Development and validation of a predictive model for fetal cerebral maturation using ultrasound for fetuses with normal growth and fetal growth restriction
Introduction
Fetal brain structure develops rapidly, and cortical folding drastically changes the brain shape during the second and third trimesters, from a largely smooth surface to a complex convoluted one (1). Investigation of fetal cerebral maturation (FCM) is necessary and important to provide crucial prognostic information for normal and high-risk fetuses. Fetal brain maldevelopment has been reported in fetuses with congenital heart disease (2-4), fetal growth restriction (FGR) (5), fragile-X syndrome (6), Down syndrome (7), and agenesis of the corpus callosum (8). Long-term neurodevelopmental impairment is the most common complication in patients with congenital heart disease (9) and FGR (10,11). Therefore, evaluation of FCM is pertinent in peripheral or central nervous system anomalies.
Numerous publications have reported characteristics of fetal brain development at different stages and quantitative measurements of gyral formation and sulcation with ultrasound screening (12-15). However, it is difficult to interpret FCM in an individual fetus, particularly because the growth patterns differ by regions (16,17). Developing a complete and comprehensive approach that could be routinely used in clinics to assess FCM might solve this problem. The fetal total maturation score (fTMS) (18) and cumulative maturity score (19) have been used for clinical interpretation; these were developed using magnetic resonance imaging (MRI). However, MRI cannot always be performed during prenatal screening and fetal MRI always is for the evaluation of suspected or proven central nervous system anomalies (20). Establishing a model using ultrasound to predict FCM has important implications in pregnancy care. Semi-quantitative morphological grading of evaluation including cortical grading of the sulci, sylvian fissure (SF) and cortical gyration by ultrasound has been established.
The primary aim of our study was to develop a quantitative model to predict FCM considering sonographic measurements of fetal sulcation, and the secondary aim was to validate the predictive model for FCM in fetuses with normal growth and FGR. We present this article in accordance with the TRIPOD reporting checklist (available at https://qims.amegroups.com/article/view/10.21037/qims-23-786/rc).
Methods
Study population and design
The data for this study were derived from prospective screening for routine pregnancy care in the second and third trimesters (from 19+6 to 40+2 gestational weeks) at The First Affiliated Hospital of Sun Yat-sen University (Center 1) and Dalian Municipal Women and Children’s Medical Center (Center 2), between October 10th 2019 and November 10th 2022. Written informed consent was obtained from participants’ parents or legal guardians before screening, and Ethics Committees of The First Affiliated Hospital of Sun Yat-sen University and Dalian Municipal Women and Children’s Medical Center approved the study. The study was conducted in accordance with the Declaration of Helsinki (as revised in 2013).
A normal last menstrual period (LMP) was defined as the most recent menstrual period of a regular cycle without the use of oral contraceptives for 6 months. For the cases with regular menstrual period, gestational age (GA) was calculated considering the first day of the last normal menstrual period and confirmed on first-trimester sonography at 11–13+6 gestational weeks. GA estimations in the first trimester were based on measurements of crown-rump length (21). If there is uncertainty about the date of the LMP or irregular menstrual cycles, or if there was a discrepancy of more than 1 week between menstrual age and sonographic measurements, GA was determined based on sonographic age.
Measurements of fetal biparietal diameter (BPD), head circumference (HC), abdominal circumference (AC), and femur length were performed to calculate the estimated fetal weight (EFW) using the Hadlock formula (22). Birth weight (BW) and HC at birth were calculated as Z-scores, considering reference ranges of the local population (23). The International Society of Ultrasound in Obstetrics and Gynecology (ISUOG)-FGR definition was used in this study, which follows the Delphi consensus criteria and includes either EFW or AC <3rd percentile, or EFW or AC <10th percentile combined with abnormal Doppler findings, or a decrease in growth centiles (24). Only singleton pregnancies were included in this study. Exclusion criteria for normal-growth fetuses included congenital infection, maternal complications (gestational diabetes, chronic hypertension, preeclampsia, or autoimmune diseases), ambiguous ultrasonographic images for analysis, delivery before 37 gestational weeks, and BW Z-scores <−1.29. Exclusion criteria for fetuses with FGR included structural malformations, recorded genetic anomalies (chromosomal anomalies, pathogenic copy number variations, or single gene disease), pregnancy termination, and loss to follow-up. FGR cases were classified, based on GA at the time of diagnosis, into early-onset FGR (<32 weeks) and late-onset FGR (≥32 weeks) (25). All normal-growth fetuses included in the study were confirmed via postnatal ultrasonography or MRI to have normal brain structures and normal prenatal development. Delayed development of sulci and gyri were confirmed in FGR fetuses via postnatal ultrasonography or MRI.
Sonographic measurements
Ultrasonography was performed using a Voluson E8 and E10 ultrasonography machine (GE Healthcare, Zipf, Austria) with a RAB2-5 (2–5 MHz) convex probe and RIC5-9-D (4–8 MHz) probe. Evaluation of structures and biological measurements in the second and third trimesters were performed by experienced operators, following the guidelines published by ISUOG (26). Both of the left and right hemisphere of the brain were evaluated and the average value was recorded for final analysis.
First, we obtained a standard trans-ventricular plane of the fetal head to perform measurements of BPD and HC per ISUOG guidelines, in which the atrium and posterior horns of the left and right lateral ventricles, the cavum septi pellucidum (CSP), and the choroid plexus could be clearly identified. The depth of the insula (INS) was measured in the trans-ventricular plane by drawing a perpendicular line from the midline towards the upper border of the insular cortex (Figure 1). The depth of the SF was also measured in the trans-ventricular plane by drawing a line in continuation with the insular line from the insular cortex to the inner border of the parietal bone (Figure 1).
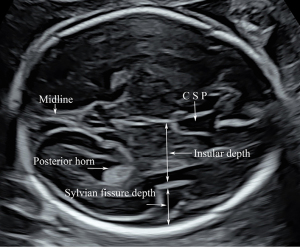
A plane above the level of the trans-ventricular plane was used to measure the depth of the parieto-occipital fissure (POF). After visualizing the BPD plane, the probe was moved cranially to obtain a plane for measurement of the POF, which was parallel to the BPD and HC planes. We moved the probe up and down in parallel to confirm that the full fissure depth could be visualized. In this view, the POF appeared as a triangular shape in the second and early third trimesters, and the apex of the POF was lateral to the midline. We drew a perpendicular line from the midline to the apex of the POF, representing the depth of the POF (Figure 2). After visualizing the trans-cerebellar plane, the probe was rotated 90° to obtain the coronal view of the cisterna magna and cerebellum, in which plane the calcarine fissure (CF) was measured. In this plane, a perpendicular line was drawn from the CF apex to the tentorium cerebelli, the length of which was obtained as the CF depth (Figure 3). All measurements were performed by two operators (authors 1 and 3, with 13- and 15-year experience of prenatal ultrasonographic image analysis, respectively) who were blinded to the clinical data and outcomes. Each parameter was measured in triplicate from three separately obtained ultrasound images, and the mean of the three measurements was used for calculations.
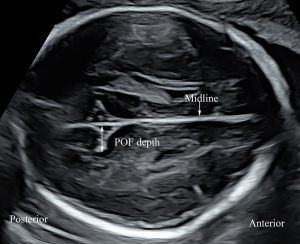
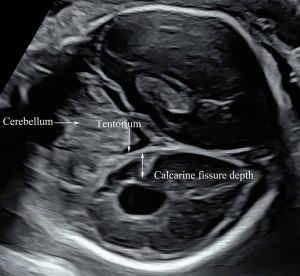
Clinical data
Recorded variables for mothers included age at ultrasound examination, pre-pregnancy body mass index (BMI), delivery history, complications, smoking history, alcohol consumption, or drug abuse. Recorded variables for neonates included sex, BW, GA at birth, HC, Apgar score, transfer to the neonatal intensive care unit, length of hospitalization, and neonatal complications. Neonatal complications included respiratory distress syndrome, sepsis, intraventricular hemorrhage (grade III or IV), cystic periventricular leukomalacia, hypoglycemia (<2.2 mmol/L), and necrotizing enterocolitis.
Statistical analyses
In 30 cases, paired measurements were performed by one operator (author 1) to determine the intraobserver reproducibility and by a second operator (author 5) to determine the interobserver reproducibility. The between-observer 95% limits of agreement were depicted with Bland-Altman plots using MedCalc 9 Software (MedCalc Software, Mariakerke, Belgium). Furthermore, we calculated the intraclass correlation coefficient (ICC) to evaluate the interobserver and intraobserver reliabilities. Medium, good, and perfect agreement was defined as 0.4< ICC <0.6, 0.61< ICC <0.8, and 0.81< ICC <1.0, respectively.
Data from normal or non-normal continuous variables are expressed as mean ± standard deviation or median (interquartile range), respectively, and categorical data as n (%). Comparisons between the different datasets (fetuses with normal growth and FGR) were performed using the chi-square test for categorical variables and Student’s t-test or the Mann-Whitney U-test for continuous variables. The 95% confidence intervals (CIs) of the variables were reported, if necessary.
Normal-growth fetuses recruited from Centers 1 and 2 were included as a training set and an external validation set 1, respectively. Fetuses with FGR recruited from Center 1 underwent FCM assessment as validation set 2. The FGR fetuses recruited from Center 1 and included in the validation set were excluded from the training set. Normality tests for INS, SF, POF, and CF were performed using the Kolmogorov-Smirnov test. The predictive model for FCM using ultrasound images was developed considering five morphological variables: HC, INS, SF, POF, and CF. The fetal total cranial parameter (fTCP) was calculated by summing the measurements of HC, INS, SF, POF, and CF. A regression model for FCM was constructed considering fTCP. Linear, quadratic, cubic, logarithmic, and exponential models were compared and analyzed, and the optimal model was determined. When a more complex model could not significantly improve the goodness of fit, a simpler model was used. The prediction model developed from the training dataset was applied to the validation dataset to assess the predictive performance. P<0.05 indicated statistical significance. The Statistical Package for the Social Sciences (SPSS) 22.0 software (IBM SPSS Statistics for Windows, Version 22.0. Armonk, NY: IBM Corp, 2013) was used for data analyses. We use complete-case analysis for handling missing data. The study size was acquired according to the formula that n= [(Z1−α/2×σ)/δ]2, α=0.05, δ=0.2, σ=1.0.
Results
First, a total of 432 fetuses were examined, and their data were used to construct the predictive model after excluding 17 fetuses with BW Z-score <−1.29, 6 fetuses with delivery before 37 gestational weeks, and 21 fetuses with incomplete measurements of cranial parameters. A total of 214 normal-growth fetuses recruited from Center 2 were included in the external validation dataset. In addition, the developed predictive model was also applied to 124 fetuses with FGR from Center 1, after excluding 10 cases of lost to follow-up, 11 cases of terminated pregnancies and 2 cases of intrauterine death (Figure 4). Follow-up was performed with a median infant age of 15 months in all patients, ranging from 4 to 31 months. Fifty-eight (46.7%, 58/124) were identified as early-onset FGR and 66 (56.4%) were as late-onset FGR. The baseline maternal, obstetric, and neonatal characteristics in the training and validation datasets are summarized in Table 1.
Table 1
Characteristics | Training dataset (n=432) | Validation dataset (n=214) | FGR fetuses (n=124) | P |
---|---|---|---|---|
Maternal characteristics | ||||
Age in years | 31 [21–44] | 31 [20–46] | 31 [20–41] | 0.853 |
Pre-pregnancy BMI, kg/cm2 | 20.6 [15.2–34] | 20.6 [14.2–31.7] | 20.6 [15.6–30.8] | 0.241 |
Cigarette smoking | 2 (0.5) | 1 (0.5) | 1 (0.8) | 0.889 |
Nulliparous | 267 (61.8) | 132 (61.7) | 82 (66.1) | 0.665 |
IVF-ET | 21 (4.9) | 12 (5.6) | 8 (6.5) | 0.893 |
Ultrasound examination | ||||
Gestational age in weeks | 29+6 [19+6–40+2] | 28+5 [20+3–40] | 31+3 [22+4–40+1] | 0.766 |
Cephalic presentation | 371 (85.9) | 181 (84.6) | 102 (82.3) | 0.601 |
Delivery and neonate | ||||
Gestational age at birth, weeks | 39+1 [37–41+3] | 39+2 [37–41+1] | 37+5 [27+3–40+6] | 0.016 |
Cesarean delivery | 209 (48.4) | 89 (41.6) | 63 (50.8) | 0.168 |
Birth weight, g | 3,150 [2,460–4,250] | 3,180 [2,440–4,170] | 2,310 [700–2,950] | <0.001 |
Birth weight Z-score | 0.18 [−1.23 to 4.26] | 0.15 [−1.22 to 4.24] | −2.19 [−5.16 to −1.33] | <0.001 |
Neonatal head circumference, cm | 33 [31–37] | 33.5 [31–37] | 31 [23–34] | <0.001 |
Neonatal head circumference Z-score | 0.33 [−1.96 to 4.21] | 0.40 [−1.77 to 4.18] | −0.85 [−3.88 to 1.45] | <0.001 |
Male sex | 250 (57.9) | 108 (50.5) | 59 (47.6) | 0.061 |
5-min Apgar score ≤7 | 9 (2.1) | 5 (2.3) | 8 (6.5) | 0.028 |
Lower umbilical artery PH (≤7.20)* | 23 (7.3)* | 11 (6.7)* | 7 (8.5)* | 0.868 |
Transferred to NICU | 35 (8.1) | 17 (7.9) | 35 (28.2) | <0.001 |
Length of hospitalization in days | 3 [2–15] | 3 [2–28] | 5 [2–91] | <0.001 |
Neonatal complications | 30 (6.9) | 13 (6.1) | 20 (16.1) | <0.001 |
Data are presented as n (%) or median [range]. *, umbilical artery PH was obtained from 314 fetuses in the training dataset, 165 fetuses in the external validation dataset, and 82 fetuses with FGR. FGR, fetal growth restriction; BMI, body mass index; IVF-ET, in vitro fertilization and embryo transfer; PH, potential of hydrogen; NICU, neonatal intensive care unit.
The intra- and interobserver ICCs for the HC and depths of INS, SF, POF, and CF were >0.90, indicating good reliability. Table 2 presents the interobserver agreement and intra/interobserver reliability for ultrasonographic measurements of HC and depths of INS, SF, POF, and CF.
Table 2
Parameters | Mean difference (95% CI) (mm) |
95% LOA (mm) | Intraobserver ICC (95% CI) |
Interobserver ICC (95% CI) |
---|---|---|---|---|
HC | −1.70 (−6.04–2.64) | −24.50–21.10 | 0.98 (0.97–0.99) | 0.97 (0.94–0.98) |
INS | 0.29 (−0.16–0.74) | −2.08–2.66 | 0.96 (0.93−0.98) | 0.95 (0.90–0.98) |
SF | −0.43 (−0.70 to −0.15) | −2.34–0.54 | 0.98 (0.97–0.99) | 0.97 (0.95–0.98) |
POF | −0.05 (−0.36–0.26) | −1.45–1.42 | 0.98 (0.97–0.99) | 0.97 (0.95–0.98) |
CF | −0.30 (−0.70 to −0.10) | −2.40–1.80 | 0.97 (0.95–0.99) | 0.96 (0.92–0.98) |
HC, head circumference; INS, insula; SF, sylvian fissure; POF, parieto-occipital fissure; CF, calcarine fissure; CI, confidence interval; LOA, limits of agreement; ICC, intraclass correlation coefficient.
HC and depths of INS, SF, POF, and CF were normally distributed (P=0.118, P=0.200, P=0.073, P=0.166, and P=0.200, respectively). The relationship of depths of INS, SF, POF, and CF to GA is shown in Figure 5. The individual measurements of fetal cranial parameters for predicting FCM are shown in Table 3. An exponential regression equation was yielded to predict FCM: predicted gestational week of FCM (weeks) = 11.16× exp (0.003× fTCP) (P<0.001), standard error of estimate, 0.67 weeks (Figure 6). The mean model standard error for prediction of the GA of FCM was ±4.7 days. The correlation between FCM and fTCP was excellent (adjusted R2=0.973). The fTCP increased with increasing GA.
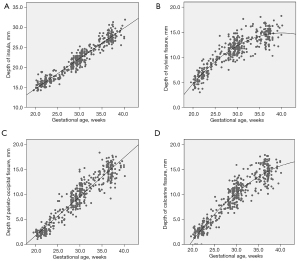
Table 3
Parameters for predicting fetal cerebral maturation | Formula | R2 | Standard error (days) | P |
---|---|---|---|---|
HC | 9.996*exp (0.004*HC) | 0.968 | 7.84 | <0.001 |
INS | −4.332+1.884*INS−0.015*INS2 | 0.934 | 10.08 | <0.001 |
SF | 16.17*exp (0.052*SF) | 0.815 | 18.41 | <0.001 |
POF | 20.09*exp (0.040*POF) | 0.900 | 15.40 | <0.001 |
CF | 20.16*exp (0.039*CF) | 0.877 | 15.26 | <0.001 |
HC, head circumference; INS, insula; SF, sylvian fissure; POF, parieto-occipital fissure; CF, calcarine fissure.
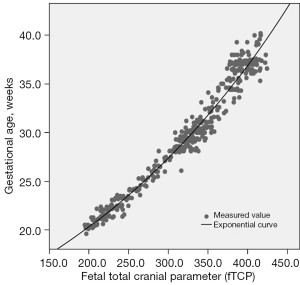
The predictive model then underwent external validation testing to assess 214 normal-growth fetuses from Center 2. The model was found to well predict FCM (standard error of estimate, 0.97±0.81 weeks).
Among the 124 fetuses with FGR, the predictive model showed that FCM was significantly delayed (2.10±1.31 weeks, P<0.001), considering the GA calculated with first-trimester ultrasound. FCM, which was expressed as GA, was similar to sonographic age, with a difference of 0.75±0.79 weeks. The predictive model showed that FCM was delayed 1.38±1.06 weeks in early-onset FGR fetuses and 2.58±1.47 weeks in late-onset FGR fetuses.
Discussion
Principal findings
The fetal brain maturation predictive model could help identify fetuses with abnormal or delayed brain development, thus enabling close monitoring and clinical intervention after birth. In this analysis, we confirmed that measuring fetal brain parameters to predict brain maturation was a valid approach, and the predictive models developed in this study were found to have a good predictive value and can be used in clinical settings to allow effective investigation of FCM.
Main results
Fetal brain development occurs during the entire pregnancy and mainly during the second half of pregnancy. Early in fetal life, the surface of the fetal brain is very smooth, and the cortical deep primary and more superficial secondary infoldings appear gradually, thus drastically changing the brain shape from a largely smooth to a complex convoluted one (1). Cortical folding of the neocortex is initiated at around 20 weeks and drastically changes the brain shape throughout the third trimester (1).
The earliest cerebral fissure observed using MRI is the SF, which appears at 16 gestational weeks, followed by the POF at 18 weeks (1). Some of these sulci can be used as landmarks for certain GAs. Previous study has suggested that the INS, SF, POF, and CF increase in depth with increasing gestation between 19 and 30 weeks (15); thus, we could establish a predictive model based on the intracranial structure. During ultrasound screening, we found that the CF could usually be visualized at 20 gestational weeks, presenting as a tiny dot that deepened gradually during the second and third trimesters. In the training dataset, the CF was identified with a detection rate of 96.5%.
The findings of our study indicate the feasibility and efficacy of developing a predictive model for assessing fetal brain maturation. The predictive model was established from measurements performed in standard ultrasound planes for biometric measurements, and landmarks for measurements were easy to identify; therefore, we can evaluate fetal maturation during routine scanning, with excellent predictive accuracy. The reported fTMS was developed using MRI for the evaluation of FCM, and the standard error of the model for the calculation of fetal GA from the visual fTMS scale was 4.8 days (18). Previous study also showed that fetal cerebral cortical folding measures could be used to predict GA in the third trimester, with a mean absolute error of 0.43±0.45 weeks (27). Our results are consistent with these two studies; however, these two approaches were established using MRI, which is not routinely performed in daily prenatal care.
Clinical implications
The fTMS has also been confirmed to be sensitive to differences in brain maturation between fetuses with isolated congenital heart disease and healthy controls (2). The fTMS is an MRI model and included different criteria such as frontal and occipital cortex, insular cortex, germinal matrix, myelination, and temporal sulci. We developed the score using measurements of sulci and HC by ultrasound. The fTMS is highly reproducible and has high interrater reliability (18). The performance of these two models were similar. The predictive model established in this study used ultrasound images that are easily obtained in prenatal care and can be applied in routine clinical settings.
Prior studies support prenatal neurodevelopmental vulnerability in fetuses with FGR by showing decreased brain volumes (28,29), reduced frontal lobe growth (30), and abnormal metabolic ratios using MRI (5,31). FGR is associated with lower neurobehavioral test scores after birth (32,33), and gyrification development across cortical areas in the brain conveys prematurity effects on adult IQ critical for cognitive performance after premature birth (34). Therefore, we speculate that gyrification could contribute to impaired cognitive performance in fetuses with growth restriction and that intracranial parameters could be used to predict FCM. In our study, the fTCP was calculated as the sum of HC and depths of INS, SF, POF, and CF. Our data indicate that this quantitative predictive model is sensitive to delays in brain maturation that are present during the second and third trimesters in fetuses with FGR. The external validity of the main findings of this study was pretty well. The model is applicable to the Asian population studied.
Research implications
This quantitative predictive model is a valuable tool to assess FCM. However, the relationship between the predictive model and neurodevelopmental outcome is not performed in this study. Future studies can be performed to evaluate the association between predictive models and neurodevelopmental outcomes.
Strengths and limitations
Our study has several strengths. First, the predictive model can be applied directly and routinely in clinical settings. Second, for normal fetuses, MRI or postnatal ultrasonography was performed to evaluate for and confirm normal prenatal development. Third, the cranial parameters used were highly reproducible and had high interobserver reliability; thus, the reliability of the predictive model was excellent. Previous literature has also demonstrated that evaluation of cortical development and the corpus callosum could detect those small fetuses with brain reorganization (35) which may benefit from early intervention, including paying attention to maternal psychological health (36), supporting health care teams who care for the infants (37), and so on. The findings of this study support the use of neurosonography for assessing neurodevelopment in appropriate growth and FGR fetuses. The predictive model of this research can be applied directly and routinely in clinical settings and to identify the fetuses with delayed cerebral maturation. Pediatricians and care providers may provide specific developmental supports to FGR infants.
Previous MRI studies have demonstrated that cortical morphometry was significantly different in late-onset small GA fetuses and was associated with poorer neurobehavioral performance compared with controls (38). However, there is no follow up of neurodevelopmental outcome in FGR fetuses, which is a main limitation of our study. In addition, cases with no follow-up were left out of the study, which may have led to selection bias. A large sample study is needed to evaluate the association between predictive models and long-term neurodevelopmental outcomes.
Conclusions
The predictive performance of the model developed in this study for FCM was excellent. It may serve as a valuable tool to effectively investigate FCM during clinical implementation. This quantitative predictive model is sensitive to delays in cerebral maturation that occur during the second and third trimesters in fetuses with FGR.
Acknowledgments
We are grateful to Dr. Hua-Song Cai (Department of Medical Imaging, The First Affiliated Hospital of Sun Yat-sen University, Guangzhou, China) for his help with the preparation of the postnatal screening for infants.
Funding: This study was supported by a research grant from
Footnote
Reporting Checklist: The authors have completed the TRIPOD reporting checklist. Available at https://qims.amegroups.com/article/view/10.21037/qims-23-786/rc
Conflicts of Interest: All authors have completed the ICMJE uniform disclosure form (available at https://qims.amegroups.com/article/view/10.21037/qims-23-786/coif). The authors have no conflicts of interest to declare.
Ethical Statement: The authors are accountable for all aspects of the work in ensuring that questions related to the accuracy or integrity of any part of the work are appropriately investigated and resolved. Ethics Committees of The First Affiliated Hospital of Sun Yat-sen University and Dalian Municipal Women and Children's Medical Center approved this study. The study was conducted in accordance with the Declaration of Helsinki (as revised in 2013). Written informed consent was obtained from participants’ parents or legal guardians before screening.
Open Access Statement: This is an Open Access article distributed in accordance with the Creative Commons Attribution-NonCommercial-NoDerivs 4.0 International License (CC BY-NC-ND 4.0), which permits the non-commercial replication and distribution of the article with the strict proviso that no changes or edits are made and the original work is properly cited (including links to both the formal publication through the relevant DOI and the license). See: https://creativecommons.org/licenses/by-nc-nd/4.0/.
References
- Glenn OA. Normal development of the fetal brain by MRI. Semin Perinatol 2009;33:208-19. [Crossref] [PubMed]
- Jaimes C, Rofeberg V, Stopp C, Ortinau CM, Gholipour A, Friedman KG, Tworetzky W, Estroff J, Newburger JW, Wypij D, Warfield SK, Yang E, Rollins CK. Association of Isolated Congenital Heart Disease with Fetal Brain Maturation. AJNR Am J Neuroradiol 2020;41:1525-31. [Crossref] [PubMed]
- Kelly CJ, Christiaens D, Batalle D, Makropoulos A, Cordero-Grande L, Steinweg JK, O'Muircheartaigh J, Khan H, Lee G, Victor S, Alexander DC, Zhang H, Simpson J, Hajnal JV, Edwards AD, Rutherford MA, Counsell SJ. Abnormal Microstructural Development of the Cerebral Cortex in Neonates With Congenital Heart Disease Is Associated With Impaired Cerebral Oxygen Delivery. J Am Heart Assoc 2019;8:e009893. [Crossref] [PubMed]
- Paladini D, Finarelli A, Donarini G, Parodi S, Lombardo V, Tuo G, Birnbaum R. Frontal lobe growth is impaired in fetuses with congenital heart disease. Ultrasound Obstet Gynecol 2021;57:776-82. [Crossref] [PubMed]
- Simões RV, Muñoz-Moreno E, Cruz-Lemini M, Eixarch E, Bargalló N, Sanz-Cortés M, Gratacós E. Brain metabolite alterations in infants born preterm with intrauterine growth restriction: association with structural changes and neurodevelopmental outcome. Am J Obstet Gynecol 2017;216:62.e1-62.e14. [Crossref] [PubMed]
- Mazzocco MM, Hagerman RJ, Cronister-Silverman A, Pennington BF. Specific frontal lobe deficits among women with the fragile X gene. J Am Acad Child Adolesc Psychiatry 1992;31:1141-8. [Crossref] [PubMed]
- Baburamani AA, Vontell RT, Uus A, Pietsch M, Patkee PA, Wyatt-Ashmead J, Chin-Smith EC, Supramaniam VG, Donald Tournier J, Deprez M, Rutherford MA. Assessment of radial glia in the frontal lobe of fetuses with Down syndrome. Acta Neuropathol Commun 2020;8:141. [Crossref] [PubMed]
- Sileo FG, Di Mascio D, Rizzo G, Caulo M, Manganaro L, Bertucci E, Masmejan S, Liberati M, D'Amico A, Nappi L, Buca D, Van Mieghem T, Khalil A, D'Antonio F. Role of prenatal magnetic resonance imaging in fetuses with isolated agenesis of corpus callosum in the era of fetal neurosonography: A systematic review and meta-analysis. Acta Obstet Gynecol Scand 2021;100:7-16. [Crossref] [PubMed]
- Morton SU, Maleyeff L, Wypij D, Yun HJ, Newburger JW, Bellinger DC, Roberts AE, Rivkin MJ, Seidman JG, Seidman CE, Grant PE, Im K. Abnormal Left-Hemispheric Sulcal Patterns Correlate with Neurodevelopmental Outcomes in Subjects with Single Ventricular Congenital Heart Disease. Cereb Cortex 2020;30:476-87. [Crossref] [PubMed]
- Murray E, Fernandes M, Fazel M, Kennedy SH, Villar J, Stein A. Differential effect of intrauterine growth restriction on childhood neurodevelopment: a systematic review. BJOG 2015;122:1062-72. [Crossref] [PubMed]
- Bar J, Weiner E, Levy M, Gilboa Y. The thrifty phenotype hypothesis: The association between ultrasound and Doppler studies in fetal growth restriction and the development of adult disease. Am J Obstet Gynecol MFM 2021;3:100473. [Crossref] [PubMed]
- Spinelli M, Sica C, Ghezzi F, Cromi A, Surbek D, Raio L. Nomograms of the Fetal Sylvian Fissure and Insular Lobe throughout Gestation: A Multicentric, Ultrasonographic Cross-Sectional Study. Fetal Diagn Ther 2019;45:325-31. [Crossref] [PubMed]
- Gindes L, Malach S, Weisz B, Achiron R, Leibovitz Z, Weissmann-Brenner A. Measuring the perimeter and area of the Sylvian fissure in fetal brain during normal pregnancies using 3-dimensional ultrasound. Prenat Diagn 2015;35:1097-105. [Crossref] [PubMed]
- Poon LC, Sahota DS, Chaemsaithong P, Nakamura T, Machida M, Naruse K, Wah YM, Leung TY, Pooh RK. Transvaginal three-dimensional ultrasound assessment of Sylvian fissures at 18-30 weeks' gestation. Ultrasound Obstet Gynecol 2019;54:190-8. [Crossref] [PubMed]
- Alonso I, Borenstein M, Grant G, Narbona I, Azumendi G. Depth of brain fissures in normal fetuses by prenatal ultrasound between 19 and 30 weeks of gestation. Ultrasound Obstet Gynecol 2010;36:693-9. [Crossref] [PubMed]
- Fogliarini C, Chaumoitre K, Chapon F, Fernandez C, Lévrier O, Figarella-Branger D, Girard N. Assessment of cortical maturation with prenatal MRI. Part I: Normal cortical maturation. Eur Radiol 2005;15:1671-85. [Crossref] [PubMed]
- Levine D, Barnes PD. Cortical maturation in normal and abnormal fetuses as assessed with prenatal MR imaging. Radiology 1999;210:751-8. [Crossref] [PubMed]
- Vossough A, Limperopoulos C, Putt ME, du Plessis AJ, Schwab PJ, Wu J, Gee JC, Licht DJ. Development and validation of a semiquantitative brain maturation score on fetal MR images: initial results. Radiology 2013;268:200-7. [Crossref] [PubMed]
- Pittet MP, Vasung L, Huppi PS, Merlini L. Newborns and preterm infants at term equivalent age: A semi-quantitative assessment of cerebral maturity. Neuroimage Clin 2019;24:102014. [Crossref] [PubMed]
- Bahado-Singh RO, Goncalves LF. Techniques, terminology, and indications for MRI in pregnancy. Semin Perinatol 2013;37:334-9. [Crossref] [PubMed]
- Hadlock FP, Shah YP, Kanon DJ, Lindsey JV. Fetal crown-rump length: reevaluation of relation to menstrual age (5-18 weeks) with high-resolution real-time US. Radiology 1992;182:501-5. [Crossref] [PubMed]
- Hadlock FP, Harrist RB, Sharman RS, Deter RL, Park SK. Estimation of fetal weight with the use of head, body, and femur measurements--a prospective study. Am J Obstet Gynecol 1985;151:333-7. [Crossref] [PubMed]
- Leung TN, Pang MW, Daljit SS, Leung TY, Poon CF, Wong SM, Lau TK. Fetal biometry in ethnic Chinese: biparietal diameter, head circumference, abdominal circumference and femur length. Ultrasound Obstet Gynecol 2008;31:321-7. [Crossref] [PubMed]
- Lees CC, Stampalija T, Baschat A, da Silva Costa F, Ferrazzi E, Figueras F, Hecher K, Kingdom J, Poon LC, Salomon LJ, Unterscheider J. ISUOG Practice Guidelines: diagnosis and management of small-for-gestational-age fetus and fetal growth restriction. Ultrasound Obstet Gynecol 2020;56:298-312. [Crossref] [PubMed]
- Figueras F, Gratacós E. Update on the diagnosis and classification of fetal growth restriction and proposal of a stage-based management protocol. Fetal Diagn Ther 2014;36:86-98. [Crossref] [PubMed]
- Salomon LJ, Alfirevic Z, Berghella V, Bilardo CM, Chalouhi GE, Da Silva Costa F, Hernandez-Andrade E, Malinger G, Munoz H, Paladini D, Prefumo F, Sotiriadis A, Toi A, Lee W. ISUOG Practice Guidelines (updated): performance of the routine mid-trimester fetal ultrasound scan. Ultrasound Obstet Gynecol 2022;59:840-56. [Crossref] [PubMed]
- Wu J, Awate SP, Licht DJ, Clouchoux C, du Plessis AJ, Avants BB, Vossough A, Gee JC, Limperopoulos C. Assessment of MRI-Based Automated Fetal Cerebral Cortical Folding Measures in Prediction of Gestational Age in the Third Trimester. AJNR Am J Neuroradiol 2015;36:1369-74. [Crossref] [PubMed]
- Benavides-Serralde A, Hernández-Andrade E, Fernández-Delgado J, Plasencia W, Scheier M, Crispi F, Figueras F, Nicolaides KH, Gratacós E. Three-dimensional sonographic calculation of the volume of intracranial structures in growth-restricted and appropriate-for-gestational age fetuses. Ultrasound Obstet Gynecol 2009;33:530-7. [Crossref] [PubMed]
- Caetano AC, Zamarian AC, Araujo Júnior E, Cavalcante RO, Simioni C, Silva CP, Rolo LC, Moron AF, Nardozza LM. Assessment of Intracranial Structure Volumes in Fetuses With Growth Restriction by 3-Dimensional Sonography Using the Extended Imaging Virtual Organ Computer-Aided Analysis Method. J Ultrasound Med 2015;34:1397-405. [Crossref] [PubMed]
- Makhoul IR, Soudack M, Goldstein I, Smolkin T, Tamir A, Sujov P. Sonographic biometry of the frontal lobe in normal and growth-restricted neonates. Pediatr Res 2004;55:877-83. [Crossref] [PubMed]
- Sanz-Cortes M, Egaña-Ugrinovic G, Simoes RV, Vazquez L, Bargallo N, Gratacos E. Association of brain metabolism with sulcation and corpus callosum development assessed by MRI in late-onset small fetuses. Am J Obstet Gynecol 2015;212:804.e1-8. [Crossref] [PubMed]
- Lees CC, Marlow N, van Wassenaer-Leemhuis A, Arabin B, Bilardo CM, Brezinka C, et al. 2 year neurodevelopmental and intermediate perinatal outcomes in infants with very preterm fetal growth restriction (TRUFFLE): a randomised trial. Lancet 2015;385:2162-72. [Crossref] [PubMed]
- Sanz-Cortes M, Egaña-Ugrinovic G, Zupan R, Figueras F, Gratacos E. Brainstem and cerebellar differences and their association with neurobehavior in term small-for-gestational-age fetuses assessed by fetal MRI. Am J Obstet Gynecol 2014;210:452.e1-8. [Crossref] [PubMed]
- Hedderich DM, Bäuml JG, Berndt MT, Menegaux A, Scheef L, Daamen M, Zimmer C, Bartmann P, Boecker H, Wolke D, Gaser C, Sorg C. Aberrant gyrification contributes to the link between gestational age and adult IQ after premature birth. Brain 2019;142:1255-69. [Crossref] [PubMed]
- Paules C, Miranda J, Policiano C, Crovetto F, Youssef L, Hahner N, Nakaki A, Crispi F, Gratacós E, Eixarch E. Fetal neurosonography detects differences in cortical development and corpus callosum in late-onset small fetuses. Ultrasound Obstet Gynecol 2021;58:42-7. [Crossref] [PubMed]
- Shaw E, Levitt C, Wong S, Kaczorowski J. McMaster University Postpartum Research Group. Systematic review of the literature on postpartum care: effectiveness of postpartum support to improve maternal parenting, mental health, quality of life, and physical health. Birth 2006;33:210-20. [Crossref] [PubMed]
- Raizada RD, Kishiyama MM. Effects of socioeconomic status on brain development, and how cognitive neuroscience may contribute to levelling the playing field. Front Hum Neurosci 2010;4:3.
- Egaña-Ugrinovic G, Sanz-Cortes M, Figueras F, Couve-Perez C, Gratacós E. Fetal MRI insular cortical morphometry and its association with neurobehavior in late-onset small-for-gestational-age fetuses. Ultrasound Obstet Gynecol 2014;44:322-9. [Crossref] [PubMed]