A model for predicting lymph node metastasis of thyroid carcinoma: a multimodality convolutional neural network study
Introduction
With an estimated 19.3 million patients with thyroid cancer newly diagnosed each year, thyroid cancer is a disease which severely threatens human health (1). Papillary thyroid carcinoma (PTC) is the most common variant among the thyroid malignancies. For the early detection of PTC, ultrasound has become an important thyroid imaging tool due to its advantages of being noninvasive, radiation-free, and cost-effective. Moreover, careful evaluation of cervical lymph node metastasis (LNM) preoperatively is essential for the surgeon to plan further surgical therapy (2). The diagnostic sensitivity of LNM from cervical ultrasound examination or computed tomography is generally insufficient, especially for central compartment metastasis (3). Therefore, accurate assessment of LNM of PTC in the early stage based on ultrasound images is urgently needed.
Multiple studies have confirmed that the observed B-mode and contrast-enhanced ultrasound (CEUS) features can be used to predict LNM in PTC, which may be related to the biological behavior of malignant nodules (4-6). However, there remain some imaging features that cannot be distinguished by human experts. Of the existing methods for predicting LNM in PTC, approaches based on radiomics features are widely used and have been proven to have certain predictive abilities in LNM (7).
Deep learning has developed rapidly in recent years, and multiple network structures have been successively proposed and applied in various fields (8). Researchers have also begun to use deep learning methods to explore medical problems (9), with the results indicating that deep learning has value as an auxiliary tool for completing medical tasks (10,11). In the prediction of cancer LNM status, an increasing number of studies have begun to use deep learning methods to conduct related research (12,13). B-mode ultrasound images have been widely used for the prediction of LNM in breast cancer. As for PTC, researchers have developed deep learning models based on B-mode, color Doppler flow imaging (CDFI), and clinical records, which have demonstrated good performance for LNM prediction in patients with PTC (14,15). With the boom of deep learning, convolutional neural network (CNN)-based methods are gaining momentum in computer-aided thyroid cancer diagnosis. However, diagnosis with single modal data involves inherent drawbacks and is not consistent with the diagnostic methods of clinicians. To our knowledge, no study has been conducted on the use of CEUS imaging and deep learning models for LNM status prediction in PTC. In this paper, we propose a multimodal neural network model combining B-mode ultrasound and CEUS that extracts the ultrasound features of B-mode and CEUS images and combines them to finally predict the LNM status of PTC. We present this article in accordance with the TRIPOD reporting checklist (available at https://qims.amegroups.com/article/view/10.21037/qims-23-318/rc).
Methods
Study population
This study consecutively selected patients from Beijing Tiantan Hospital, Capital Medical University for whom B-mode ultrasound and CEUS examination was used to collect PTC lymph node status information from August 2018 to April 2022. For each patient, the respective B-mode image and CEUS image were selected manually from as close a time as possible; that is, the two images from a given patient were time-aligned.
The inclusion criteria were as follows: (I) aged ≥18 years; (II) first diagnosed as PTC and without chemotherapy or radiation therapy before surgery; (III) B-mode ultrasound and CEUS examination performed before surgery, with complete clinical data; and (IV) unilateral lobectomy or total thyroidectomy with central or lateral lymph node dissection.
Meanwhile, the exclusion criteria were as follows: (I) incomplete relevant information; (II) poor image quality; (III) women who were pregnant; (IV) allergy to contrast agents; and (V) refusal to cooperate or undergo examination. The study was conducted in accordance with the Declaration of Helsinki (as revised in 2013) and was approved by the Medical Ethics Committee of Beijing Tiantan Hospital, Capital Medical University (No. KY 2020-007-02). All patients provided their written informed consent to participate in this study. The study flowchart is shown in Figure 1.
Instruments and methods
Apparatus: the study was conducted with Aplio i500 and Aplio i900 (Canon Medical Systems, Tokyo, Japan) color Doppler ultrasound diagnostic instruments under contrast-enhanced thyroid ultrasound conditions. Additionally, L5–14 and L4–18 linear array probes were used, with probe frequencies of 5–14 and 4–18 MHz, respectively.
Examination method: the patient was placed in a supine position, with the front of the neck fully exposed. The examinations of all patients were completed by a human expert with 15 years of work experience and 10 years of skilled operation with the abovementioned techniques. The expert was blinded to the pathological results. The thyroid conditions were preset by the machine, and suspicious nodules were continuously and multiview scanned by the human expert. CEUS was completed with pulsed inverse harmonic imaging using a low mechanical index of refraction. SonoVue (Bracco, Milan, Italy) was injected into the superficial ulnar vein via mass injection and was immediately followed by the addition of 5 mL of saline. Dynamic contrast-enhanced images were continuously observed for a minimum of 2 min and recorded on the internal hard drive of the ultrasound system. Informed consent from all participants for CEUS imaging was obtained. The gray-scale ultrasound images and the representative frames from CEUS video of the suspicious nodules were saved. Following this, the ultrasound examination of cervical lymph node metastasis (US-LNM) was performed to assess possible metastasis.
Deep learning methods
Data preprocessing
We finally selected the data of 308 nodules as the dataset for this experiment. These included data on 158 nodules with LNM and 150 nodules without LNM. In the data division stage, we divided data into a training set and test set in an 8:2 ratio and then split the training set again into a training set and validation set in an 8:2 ratio. Finally, the training set contained data from 100 nodules with LNM and 96 nodules without LNM, the validation set contained data from 26 nodules with LNM and 24 nodules without LNM, and the test set contained 32 nodules with LNM and 30 nodules without LNM.
In the data preprocessing stage, we conducted a degree of data enhancement to improve the generalization ability of the network. Data enhancement methods included operations of translation, flipping, and contrast change. All the B-mode images were normalized with a mean vector of [0.190, 0.201, 0.223] and a standard deviation vector of [0.123, 0.129, 0.142]. All the CEUS images were normalized with a mean vector of [0.226, 0.153, 0.092] and a standard deviation vector of [0.231, 0.169, 0.114]. The mean vectors and the standard deviation vectors were derived from the respective training datasets. Figure 2 shows a part of enhancement method for images, including scaling and adjustments for contrast and brightness.
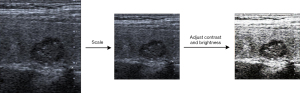
Development and validation of the CNN models
We classified each PTC case as LNM or non-LNM based on multiple complementary features. Specifically, our main technical contributions can be summarized as follows: (I) a novel multimodality neural network is proposed to perform LNM diagnosis in PTC rather than a single B-mode modality. (II) In our multimodality network, we use the visual modalities (i.e., B-mode images and CEUS images) as well as the textural patient clinical modality (i.e., the lymph node abnormality information). (III) Class activation map (CAM)-based heatmaps are proposed to enhance the interpretability of the “black-box” deep neural network. Moreover, the heatmaps can provide auxiliary information for clinicians when making decisions. The feature extraction part is divided into 3 branches: the B-mode image branch, CEUS image branch, and auxiliary data branch. The specific structure is illustrated in Figure 3.
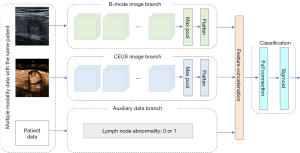
As shown in Figure 3, the B-mode ultrasound image and the CEUS image each enters the feature extraction network after the preprocessing operation, wherein operations such as translation and flipping are completed. The feature extraction network uses the residual network 50 (Resnet50) as the basic structure, which extracts features from B-mode ultrasound images and CEUS images. After the feature extraction with the module, features respectively based on B-mode ultrasound images and CEUS images can be obtained.
After the features extracted from the two images are obtained, they are fused. In the fusion part, all of the features extracted from the three branches are concatenated together to form a single feature vector. The dimension of the B-mode feature vector and the CEUS feature vector are both 2,048, so the dimension of the final feature vector is 4,097. The concatenation operation splices the features in the channel dimension and increases the feature representation while keeping the size of the feature map unchanged, which plays a certain role in the final training.
After feature fusion, the combined features are extracted from two types of images, which are input into the feature classification module composed of full connection and sigmoid layers, which finally outputs the classification result. In this study, binary cross entropy was used as the loss function, and the Adam optimizer was used to optimize the loss function. The initial learning rate was set to 0.001, and an adaptive drop of the learning rate was implemented to optimize the model performance.
Furthermore, in order to evaluate the performance of backbone feature extraction models, 3 popular backbone networks were compared for the B mode classification and CEUS classification, respectively. The networks included were Resnet50, visual geometry group (VGG), and Inception V3. After a comprehensive comparison, we found that the Resnet50 network performed better than did the others, and it was adopted in our proposed algorithm as the basic feature extraction module.
In practice, deep learning models are treated as “black-box” methods. The gradient-weighted class activation mapping method was used to generate heatmaps in order to enhance the explainability of our model. Gradient-weighted class activation mapping uses the gradients of any target concept (e.g., logits for “metastasis” or “nonmetastasis”) and flows into the final convolutional layer to produce a coarse localization map, highlighting the important regions in the image for predicting the concept.
Statistical analysis
Data management and analysis were performed using SPSS 26.0 (IBM Corp., Armonk, NY, USA). The characteristics are described as means and SDs for normally distributed variables, as medians and interquartile range (IQR) for nonnormally distributed continuous variables, and as percentages for categorical variables. The Mann-Whitney test was used for continuous variables, and the Pearson χ2 test was used for categorical variables. Sensitivity, specificity, accuracy, and the area under the receiver operating characteristic curve (AUC) were used to evaluate the final classification of the model. Permutation tests were used to calculate P values for the indicators of all classification models, with scikit-learn 1.3 being used to perform the permutation tests (16).
Results
Patient characteristics
Between August 2018 to April 2022, a total of 308 patients (mean age 43.3 years; range, 18–77 years) comprising 308 thyroid nodules were enrolled for analysis, including 75 males and 233 females. Unilateral lymph node dissection was performed in 258 patients (83.8%) and bilateral lymph node dissection in 50 patients (16.2%). The number of lymph node dissections in the central and lateral neck ranged from 1 to 41, with a mean of 8.9±7. The number of LNMs in central and lateral neck dissections ranged from 0 to 14, with a mean of 1.8±2.5. According to the pathological results, 158 nodules were malignant tumors with LNM and 150 nodules were non-LNM. Additionally, 246 nodules were randomly assigned as a training cohort and the other 62 nodules as the independent test cohort. The detailed characteristics including patient age, gender, tumor location, and maximum size are presented in Table 1.
Table 1
Characteristics | Training and validation sets (n=246) | Test set (n=62) | P value |
---|---|---|---|
Pathological results | 0.956 | ||
LNM | 126 (51.0) | 32 (51.6) | |
Non-LNM | 120 (49.0) | 30 (48.4) | |
Age (years) | 41.0 (33.0, 52.0) | 44.0 (36.0, 54.7) | 0.137 |
Gender | 0.305 | ||
Male | 63 | 12 | |
Female | 183 | 50 | |
Location | 0.109 | ||
Isthmus | 6 | 5 | |
Left lobe | 115 | 27 | |
Right lobe | 125 | 30 | |
Maximum size (cm) | 0.8 (0.6, 1.2) | 0.8 (0.7, 1.2) | 0.598 |
US examination | 0.817 | ||
US-LNM (+) | 60 | 16 | |
US-LNM (−) | 186 | 46 |
Data are expressed as the median (IQR) or n (%). LNM, lymph node metastasis; US, ultrasound; US-LNM, ultrasound examination of cervical lymph node metastasis; IQR, interquartile range.
Diagnostic performance of the CNN model
For CNN backbone selection, we first evaluated 3 different ad hoc CNN models for feature selection on B-mode images and CEUS images, respectively. The three CNN models we evaluated were Resnet50, VGG11, and InceptionV3. The training set randomly partitioned in a ratio of 8:2 was adopted as the evaluation data set. Quantitative index comparisons results on the three backbones for different modalities are summarized in Table 2. The corresponding AUCs are shown in Figures 4,5. From these results, one can see that Resnet50 performed better than did the others and hence was adopted as the feature extraction module in our proposed algorithm.
Table 2
Backbone | Sensitivity, % | Specificity, % | Accuracy, % | AUC |
---|---|---|---|---|
Results for B-mode images | ||||
Resnet50 | 75.00 | 72.73 | 71.12 | 0.758 |
VGG11 | 70.00 | 72.73 | 69.38 | 0.683 |
InceptionV3 | 62.50 | 70.83 | 69.38 | 0.675 |
Results for CEUS images | ||||
Resnet50 | 72.00 | 77.27 | 71.43 | 0.722 |
VGG11 | 68.00 | 72.73 | 67.35 | 0.700 |
InceptionV3 | 76.00 | 63.64 | 69.39 | 0.694 |
AUC, area under the curve; Resnet50, residual network 50; VGG, visual geometry group; CEUS, contrast-enhanced ultrasound.
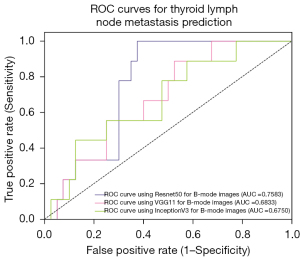
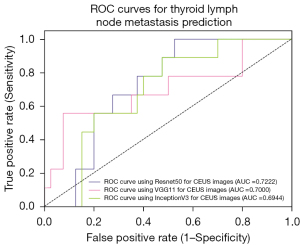
In cervical ultrasound for the diagnosis of LNM, the sensitivity was 38.60%, the specificity was 90.00%, the accuracy was 63.60%, and the AUC was 0.643 in the test set. In the experimental portion of the study, we first carried out the experiment using B-mode ultrasound image data alone for classification and then CEUS image data alone for classification. B-mode ultrasound image data used alone obtained a sensitivity of 70.00%, a specificity of 73.00%, an accuracy of 69.00%, and an AUC of 0.720. CEUS image data use alone for classification obtained a sensitivity of 72.00%, a specificity of 74.00%, an accuracy of 71.00%, and an AUC of 0.730. We then extracted features from the 2 kinds of images separately and then fused these features for classification. This proposed dual-modality model (B-mode + CEUS) yielded a sensitivity of 74.19%, a specificity of 77.42%, an accuracy of 75.81%, and an AUC of 0.742.
Finally, a triple-modality model (US-LNM + B-mode + CEUS) was evaluated in the same test dataset. In this experiment, all 3 modal features were fused together to make a final prediction. This yielded a sensitivity of 80.65%, a specificity of 82.26%, an accuracy of 80.65%, and an AUC of 0.831. The specific indicators are shown in Table 3.
Table 3
Method | Sensitivity, % (P value) |
Specificity, % (P value) |
Accuracy, % (P value) |
AUC (95% CI) |
---|---|---|---|---|
US-LNM | 38.60 (0.001) | 90.00 (0.001) | 63.60 (0.001) | 0.643 (0.581, 0.705) |
B-mode | 70.00 (0.010) | 73.00 (0.007) | 69.00 (0.004) | 0.720 (0.680, 0.760) |
CEUS | 72.00 (0.010) | 74.00 (0.010) | 71.00 (0.010) | 0.730 (0.679, 0.781) |
Dual-modality | 74.19 (0.003) | 77.42 (0.001) | 75.81 (0.011) | 0.742 (0.681, 0.803) |
Triple-modality | 80.65 (0.001) | 82.26 (0.001) | 80.65 (0.001) | 0.831 (0.812, 0.850) |
LNM, cervical lymph node metastasis; AUC, area under the curve; 95% CI, 95% confidence interval; US-LNM, ultrasound examination of cervical lymph node metastasis; CEUS, contrast-enhanced ultrasound.
The convergence curves of the model training are shown in Figure 6. The final AUCs of our proposed dual- and triple-modality models are shown in Figure 7. It can be seen from the experimental results that the method of extracting features from three different modalities and merging them together for classification produced the best indicators. This indicates that features of different modes have certain effects on LNM of PTC, and the fusion of the three provides a degree of improvement in prediction ability. The proposed method was demonstrated to be effective and can assist clinicians in predicting LNM status of PTC to a certain extent.
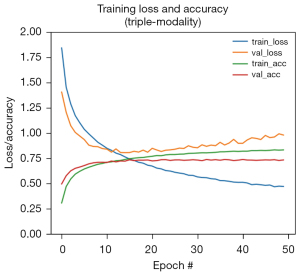
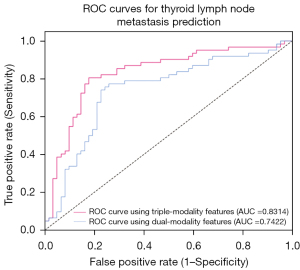
Decision visualization
Heatmaps can help ascertain whether the neural network is looking at appropriate parts of the image or if the neural network is cheating. In this study, gradient-weighted class activation mapping was adopted for this purpose; it uses the class-specific gradient information flowing into the final convolutional layer of a CNN to produce a coarse localization map of the important regions in the image. The heatmap of our triple-modality model is shown in Figures 8,9; the left part is an image sample from a patient with LNM, and the right part is an image sample from a patient without LNM. A high temperature in a heatmap indicates a higher attention region for the deep learning model. These figures clearly depict how the neural network model makes decisions on a B-mode image and a CEUS image.
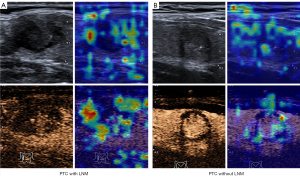
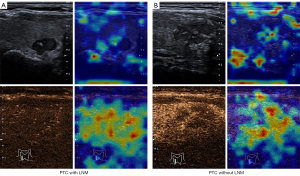
Discussion
LNM is often observed in early-stage thyroid malignancies, and there is an approximate 50% incidence of LNM among patients with PTC (17). The 2015 American Thyroid Association Management guidelines strongly recommended that cervical lymph node sonography be performed in all patients with suspected thyroid nodules (18). However, in the present study, the sensitivity of ultrasound for diagnosing LNM was found to be insufficient (38.6%), which is in line with previous reports (19,20). The utility of ultrasonography is limited for LNM, especially in the central region. This may be due to ultrasound unsatisfactorily depicting the complex structure in the central neck compartment and imperceptible minimal metastasis, which may cause recurrence or resistance.
Deep learning algorithms have recently exhibited remarkable performance in the medical field, such as in disease diagnosis and early prognosis or in the design of a patient’s individualized treatment plan. Computer-aided diagnosis systems on computed tomography images may help clinicians predict LNM status in patients with thyroid malignancies. Instead of detecting lymph nodes automatically, users of these systems have to identify lymph nodes and input the images into the artificial intelligence (AI) system, which restrains its clinical application (21). Multiple studies have reported that deep learning is good at predicting LNM based on ultrasonography of primary tumors, especially in breast cancer (13,22). However, modern deep neural network architectures cannot be applied directly to the PTC metastasis prediction due to several limitations. First, a massive amount of data (typically tens of thousands data points) is required for training a deep neural network (CNN or Transformer) in a natural image recognition task. Although transfer learning is a useful strategy to bypassing the data-lack problem, the structures of the PTC ultrasound image dataset and the natural image dataset are essentially different. Second, most of the existing cancer recognition methods only focus on one type of image data, and all the other types of image data and expert prior knowledge are completely ignored. These methods are not consistent with the clinical diagnostic process, and a lack of data may yield low diagnostic accuracy and reliability.
In order to overcome these challenges, we developed a multimodality CNN-based model to predict LNM in PTC. It is reasonable to conclude that features from different modalities of can provide complementary information for clinical diagnosis. Intrigued by this observation, researchers have paid greater attention to multimodality learning in the domain of computer vision-aided medical image analysis (23-25). By leveraging three modalities, such as B-mode image, the CEUS image, and US-LNM, complementary features can be learned to depict a patient from different views. The experimental findings of our study showed that our multimodality deep learning model had good performance in predicting LNM of PTC, with a sensitivity of 80.65%, a specificity of 82.26%, an accuracy of 80.65%, and an AUC of 0.831. Based on the above results, we consider that this model may be further used for the preliminary prediction of LNM in patients with PTC. B-mode images show the texture and shape features of thyroid cancer, while ultrasound contrast of microbubbles visualized the microvascular perfusion of malignant lesions. Our study showed that compared with using a single modality image to extract features and classifiers, using the combination of image features from three modalities and classifying the indicators provides a superior prediction performance. To the best of our knowledge, this is one of the first studies to leverage multimodality ultrasonic features including CEUS in predicting lymph node status in patients with PTC. Despite the promising performance achieved in previous work, fusing multiple visual and nonvisual features together still remains challenging (26). In combining the thyroid lymph node abnormality information together with the visual features, the two image feature learning branches can learn much more representative features in our proposed multimodality model. More specifically, the B-mode and CEUS ultrasound branches can provide complementary descriptions of a patient.
The black-box nature of deep learning limits human understanding of AI decision-making. To further illustrate the CNN model mechanism in a clinical setting, heatmaps were created by visualizing attention (Figure 8). This can help human experts understand the areas of particular interest in the different modal images when our model is working and thus gain more diagnostic experience. Based on the highlighted region in heatmaps of thyroid nodules, human experts can better reconsider the status of LNM, which may help in efficient decision-making. For example, the deep learning system seems to focus on the area surrounding the nodules rather than on the inside of the nodule. This indicates that the peripheral thyroid parenchyma may contain abundant information about LNM, which is consistent with a previous study (27). The heatmaps also reveal some of the model flaws. In Figure 9, we can see the heat maps of 2 incorrect prediction cases in which the attention regions for these samples are confused with the surrounding areas of the focus region, especially in B-mode imaging.
Despite the effectiveness of our proposed method in PTC prediction, there are several limitations that should be further considered in future studies. First, our research was developed based on just a single type of ultrasonic equipment. Moreover, the generalization performance of the CNN model should be verified by extending the test set. Second, our model requires all 3 modalities, and it is not suitable for an incomplete-data scenario (e.g., missing LNM status information), which could occur in real-world practice. Third, for the CEUS branch in our model, we only considered 1 static CEUS image for model training while completely ignoring the interframe video information. It makes more sense to use CEUS dynamic information, as this is more in line with the human expert-specific working pattern. Recent studies have reported that three-dimensional (3D) CNNs and hierarchical temporal attention networks are highly valuable in breast and thyroid cancer diagnosis based on CEUS videos (28,29). The application of AI predictive systems in medicine has limitations, as people may question the decisions made by these systems and have concerns regarding privacy breaches resulting from the collection of large amounts of data. However, the increased risk of recurrent laryngeal nerve injury and hypoparathyroidism with prophylactic central cervical lymph dissection deserves attention. Therefore, it is crucial to emphasize the interpretation of the AI system to ensure protection. Using our own models also enables us to protect the privacy and consent of study participants. This approach allowed us to further explore the mechanisms of LNM by ensuring that the input was medically meaningful. In future work, our work will focus on designing a multimodality model that includes both image and video inputs, with the domain knowledge also be taken into account. Furthermore, our study found no significant correlation between elastography or CDFI imaging and LNM, which is inconsistent with other reports (30,31). This may be due to the limitation of the training sample size, and our conclusions require further testing. Furthermore, we did not extensively analyze the characteristics of LNM, including the number, location, and size of suspicious lymph nodes. Previous research has demonstrated that there is a positive correlation between the LNM risk score and the number of LNMs in patients with PTC (5). Additionally, we did not incorporate biopsy molecular markers to assist in predicting LNM involvement before surgery, as not all patients underwent preoperative biopsy. In future studies, we plan to incorporate these factors into our model, which could potentially enhance the predictive performance of the model.
Conclusions
We developed an automatic prediction model for LNM based on Resnet50. The model combined with cervical ultrasonography, B-mode, and CEUS demonstrated good predictive potential of LNM status in independent test data. A heatmap was created to clarify the regions of interest of the model during the deep learning system diagnosis. This deep learning model mimics the workflow of a human expert and leverages multimodal data from patients with PTC, which can further support clinical decision-making.
Acknowledgments
We appreciate the assistance provided by CHISON Medical Technologies Co., Ltd. during the preparation of this manuscript.
Funding: This work was supported by
Footnote
Reporting Checklist: The authors have completed the TRIPOD reporting checklist. Available at https://qims.amegroups.com/article/view/10.21037/qims-23-318/rc
Conflicts of Interest: All authors have completed the ICMJE uniform disclosure form (available at https://qims.amegroups.com/article/view/10.21037/qims-23-318/coif). C.G. and P.D. are engineers in CHISON Medical Technologies Co., Ltd., who provided technical support to our research. The other authors have no conflicts of interest to declare.
Ethical Statement: The authors are accountable for all aspects of the work in ensuring that questions related to the accuracy or integrity of any part of the work are appropriately investigated and resolved. This study was conducted in accordance with the Declaration of Helsinki (as revised in 2013) and was reviewed and approved by the ethics committee of Beijing Tiantan Hospital, Capital Medical University (No. KY 2020-007-02). All patients provided their written informed consent to participate in this study.
Open Access Statement: This is an Open Access article distributed in accordance with the Creative Commons Attribution-NonCommercial-NoDerivs 4.0 International License (CC BY-NC-ND 4.0), which permits the non-commercial replication and distribution of the article with the strict proviso that no changes or edits are made and the original work is properly cited (including links to both the formal publication through the relevant DOI and the license). See: https://creativecommons.org/licenses/by-nc-nd/4.0/.
References
- Sung H, Ferlay J, Siegel RL, Laversanne M, Soerjomataram I, Jemal A, Bray F. Global Cancer Statistics 2020: GLOBOCAN Estimates of Incidence and Mortality Worldwide for 36 Cancers in 185 Countries. CA Cancer J Clin 2021;71:209-49. [Crossref] [PubMed]
- Sakorafas GH, Sampanis D, Safioleas M. Cervical lymph node dissection in papillary thyroid cancer: current trends, persisting controversies, and unclarified uncertainties. Surg Oncol 2010;19:e57-70. [Crossref] [PubMed]
- Alabousi M, Alabousi A, Adham S, Pozdnyakov A, Ramadan S, Chaudhari H, Young JEM, Gupta M, Harish S. Diagnostic Test Accuracy of Ultrasonography vs Computed Tomography for Papillary Thyroid Cancer Cervical Lymph Node Metastasis: A Systematic Review and Meta-analysis. JAMA Otolaryngol Head Neck Surg 2022;148:107-18. [Crossref] [PubMed]
- Zhan J, Zhang LH, Yu Q, Li CL, Chen Y, Wang WP, Ding H. Prediction of cervical lymph node metastasis with contrast-enhanced ultrasound and association between presence of BRAF(V600E) and extrathyroidal extension in papillary thyroid carcinoma. Ther Adv Med Oncol 2020;12:1758835920942367. [Crossref] [PubMed]
- Liu C, Xiao C, Chen J, Li X, Feng Z, Gao Q, Liu Z. Risk factor analysis for predicting cervical lymph node metastasis in papillary thyroid carcinoma: a study of 966 patients. BMC Cancer 2019;19:622. [Crossref] [PubMed]
- Zhan WW, Zhou P, Zhou JQ, Xu SY, Chen KM. Differences in sonographic features of papillary thyroid carcinoma between neck lymph node metastatic and nonmetastatic groups. J Ultrasound Med 2012;31:915-20. [Crossref] [PubMed]
- Jiang M, Li C, Tang S, Lv W, Yi A, Wang B, Yu S, Cui X, Dietrich CF. Nomogram Based on Shear-Wave Elastography Radiomics Can Improve Preoperative Cervical Lymph Node Staging for Papillary Thyroid Carcinoma. Thyroid 2020;30:885-97. [Crossref] [PubMed]
- Wu B, Waschneck B, Mayr CG. Convolutional Neural Networks Quantization with Double-Stage Squeeze-and-Threshold. Int J Neural Syst 2022;32:2250051. [Crossref] [PubMed]
- Kermany DS, Goldbaum M, Cai W, Valentim CCS, Liang H, Baxter SL, et al. Identifying Medical Diagnoses and Treatable Diseases by Image-Based Deep Learning. Cell 2018;172:1122-1131.e9. [Crossref] [PubMed]
- De Fauw J, Ledsam JR, Romera-Paredes B, Nikolov S, Tomasev N, Blackwell S, et al. Clinically applicable deep learning for diagnosis and referral in retinal disease. Nat Med 2018;24:1342-50. [Crossref] [PubMed]
- Zhu J, Zhang S, Yu R, Liu Z, Gao H, Yue B, Liu X, Zheng X, Gao M, Wei X. An efficient deep convolutional neural network model for visual localization and automatic diagnosis of thyroid nodules on ultrasound images. Quant Imaging Med Surg 2021;11:1368-80. [Crossref] [PubMed]
- Sun Q, Lin X, Zhao Y, Li L, Yan K, Liang D, Sun D, Li ZC. Deep Learning vs. Radiomics for Predicting Axillary Lymph Node Metastasis of Breast Cancer Using Ultrasound Images: Don't Forget the Peritumoral Region. Front Oncol 2020;10:53. [Crossref] [PubMed]
- Zhou LQ, Wu XL, Huang SY, Wu GG, Ye HR, Wei Q, Bao LY, Deng YB, Li XR, Cui XW, Dietrich CF. Lymph Node Metastasis Prediction from Primary Breast Cancer US Images Using Deep Learning. Radiology 2020;294:19-28. [Crossref] [PubMed]
- Yu J, Deng Y, Liu T, Zhou J, Jia X, Xiao T, Zhou S, Li J, Guo Y, Wang Y, Zhou J, Chang C. Lymph node metastasis prediction of papillary thyroid carcinoma based on transfer learning radiomics. Nat Commun 2020;11:4807. [Crossref] [PubMed]
- Wu X, Li M, Cui XW, Xu G. Deep multimodal learning for lymph node metastasis prediction of primary thyroid cancer. Phys Med Biol 2022; [Crossref]
- Pedregosa F, Varoquaux G, Gramfort A, Michel V, Thirion B, Grisel O, Blondel M, Müller A, Nothman J, Louppe G. Scikit-learn: Machine Learning in Python. Journal of Machine Learning Research 2011;12:2825-30.
- Feng Y, Min Y, Chen H, Xiang K, Wang X, Yin G. Construction and validation of a nomogram for predicting cervical lymph node metastasis in classic papillary thyroid carcinoma. J Endocrinol Invest 2021;44:2203-11. [Crossref] [PubMed]
- Haugen BR, Alexander EK, Bible KC, Doherty GM, Mandel SJ, Nikiforov YE, Pacini F, Randolph GW, Sawka AM, Schlumberger M, Schuff KG, Sherman SI, Sosa JA, Steward DL, Tuttle RM, Wartofsky L. 2015 American Thyroid Association Management Guidelines for Adult Patients with Thyroid Nodules and Differentiated Thyroid Cancer: The American Thyroid Association Guidelines Task Force on Thyroid Nodules and Differentiated Thyroid Cancer. Thyroid 2016;26:1-133. [Crossref] [PubMed]
- Hwang HS, Orloff LA. Efficacy of preoperative neck ultrasound in the detection of cervical lymph node metastasis from thyroid cancer. Laryngoscope 2011;121:487-91. [Crossref] [PubMed]
- Choi YJ, Yun JS, Kook SH, Jung EC, Park YL. Clinical and imaging assessment of cervical lymph node metastasis in papillary thyroid carcinomas. World J Surg 2010;34:1494-9. [Crossref] [PubMed]
- Lee JH, Ha EJ, Kim D, Jung YJ, Heo S, Jang YH, An SH, Lee K. Application of deep learning to the diagnosis of cervical lymph node metastasis from thyroid cancer with CT: external validation and clinical utility for resident training. Eur Radiol 2020;30:3066-72. [Crossref] [PubMed]
- Zheng X, Yao Z, Huang Y, Yu Y, Wang Y, Liu Y, Mao R, Li F, Xiao Y, Wang Y, Hu Y, Yu J, Zhou J. Deep learning radiomics can predict axillary lymph node status in early-stage breast cancer. Nat Commun 2020;11:1236. [Crossref] [PubMed]
- Korot E, Guan Z, Ferraz D, Wagner SK, Keane PA. Code-free deep learning for multi-modality medical image classification. Nature Machine Intelligence 2021;3:288-98.
- Pan Y, Liu M, Xia Y, Shen D. Disease-Image-Specific Learning for Diagnosis-Oriented Neuroimage Synthesis With Incomplete Multi-Modality Data. IEEE Trans Pattern Anal Mach Intell 2022;44:6839-53. [Crossref] [PubMed]
- Shi Y, Zu C, Hong M, Zhou L, Wang L, Wu X, Zhou J, Zhang D, Wang Y. ASMFS: Adaptive-similarity-based multi-modality feature selection for classification of Alzheimer's disease. Pattern Recognition 2022;126:108566.
- Xiang Z, Zhuo Q, Zhao C, Deng X, Zhu T, Wang T, Jiang W, Lei B. Self-supervised multi-modal fusion network for multi-modal thyroid ultrasound image diagnosis. Comput Biol Med 2022;150:106164. [Crossref] [PubMed]
- Tao L, Zhou W, Zhan W, Li W, Wang Y, Fan J. Preoperative Prediction of Cervical Lymph Node Metastasis in Papillary Thyroid Carcinoma via Conventional and Contrast-Enhanced Ultrasound. J Ultrasound Med 2020;39:2071-80. [Crossref] [PubMed]
- Chen C, Wang Y, Niu J, Liu X, Li Q, Gong X. Domain Knowledge Powered Deep Learning for Breast Cancer Diagnosis Based on Contrast-Enhanced Ultrasound Videos. IEEE Trans Med Imaging 2021;40:2439-51. [Crossref] [PubMed]
- Wan P, Chen F, Liu C, Kong W, Zhang D. Hierarchical Temporal Attention Network for Thyroid Nodule Recognition Using Dynamic CEUS Imaging. IEEE Trans Med Imaging 2021;40:1646-60. [Crossref] [PubMed]
- Wang B, Cao Q, Cui XW, Dietrich CF, Yi AJ. A model based on clinical data and multi-modal ultrasound for predicting cervical lymph node metastasis in patients with thyroid papillary carcinoma. Front Endocrinol (Lausanne) 2022;13:1063998. [Crossref] [PubMed]
- Liu Y, Huang J, Zhang Z, Huang Y, Du J, Wang S, Wu Z. Ultrasonic Characteristics Improve Prediction of Central Lymph Node Metastasis in cN0 Unifocal Papillary Thyroid Cancer. Front Endocrinol (Lausanne) 2022;13:870813. [Crossref] [PubMed]