Investigating the spontaneous brain activities of patients with subjective cognitive decline and mild cognitive impairment: an amplitude of low-frequency fluctuation functional magnetic resonance imaging study
Introduction
According to the China Population and Development Research Center, by the middle of the 21st century, the aging ratio of China will reach 34.6%, entering the process of severe aging (1). And it anticipates that Alzheimer’s disease (AD), one of the most common types of dementia in the elderly, will affect one in every 2–3 elderly people over 85 years old by 2030 (2). In 2018, the National Institute of Aging and Alzheimer’s Association jointly revised the diagnostic criteria of AD, emphasized the continuity of disease, and suggested that the pathophysiological process had begun 15 to 20 years before the onset of dementia symptoms (3). The constant pathophysiological process of AD includes subjective cognitive decline (SCD), mild cognitive impairment (MCI), and the stage of dementia (3). Patients with MCI and SCD have significantly higher progression rate to AD compared to cognitive intact elderly (4-6). An early screening of individuals with a high-risk of AD in a timely and accurate manner, as well as an early cognitive intervention in those elderly, may significantly reduce the incidence of AD.
Neuroimaging technology could help in the early detection of brain structural and functional abnormalities before objective defects are identified (7-11). As a safe and non-invasive neuroimaging methodology, resting-state functional magnetic resonance imaging (rs-fMRI), measures the internal spontaneous activities of brain blood oxygen level-dependent signals without any external stimulus (12), which could accurately reflect the intrinsic physiological status of brain activity. As a result, rs-fMRI is frequently utilized to examine the neurophysiological mechanism and neural process of depression (13), schizophrenia (14), or cognitive impairment (15), and to identify the functional integrity of relative brain networks. Functional changes measured by rs-fMRI in the brain are independent of and more sensitive than changes in brain structure during the early stages of AD (8,11).
The amplitude of low-frequency fluctuation (ALFF), one of the most commonly used features of rs-fMRI (16), reflects the intensity of the spontaneous neural activities. ALFF abnormality was found to be a sensitive indicator of AD-related neurodegeneration, as reported in previous studies (9,15,17,18). Therefore, it was used to describe the physiology of AD and MCI and reveal the intrinsic network disruption. Neurodegeneration appears to progress over time in AD. For example, SCD exhibits structural and functional changes similar to those of AD and MCI (19,20). In previous MCI and AD studies, ALFF values were revealed to be altered in the frontal gyrus, paracentral gyrus, precuneus, and other brain regions (9,18). However, the continuous process of intrinsic activity from healthy aging to SCD and then further to MCI yielded contradictory results (11,17), and few studies have been performed to explore the possible brain regions that play dominant roles in the influence of brain internal activities on cognitive function and the neuropsychological tests which are sensitive to changes in brain activity.
Therefore, the present study aimed to investigate the altered intrinsic brain activities in the neurodegenerative processing phases of AD and to explore the brain regions playing more important roles in cognitive decline, as well as the cognitive field which is more sensitive to changes in the altered intrinsic brain activity. To achieve these goals, we first measured the ALFF of each subject through rs-fMRI and compared the differences among NC, SCD, and MCI patients; secondly, we determined the behavioral significance of these altered brain activities. Canonical correlation analysis (CCA) was performed on the ALFF values of the altered regions with neuropsychological tests of the three groups. We present this article in accordance with the STROBE reporting checklist (available at https://qims.amegroups.com/article/view/10.21037/qims-23-808/rc).
Methods
Subjects
This cross-sectional study was conducted in accordance with the Declaration of Helsinki (as revised in 2013). The study was approved by the Ethics Committee of the First Affiliated Hospital of Nanjing Medical University (No. 2019-SR-015), and informed consent was taken from all the patients. Besides, the study was registered at the Chinese Clinical Trial Registry (ChiCTR1800015052). One hundred and seventy-one patients were recruited from the memory clinic of the First Affiliated Hospital of Nanjing Medical University from July 2019 to May 2021. Fifty demographically similar healthy volunteers were enrolled via advertisements in the local area. Elder individuals were recruited if they satisfied the following criteria: (I) age between 55 and 85 years old; (II) junior high school education or above; (III) no history of stroke, cerebral hemorrhage, brain tumor, head trauma, or Parkinson’s disease, and no history of alcohol and drug addiction; (IV) no serious diseases such as cardiovascular, cerebrovascular, liver and kidney dysfunction; (V) no blindness, deafness and other conditions that cannot cooperate with the cognitive function examination; (VI) have not used drugs to improve cognitive function or other drugs to improve cerebral blood circulation in the past two months. According to the recommendations for SCD and MCI diagnosis in China (6,21,22), participants were divided into SCD, MCI, and normal controls (NC) groups. All participants were right-handed and fluent in Chinese.
Neuropsychological tests
Clinical Dementia Rating (23), Hachinski Ischemic Score (24), and Mini-Mental State Examination (MMSE) (25) were first evaluated to exclude patients with vascular cognitive impairment and dementia. Then, neuropsychological tests covering three cognitive domains were evaluated to complete the diagnosis: (I) memory assessed by Auditory-Verbal Learning Test-Huashan version (AVLT-H) (26), and the test scores of the AVLT-H are as follows: (i) AVLT-N1/N2/N3, number of the correct responses given in the first/second/third consecutive trial; (ii) AVLT-N4, number of words answered correctly in the short-term delayed free recall; (iii) AVLT-N5, number of words answered correctly in the long-term delayed free recall; (iv) AVLT-N6, number of words responded correctly with category-cued recall; (v) AVLT-N7, number of words responded correctly in the recognition test; (II) executive function assessed by trail-making test (TMT) (27) parts A and B; and (III) language function assessed by Animal fluency test (AFT) (28) and Boston Naming Test (BNT) (29). Further cognitive function tests and daily life evaluations including the Montreal Cognitive Assessment (MoCA), the logical memory subtest of the Wechsler Memory Scale-Revised (WMS-RLM) (30,31), the forward and backward Digit Span Task (DST) (32), the Symbol Digit Modalities Test (SDMT) (33), and the 36-item short-form health survey (SF-36) (34) were also conducted.
Exclusion and Inclusion criteria
We focused on SCD and MCI patients who were at high risk for AD. Therefore, participants with dementia were excluded: (I) diagnosis of vascular dementia, with Hachinski Ischemic Score more than 4; (II) diagnosis of dementia, with the score of Clinical Dementia Rating more than 1 or MMSE less than 24.
MCI was defined as the initial stage of cognitive impairment, which is more serious than normal age-related cognitive decline, but not enough to cause serious damage to activities of daily living (35). The participants were considered to have MCI if they met at least one of the following criteria: (I) impaired score, defined as >1 standard deviation (SD) below the age-corrected normative means, on both scores for at least one cognitive domain (memory, executive function, or language); (II) one impaired score, defined as >1 SD below the age-corrected normative mean, in each of the three cognitive domains (memory, executive function, or language). The normative means used in this study were taken and summarized from previous studies in the Chinese population (26,35).
Self-reported questionnaires (22) in line with the suggestions of the SCD Initiative Working Group (6) were used to discriminate SCD from NC. The inclusion criteria for SCD were as follows: (I) self-experienced persistent decline in memory rather than other domains of cognition for more than 6 months; (II) concerns related to SCD and feeling of deteriorating performance compared to the same age-group individuals; and (III) performance on standardized cognitive tests with age, gender, and education adjusted norms, i.e., without meeting the diagnostic criteria for MCI or dementia.
In addition, the inclusion criteria for the NC group were as follows: (I) not fulfilling the diagnosis of SCD or MCI; and (II) no complaints of cognitive impairment or memory loss.
MRI acquisition
MRI images were collected with a 3.0T-MR scanner (Discovery 750 W, GE Healthcare, Chicago, IL, USA) using a standard 24-channel head coil. The subjects were required to keep their eyes closed and stay still, awake, and relaxed. Earplugs were used to reduce scan noise, and foam pads were inserted into the gap to reduce head movements as well as to avoid artifacts. 3D high-resolution BRAVO imaging based on a fast rotating gradient echo sequence was used to obtain T1 weighted MR images (scanning parameters: field of view =256×256 mm2, repetition time =8.5 ms, echo time =3.2 ms, inversion time =450 ms, flip angle =12°, number of layers =188, layer thickness =1 mm, and acquisition matrix =256×256), excluding intracranial organic lesions. The echo planar imaging-based fMRI imaging was applied to collect functional images (scanning parameter: field of view =224×224 mm2, repetition time =2,000 ms, echo time =30 ms, flip angle =90°, number of layers =33, layer thickness =3.5 mm and matrix =64×64, and 240 phases). The total scanning time was less than 15 minutes.
Image data pre-processing
T1 weighted images were manually evaluated by an experienced neurologist and a radiologist for quality control. The standard preprocessing steps were performed via the Data Processing Assistant for Resting-state fMRI (DPABI, V6.1, SCR_010501), which included removing the first ten images, slice-timing, realignment, and spatial normalization. Functional images were smoothed with 6-mm full-width at half-maximum Gaussian kernel. The co-registered functional images were then transformed and normalized into the Montreal Neurological Institute (MNI) template with 3×3×3 mm3 resolution. Subjects who had excessive head motion (>2.0 mm translation and/or 2.0˚ rotation) were excluded for further analysis. Finally, imaging covariates were regressed using a least squares approach and a Friston 24-parameter linear transformation.
After pre-processing, the conventional ALFF was calculated as the average square root of the power spectrum across 0.01–0.08 Hz at each voxel. Then, the ALFF of each voxel was divided by the global mean ALFF to normalize the global effects.
Statistical analysis
Statistical analysis was performed using SPSS Version 25.0 (IBM Corp., Armonk, NY, USA). Demographic and neuropsychological scores were compared among the three groups. One-way analysis of variance (ANOVA) was applied to test the age differences, chi-square tests were used for gender and comorbidities, and nonparametric test was used for education. Basic cognitive scores and basic health status were also compared among the three groups. The level of statistical significance was set at P<0.05 (two-tailed).
The difference in ALFF was evaluated among the three groups using one-way ANOVA with age as a covariate based on DPASF software. Multiple comparison correction was performed using the Gaussian random field (GRF) method by setting P<0.001 at the voxel level and P<0.05 at the cluster level. Brain areas that survived the GRF correlation were used for the following post-hoc analysis to identify the difference between MCI vs. NC, MCI vs. SCD, and SCD vs. NC (voxel-level P<0.001, cluster level P<0.05). A statistical level of P<0.05 was considered significant.
Brain activity associations with cognitive behavior
CCA, a multivariate statistical approach, was conducted to investigate the associations between imaging measures and behaviors (36). Specifically, CCA finds a linear combination of brain activity that is maximally correlated with a linear combination of cognitive behavior variables, as defined in Y * A = U ~ V = X * B, where Y is the set of brain measures, X is the set of cognitive behaviors, A and B are the linear weights for X and Y, respectively, and U and V are the canonical variate pair. The canonical correlation between U and V is defended as the canonical correlation. The canonical loadings indicate the importance of the variables of the observations, in this case, instinct brain activity (denoted as Y) and cognitive behavior (denoted as X).
Results
Demographics and neuropsychological tests
A total of 221 Han Chinese subjects were enrolled in this study, of whom 178 met the inclusion criteria and completed the baseline assessments. Thirty-nine NC, 73 SCD, and 46 MCI were enrolled in this study after excluding subjects with poor cooperation, refusing to sign the written consent or loss of contact. Finally, 36 NC, 72 SCD, and 44 MCI were included in the ALFF analysis after restricting head motion to less than 2.0 mm or 2.0 degrees (Figure 1). Demographic characteristics are presented in Table 1. Demographic characteristics were balanced in gender, education years, and comorbidities among the three groups (P>0.05). There are differences in age distribution among the three groups (P=0.035), mainly due to the significant age difference between SCD and NC (P=0.010).
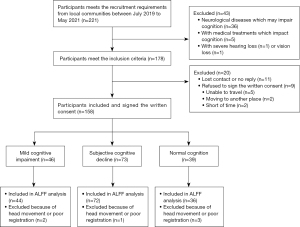
Table 1
Characteristics | NC (n=36) | SCD (n=72) | MCI (n=44) | F/χ2 | P |
---|---|---|---|---|---|
Female†, n (%) | 15 (41.7) | 47 (65.3) | 24 (54.5) | 5.550 | 0.062 |
Age (years)†, mean (SD) | 71.72 (6.497) | 67.93 (7.362) | 69.46 (7.225) | 3.416 | 0.035 |
Education years†, M [Q1, Q3] | 12 [12, 16] | 12 [12, 14] | 12 [9, 15] | 3.685 | 0.158 |
High blood pressure‡, n (%) | 19 (52.8) | 32 (44.4) | 23 (52.3) | 0.986 | 0.611 |
Diabetes†, n (%) | 6 (16.7) | 11 (15.3) | 11 (25.0) | 1.814 | 0.404 |
Demographic characteristics were balanced comorbidities in gender, education years, and comorbidities among the three groups (P>0.05). There are differences in age distribution among the three groups (P=0.035). †, nonparametric test was applied in non-normally distributed continuous data; ‡, high blood pressure is defined as systolic blood pressure >140 mmHg or diastolic blood pressure >90 mmHg. NC, normal control; SCD, subjective cognitive decline; MCI, mild cognitive impairment; SD, standard deviation; m, median; Q1, first quartile; Q3, third quartile.
Participants’ cognitive behaviors are summarized in Table 2. Global cognition assessed using MMSE and MoCA and neuropsychological tests in other cognitive fields was statistically significant among the three groups (P<0.05). Bonferroni post-hoc test was applied to explore the difference between MCI/NC, SCD/NC, and MCI/SCD (Table 2). Comparisons confirmed the poorer performance of MCI than NC in MMSE, MoCA, BNT, AFT, delayed recall (AVLT-N5), recognition (AVLT-N7), TMT A&B, WMS-RLM, SDMT, DST, and other cognitive subitems (AVLT-N2, AVLT-N3, AVLT-N4, and AVLT-N6) (37) (adjusted P<0.05). SCD performed worse than NC in MMSE, MoCA, BNT, AFT, TMT A, WMS-RLM, SDMT, DST, and other cognitive subitems (AVLT-N3 and AVLT-N6) (adjusted P<0.05). Meanwhile, MCI performed poorer than SCD in AFT, delayed recall (AVLT-N5), recognition (AVLT-N7), TMT A&B, DST, SDMT, and other cognitive subitems (AVLT-N1, AVLT-N3, AVLT-N4, and AVLT-N6) (adjusted P<0.05). Overall, compared with NC, the cognitive function of MCI and SCD decreased, and MCI performed the worst.
Table 2
Variables | NC (n=36) | SCD (n=72) | MCI (n=44) | F/χ2 (P) | Absolute difference (P value) | ||
---|---|---|---|---|---|---|---|
MCI vs. NC§ | SCD vs. NC§ | MCI vs. SCD§ | |||||
MMSE | 29 [28, 30] | 27 [26, 28] | 27 [26, 28] | 24.638 (<0.001)† | 4.775 (<0.001) | 3.969 (<0.001) | 1.375 (0.508) |
MoCA | 27 [27, 28] | 23 [21, 25] | 22 [19, 24] | 57.146 (<0.001)† | 7.231 (<0.001) | 6.132 (<0.001) | 1.951 (0.153) |
BNT | 26 [22, 27] | 23 [21, 26] | 22 [18, 24] | 18.205 (<0.001)† | 4.260 (<0.001) | 2.768 (0.017) | 2.050 (0.121) |
AFT | 21 [18, 24] | 17 [14, 20] | 14 [11, 18] | 32.382 (<0.001)† | 5.668 (<0.001) | 3.837 (<0.001) | 2.564 (0.031) |
AVLT | |||||||
N5 | 4 [3, 6] | 4 [3, 6] | 2 [0, 4] | 29.180 (<0.001)† | 4.498 (<0.001) | 0.343 (1.000) | 4.917 (<0.001) |
N7 | 22 [21, 23] | 21 [20, 22] | 18 [16, 20] | 36.602 (<0.001)† | 5.834 (<0.001) | 2.258 (0.072) | 4.443 (<0.001) |
N1 | 4 [3, 5] | 4 [3, 4] | 3 [2, 4] | 9.850 (0.007) † | 3.023 (0.008) | 1.142 (0.253) | 2.325 (0.060) |
N2 | 6 [5, 8] | 5 [5, 7] | 4 [3, 5] | 30.062 (<0.001)† | 5.229 (<0.001) | 1.823 (0.205) | 4.182 (<0.001) |
N3 | 8 [6, 9] | 7 [6, 8] | 6 [4, 6] | 29.370 (<0.001)† | 5.333 (<0.001) | 2.433 (0.045) | 3.658 (0.001) |
N4 | 6 [4, 7] | 5 [3, 6] | 3 [1, 4] | 34.718 (<0.001)† | 5.492 (<0.001) | 1.594 (0.333) | 4.733 (<0.001) |
N6 | 5 [4, 7] | 4 [2, 6] | 2 [1, 3] | 36.022 (<0.001)† | 5.865 (<0.001) | 2.506 (0.037) | 4.203 (<0.001) |
TMT_A | 52.5 [44.3, 69.0] | 65.0 [54.5, 80.5] | 82.5 [62.0, 100.3] | 24.573 (<0.001)† | 4.944 (<0.001) | 2.697 (0.021) | 2.915 (0.011) |
TMT_B | 142.5 [108.3, 175.3] | 169.0 [134.0, 196.0] | 221.5 [182.8, 261.5] | 36.671 (<0.001)† | 5.859 (<0.001) | 2.298 (0.065) | 4.416 (<0.001) |
WMS-RLM | 18 [15, 23] | 13 [8, 18] | 11 [6, 16] | 25.116 (<0.001)† | 4.867 (<0.001) | 3.816 (<0.001) | 1.761 (0.235) |
DST | 18.0 (3.0) | 15.5 (3.3) | 15.1 (3.2) | 8.906 (<0.001)‡ | −2.947 (<0.001) | −2.375 (0.001) | −0.572 (0.764) |
SDMT | 40.6 (11.0) | 32.0 (9.8) | 27.3 (8.9) | 17.285 (<0.001)‡ | −13.341 (<0.001) | −8.583 (<0.001) | −4.758 (0.019) |
SF-36 | 123 [115, 134] | 119 [111, 125] | 117 [102, 131] | 3.690 (0.158)† | – | – | – |
Global cognition assessed using MMSE and MoCA and neuropsychological tests in other cognitive fields was statistically significant among the three groups (P<0.001). Comparisons confirmed the poorer performance of MCI than SCD and NC participants in BNT, AFT, six subitems of AVLT, TMT A&B, WMS-RLM, SDMT, DST, and SF-36 (P<0.001). There are also significant differences among the three groups for the first immediate recall (AVLT-N1) (F=9.850, P=0.007). The worst cognitive performance was observed in MCI, with intermediate cognitive performance in SCD, and the best cognitive performance in NC. Data are shown as mean (SD) or median (Q1, Q3). †, nonparametric test was applied in non-normally distributed continuous data; ‡, one-way ANOVA was applied in normally distributed continuous data; §, Bonferroni correction was used in post-hoc comparisons across the three groups. NC, normal control; SCD, subjective cognitive decline; MCI, mild cognitive impairment; MMSE, Mini-Mental State Examination; MoCA, Montreal Cognitive Assessment; BNT, Boston Naming Test; AFT, Animal fluency test; AVLT, Auditory-Verbal Learning Test; TMT_A, trail-making test, part A; TMT_B, trail-making test, part B; WMS-RLM, Wechsler Memory Scale-Revised; DST, the forward and backward Digit Span Task; SDMT, Symbol Digit Modalities Test; SF-36, the 36-item short-form health survey; SD, standard deviation; Q1, first quartile; Q3, third quartile.
ALFF results
The ALFF values were quantified at each voxel for all MCI, SCD, and normal participants (Figure 2). There were significant group differences among these three groups on the ALFF values in the orbital region of right inferior frontal gyrus (Frontal_Inf_Orb_2_R), opercular part of the right inferior frontal gyrus (Frontal_Inf_Oper_R), right middle frontal gyrus (Frontal_Mid_2_R), left superior frontal gyrus (Frontal_Sup_2_L), left superior parietal gyrus (Parietal_Sup_L), right superior parietal gyrus (Parietal_Sup_R), left middle occipital gyrus (Occipital_Mid_L), left superior temporal gyrus (Temporal_Sup_L), left angular (Angular_L), left precentral gyrus (Precentral_L), right precentral gyrus (Precentral_R), left postcentral gyrus (Postcentral_L), and right supramarginal gyrus (SupraMarginal_R) (GRF correction, voxel P<0.001, cluster P<0.01). The clusters with significant differences among the three groups are shown in Figure 2A and Figure S1. The corresponding anatomical location, cluster voxels, MNI coordinates, intensity of the significance, and automated anatomical labeling (AAL) partition of these clusters were labeled (Table 3).
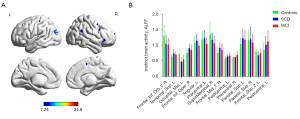
Table 3
Regions | Clusters Voxels | Peak MNI coordinate | Peak intensity | ||
---|---|---|---|---|---|
X | Y | Z | |||
Frontal_Inf_Orb_2_R | 23 | 54 | 30 | −3 | 13.1006 |
Temporal_Mid_L | 13 | −45 | −45 | 12 | 13.1393 |
Occipital_Mid_L | 34 | −39 | −87 | 27 | 34.8036 |
Frontal_Inf_Oper_R | 42 | 48 | 9 | 21 | 20.5463 |
Angular_L | 16 | −51 | −54 | 24 | 15.1053 |
Precentral_L | 10 | −45 | 9 | 33 | 10.4635 |
SupraMarginal_R | 19 | 60 | −48 | 27 | 12.9526 |
Frontal_Mid_2_R | 17 | 33 | 30 | 36 | 14.0897 |
Postcentral_L | 15 | −36 | −27 | 42 | 14.4983 |
Precentral_R | 13 | 33 | −15 | 45 | 14.8993 |
Precuneus_L | 27 | −15 | −66 | 54 | 14.1771 |
Parietal_Sup_R | 17 | 21 | −63 | 57 | 13.6942 |
Frontal_Sup_2_L | 21 | −18 | 12 | 54 | 23.3586 |
Postcentral_L | 14 | −48 | −27 | 57 | 9.2403 |
The corresponding anatomical location, cluster voxels, MNI coordinates, intensity of the significance, and AAL partition of these clusters of different brain regions with different ALFF values between NC/SCD/MCI groups (GRF correction, voxel P<0.001, cluster P<0.01). MNI, Montreal Neurological Institute; Frontal_Inf_Orb_2_R, orbital region of right inferior frontal gyrus; Temporal_Mid_L, left middle temporal gyrus; Occipital_Mid_L, left middle occipital gyrus; Frontal_Inf_Oper_R, opercular part of the right inferior frontal gyrus; Angular_L, left angular; Precentral_L, left precentral gyrus; SupraMarginal_R, right supramarginal gyrus; Frontal_Mid_2_R, right middle frontal gyrus; Postcentral_L, left postcentral gyrus; Precentral_R, right precentral gyrus; Precuneus_L, left precuneus; Parietal_Sup_R, right superior parietal gyrus; Frontal_Sup_2_L, left superior frontal gyrus; Postcentral_L, left postcentral gyrus; AAL, automated anatomical labeling.
The post-hoc two-sample analyses showed that patients with MCI exhibited significantly higher ALFF values in Postcentral_L, Precentral_R, and Parietal_Sup_R, as well as lower ALFF values in Angular_L and Precentral_L, compared with SCD (P<0.001, GRF corrected). Relative to NC, MCI had significantly higher ALFF values in Precentral_R, Frontal_Inf_Oper_R, Frontal_Mid_2_R, Parietal_Sup_R, and Postcentral_L, and significantly lower ALFF values in Frontal_Inf_Orb_2_R, SupraMarginal_R, and Occipital_Mid_L (P<0.001, GRF corrected). In addition, compared with NC, SCD patients significantly showed higher ALFF values in Frontal_Inf_Oper_R, Frontal_Mid_2_R, left precuneus (Precuneus_L), left middle temporal gyrus (Temporal_Mid_L), and Parietal_Sup_L, with lower ALFF values in Frontal_Inf_Orb_2_R, Frontal_Sup_2_L, SupraMarginal_R, left middle occipital gyrus (Occipital_Mid_L) (P<0.001, GRF corrected) (Figures S2-S4).
Correlation between ALFFs and neuropsychological tests
CCA indicated a significant correlation between intrinsic brain activity and behavior tests (R=0.73, P=0.002). As shown in Figure 3, the brain activities of the right middle frontal gyrus, left middle occipital gyrus, left superior frontal gyrus, left angular, and left superior temporal gyrus are more important in neuropsychological tests. The scores of MoCA, AVLT-N5, AVLT-N1, AVLT-N2, and AVLT-N3 are important in intrinsic brain activity.
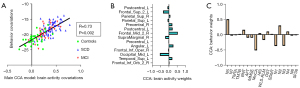
Discussion
AD is the most common neurodegenerative disease of the elderly, which seriously affects the elderly’s cognition and independent self-care while its long course and severe disability bring a heavy burden to families and society (2,38). Both MCI and SCD elderlies have a higher progression rate of AD compared to normal individuals (4,5). It is important to recognize high-risk populations of AD and treat them timely and actively in the early preclinical stage. The purpose of this study was to explore the internal activity changes of subjects at high-risk of AD and find out the potential biomarkers of cognitive decline in these special populations, as well as more sensitive neuropsychological tests.
In this study, we scrutinized the differences in neuropsychological tests and ALFF values among the three groups and found that the MCI and SCD groups performed significantly different on several behavioral tests and ALFF values compared with NC. The elderly’s global cognitive function, memory, language, and executive function all decreased significantly as the cognitive impairment progressed from healthy aging to MCI, but their activities of daily living did not significantly deteriorate. Also, the ALFF values of the prefrontal, parietal, and temporal lobes, as well as other related important brain regions, were significantly different from those of the healthy elderly, indicating that the changes in intrinsic activities were closely related to the decline in cognitive function in patients with MCI and SCD. These findings confirmed that the alteration of ALFF values indicates cognitive impairment in elder people, in accordance with previous studies (9,11,15).
By further analyzing the ALFF values with neuropsychological tests, we discovered a significant correlation (R=0.73) between behavior tests and intrinsic brain activity. In addition, the ALFF values in the right middle frontal gyrus, left superior frontal gyrus, left middle occipital gyrus, left angular, and left superior temporal gyrus all play significant roles in the connection, indicating that intrinsic activities in these areas have greater impacts on cognitive impairment.
According to previous research, higher left frontal cortex-hub connectivity was partially responsible for higher resilience against cognitive impairment in the early stages of AD (37), and AD had a smaller regional volume of gray matter in the occipital cortex and frontal gyrus than non-AD (38,39), indicating that abnormal instinct activity and structural changes in the prefrontal cortex and occipital cortex reduce cognitive control. Similarly in our study, the prefrontal cortex and occipital cortex, as the most correlated brain regions in CCA, play an important role in the cognitive control and coordinated processing of multiple regions. Additionally, the occipital lobe is in charge of processing and integrating visual information into cognition. A previous study using PET revealed a significant increase in metabolism in the MCI group’s inferior occipital gyrus (39). Moreover, compared with NC, the SCD group exhibited increased local functional activity in the right middle occipital gyrus, which significantly negatively correlated with the MMSE and AFT scores (40). It implies that the increase in local functional activity in the occipital gyrus is a compensatory manifestation of general cognitive and language dysfunction and is related to the progression of the condition. In this study, the increased activity of the left angular gyrus also accounts for a large proportion of the changes in local brain activity, with high importance and weight. The angular gyrus, part of the default network, which is thought to be suppressed in AD (41), is involved in many cognitive tasks, including semantic processing, spatial cognition, memory retrieval, attention, and word reading and understanding (42,43). It is also thought to play a significant role in cognitive dysfunction caused by neurodegenerative diseases. Therefore, the abnormal local activity of the angular gyrus may at least partially be the cause of the decline in cognitive impairment scores on neuropsychological tests. Furthermore, a randomized controlled trial suggested that repetitive transcranial magnetic stimulation could improve cognitive function by improving the local activities of the prefrontal lobe and angular gyrus in MCI patients, further demonstrating the important role of these brain regions in cognitive function (44). The temporal gyrus collaborates with the hippocampus complex to create episodic memory and working memory and responds to anticipation (45,46). Previous research found that cognitive apathy is related to higher connectivity between the temporal gyrus and hypothalamic (47) in AD compared with MCI, which may reflect functionally compensatory processes in link with apathy. This also indicates the important role of intrinsic activity in the temporal lobe in cognitive decline, consistent with our results.
However, there is not any significant group difference among these three groups on the ALFF values in the hippocampus, and the intrinsic activity of the hippocampus did not appear to play a significant role in cognitive impairment in the further CCA, which is quite different from previous studies that believed hippocampal dysfunction represented a typical etiological marker of age-related cognitive decline and emotional processing dysfunction (48-51). This may be due to the study’s preference for local activation levels over local volume changes and the peculiarities of the connections between different regions of the brain.
MoCA and AVLT scores were also found to be more sensitive to ALFF changes in the CCA analysis. The screening tool MoCA is superior to MMSE in the identification of MCI for overall cognitive function (52) and reflects cognitive reserve more sensitively (53). Among MCI patients with lacunar infarctions, MoCA scores showed a relatively weak correlation with the ALFF values in the medial frontal gyrus (54). Therefore, it is suggested that MoCA is more sensitive to determining the change in ALFF value and early cognitive decline. Also, AVLT is often used to assess memory, an important domain of cognitive function (55,56). In our study, the scores of AVLT-N1, AVLT-N2, and AVLT-N3, reflecting short-term memory, and the scores of AVLT-N5, reflecting delayed recall, were sensitive to ALFF changes. It is possible to think of the AVLT-N1, AVLT-N2, and AVLT-N3 as a voice loop because they include both short-term acoustic storage of sound input and word repetition. In addition, the AVLT-N5 is a significant predictor of MCI-to-AD conversion, providing the best values in predicting MCI outcomes (26), which is consistent with our results that the scores of AVLT-N5 show a trend of gradual deterioration with cognitive decline. The previous study showed that the involvement of the bilateral supramarginal gyrus in auditory-vocal integration (57), and the left supramarginal gyrus is specifically engaged in the detection of changes in phonological units (58), which is a significantly different area among the three groups. Furthermore, significantly positive correlations were observed between changes in response accuracy assessed by a short memorized test of letters and numbers and the changes in ALFF of the inferior frontal gyrus and supramarginal gyrus after repetitive transcranial magnetic stimulation (59). Taken together, short-term memory generated by auditory stimulation as well as working memory are more accurate for the evaluation of cognitive impairment and help identify early local brain activity abnormalities in cognitive impairment patients.
Limitations
There are several limitations in the present study. First, the sample size is relatively moderate, resulting in insufficient sensitivity of the results. In addition, we did not follow-up these participants on their progression of cognitive status longitudinally and thus could not further clarify the changes of ALFF during cognitive progression. Additionally, compared to ALFF, investigations have shown that fractional ALFF (fALFF) may greatly increase the sensitivity and specificity in identifying localized spontaneous brain activity (16,60). Therefore, further studies involving a more comprehensive population and using more advanced research methods should be conducted to accurately explore the changes in brain function during the development of AD.
Conclusions
In conclusion, this preliminary study provides evidence for changes in ALFF of the prefrontal, parietal lobe, temporal lobe, and other related important brain regions among MCI and SCD patients and NC, and associations between ALFF values for abnormal brain areas and cognitive performance in the elderly. Therefore, spontaneous brain activity may be a novel imaging biomarker of cognitive impairment. In addition, the changes in ALFF of the prefrontal, occipital gyrus, and the left angular were sensitive to identifying cognitive impairment, and the scores of AVLT and MoCA could predict the intrinsic activity of abnormal brain areas.
Acknowledgments
We would like to express our gratitude for the dedication of all the participants. Besides, we thank Dr. Long Qian (GE Healthcare, MR Research China, Beijing, China) for his support in the design of the MRI protocol and image analysis and thank Dr. Jin Liu (Clinical Medicine Research Institution, The First Affiliated Hospital of Nanjing Medical University, Jiangsu Province Hospital, Nanjing, China) for his kind statistical advice for this manuscript.
Funding: This work was supported by
Footnote
Reporting Checklist: The authors have completed the STROBE reporting checklist. Available at https://qims.amegroups.com/article/view/10.21037/qims-23-808/rc
Conflicts of Interest: All authors have completed the ICMJE uniform disclosure form (available at https://qims.amegroups.com/article/view/10.21037/qims-23-808/coif). WD is an employee of GE Healthcare. The other authors have no conflicts of interest to declare.
Ethical Statement: The authors are accountable for all aspects of the work in ensuring that questions related to the accuracy or integrity of any part of the work are appropriately investigated and resolved. This study was conducted in accordance with the Declaration of Helsinki (as revised in 2013). The study was approved by the Ethics Committee of the First Affiliated Hospital of Nanjing Medical University (No. 2019-SR-015) and written informed consent was collected from each of the participants.
Open Access Statement: This is an Open Access article distributed in accordance with the Creative Commons Attribution-NonCommercial-NoDerivs 4.0 International License (CC BY-NC-ND 4.0), which permits the non-commercial replication and distribution of the article with the strict proviso that no changes or edits are made and the original work is properly cited (including links to both the formal publication through the relevant DOI and the license). See: https://creativecommons.org/licenses/by-nc-nd/4.0/.
References
- Liu H. Trends of Population Aging in China and the World as a Whole. Scientific Research on Aging 2021;9:1-16.
- McDade E, Bateman RJ. Stop Alzheimer's before it starts. Nature 2017;547:153-5. [Crossref] [PubMed]
- Jack CR Jr, Bennett DA, Blennow K, Carrillo MC, Dunn B, Haeberlein SB, et al. NIA-AA Research Framework: Toward a biological definition of Alzheimer's disease. Alzheimers Dement 2018;14:535-62. [Crossref] [PubMed]
- Mitchell AJ, Beaumont H, Ferguson D, Yadegarfar M, Stubbs B. Risk of dementia and mild cognitive impairment in older people with subjective memory complaints: meta-analysis. Acta Psychiatr Scand 2014;130:439-51. [Crossref] [PubMed]
- Jessen F, Wolfsgruber S, Wiese B, Bickel H, Mösch E, Kaduszkiewicz H, Pentzek M, Riedel-Heller SG, Luck T, Fuchs A, Weyerer S, Werle J, van den Bussche H, Scherer M, Maier W, Wagner M. AD dementia risk in late MCI, in early MCI, and in subjective memory impairment. Alzheimers Dement 2014;10:76-83. [Crossref] [PubMed]
- Jessen F, Amariglio RE, van Boxtel M, Breteler M, Ceccaldi M, Chételat G, et al. A conceptual framework for research on subjective cognitive decline in preclinical Alzheimer's disease. Alzheimers Dement 2014;10:844-52. [Crossref] [PubMed]
- Dubois B, Feldman HH, Jacova C, Dekosky ST, Barberger-Gateau P, Cummings J, Delacourte A, Galasko D, Gauthier S, Jicha G, Meguro K, O'brien J, Pasquier F, Robert P, Rossor M, Salloway S, Stern Y, Visser PJ, Scheltens P. Research criteria for the diagnosis of Alzheimer's disease: revising the NINCDS-ADRDA criteria. Lancet Neurol 2007;6:734-46. [Crossref] [PubMed]
- Gonneaud J, Baria AT, Pichet Binette A, Gordon BA, Chhatwal JP, Cruchaga C, et al. Accelerated functional brain aging in pre-clinical familial Alzheimer's disease. Nat Commun 2021;12:5346. [Crossref] [PubMed]
- Liu X, Wang S, Zhang X, Wang Z, Tian X, He Y. Abnormal amplitude of low-frequency fluctuations of intrinsic brain activity in Alzheimer's disease. J Alzheimers Dis 2014;40:387-97. [Crossref] [PubMed]
- Lai Z, Zhang Q, Liang L, Wei Y, Duan G, Mai W, Zhao L, Liu P, Deng D. Efficacy and Mechanism of Moxibustion Treatment on Mild Cognitive Impairment Patients: An fMRI Study Using ALFF. Front Mol Neurosci 2022;15:852882. [Crossref] [PubMed]
- Zhang Q, Wang Q, He C, Fan D, Zhu Y, Zang F, Tan C, Zhang S, Shu H, Zhang Z, Feng H, Wang Z, Xie C. Altered Regional Cerebral Blood Flow and Brain Function Across the Alzheimer's Disease Spectrum: A Potential Biomarker. Front Aging Neurosci 2021;13:630382. [Crossref] [PubMed]
- Jurkiewicz MT, Crawley AP, Mikulis DJ. Is Rest Really Rest? Resting-State Functional Connectivity During Rest and Motor Task Paradigms. Brain Connect 2018;8:268-75. [Crossref] [PubMed]
- Tian S, Zhu R, Chattun MR, Wang H, Chen Z, Zhang S, Shao J, Wang X, Yao Z, Lu Q. Temporal dynamics alterations of spontaneous neuronal activity in anterior cingulate cortex predict suicidal risk in bipolar II patients. Brain Imaging Behav 2021;15:2481-91. [Crossref] [PubMed]
- Huang XQ, Lui S, Deng W, Chan RC, Wu QZ, Jiang LJ, Zhang JR, Jia ZY, Li XL, Li F, Chen L, Li T, Gong QY. Localization of cerebral functional deficits in treatment-naive, first-episode schizophrenia using resting-state fMRI. Neuroimage 2010;49:2901-6. [Crossref] [PubMed]
- Pan P, Zhu L, Yu T, Shi H, Zhang B, Qin R, Zhu X, Qian L, Zhao H, Zhou H, Xu Y. Aberrant spontaneous low-frequency brain activity in amnestic mild cognitive impairment: A meta-analysis of resting-state fMRI studies. Ageing Res Rev 2017;35:12-21. [Crossref] [PubMed]
- Zang YF, He Y, Zhu CZ, Cao QJ, Sui MQ, Liang M, Tian LX, Jiang TZ, Wang YF. Altered baseline brain activity in children with ADHD revealed by resting-state functional MRI. Brain Dev 2007;29:83-91. [Crossref] [PubMed]
- Yang L, Yan Y, Wang Y, Hu X, Lu J, Chan P, Yan T, Han Y. Gradual Disturbances of the Amplitude of Low-Frequency Fluctuations (ALFF) and Fractional ALFF in Alzheimer Spectrum. Front Neurosci 2018;12:975. [Crossref] [PubMed]
- Liang P, Xiang J, Liang H, Qi Z, Li K. Alzheimer's Disease NeuroImaging Initiative. Altered amplitude of low-frequency fluctuations in early and late mild cognitive impairment and Alzheimer's disease. Curr Alzheimer Res 2014;11:389-98. [Crossref] [PubMed]
- Wang S, Rao J, Yue Y, Xue C, Hu G, Qi W, Ma W, Ge H, Zhang F, Zhang X, Chen J. Altered Frequency-Dependent Brain Activation and White Matter Integrity Associated With Cognition in Characterizing Preclinical Alzheimer's Disease Stages. Front Hum Neurosci 2021;15:625232. [Crossref] [PubMed]
- Sun Y, Dai Z, Li Y, Sheng C, Li H, Wang X, Chen X, He Y, Han Y. Subjective Cognitive Decline: Mapping Functional and Structural Brain Changes-A Combined Resting-State Functional and Structural MR Imaging Study. Radiology 2016;281:185-92. [Crossref] [PubMed]
- Han Y. Recommendations for diagnosis and treatment of subjective cognitive decline due to preclinical Alzheimer disease in China. Journal of China Clinic Medical Imaging 2018;29:534-8.
- Blom K, Koek HL, Zwartbol MHT, van der Graaf Y, Kesseler L, Biessels GJ, Geerlings MI. Subjective cognitive decline, brain imaging biomarkers, and cognitive functioning in patients with a history of vascular disease: the SMART-Medea study. Neurobiol Aging 2019;84:33-40. [Crossref] [PubMed]
- Lam LC, Tam CW, Lui VW, Chan WC, Chan SS, Ho KS, Chan WM, Chiu HF. Use of clinical dementia rating in detecting early cognitive deficits in a community-based sample of Chinese older persons in Hong Kong. Alzheimer Dis Assoc Disord 2008;22:153-7. [Crossref] [PubMed]
- Swanwick GR, Coen RF, Lawlor BA, O'Mahony D, Walsh JB, Coakley D. Utility of ischemic scores in the differential diagnosis of Alzheimer's disease and ischemic vascular dementia. Int Psychogeriatr 1996;8:413-24. [Crossref] [PubMed]
- Folstein MF, Folstein SE, McHugh PR. “Mini-mental state”. A practical method for grading the cognitive state of patients for the clinician. J Psychiatr Res 1975;12:189-98. [Crossref] [PubMed]
- Zhao Q, Lv Y, Zhou Y, Hong Z, Guo Q. Short-term delayed recall of auditory verbal learning test is equivalent to long-term delayed recall for identifying amnestic mild cognitive impairment. PLoS One 2012;7:e51157. [Crossref] [PubMed]
- Perrochon A, Kemoun G. The Walking Trail-Making Test is an early detection tool for mild cognitive impairment. Clin Interv Aging 2014;9:111-9. [Crossref] [PubMed]
- Ardila A. A cross-linguistic comparison of category verbal fluency test (ANIMALS): a systematic review. Arch Clin Neuropsychol 2020;35:213-25. [Crossref] [PubMed]
- Chen TB, Lin CY, Lin KN, Yeh YC, Chen WT, Wang KS, Wang PN. Culture qualitatively but not quantitatively influences performance in the Boston naming test in a chinese-speaking population. Dement Geriatr Cogn Dis Extra 2014;4:86-94. [Crossref] [PubMed]
- Wang J, Zou YZ, Cui JF, Fan HZ, Chen R, Chen N, Yao J, Duan JH, Yan LJ, He XL, Jiang X. Revision of the Wechsler memory scale fourth edition of Chinese version (adult battery). Chinese Mental Health Journal 2013;29:53-9.
- Sullivan K. Estimates of interrater reliability for the Logical Memory subtest of the Wechsler Memory Scale-Revised. J Clin Exp Neuropsychol 1996;18:707-12. [Crossref] [PubMed]
- Hester RL, Kinsella GJ, Ong B. Effect of age on forward and backward span tasks. J Int Neuropsychol Soc 2004;10:475-81. [Crossref] [PubMed]
- Cherbuin N, Sachdev P, Anstey KJ. Neuropsychological predictors of transition from healthy cognitive aging to mild cognitive impairment: The PATH through life study. Am J Geriatr Psychiatry 2010;18:723-33. [Crossref] [PubMed]
- Lam CL, Gandek B, Ren XS, Chan MS. Tests of scaling assumptions and construct validity of the Chinese (HK) version of the SF-36 Health Survey. J Clin Epidemiol 1998;51:1139-47. [Crossref] [PubMed]
- Li X, Wang X, Su L, Hu X, Han Y. Sino Longitudinal Study on Cognitive Decline (SILCODE): protocol for a Chinese longitudinal observational study to develop risk prediction models of conversion to mild cognitive impairment in individuals with subjective cognitive decline. BMJ Open 2019;9:e028188. [Crossref] [PubMed]
- Winkler AM, Renaud O, Smith SM, Nichols TE. Permutation inference for canonical correlation analysis. Neuroimage 2020;220:117065. [Crossref] [PubMed]
- Guo QH, Sun YT, Yu PM, Hong Z. Norms of Auditory Word Learning Test for Community Elderly. Chinese Journal of Clinical Psychology 2007;132-134, 141.
- Scheltens P, De Strooper B, Kivipelto M, Holstege H, Chételat G, Teunissen CE, Cummings J, van der Flier WM. Alzheimer's disease. Lancet 2021;397:1577-90. [Crossref] [PubMed]
- Truchot L, Costes N, Zimmer L, Laurent B, Le Bars D, Thomas-Antérion C, Mercier B, Hermier M, Vighetto A, Krolak-Salmon P. A distinct [18F]MPPF PET profile in amnestic mild cognitive impairment compared to mild Alzheimer's disease. Neuroimage 2008;40:1251-6. [Crossref] [PubMed]
- Cui L, Zhang Z, Zac Lo CY, Guo Q. Local Functional MR Change Pattern and Its Association With Cognitive Function in Objectively-Defined Subtle Cognitive Decline. Front Aging Neurosci 2021;13:684918. [Crossref] [PubMed]
- Peterson AC, Li CR. Noradrenergic Dysfunction in Alzheimer's and Parkinson's Diseases-An Overview of Imaging Studies. Front Aging Neurosci 2018;10:127. [Crossref] [PubMed]
- Raichle ME. The brain's default mode network. Annu Rev Neurosci 2015;38:433-47. [Crossref] [PubMed]
- Smallwood J, Bernhardt BC, Leech R, Bzdok D, Jefferies E, Margulies DS. The default mode network in cognition: a topographical perspective. Nat Rev Neurosci 2021;22:503-13. [Crossref] [PubMed]
- Yuan LQ, Zeng Q, Wang D, Wen XY, Shi Y, Zhu F, Chen SJ, Huang GZ. Neuroimaging mechanisms of high-frequency repetitive transcranial magnetic stimulation for treatment of amnestic mild cognitive impairment: a double-blind randomized sham-controlled trial. Neural Regen Res 2021;16:707-13. [Crossref] [PubMed]
- Cao Z, Bennett M, Orr C, Icke I, Banaschewski T, Barker GJ, et al. Mapping adolescent reward anticipation, receipt, and prediction error during the monetary incentive delay task. Hum Brain Mapp 2019;40:262-83. [Crossref] [PubMed]
- Liu X, Chen W, Tu Y, Hou H, Huang X, Chen X, Guo Z, Bai G, Chen W. The Abnormal Functional Connectivity between the Hypothalamus and the Temporal Gyrus Underlying Depression in Alzheimer's Disease Patients. Front Aging Neurosci 2018;10:37. [Crossref] [PubMed]
- Chaudhary S, Zhornitsky S, Chao HH, van Dyck CH, Li CR. Hypothalamic Functional Connectivity and Apathy in People with Alzheimer's Disease and Cognitively Normal Healthy Controls. J Alzheimers Dis 2022;90:1615-28. [Crossref] [PubMed]
- Lin L, Xing G, Han Y. Advances in Resting State Neuroimaging of Mild Cognitive Impairment. Front Psychiatry 2018;9:671. [Crossref] [PubMed]
- Mak E, Gabel S, Su L, Williams GB, Arnold R, Passamonti L, Vazquez Rodríguez P, Surendranathan A, Bevan-Jones WR, Rowe JB, O'Brien JT. Multi-modal MRI investigation of volumetric and microstructural changes in the hippocampus and its subfields in mild cognitive impairment, Alzheimer's disease, and dementia with Lewy bodies. Int Psychogeriatr 2017;29:545-55. [Crossref] [PubMed]
- Zhu Y, Gao Y, Guo C, Qi M, Xiao M, Wu H, Ma J, Zhong Q, Ding H, Zhou Q, Ali N, Zhou L, Zhang Q, Wu T, Wang W, Sun C, Thabane L, Zhang L, Wang T. Effect of 3-Month Aerobic Dance on Hippocampal Volume and Cognition in Elderly People With Amnestic Mild Cognitive Impairment: A Randomized Controlled Trial. Front Aging Neurosci 2022;14:771413. [Crossref] [PubMed]
- Chaudhary S, Zhornitsky S, Chao HH, van Dyck CH, Li CR. Emotion Processing Dysfunction in Alzheimer's Disease: An Overview of Behavioral Findings, Systems Neural Correlates, and Underlying Neural Biology. Am J Alzheimers Dis Other Demen 2022;37:15333175221082834. [Crossref] [PubMed]
- Pinto TCC, Machado L, Bulgacov TM, Rodrigues-Júnior AL, Costa MLG, Ximenes RCC, Sougey EB. Is the Montreal Cognitive Assessment (MoCA) screening superior to the Mini-Mental State Examination (MMSE) in the detection of mild cognitive impairment (MCI) and Alzheimer’s Disease (AD) in the elderly? Int Psychogeriatr 2019;31:491-504. [Crossref] [PubMed]
- Kang JM, Cho YS, Park S, Lee BH, Sohn BK, Choi CH, Choi JS, Jeong HY, Cho SJ, Lee JH, Lee JY. Montreal cognitive assessment reflects cognitive reserve. BMC Geriatr 2018;18:261. [Crossref] [PubMed]
- Ni L, Liu R, Yin Z, Zhao H, Nedelska Z, Hort J, Zhou F, Wu W, Zhang X, Li M, Yu H, Zhu B, Xu Y, Zhang B. Aberrant Spontaneous Brain Activity in Patients with Mild Cognitive Impairment and concomitant Lacunar Infarction: A Resting-State Functional MRI Study. J Alzheimers Dis 2016;50:1243-54. [Crossref] [PubMed]
- Whyte AR, Rahman S, Bell L, Edirisinghe I, Krikorian R, Williams CM, Burton-Freeman B. Improved metabolic function and cognitive performance in middle-aged adults following a single dose of wild blueberry. Eur J Nutr 2021;60:1521-36. [Crossref] [PubMed]
- Kongkeaw C, Dilokthornsakul P, Thanarangsarit P, Limpeanchob N, Norman Scholfield C. Meta-analysis of randomized controlled trials on cognitive effects of Bacopa monnieri extract. J Ethnopharmacol 2014;151:528-35. [Crossref] [PubMed]
- Li T, Zhu X, Wu X, Gong Y, Jones JA, Liu P, Chang Y, Yan N, Chen X, Liu H. Continuous theta burst stimulation over left and right supramarginal gyri demonstrates their involvement in auditory feedback control of vocal production. Cereb Cortex 2022;33:11-22. [Crossref] [PubMed]
- Celsis P, Boulanouar K, Doyon B, Ranjeva JP, Berry I, Nespoulous JL, Chollet F. Differential fMRI responses in the left posterior superior temporal gyrus and left supramarginal gyrus to habituation and change detection in syllables and tones. Neuroimage 1999;9:135-44. [Crossref] [PubMed]
- Guo Z, Jiang Z, Jiang B, McClure MA, Mu Q. High-Frequency Repetitive Transcranial Magnetic Stimulation Could Improve Impaired Working Memory Induced by Sleep Deprivation. Neural Plast 2019;2019:7030286. [Crossref] [PubMed]
- Zou QH, Zhu CZ, Yang Y, Zuo XN, Long XY, Cao QJ, Wang YF, Zang YF. An improved approach to detection of amplitude of low-frequency fluctuation (ALFF) for resting-state fMRI: fractional ALFF. J Neurosci Methods 2008;172:137-41. [Crossref] [PubMed]