Development of a nomogram based on body composition analysis of quantitative computed tomography combined with clinical prognostic factors to predict disease-free survival after surgery and adjuvant chemotherapy in patients with gastric cancer
Introduction
Gastric cancer (GC) is the fifth most common cancer in the world and the third most common cause of cancer death (1). The early symptoms of GC are hidden, and most patients are in a progressive stage when diagnosed. The primary treatment for early- and middle-stage GC is surgical resection combined with adjuvant chemotherapy. Even with reasonable and effective treatment, the rate of postoperative recurrence and metastasis in patients ranges as high as 50–70% (2,3).
Therefore, it is essential to predict the prognosis of patients after surgery. Currently, the primary methods for postoperative reexamination of patients with GC include routine computed tomography (CT) scans and clinical prognostic factors (CPFs) such as carcinoembryonic antigen (CEA) screening. However, sometimes the results of these examinations differ from the patient’s actual condition. Unobvious in situ tumor recurrence or nonenlarged lymph node metastasis are easily missed. CPFs such as CEA can lead to false-negative and false-positive results. Therefore, it is necessary to establish a reliable prognosis model to predict the prognosis of patients with GC after surgery and provide opportunities for improving the follow-up strategy and quality of life.
The features examined in this study included bone mineral density (BMD), adipose tissue, and skeletal muscle. Most patients with cancer are prone to complications such as malnutrition and loss of muscle mass due to decreased physical activity, insufficient food intake, and catabolic disorders during treatment, resulting in changes in body composition and even cachexia (4,5), which seriously affect the prognosis and quality of life of these patients. Related studies have shown that a decrease in BMD and muscle mass and an increase in adipose tissue occur during cancer treatment, and these are strongly associated with overall survival (OS) and disease-free survival (DFS) in patients with cancer after surgery (6-9). Currently, there is a lack of relevant research on the body composition changes (BCCs) before surgery and after adjuvant chemotherapy for GC.
CT is widely used in the follow-up of patients with cancer, and imaging data are easy to obtain. Quantitative computed tomography (QCT) is an accurate and reproducible 3-dimensional imaging technique that measures changes in BMD, skeletal muscle, and adipose tissue during a patient’s treatment.
The purpose of this study was to explore the relationship between body composition analysis based on CT and DFS of patients with GC receiving surgery combined with adjuvant chemotherapy and to construct a prognostic nomogram of BCC combined with CPF to provide a dependable method for assessing the prognosis of patients with GC who undergo surgery and adjuvant chemotherapy. We present this article in accordance with the TRIPOD reporting checklist (available at https://qims.amegroups.com/article/view/10.21037/qims-23-309/rc).
Methods
This retrospective study was conducted in accordance with the Declaration of Helsinki (as revised in 2013) and was approved by the Institutional Ethics Committee of Anhui Provincial Cancer Hospital (No. 2023-YXK-01). Individual consent for this retrospective analysis was waived.
Patients
A retrospective study was conducted on patients with GC confirmed by pathology from February 2015 to June 2019 who had received surgery and chemotherapy in Anhui Provincial Cancer Hospital. Patients who met the following criteria were included in the study: (I) with complete clinical data; (II) no other primary tumors; (III) high-quality, interpretable CT follow-up images; (IV) no other treatment, except for surgery and chemotherapy for GC, performed before or after the CT scan.
Patients with GC should be followed up every 3 months for 1–2 years, every 6 months for 2–5 years, and every year after for 5 years. In this study, patients with GC were followed up mainly through outpatient, inpatient, and telephone contact. All patients were followed up until the appearance of recurrence, metastasis, or the follow-up cut-off time (June 2022) was reached. Clinical data for study enrollment included age, gender, CEA, human epidermal growth factor receptor-2 (HER2), Ki-67, clinical stage, maximum tumor diameter (MTD), number of preoperative lymph node metastases, and vessel carcinoma embolus. DFS was considered to be the time from surgery to the first local recurrence, distant metastasis, or the last follow-up.
Treatment
All operations were performed by experienced gastrointestinal surgeons. The standard surgical approach includes proximal and distal partial gastrectomy or total gastrectomy, which may be accompanied by peripheral lymph node dissection. Postoperatively, 5-fluorouracil (S-1 and capecitabine) and platinum (oxaliplatin and cisplatin) chemotherapeutic agents were administered, and patients received 1 course of chemotherapy every 3 weeks for a total of 6 courses. Chemotherapy modalities included intravenous drip or oral administration.
CT protocol
The CT image before treatment was obtained by scanning within 1 month before surgery, and the CT image after treatment was obtained by scanning 1 month after the end of adjuvant chemotherapy. CT images were analyzed before and after treatment. All patients were examined on a 64-MDCT scanner (Discovery CT 750 HD, GE HealthCare, Chicago, IL, USA). The CT scan parameters were as follows: a scan layer thickness of 5 mm, a reconstruction layer thickness of 1.25 mm, a voltage of 120 kVp, a current of 250 mA, and a 50-cm display field of view (DFOV).
CT-based body composition measurements and analysis
All CT images were transferred to a QCT Pro workstation (version 4.2.3, Mindways Software, Austin, TX, USA). According to the guidelines of the American Radiological Society for QCT measurement of BMD (10), the first lumbar vertebra (L1) and the second lumbar vertebra (L2) were used to measure BMD. On the axial, sagittal, and coronal images, the region of interest (ROI) was located at the center of the L1 and L2 vertebral bodies. The area of the ROI was about 300 mm2 and included as much cancellous bone as possible and as little cortical bone as possible. The average BMD of the L1 and L2 vertebrae was calculated using the “3D spine exam analysis” function in the QCT Pro workstation. According to previous studies, the area of adipose tissue and skeletal muscle corresponding to the middle level of the third lumbar vertebra (L3) can be used to estimate whole body fat and muscle (11,12). The total fat area (TFA), visceral fat area (VFA), and subcutaneous fat area (SFA) at the level of L3 were automatically obtained by using the “tissue composition” and “auto snake” functions in the QCT Pro workstation. The “Tissue Composition Module” function was then used to manually contour the paravertebral muscle (PMA) group, including the multifidus muscle and the erector spine muscle, to obtain the cross-sectional area of PMA. The measurement of body composition is presented in Figure 1.
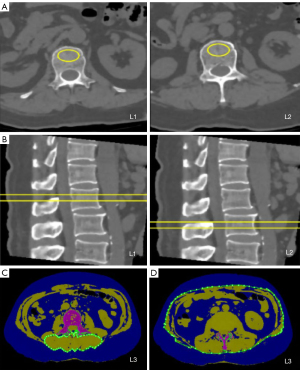
The QCT measurement of body composition was separately performed by 2 attending physicians. Due to the difference in CT scanning time interval between each patient before and after treatment, standardization was required, which involved calculating the change rate of BMD, SFA, VFA, TFA, and PMA for 120 days and expressing these as ΔBMD, ΔSFA, ΔVFA, ΔTFA, and ΔPMA, respectively. The BCC rate calculation formula was as follows: (parameterposttreatment − parameterpretreatment) × 120 d/(parameterpretreatment × timeCT actual interval) × 100%.
Statistical analysis
SPSS 26.0 (IBM Corp., Armonk, NY, USA) and R 4.2.2 software (The R Foundation for Statistical Computing) were used for statistical analysis. This study converted continuous variables into binary variables using the maximum Youden index and clinical reference range value. Kaplan-Meier analysis was used to calculate DFS and construct the survival curve. The log-rank test was used to compare the survival curves between groups. According to the median DFS, all patients were divided into a good prognosis group and a poor prognosis group. The Chi-squared test or Fisher exact test was used to determine the correlation of each variable with patient prognosis. The independent prognostic factors affecting the prognosis of patients with GC were identified using univariate and multivariate Cox regression analysis. A nomogram was constructed based on the results of multivariate Cox regression analysis (P<0.05 for variables) to predict the probability of 2-year DFS and 3-year DFS. The predictive performance of the model was assessed via receiver operating characteristic (ROC) curves, the Harrell concordance index (C-index), and decision curve analysis (DCA).
Results
In the study, 80 patients with GC who underwent surgical adjuvant chemotherapy between 2015 and 2019 were initially identified. After 20 patients were excluded (nine patients received neoadjuvant chemotherapy, one patient received radiotherapy, two patients had other primary tumors, three patients had incomplete CT images before or after treatment, and five patients had poor CT scanning quality), 60 patients were finally included in the study. Referring to the study of Ding et al. (13), we conducted a small sample study. The detailed flowchart is shown in Figure 2.
A total of 120 CT scans were performed in 60 patients, who ranged in age from 29 to 78 years old, with an average age of 57.50±9.98 years. There were 48 male patients (80%) and 12 female patients (20%). The median DFS was 55 months, and the median follow-up was 48 months. There were 29 cases of recurrence and metastasis and 31 cases of nonrecurrence and metastasis. The best critical points for ΔBMD, ΔSFA, ΔVFA, ΔTFA, and ΔPMA were −0.41%, −1.81%, −10.64%, −6.70%, and 0.83%, respectively. The correlation between each variable and DFS is shown in Table 1.
Table 1
Variable | DFS <55 months | DFS ≥55 months | Ratio (%) | P value |
---|---|---|---|---|
ΔBMD | ||||
<−0.41% | 30 | 11 | 68.33 | <0.001† |
≥−0.41% | 4 | 15 | 31.67 | |
ΔSFA | ||||
<−1.81% | 26 | 15 | 68.33 | 0.121† |
≥−1.81% | 8 | 11 | 31.67 | |
ΔVFA | ||||
<−10.64% | 23 | 12 | 58.33 | 0.568† |
≥−10.64% | 11 | 14 | 41.67 | |
ΔTFA | ||||
<−6.70% | 26 | 14 | 66.67 | 0.065† |
≥−6.70% | 8 | 12 | 33.33 | |
ΔPMA | ||||
<0.83% | 32 | 15 | 78.33 | 0.001† |
≥0.83% | 2 | 11 | 21.67 | |
Gender | ||||
Male | 27 | 21 | 80.00 | 0.896† |
Female | 7 | 5 | 20.00 | |
Age | ||||
<50 years | 5 | 5 | 16.67 | 0.733‡ |
≥50 years | 29 | 21 | 83.33 | |
CEA | ||||
<5 | 30 | 25 | 91.67 | 0.377‡ |
≥5 | 4 | 1 | 8.33 | |
Ki-67 | ||||
<50 | 8 | 5 | 21.67 | 0.689† |
≥50 | 26 | 21 | 78.33 | |
HER2 | ||||
Negative | 17 | 20 | 61.67 | 0.034† |
Positive | 17 | 6 | 38.33 | |
Vessel carcinoma embolus | ||||
No | 26 | 16 | 70.00 | 0.211† |
Yes | 8 | 10 | 30.00 | |
Lymph node metastasis | ||||
No | 6 | 12 | 30.00 | 0.017† |
Yes | 28 | 14 | 70.00 | |
Maximum tumor diameter | ||||
<5 cm | 15 | 20 | 58.33 | 0.011† |
≥5 cm | 19 | 6 | 41.67 | |
Tumor stage | ||||
I | 2 | 3 | 8.33 | 0.099‡ |
IIA | 0 | 3 | 5.00 | |
IIB | 5 | 6 | 18.33 | |
IIIA | 3 | 2 | 8.33 | |
IIIB | 7 | 10 | 28.33 | |
IIIC | 17 | 2 | 31.67 |
†, Chi-squared test; ‡, Fisher exact test. GC, gastric cancer; DFS, disease-free survival; BMD, bone mineral density; SFA, subcutaneous fat area; VFA, visceral fat area; TFA, total fat area; PMA, paravertebral muscle area; CEA, carcinoembryonic antigen; HER2, human epidermal growth factor receptor-2.
BCCs during treatment
The mean time interval between pre- and posttreatment CT scans was 133 days in 60 patients, with a median time interval of 129 days. Among them, the mean intervals of CT scan for DFS <55 months (n=34) and DFS ≥55 months (n=26) were 134 and 132 days, respectively, and the median intervals were 129 and 135 days, respectively. The intraobserver consistency of the measurements for ΔBMD, ΔSFA, ΔVFA, ΔTFA, and ΔPMA was high, with pretreatment intraclass correlation coefficients (ICCs) of 0.970, 0.908, 0.902, 0.938, and 0.953, respectively, and posttreatment ICCs of 0.914, 0.903, 0.955, 0.928, and 0.947, respectively. BCC rate ≥0 or <0 was defined as “increase” or “loss”, respectively. Table 2 shows the changes in body composition during treatment every 120 days; the rates of change for ΔBMD, ΔSFA, ΔVFA, ΔTFA, and ΔPMA were −3.86%, −23.44%, −19.57%, −22.45%, and −5.94%, respectively. There were 19 patients with increased BMD and 41 patients with decreased BMD, 15 patients with increased SFA and 45 patients with decreased SFA, 12 patients with increased VFA and 48 patients with decreased VFA, 16 patients with increased TFA and 44 patients with decreased TFA, and 14 patients with increased PMA and 46 patients with decreased PMA.
Table 2
Project | BMD (mg/cm3) | SFA (cm2) | VFA (cm2) | TFA (cm2) | PMA (cm2) |
---|---|---|---|---|---|
Pretreatment | 119.17±31.68 | 80.77±40.80 | 108.20±65.31 | 188.97±97.97 | 48.01±8.72 |
Posttreatment | 113.51±30.37 | 58.43±41.46 | 74.94±52.82 | 133.50±85.92 | 44.75±8.41 |
Change rate (%) | −3.86±9.56 | −23.44±31.75 | −19.57±38.74 | −22.45±33.33 | −5.94±8.11 |
Data are expressed as the mean ± standard deviation. GC, gastric cancer; BMD, bone mineral density; SFA, subcutaneous fat area; VFA, visceral fat area; TFA, total fat area; PMA, paravertebral muscle area.
BCCs and CPFs for survival
During treatment, BCC (including ΔBMD, ΔSFA, ΔVFA, ΔTFA, and ΔPMA) and CPF [including gender, age, CEA, Ki-67, HER2, vessel carcinoma embolus, preoperative lymph node metastasis, MTD, and the American Joint Commission on Cancer (AJCC) tumor-node-metastasis (TNM) stage] were analyzed by univariate analysis, which showed that ΔSFA, ΔVFA, ΔTFA, gender, age, CEA, Ki-67, vascular cancer thrombus, and tumor stage were not associated with DFS (P>0.05), while ΔBMD, ΔPMA, HER2, preoperative lymph node metastasis, and MTD were associated with DFS (P<0.05). The variables with P<0.05 were subjected to multivariate analysis, and the results showed that ΔBMD [hazard ratio (HR): 4.577; 95% confidence interval (CI): 1.483–14.132; P=0.008], ΔPMA (HR: 5.784; 95% CI: 1.251–26.740; P=0.025), HER2 (HR: 4.819; 95% CI: 2.201–10.549; P<0.001), and MTD (HR: 3.973; 95% CI: 1.893–8.337; P<0.001) were independent influencing factors of DFS (Table 3). The Kaplan-Meier method was used to draw the survival curve of independent influencing factors (Figure 3).
Table 3
Variable | Univariate Cox regression analysis | Multivariate Cox regression analysis | |||
---|---|---|---|---|---|
HR (95% CI) | P value | HR (95% CI) | P value | ||
ΔBMD | 5.784 (2.021–16.555) | 0.001 | 4.577 (1.483–14.132) | 0.008 | |
ΔSFA | 1.886 (0.851–4.176) | 0.118 | – | – | |
ΔVFA | 1.828 (0.889–3.757) | 0.101 | – | – | |
ΔTFA | 2.034 (0.919–4.505) | 0.080 | – | – | |
ΔPMA | 0.141 (0.034–0.592) | 0.007 | 5.784 (1.251–26.740) | 0.025 | |
Gender | 0.946 (0.412–2.173) | 0.896 | – | – | |
Age | 1.384 (0.535–3.578) | 0.503 | – | – | |
CEA | 2.453 (0.861–6.993) | 0.093 | – | – | |
Ki-67 | 1.171 (0.560–2.450) | 0.675 | – | – | |
HER2 | 2.218 (1.129–4.356) | 0.021 | 4.819 (2.201–10.549) | <0.001 | |
Vessel carcinoma embolus | 0.623 (0.282–1.376) | 0.242 | – | – | |
Lymph node metastasis | 3.064 (1.261–7.444) | 0.013 | 2.211 (0.802–6.093) | 0.125 | |
Maximum tumor diameter | 2.617 (1.322–5.178) | 0.006 | 3.973 (1.893–8.337) | <0.001 | |
Tumor stage | |||||
I | – | – | – | – | |
II | 1.005 (0.195–5.183) | 0.995 | – | – | |
III | 2.600 (0.616–10.970) | 0.193 | – | – |
DFS, disease-free survival; GC, gastric cancer; HR, hazard ratio; CI, confidence interval; BMD, bone mineral density; SFA, subcutaneous fat area; VFA, visceral fat area; TFA, total fat area; PMA, paravertebral muscle area; CEA, carcinoembryonic antigen; HER2, human epidermal growth factor receptor-2.
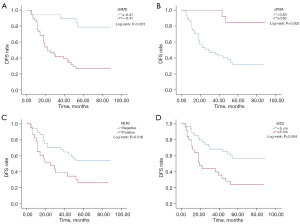
Predictive efficacy of BCCs and CPFs
An area under the curve (AUC) of the model greater than 0.7 was considered to indicate a good predictive effect. According to ROC curve analysis (Table 4), the AUCs of BCC (AUC =0.721; 95% CI: 0.577–0.865; P=0.004), CPF (AUC =0.791; 95% CI: 0.674–0.908; P<0.001), and BCC combined with CPF (AUC =0.844; 95% CI: 0.745–0.943; P<0.001) were all greater than 0.7, and BCC combined with CPF had the largest AUC, with specificity and sensitivity of 88.5% and 70.6%, respectively. The ROC curves are presented in Figure 4.
Table 4
Variable | AUC | 95% CI | P value | Sensitivity (%) | Specificity (%) |
---|---|---|---|---|---|
Overall BCC | 0.721 | 0.577–0.865 | 0.004 | 82.4 | 69.2 |
Overall CPF | 0.791 | 0.674–0.908 | <0.001 | 85.3 | 61.5 |
BCC with CPF | 0.844 | 0.745–0.943 | <0.001 | 70.6 | 88.5 |
ROC, receiver operating characteristic; BCC, body composition change; CPF, clinical prognostic factor; AUC, area under the curve; CI, confidence interval.
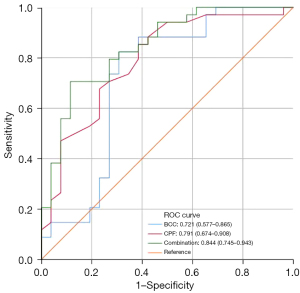
Construction of nomogram for prognosis
Prognostic nomograms were drawn based on independent influences (ΔBMD, ΔPMA, HER2, MTD) obtained from multivariate analysis. In the nomogram, each variable was assigned a score point, and the probability of occurrence of 2- and 3-year DFS was obtained by calculating the total score of all variables (Figure 5), providing a visual result for the prognosis prediction of patients with GC. The C-index of the nomogram was 0.814. ROC curves showed that the AUCs of the predicted probability of 2-year DFS and 3-year DFS were 0.879 and 0.928, respectively (Figure 6). In addition, DCA was used to evaluate whether the nomogram was helpful for devising clinical treatment strategies. The DCA of the prognosis model showed that the BCC combined with the CPF model provided markedly more net benefit than did the BCC model or the CPF model alone (Figure 7). The fitted lines of the predicted 2- and 3-year DFS in the calibration plots roughly coincided with the reference line, indicating that our constructed nomogram had a good prediction ability (Figure 8).
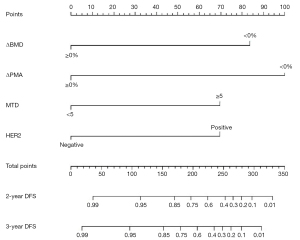
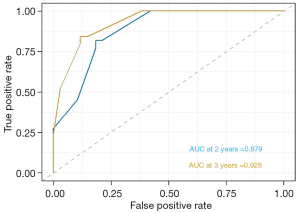
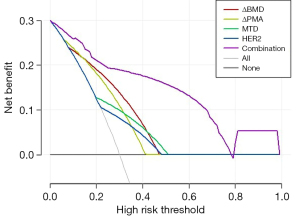
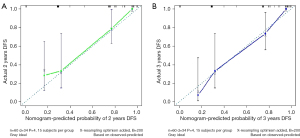
Discussion
This study demonstrated a relationship between the changes in BMD, muscle tissue, and adipose tissue and the prognosis of patients with GC through the analysis of body compositions via QCT. Among these factors, ΔBMD and ΔPMA were found to be independent influencing factors for DFS in patients with GC. Furthermore, CPF (HER2, MTD) was also an independent influencing factor of DFS. In order to predict DFS, based on this study, we built a prognostic nomogram including BCC and CPF that had a good prediction ability.
Many studies have shown that BMD loss is an independent prognostic factor for patients with cancer (14-16). Our study was similar in this regard, as ΔBMD (HR: 4.577; 95% CI: 1.483–14.132; P=0.008) was found to be a risk factor for DFS in patients with GC. During treatment, the BMD of patients with GC decreased by 3.86% every 120 days, and BMD loss was associated with a greater risk of poor prognosis in patients with GC. This may be explained by the decreased calcium absorption, vitamin D deficiency, and secondary hyperparathyroidism caused by the change in gastrointestinal physiological function after gastrectomy (17).
Patients with cancer frequently experience cachexia during treatment, which is characterized by persistent muscle loss, most commonly occurring in liver cancer, pancreatic cancer, stomach cancer, esophageal cancer, and intestinal cancer (18). Kim et al. and Wang et al. (19,20) investigated the prognosis of patients with ovarian cancer during initial treatment and patients with advanced ovarian cancer and consistently concluded that posttreatment muscle loss was an adverse factor for OS in ovarian cancer. Muscle loss also exerts numerous adverse effects on the treatment results of patients with abdominal malignant tumors. It has been proven that reduced muscle mass is closely related to severe complications after gastrectomy (21,22). Other studies have shown that sarcopenia is an independent influencing factor of OS in patients with GC (23-25). However, these studies only predicted the prognosis of GC by measuring body composition through preoperative CT images. In contrast, our study predicted the DFS of GC by analyzing the CT images of patients with GC before surgery and after adjuvant chemotherapy and calculating the rate of change in body composition during treatment. The results of this study showed that ΔPMA (HR: 5.784; 95% CI: 1.251–26.740; P=0.025) can be used as a prognostic factor for GC, and the ΔPMA was −5.94% per 120-day period. When PMA decreased during treatment, DFS was shortened in patients with GC. This result may be due to cachexia caused by reduced food intake, changes in body metabolism, and inflammation (26). Inflammation plays a crucial role in muscle loss in patients with cancer: tumor cells can secrete inflammatory factors to alter the body’s metabolic balance, promoting muscle proteolysis, and the stimulation of the hypothalamus via inflammation can produce an acute disease response with altered activity of neuronal populations that regulate appetite and metabolic processes, leading to anorexia and muscle atrophy (18). Cancer cells invade the intestinal wall, causing intestinal obstruction and affecting the absorption of food (27).
Obesity has been considered to be a significant risk factor for increased disease burden (28). In particular, white adipose tissue (WAT) acts as an active endocrine organ releasing inflammatory factors that promote the formation of the tumor microenvironment and increase tumor aggressiveness or decrease the treatment effect (29-31). Our study found that SFA, VFA, and TFA were reduced during treatment in patients with GC, with change rates per 120 days of −23.44%, −19.57%, and −22.45%, respectively. The reasons for this result may be as follows: (I) proinflammatory cytokines such as interleukin-6 and tumor necrosis factor alpha can mediate chronic inflammation leading to lipolysis (mainly WAT) (32); (II) the diet of patients with GC change after surgery, and a light diet is helpful for fat consumption; (III) the intestinal physiology is altered after gastrectomy, and rapid intestinal transit and changes in gastrointestinal hormone levels can lead to fat loss (33). Related studies have shown that obesity is closely related to the prognosis of cancer (34). However, in this study, univariate Cox regression analysis showed that the P values of ΔSFA, ΔVFA, and ΔTFA were 0.118, 0.101, and 0.080, respectively. Therefore, SFA loss, VFA loss, and TFA loss were not independent prognostic factors for DFS in patients with GC.
Chemotherapy drugs (such as platinum and 5-fluorouracil) also have certain toxic effects on the digestive system (35,36), affecting the absorption of key nutrients and leading to changes in body composition.
HER2 is related to the proliferation, migration, differentiation, apoptosis, and adhesion of tumor cells (37) and can be used as a therapeutic target for tumors. The results of HER2 studies on the prognosis of GC are inconsistent. In some studies, HER2 has been shown to be an independent influencing factor of OS and recurrence-free survival in GC (38,39). The survival period of patients expressing HER2 is shorter than that of HER2-negative patients. Other studies have reported that HER2 is not associated with the prognosis of GC (40,41). However, these studies also showed that MTD could affect the prognosis of GC. In our study, both HER2 positivity and MTD were closely related to DFS in GC.
Based on the above findings, we attempted to combine BCCs (ΔBMD, ΔPMA) with CPFs (HER2, MTD) to predict the prognosis. The results of our study showed that the AUC of BCC combined with CPF to predict the prognosis was 0.844, which was superior to that of BCC (AUC =0.721) or CPF (AUC =0.791) alone. Nomograms typically achieve excellent results in predicting the prognosis of various cancers (42-44). We developed a prognostic nomogram model combining BCC with CPF, which showed high accuracy and discrimination in predicting the survival probability of patients with GC, with a C-index of 0.814, and the AUC for predicting DFS in 2 and 3 years was 0.879 and 0.928, respectively. Figure 8 showed that the DFS probabilities predicted by the nomogram were consistent with the actual DFS probabilities. In addition, the model combined with BCC and CPF had the maximum net benefits on the threshold probability of the decision curve, which was better than that of either BCC or CPF alone.
Clinically, BCC (ΔBMD, ΔPMA) and CPF (HER2, MTD) are easily accessible prognostic indicators. Clinicians can conduct systematic scoring according to the nomogram we constructed to obtain the probability of occurrence of 2- and 3-year DFS in patients with GC, which helps develop effective and individualized treatment plans for patients with GC to improve the quality of life and prolong survival.
Limitations
Our study has several limitations: (I) we employed retrospective design with a small sample size. The generalizability of the prognostic nomogram needs to confirmed in a multicenter, large-cohort study and externally validated. (II) In this study, body composition was measured only at a single horizontal plane of the L1, L2, and L3 vertebrae. (III) It is impossible to dynamically measure the impact of daily food intake and physical activity on patients’ BCC. Despite some limitations, the patients included in this study underwent similar treatment methods, had complete clinical data, and had good-quality CT images before and after treatment. To our knowledge, among the many studies on the body composition of GC, this is the first to examine the rate of change in body composition before surgery and after adjuvant chemotherapy as calculated from CT images and to combine this with CPF to predict DFS.
Conclusions
ΔBMD, ΔPMA, HER2, and MDT are independent prognostic factors for GC. The prognostic nomogram of BCC combined with CPF had a good predictive performance for DFS. Our findings may help to formulate clinical treatment strategies and improve the prognosis of patients with GC.
Acknowledgments
We sincerely thank all the patients with cancer who participated in this study.
Funding: This study was supported by
Footnote
Reporting Checklist: The authors have completed the TRIPOD reporting checklist. Available at https://qims.amegroups.com/article/view/10.21037/qims-23-309/rc
Conflicts of Interest: All authors have completed the ICMJE uniform disclosure form (available at https://qims.amegroups.com/article/view/10.21037/qims-23-309/coif). J.D. reports that this study was supported by the Key Specialties in Clinical Medicine Construction Funding Project of Anhui Province (No. 2019sjlczdzk), the Key Research and Development Projects of Anhui Province (No. 2022e07020008), and the National Cancer Center Climbing Foundation for Clinical Research (No. NCC201912B03). The other authors have no conflicts of interest to declare.
Ethical Statement: The authors are accountable for all aspects of the work in ensuring that questions related to the accuracy or integrity of any part of the work are appropriately investigated and resolved. This retrospective study was conducted in accordance with the Declaration of Helsinki (as revised in 2013) and was approved by the Institutional Ethics Committee of Anhui Provincial Cancer Hospital (No. 2023-YXK-01). Individual consent for this retrospective analysis was waived.
Open Access Statement: This is an Open Access article distributed in accordance with the Creative Commons Attribution-NonCommercial-NoDerivs 4.0 International License (CC BY-NC-ND 4.0), which permits the non-commercial replication and distribution of the article with the strict proviso that no changes or edits are made and the original work is properly cited (including links to both the formal publication through the relevant DOI and the license). See: https://creativecommons.org/licenses/by-nc-nd/4.0/.
References
- Smyth EC, Nilsson M, Grabsch HI, van Grieken NC, Lordick F. Gastric cancer. Lancet 2020;396:635-48. [Crossref] [PubMed]
- Hu Y, Pan X, Nie M, Liu Y, Zou X, Liu S, Liu Q, Wang R, Zhang L. A clinical study of Yiqi Huayu Jiedu decoction reducing the risk of postoperative gastric cancer recurrence and metastasis: Study protocol for a randomized controlled trail. Medicine (Baltimore) 2020;99:e21775. [Crossref] [PubMed]
- Xie H, Lu Q, Wang H, Zhu X, Guan Z. Two postoperative chemotherapies for gastric cancer: FOLFOX4 vs. TPF. Oncol Lett 2019;17:933-6. [PubMed]
- Cereda E, Turri A, Klersy C, Cappello S, Ferrari A, Filippi AR, Brugnatelli S, Caraccia M, Chiellino S, Borioli V, Monaco T, Stella GM, Arcaini L, Benazzo M, Grugnetti G, Pedrazzoli P, Caccialanza R. Whey protein isolate supplementation improves body composition, muscle strength, and treatment tolerance in malnourished advanced cancer patients undergoing chemotherapy. Cancer Med 2019;8:6923-32. [Crossref] [PubMed]
- Arends J, Bachmann P, Baracos V, Barthelemy N, Bertz H, Bozzetti F, et al. ESPEN guidelines on nutrition in cancer patients. Clin Nutr 2017;36:11-48. [Crossref] [PubMed]
- Meister FA, Verhoeven S, Mantas A, Liu WJ, Jiang D, Heij L, Heise D, Bruners P, Lang SA, Ulmer TF, Neumann UP, Bednarsch J, Czigany Z. Osteopenia is associated with inferior survival in patients undergoing partial hepatectomy for hepatocellular carcinoma. Sci Rep 2022;12:18316. [Crossref] [PubMed]
- Chakedis J, Spolverato G, Beal EW, Woelfel I, Bagante F, Merath K, Sun SH, Chafitz A, Galo J, Dillhoff M, Cloyd J, Pawlik TM. Pre-operative Sarcopenia Identifies Patients at Risk for Poor Survival After Resection of Biliary Tract Cancers. J Gastrointest Surg 2018;22:1697-708. [Crossref] [PubMed]
- Dong QT, Cai HY, Zhang Z, Zou HB, Dong WX, Wang WB, Song HN, Luo X, Chen XL, Huang DD. Influence of body composition, muscle strength, and physical performance on the postoperative complications and survival after radical gastrectomy for gastric cancer: A comprehensive analysis from a large-scale prospective study. Clin Nutr 2021;40:3360-9. [Crossref] [PubMed]
- Blanc-Durand P, Campedel L, Mule S, Jegou S, Luciani A, Pigneur F, Itti E. Prognostic value of anthropometric measures extracted from whole-body CT using deep learning in patients with non-small-cell lung cancer. Eur Radiol 2020;30:3528-37. [Crossref] [PubMed]
- ACR-SPRSSR practice guideline for the performance of quantitative computed tomography (QCT) bone densitometry. 2014. Available online: https://www.acr.org/-/media/ACR/Files/Practice-Parameters/QCT
- Mourtzakis M, Prado CM, Lieffers JR, Reiman T, McCargar LJ, Baracos VE. A practical and precise approach to quantification of body composition in cancer patients using computed tomography images acquired during routine care. Appl Physiol Nutr Metab 2008;33:997-1006. [Crossref] [PubMed]
- Shen W, Punyanitya M, Wang Z, Gallagher D, St-Onge MP, Albu J, Heymsfield SB, Heshka S. Total body skeletal muscle and adipose tissue volumes: estimation from a single abdominal cross-sectional image. J Appl Physiol (1985) 2004;97:2333-8. [Crossref] [PubMed]
- Ding P, Guo H, He X, Sun C, Lowe S, Bentley R, Zhou Q, Yang P, Tian Y, Liu Y, Yang L, Zhao Q. Effect of skeletal muscle loss during neoadjuvant imatinib therapy on clinical outcomes in patients with locally advanced GIST. BMC Gastroenterol 2022;22:399. [Crossref] [PubMed]
- Takahashi K, Nishikawa K, Furukawa K, Tanishima Y, Ishikawa Y, Kurogochi T, Yuda M, Tanaka Y, Matsumoto A, Mitsumori N, Ikegami T. Prognostic Significance of Preoperative Osteopenia in Patients Undergoing Esophagectomy for Esophageal Cancer. World J Surg 2021;45:3119-28. [Crossref] [PubMed]
- Watanabe J, Saitsu A, Miki A, Kotani K, Sata N. Prognostic value of preoperative low bone mineral density in patients with digestive cancers: a systematic review and meta-analysis. Arch Osteoporos 2022;17:33. [Crossref] [PubMed]
- Tamura S, Ashida R, Sugiura T, Okamura Y, Ito T, Yamamoto Y, Ohgi K, Uesaka K. The prognostic impact of skeletal muscle status and bone mineral density for resected distal cholangiocarcinoma. Clin Nutr 2021;40:3552-8. [Crossref] [PubMed]
- Oh HJ, Yoon BH, Ha YC, Suh DC, Lee SM, Koo KH, Lee YK. The change of bone mineral density and bone metabolism after gastrectomy for gastric cancer: a meta-analysis. Osteoporos Int 2020;31:267-75. [Crossref] [PubMed]
- Baracos VE, Martin L, Korc M, Guttridge DC, Fearon KCH. Cancer-associated cachexia. Nat Rev Dis Primers 2018;4:17105. [Crossref] [PubMed]
- Kim SI, Yoon S, Kim TM, Cho JY, Chung HH, Song YS. Prognostic implications of body composition change during primary treatment in patients with ovarian cancer: A retrospective study using an artificial intelligence-based volumetric technique. Gynecol Oncol 2021;162:72-9. [Crossref] [PubMed]
- Wang X, Zhang C, Cao F, Wang CB, Dong JN, Wang ZH. Nomogram of Combining CT-Based Body Composition Analyses and Prognostic Inflammation Score: Prediction of Survival in Advanced Epithelial Ovarian Cancer Patients. Acad Radiol 2022;29:1394-403. [Crossref] [PubMed]
- Shi B, Liu S, Chen J, Liu J, Luo Y, Long L, Lan Q, Zhang Y. Sarcopenia is Associated with Perioperative Outcomes in Gastric Cancer Patients Undergoing Gastrectomy. Ann Nutr Metab 2019;75:213-22. [Crossref] [PubMed]
- Lan Q, Guan X, Lu S, Yuan W, Jiang Z, Lin H, Long L. Radiomics in Addition to Computed Tomography-Based Body Composition Nomogram May Improve the Prediction of Postoperative Complications in Gastric Cancer Patients. Ann Nutr Metab 2022;78:316-27. [Crossref] [PubMed]
- Bir Yucel K, Karabork Kilic AC, Sutcuoglu O, Yazıcı O, Aydos U, Kilic K, Özdemir N. Effects of Sarcopenia, Myosteatosis, and the Prognostic Nutritional Index on Survival in Stage 2 and 3 Gastric Cancer Patients. Nutr Cancer 2023;75:368-75. [Crossref] [PubMed]
- Eo W, Kwon J, An S, Lee S, Kim S, Nam D, Han GY, Choi SI, Chung HY. Clinical Significance of Paraspinal Muscle Parameters as a prognostic factor for survival in Gastric Cancer Patients who underwent Curative Surgical Resection. J Cancer 2020;11:5792-801. [Crossref] [PubMed]
- Zhou D, Zhang Y, Gao X, Yang J, Li G, Wang X. Long-Term Outcome in Gastric Cancer Patients with Different Body Composition Score Assessed via Computed Tomography. J Invest Surg 2021;34:875-82. [Crossref] [PubMed]
- Nishikawa H, Goto M, Fukunishi S, Asai A, Nishiguchi S, Higuchi K. Cancer Cachexia: Its Mechanism and Clinical Significance. Int J Mol Sci 2021;22:8491. [Crossref] [PubMed]
- Kim BK, Hong SP, Heo HM, Kim JY, Hur H, Lee KY, Cheon JH, Kim TI, Kim WH. Endoscopic stenting is not as effective for palliation of colorectal obstruction in patients with advanced gastric cancer as emergency surgery. Gastrointest Endosc 2012;75:294-301. [Crossref] [PubMed]
- Smith KB, Smith MS. Obesity Statistics. Prim Care 2016;43:121-35. ix. [Crossref] [PubMed]
- Pallegar NK, Christian SL. Adipocytes in the Tumour Microenvironment. Adv Exp Med Biol 2020;1234:1-13. [Crossref] [PubMed]
- Zhang Q, Song MM, Zhang X, Ding JS, Ruan GT, Zhang XW, Liu T, Yang M, Ge YZ, Tang M, Li XR, Qian L, Song CH, Xu HX, Shi HP. Association of systemic inflammation with survival in patients with cancer cachexia: results from a multicentre cohort study. J Cachexia Sarcopenia Muscle 2021;12:1466-76. [Crossref] [PubMed]
- Grenader T, Waddell T, Peckitt C, Oates J, Starling N, Cunningham D, Bridgewater J. Prognostic value of neutrophil-to-lymphocyte ratio in advanced oesophago-gastric cancer: exploratory analysis of the REAL-2 trial. Ann Oncol 2016;27:687-92. [Crossref] [PubMed]
- Han J, Meng Q, Shen L, Wu G. Interleukin-6 induces fat loss in cancer cachexia by promoting white adipose tissue lipolysis and browning. Lipids Health Dis 2018;17:14. [Crossref] [PubMed]
- Lee SJ, Kim JY, Ha TK, Choi YY. Changes in lipid indices and body composition one year after laparoscopic gastrectomy: a prospective study. Lipids Health Dis 2018;17:113. [Crossref] [PubMed]
- Pischon T, Nimptsch K. Obesity and Risk of Cancer: An Introductory Overview. Recent Results Cancer Res 2016;208:1-15. [Crossref] [PubMed]
- Humber CE, Tierney JF, Symonds RP, Collingwood M, Kirwan J, Williams C, Green JA. Chemotherapy for advanced, recurrent or metastatic endometrial cancer: a systematic review of Cochrane collaboration. Ann Oncol 2007;18:409-20. [Crossref] [PubMed]
- Kodera Y. More than 6 months of postoperative adjuvant chemotherapy results in loss of skeletal muscle: a challenge to the current standard of care. Gastric Cancer 2015;18:203-4. [Crossref] [PubMed]
- Gravalos C, Jimeno A. HER2 in gastric cancer: a new prognostic factor and a novel therapeutic target. Ann Oncol 2008;19:1523-9. [Crossref] [PubMed]
- Cho JH, Lim JY, Cho JY. Survival analysis based on human epidermal growth factor 2 status in stage II-III gastric cancer. World J Gastroenterol 2017;23:7407-14. [Crossref] [PubMed]
- Liu M, Sui LY, Yin XP, Wang JN, Li G, Song J, Ji Q. Deep learning for predicting the human epidermal growth factor receptor 2 status of breast cancer liver metastases based on contrast-enhanced computed tomography: a development and validation study. Quant Imaging Med Surg 2023;13:2837-45. [Crossref] [PubMed]
- Jiang W, Jin Z, Zhou F, Cui J, Wang L, Wang L. High co-expression of Sp1 and HER-2 is correlated with poor prognosis of gastric cancer patients. Surg Oncol 2015;24:220-5. [Crossref] [PubMed]
- Kim H, Seo S, Kim K, Park YH, An M, Baik H, Choi C, Oh S. Prognostic significance of Human epidermal growth factor receptor-2 expression in patients with resectable gastric adenocarcinoma. World J Surg Oncol 2019;17:122. [Crossref] [PubMed]
- Huang L, Balavarca Y, van der Geest L, Lemmens V, Van Eycken L, De Schutter H, Johannesen TB, Zadnik V, Primic-Žakelj M, Mägi M, Grützmann R, Besselink MG, Schrotz-King P, Brenner H, Jansen L. Development and validation of a prognostic model to predict the prognosis of patients who underwent chemotherapy and resection of pancreatic adenocarcinoma: a large international population-based cohort study. BMC Med 2019;17:66. [Crossref] [PubMed]
- Rose PG, Java JJ, Salani R, Geller MA, Secord AA, Tewari KS, et al. Nomogram for Predicting Individual Survival After Recurrence of Advanced-Stage, High-Grade Ovarian Carcinoma. Obstet Gynecol 2019;133:245-54. [Crossref] [PubMed]
- Zhou D, Wan J, Luo J. Construction and Validation of Prognostic Markers of Liver Cancer Based on Autophagy Genes. Anticancer Agents Med Chem 2021;21:1921-30. [Crossref] [PubMed]