorts, with AUCs of 0.874 (95% CI: 0.815–0.933) and 0.812 (95% CI: 0.703–0.912), respectively. In addition, the score of the nomogram based on the combined model was an independent variable that predicted postoperative DFS in patients with ESCC, providing valuable radiological support for personalized therapeutic plans.
Radiomic features analysis provides voxel-scale information about the compositional distribution profile within a tumor beyond its anatomic size. We extracted quantitative image features of tumors using contrast-enhanced CT. The LASSO method was employed to reduce dimensionality. A total of seven potential radiomics features had a proper ratio for building the models that could avoid overfitting. Elongation was the most important factor in the radiomics model. Elongation was defined as the square root of the quotient of λ-minor to λ-major, where λ-major and λ-minor were the lengths of the largest and second-largest principal component axes of the ROI, respectively (29). Elongation ranged from 1 (non-elongated) to 0 (maximally elongated, like a one-dimensional line), with convergence to 1 indicating that the ROI was nearly a circle, and convergence to 0 indicating that the ROI was nearly a straight line (30). Elongation reflected the degree of regularity of the ROI, which may be determined by the tumor growth pattern and biological behavior. Our results suggested that the more irregular the shape of the tumor, the higher the possibility of the preoperative understaging of patients.
It has been proven that the tumor length and tumor thickness of ESCC are positively correlated with T stage (19,31) and lymphatic metastasis (32,33). The present study demonstrated that tumor length and tumor thickness were also covariates for preoperative understaging in patients with cT1–2N0 ESCC. A clinical model constructed based on the tumor thickness and the TLR achieved AUCs of 0.727–0.795. However, its performance was inferior compared to that of the clinico-radiomics model that combined the TLR and radiomics score. The combined model showed satisfying diagnostic ability for preoperative understaging with AUCs of 0.812–0.874. It is notable that in the multivariate analysis, the TLR was negatively correlated with preoperative understaging.
In addition, the score of the nomogram based on the combined clinic-radiomics model was an independent predictor for postoperative DFS in patients with cT1–2N0 ESCC in our study. Compared to patients with preoperative understaging cT1–2N0 cancer, those who were accurately staged had prolonged DFS. A multimodality approach with neoadjuvant therapy followed by surgery might be appropriate for those patients with a high risk of preoperative understaging.
This study had several limitations. First, as a retrospective study with a small sample size and only one external validation cohort, selection bias might have influenced the results. Thus, the generalizability of the results needs to be further verified. Second, as EUS was not available for some patients in this retrospective study, we used CT as an imaging modality for clinical stage without reference to EUS. Future research and an analysis of patients with cT1–2N0 staging combined with CT, EUS, and positron emission tomography/CT should be performed. Third, we chose to use the venous phase images for the radiomics analysis, and it is unclear whether the combination of multiphase CT images could provide more information. Fourth, the manual ROI delineation was time consuming. Semiautomatic segmentation and deep-learning methods require further research.
Conclusions
In conclusion, we established a non-invasive and convenient CT-based radiomics model that can accurately predict the risk of preoperative understaging in patients with cT1–2N0. Patients at high risk of preoperative understaging have shortened DFS and a poor prognosis after radical surgery without neoadjuvant therapy. Thus, the CT-based radiomic model could help in clinical decision making and the individualized treatment of patients with cT1–2N0 ESCC, and could improve the survival of patients.
Acknowledgments
Funding: This research was funded by
Footnote
Conflicts of Interest: All authors have completed the ICMJE uniform disclosure form (available at https://qims.amegroups.com/article/view/10.21037/qims-23-275/coif). The authors have no conflicts of interest to declare.
Ethical Statement:
Open Access Statement: This is an Open Access article distributed in accordance with the Creative Commons Attribution-NonCommercial-NoDerivs 4.0 International License (CC BY-NC-ND 4.0), which permits the non-commercial replication and distribution of the article with the strict proviso that no changes or edits are made and the original work is properly cited (including links to both the formal publication through the relevant DOI and the license). See: https://creativecommons.org/licenses/by-nc-nd/4.0/.
References
- Bray F, Ferlay J, Soerjomataram I, Siegel RL, Torre LA, Jemal A. Global cancer statistics 2018: GLOBOCAN estimates of incidence and mortality worldwide for 36 cancers in 185 countries. CA Cancer J Clin 2018;68:394-424. [Crossref] [PubMed]
- Orazbayev BA, Musulmanbekov K, Bukenov A. Analysis of Treatment Results of the Thoracic Part of Oesophageal Cancer. Open Access Maced J Med Sci 2019;7:82-7. [Crossref] [PubMed]
- Rice TW, Ishwaran H, Hofstetter WL, Kelsen DP, Apperson-Hansen C, Blackstone EH; Worldwide Esophageal Cancer Collaboration Investigators. Recommendations for pathologic staging (pTNM) of cancer of the esophagus and esophagogastric junction for the 8th edition AJCC/UICC staging manuals. Dis Esophagus 2016;29:897-905.
- Shapiro J, van Lanschot JJB, Hulshof MCCM, van Hagen P, van Berge Henegouwen MI, Wijnhoven BPL, et al. Neoadjuvant chemoradiotherapy plus surgery versus surgery alone for oesophageal or junctional cancer (CROSS): long-term results of a randomised controlled trial. Lancet Oncol 2015;16:1090-8. [Crossref] [PubMed]
- Lordick F, Mariette C, Haustermans K, Obermannová R, Arnold DESMO Guidelines Committee. Oesophageal cancer: ESMO Clinical Practice Guidelines for diagnosis, treatment and follow-up. Ann Oncol 2016;27:v50-7. [Crossref] [PubMed]
- Sugawara K, Yamashita H, Uemura Y, Yagi K, Nishida M, Aikou S, Nomura S, Seto Y. Preoperative lymph node status on computed tomography influences the survival of pT1b, T2 and T3 esophageal squamous cell carcinoma. Surg Today 2019;49:378-86. [Crossref] [PubMed]
- Gockel I, Sgourakis G, Lyros O, Polotzek U, Schimanski CC, Lang H, Hoppo T, Jobe BA. Risk of lymph node metastasis in submucosal esophageal cancer: a review of surgically resected patients. Expert Rev Gastroenterol Hepatol 2011;5:371-84. [Crossref] [PubMed]
- Markar SR, Gronnier C, Pasquer A, Duhamel A, Beal H, Théreaux J, Gagnière J, Lebreton G, Brigand C, Meunier B, Collet D, Mariette C. FREGAT working group – FRENCH – AFC. Role of neoadjuvant treatment in clinical T2N0M0 oesophageal cancer: results from a retrospective multi-center European study. Eur J Cancer 2016;56:59-68. [Crossref] [PubMed]
- Chen WH, Chao YK, Chang HK, Tseng CK, Wu YC, Liu YH, Hsieh MJ, Liu HP. Long-term outcomes following neoadjuvant chemoradiotherapy in patients with clinical T2N0 esophageal squamous cell carcinoma. Dis Esophagus 2012;25:250-5. [Crossref] [PubMed]
- Stiles BM, Mirza F, Coppolino A, Port JL, Lee PC, Paul S, Altorki NK. Clinical T2-T3N0M0 esophageal cancer: the risk of node positive disease. Ann Thorac Surg 2011;92:491-6; discussion 496-8. [Crossref] [PubMed]
- Zhang JQ, Hooker CM, Brock MV, Shin J, Lee S, How R, Franco N, Prevas H, Hulbert A, Yang SC. Neoadjuvant chemoradiation therapy is beneficial for clinical stage T2 N0 esophageal cancer patients due to inaccurate preoperative staging. Ann Thorac Surg 2012;93:429-35; discussion 436-7. [Crossref] [PubMed]
- Speicher PJ, Ganapathi AM, Englum BR, Hartwig MG, Onaitis MW, D'Amico TA, Berry MF. Induction therapy does not improve survival for clinical stage T2N0 esophageal cancer. J Thorac Oncol 2014;9:1195-201.
- Samson P, Puri V, Robinson C, Lockhart C, Carpenter D, Broderick S, Kreisel D, Krupnick AS, Patterson GA, Meyers B, Crabtree T. Clinical T2N0 Esophageal Cancer: Identifying Pretreatment Characteristics Associated With Pathologic Upstaging and the Potential Role for Induction Therapy. Ann Thorac Surg 2016;101:2102-11. [Crossref] [PubMed]
- Goense L, Visser E, Haj Mohammad N, Mook S, Verhoeven RHA, Meijer GJ, van Rossum PSN, Ruurda JP, van Hillegersberg R. Role of neoadjuvant chemoradiotherapy in clinical T2N0M0 esophageal cancer: A population-based cohort study. Eur J Surg Oncol 2018;44:620-5. [Crossref] [PubMed]
- Wolfson P, Ho KMA, Bassett P, Haidry R, Olivo A, Lovat L, Sami SS. Accuracy of clinical staging for T2N0 oesophageal cancer: systematic review and meta-analysis. Dis Esophagus 2021;34:doab002. [Crossref] [PubMed]
- Semenkovich TR, Panni RZ, Hudson JL, Thomas T, Elmore LC, Chang SH, Meyers BF, Kozower BD, Puri V. Comparative effectiveness of upfront esophagectomy versus induction chemoradiation in clinical stage T2N0 esophageal cancer: A decision analysis. J Thorac Cardiovasc Surg 2018;155:2221-2230.e1. [Crossref] [PubMed]
- Hardacker TJ, Ceppa D, Okereke I, Rieger KM, Jalal SI, LeBlanc JK, DeWitt JM, Kesler KA, Birdas TJ. Treatment of clinical T2N0M0 esophageal cancer. Ann Surg Oncol 2014;21:3739-43. [Crossref] [PubMed]
- Hofstetter W. Treatment of clinical T2N0M0 esophageal cancer. Ann Surg Oncol 2014;21:3713-4. [Crossref] [PubMed]
- Wang Y, Huang Y, Zhao QY, Li XQ, Wang L, Wang NN, Wang JZ, Wang Q. Esophageal wall thickness on CT scans: can it predict the T stage of primary thoracic esophageal squamous cell carcinoma? Esophagus 2022;19:269-77. [Crossref] [PubMed]
- Hong SJ, Kim TJ, Nam KB, Lee IS, Yang HC, Cho S, Kim K, Jheon S, Lee KW. New TNM staging system for esophageal cancer: what chest radiologists need to know. Radiographics 2014;34:1722-40. [Crossref] [PubMed]
- Guo J, Wang Z, Qin J, Zhang H, Liu W, Zhao Y, Lu Y, Yan X, Zhang Z, Zhang T, Zhang S, Dominik NM, Kamel IR, Li H, Qu J. A prospective analysis of the diagnostic accuracy of 3 T MRI, CT and endoscopic ultrasound for preoperative T staging of potentially resectable esophageal cancer. Cancer Imaging 2020;20:64. [Crossref] [PubMed]
- Wu L, Wang C, Tan X, Cheng Z, Zhao K, Yan L, Liang Y, Liu Z, Liang C. Radiomics approach for preoperative identification of stages I-II and III-IV of esophageal cancer. Chin J Cancer Res 2018;30:396-405. [Crossref] [PubMed]
- Yang M, Hu P, Li M, Ding R, Wang Y, Pan S, Kang M, Kong W, Du D, Wang F. Computed Tomography-Based Radiomics in Predicting T Stage and Length of Esophageal Squamous Cell Carcinoma. Front Oncol 2021;11:722961. [Crossref] [PubMed]
- Tan X, Ma Z, Yan L, Ye W, Liu Z, Liang C. Radiomics nomogram outperforms size criteria in discriminating lymph node metastasis in resectable esophageal squamous cell carcinoma. Eur Radiol 2019;29:392-400. [Crossref] [PubMed]
- Ou J, Wu L, Li R, Wu CQ, Liu J, Chen TW, Zhang XM, Tang S, Wu YP, Yang LQ, Tan BG, Lu FL. CT radiomics features to predict lymph node metastasis in advanced esophageal squamous cell carcinoma and to discriminate between regional and non-regional lymph node metastasis: a case control study. Quant Imaging Med Surg 2021;11:628-40. [Crossref] [PubMed]
- Zhao B, Zhu HT, Li XT, Shi YJ, Cao K, Sun YS. Predicting Lymph Node Metastasis Using Computed Tomography Radiomics Analysis in Patients With Resectable Esophageal Squamous Cell Carcinoma. J Comput Assist Tomogr 2021;45:323-9. [Crossref] [PubMed]
- Ajani JA, D'Amico TA, Bentrem DJ, Chao J, Corvera C, Das P, et al. Esophageal and Esophagogastric Junction Cancers, Version 2.2019, NCCN Clinical Practice Guidelines in Oncology. J Natl Compr Canc Netw 2019;17:855-83. [Crossref] [PubMed]
- Rice TW, Ishwaran H, Blackstone EH, Hofstetter WL, Kelsen DP, Apperson-Hansen C; Worldwide Esophageal Cancer Collaboration Investigators. Recommendations for clinical staging (cTNM) of cancer of the esophagus and esophagogastric junction for the 8th edition AJCC/UICC staging manuals. Dis Esophagus 2016;29:913-9.
- Tonetti DA, Gross BA, Desai SM, Jadhav AP, Jankowitz BT, Jovin TG. Final Infarct Volume of <10 cm(3) is a Strong Predictor of Return to Home in Nonagenarians Undergoing Mechanical Thrombectomy. World Neurosurg 2018;119:e941-6. [Crossref] [PubMed]
- Jin H, Lv J, Li C, Wang J, Jiang Y, Meng X, Li Y. Morphological features predicting in-stent stenosis after pipeline implantation for unruptured intracranial aneurysm. Front Neurol 2023;14:1121134. [Crossref] [PubMed]
- Li H, Chen TW, Zhang XM, Li ZL, Chen XL, Tang HJ, Huang XH, Chen N, Yang Q, Hu J. Computed tomography scan as a tool to predict tumor T category in resectable esophageal squamous cell carcinoma. Ann Thorac Surg 2013;95:1749-55. [Crossref] [PubMed]
- Li H, Chen TW, Li ZL, Zhang XM, Chen XL, Wang LY, Zhou L, Li R, Li CP, Huang XH. Tumour size of resectable oesophageal squamous cell carcinoma measured with multidetector computed tomography for predicting regional lymph node metastasis and N stage. Eur Radiol 2012;22:2487-93. [Crossref] [PubMed]
- Yun JK, Kim HR, Park SI, Kim YH. Risk prediction of occult lymph node metastasis in patients with clinical T1 through T2 N0 esophageal squamous cell carcinoma. J Thorac Cardiovasc Surg 2022;164:265-275.e5. [Crossref] [PubMed]