Added-value of 3D amide proton transfer MRI in assessing prognostic factors of cervical cancer: a comparative study with multiple model diffusion-weighted imaging
Introduction
Despite improvements in prevention, screening, and treatment, cervical cancer continues to significantly impact women’s health worldwide (1). The prognosis of cervical cancer depends on tumor size, clinical stage, and some histopathological features, including tumor subtype and grade, and lymph node status (2). The presence of lymphovascular space invasion (LVSI) in early-stage tumors has a higher rate of lymph node metastases and increases the risk of recurrence from 2% to 31% at 3 years (3,4). Both the updated International Federation of Gynecology and Obstetrics (FIGO) and European Society of Urogenital Radiology (ESUR) system give added importance to magnetic resonance imaging (MRI) as a method of accurately measuring tumor size and depicting the presence of deep stromal infiltration and parametrial involvement (5,6). Although conventional MRI provides morphological evaluation for cervical cancer, it is challenging to reflect tumor microstructure without specific metrics. Different advanced MRI modalities, including molecular imaging, may provide additional complementary information for clinical diagnosis and prognostic assessment, which may help optimize the therapeutic benefit (7-9).
Diffusion-weighted imaging (DWI) plays an essential role in cervical cancer assessment by providing both excellent visual definition of tumors due to high signal intensity (SI) and quantitative information that is obtained from various mathematical models. Mono-exponential DWI-derived apparent diffusion coefficient (ADC) has been most commonly used and inversely correlated with tumor cellularity. However, the water diffusion within tissues can be complicated, for example, the capillary blood microcirculation can contribute to the water diffusion, and affect the ADC value (10). Thus, the extended intravoxel incoherent motion (IVIM) model and kurtosis model, named diffusion kurtosis imaging (DKI), have been used to reflect the biological characterization of cervical cancer (11,12). Different from DWI, amide proton transfer (APT) imaging has recently emerged as a novel contrast agent-free MRI technique in the field of cellular and molecular imaging, which generates image contrast based on endogenous cytosolic proteins and peptides with amide protons in the peptide bond (13). This technique has been successfully applied to brain tumor, prostate cancer, endometrial carcinoma, breast cancer, and bladder cancer (14-18). Some studies have reported the potential of APT SI in differentiating the histological subtype and grade of cervical cancer (9,19,20). However, in most studies, the APT imaging has been limited to a 2-dimensional (2D) acquisition, which could not provide coverage of the whole tumor (19,20). Comparatively 3-dimensional (3D) turbo spin-echo (TSE) APT imaging, with improved B0 field correction and image reconstruction, enables more and comprehensive analysis of the whole tumor volume (21).
Although several studies have applied APT imaging or multiple diffusion models in the evaluation of cervical cancer, they were all primarily focused on the application of mean values (9,11,19,20). Due to tumor heterogeneity, the maximum or minimum values of diffusion and APT metrics within the whole lesion may also reflect the underlying aggressiveness of the tumor. Ghosh et al. found that ADCmin was more effective than ADCmean in differentiating neuroblastoma with and without MYCN amplification (22). Zhuo et al. reported that higher APT SImax were observed in H3K27M-mutant glioma than those with wildtype, whereas no significant differences of APT SImean were observed between groups (23). Therefore, the purpose of our study was to (I) compare the diagnostic performances of mean, minimum, and maximum values of APT SI for preoperative identifying the prognostic factors (tumor stage, subtype, grade, and LVSI status) of cervical cancer with those of multi-model DWI, and (II) examine the additive value of 3D-APT imaging combined with multi-model DWI for the preoperative characterization of cervical cancer. We present this article in accordance with the STARD reporting checklist (available at https://qims.amegroups.com/article/view/10.21037/qims-23-324/rc).
Methods
Participants
The study was conducted in accordance with the Declaration of Helsinki (as revised in 2013). This prospective single-center study was approved by the institutional ethics board of The First Affiliated Hospital of Zhengzhou University, and all patients provided written informed consent prior to enrollment in the study. From January 2020 to April 2022, 106 patients in The First Affiliated Hospital of Zhengzhou University were selected consecutively. The inclusion criteria were as follows: (I) pathological diagnosis of cervical cancer; (II) no treatment before MR examination; (III) the availability of data from preoperative MRI, including DWI and APT imaging. The exclusion criteria were as follows: (I) rare tumors such as adenosquamous carcinoma and neuroendocrine carcinoma (n=7); (II) poor image quality (n=5); (III) tumor visibility on only one MRI slice (n=6). Finally, a total of 88 patients were enrolled in the study. Among the patients, 53 underwent surgery and 35 underwent biopsy for final diagnoses. According to the 2018 FIGO staging system (5) and histopathology results, the patients were divided into an early-stage group (< IIB) and an advanced-stage group (≥ IIB), an adenocarcinoma (AC) group and a squamous cell carcinoma (SCC) group, a high-grade group (poorly differentiated tumor) and a low-grade group (well/moderately differentiated tumor), and an LVSI-negative group and an LVSI-positive group.
Image acquisition
Cervical MR scanning was performed using a 3T system (Ingenia CX, Philips Healthcare, Best, The Netherlands) with an external pelvic phased array coil. Patients were asked to fast for at least 6 hours prior to the examination and were placed in the head-first supine position throughout the examination. The following sequences were acquired: axial T2-weighted TSE imaging, DWI, and APT imaging. APT imaging was conducted using an optimized 3D TSE pulse sequence combined with chemical shift-selective fat suppression for better robustness to field inhomogeneity and 3D volume coverage. The pre-saturation pulses consisted of three consecutive radiofrequency pulses of 500 ms duration with 500 ms interpulse delays and a time-average amplitude of 2 µT. We acquired 6 different image volumes at saturation frequencies (±3.5, ±3.42, ±3.58, and −1,560, relative to the water resonance frequency) were acquired for the Z-spectrum normalization and interpolation, and three acquisitions were performed at +3.5 ppm with different echo time shifts to obtain a Dixon-type B0 field map to correct for B0 inhomogeneities in the Z-spectrum frequency domain. The total scan time for the APT imaging (additional time for setup, 7–10 seconds; acquisition time for APT images, 6 minutes) was 6 minutes 7 seconds to 6 minutes 10 seconds. DWI was acquired via a single-shot echo-planar imaging (EPI) sequence for b =0, 10, 20, 50, 100, 200, 400, 800, 1,200, 1,600, and 2,000 s/mm2. Diffusion gradients were applied simultaneously along with 3 orthogonal directions. The scan time for DWI was 5 minutes 43 seconds to 5 minutes 48 seconds. The total scan time of this study protocol was 16 minutes 25 seconds to 16 minutes 37 seconds. Detailed descriptions of MRI parameters are listed in Table 1.
Table 1
Parameters | T2WI | DWI | APT imaging |
---|---|---|---|
Sequence/orientation | TSE/axial | EPI/axial | TSE/axial |
TR (ms) | 4,093 | 5,614 | 3,000 |
TE (ms) | 100 | 103 | 1,000 |
Field of view (mm2) | 200×200 | 240×240 | 180×180 |
Slice thickness (mm) | 5 | 5 | 5 |
No. of slices | 24 | 24 | 9 |
Matrix | 344×306 | 72×67 | 116×90 |
Voxel size (mm3) | 0.58×0.65×5.00 | 3.33×3.58×5.0 | 2×2×5.0 |
b-values (s/mm2) | NA | 0, 10, 20, 50, 100, 200, 400, 800, 1,200, 1,600, 2,000 | NA |
Flip angle (degrees) | 90 | 90 | 90 |
Bandwidth (Hz/pixel) | 250.6 | 1,784.8 | 647.2 |
Fat suppression | No | SPAIR | SPAIR |
Acquisition time | 4 min 30 s | 5 min 37 s | 6 min 0 s |
T2WI, T2-weighted imaging; DWI, diffusion-weighted imaging; APT, amide proton transfer; TSE, turbo spin-echo; EPI, echo-planar imaging; TR, repetition time; TE, echo time; NA, not applicable; SPAIR, spectral attenuation with inversion recovery.
Image analysis
APT images were acquired online at the MR control console. All data were transferred to a post-processing station (IntelliSpace Portal V10, Philips Healthcare) for quantitative analysis. The APT SI was calculated as the asymmetry of the magnetization transfer rate (MTRasym) at the frequency offset of +3.5 ppm using the following equation:
DWI data were loaded into the application of advanced diffusion analysis (ADA) to generate parameter maps of the three diffusion models. The mono-exponential model was applied to estimate the ADC with 2 b-values (0 and 800 s/mm2). The following linear fitting equation was implemented:
where S0 and Sb represent the MR SI under different b values (0 s/mm2 and other values, respectively).
The calculation of the IVIM model used 9 b-values (0, 10, 20, 50, 100, 200, 400, 800, and 1,200 s/mm2). The following fitting equation was implemented:
where f is the perfusion fraction, D is the diffusion coefficient representing water diffusion in the extravascular space, and D* is the pseudo diffusion coefficient representing incoherent microcirculation within the vascular space.
The calculation of the DKI model used 8 b-values (0, 100, 200, 400, 800, 1,200, 1,600 and 2,000 s/mm2). The following fitting equation was implemented:
where D is the corrected ADC derived from the non-Gaussian model, and K is a unitless metric of the apparent kurtosis coefficient. MD and MK are the averages of D and K among three distributed directions.
The image data were independently analyzed by two radiologists (Li S and Liu J, with over 7 years of experience in uterine MR diagnosis) who were unaware of the patient’s clinical and pathologic information. The regions of interest (ROIs) of tumor area were defined on every section of DWI original map (b =1,200 s/mm2) where mass was visible with reference to corresponding T2-weighted images. Areas of necrosis and hemorrhage were avoided. Subsequently, ROIs were copied to APT, ADC, D, D*, f, MK, and MD images, using a built-in automatic coregistration tool. Registrations were visually inspected and manually corrected if necessary. The average values of each measurement were recorded as APT SImean, ADCmean, Dmean, D*mean, fmean, MKmean, and MDmean of the tumor, respectively. The lowest APT SI, ADC, D, and MD values of the whole tumor in all selected section were selected as the APT SImin, ADCmin, Dmin, and MDmin. Similarly, the largest APT SI and MK value of the whole tumor was selected as the APT SImax, and MKmax.
Statistical analysis
The values of MR quantitative metrics were expressed as means ± standard deviations (SDs). The measured data were evaluated for normality using Shapiro-Wilk test. The unpaired Student’s t-test was used to compare the continuous variables with normal distribution, whereas Mann-Whitney U test was used to compare variables with nonnormal distribution. Interclass correlation coefficient (ICC) was calculated to evaluate interobserver agreement on the measured metrics. ICC values greater than 0.8 were considered excellent agreement, 0.6–0.8, good; 0.4–0.6, moderate; and less than 0.4, poor agreement. Receiver operating characteristic (ROC) curves were generated to examine the ability of each metric to distinguish different prognostic factors when appropriate. The discriminating power of each metric was quantified using the area under the curve (AUC). The differences of AUCs were compared using DeLong test. Logistic regression analyses were used to identify independent factors and combination diagnosis.
Statistical analyses were performed using SPSS 22.0 (IBM Corp., Armonk, NY, USA) and MedCalc V19.0 (MedCalc Software, Mariakerke, Belgium). The standard for statistical significance was set at P<0.05.
Results
Study population
The final study population consisted of 88 patients with pathologically diagnosed cervical cancer (Figure 1). Table 2 gives an overview of the clinical characteristics of the patients. The interval between MR examination and treatment was 1 to 15 days, with a median of 6 days.
Table 2
Clinicopathologic characteristics | Values |
---|---|
Age (years), mean ± SD | 53.4±12.0 |
FIGO stage, n | |
IB | 24 |
IIA | 29 |
IIB | 8 |
IIIA | 3 |
IIIB | 1 |
IIIC | 22 |
IVA | 1 |
Histological type, n | |
SCC | 72 |
AC | 16 |
Histological grade, n | |
Low-grade (well/moderately differentiated) | 36 |
High-grade (poorly differentiated) | 28 |
Unknown† | 24 |
LVSI, n | |
Positive | 27 |
Negative | 26 |
Unknown‡ | 35 |
†, cases are of unknown tumor differentiation as a result of insignificant biopsy specimen size, which was excluded when analyzing tumor grade; ‡, cases are of unknown LVSI status as a result of insignificant biopsy specimen size, which was excluded when analyzing tumor LVSI. SD, standard deviation; FIGO, International Federation of Gynecology and Obstetrics; SCC, squamous cell carcinoma; AC, adenocarcinoma; LVSI, lymphovascular space invasion.
Interobserver reliability
The interobserver agreements for the MR metrics were good or excellent. The ICCs were as follows: APT SImean, 0.820; APT SImax, 0.874; APT SImin, 0.792; ADCmean, 0.863; ADCmin, 0.845; Dmean, 0.857; Dmin, 0.817; D*mean, 0.666; fmean, 0.769; MKmean, 0.824; MKmax, 0.815; MDmean, 0.881; and MDmin, 0.856. Therefore, two observers’ mean results of each metric were used for subsequent analysis.
Comparison of metrics in different stages, subtypes, grades, and LVSI status
No significant differences were detected in APT- and DWI-derived metrics for differentiating early-stage and advanced-stage cervical cancer (all P>0.05). APT SImax, APT SImin, MKmean, and MKmax showed significant differences between SCC and AC groups (all P<0.05). APT SImax, MKmean, and MKmax were higher and APT SImin were lower in AC than in SCC groups. There were no significantly different metrics from mono-exponential and IVIM diffusion models between SCC group and AC group (all P>0.05). APT SImean, APT SImax, ADCmin, Dmean, Dmin, MDmin, and MKmax showed significant differences between the high-grade group and low-grade group (all P<0.05). APT SImean, APT SImax, and MKmax were higher and ADCmin, Dmean, Dmin, and MDmin were lower in the high-grade group. For distinguishing LVSI, only MKmean showed significant difference between LVSI-positive group and LVSI-negative group (P<0.05). The detailed results are shown in Table 3. Images from conventional T2-weighted, APT imaging, and the 3 diffusion models of representative images are shown in Figures 2,3. More corresponding case examples of patients with cervical cancer are shown in Figures S1-S6.
Table 3
Parameters | Tumor stage | Tumor subtype | Tumor grade | LVSI status | |||||||||||
---|---|---|---|---|---|---|---|---|---|---|---|---|---|---|---|
Early (n=53) |
Advanced (n=35) |
P value | SCC (n=72) |
AC (n=16) |
P value | High-grade (n=28) | Low-grade (n=36) | P value | Positive (n=27) | Negative (n=26) | P value | ||||
APT SImean (%) | 2.82±0.65 | 2.66±0.87 | 0.095† | 2.74±0.79 | 2.84±0.53 | 0.312† | 3.15±0.95 | 2.67±0.53 | 0.008**† | 2.87±0.55 | 2.74±0.75 | 0.483‡ | |||
APT SImax (%) | 5.28±1.41 | 5.68±1.77 | 0.308† | 5.24±1.45 | 6.38±1.78 | 0.017**† | 6.27±1.85 | 4.92±1.23 | 0.002**† | 5.59±1.63 | 5.02±1.09 | 0.206† | |||
APT SImin (%) | 0.07±1.47 | 0.13±1.23 | 0.846‡ | 0.30±1.23 | −0.83±1.62 | 0.002**‡ | 0.12±1.59 | 0.37±1.11 | 0.470‡ | 0.26±1.32 | 0.10±1.44 | 0.673‡ | |||
ADCmean (×10−3 mm2/s) | 0.84±0.19 | 0.84±0.14 | 0.695† | 0.84±0.18 | 0.84±0.15 | 0.948† | 0.80±0.15 | 0.88±0.19 | 0.167† | 0.81±0.10 | 0.88±0.24 | 0.119† | |||
ADCmin (×10−3 mm2/s) | 0.52±0.14 | 0.52±0.14 | 0.852‡ | 0.53±0.15 | 0.48±0.09 | 0.218‡ | 0.46±0.11 | 0.57±0.15 | 0.002**‡ | 0.53±0.11 | 0.52±0.19 | 0.745‡ | |||
Dmean (×10−3 mm2/s) | 0.70±0.12 | 0.71±0.09 | 0.831† | 0.71±0.11 | 0.70±0.12 | 0.665† | 0.68±0.13 | 0.74±0.09 | 0.038**† | 0.70±0.10 | 0.71±0.13 | 0.294† | |||
Dmin (×10−3 mm2/s) | 0.41±0.13 | 0.43±0.10 | 0.739† | 0.43±0.12 | 0.38±0.11 | 0.176‡ | 0.37±0.11 | 0.46±0.12 | 0.004**‡ | 0.43±0.11 | 0.40±0.14 | 0.374‡ | |||
D*mean (×10−3 mm2/s) | 44.26±18.93 | 43.02±17.53 | 0.759‡ | 44.92±17.48 | 38.56±21.42 | 0.210‡ | 46.74±19.51 | 45.08±18.00 | 0.726‡ | 47.94±19.57 | 44.12±18.16 | 0.464‡ | |||
fmean | 0.16±0.04 | 0.18±0.11 | 0.711† | 0.16±0.05 | 0.20±0.14 | 0.086† | 0.17±0.11 | 0.17±0.04 | 0.261† | 0.15±0.03 | 0.17±0.05 | 0.117† | |||
MKmean | 0.77±0.21 | 0.82±0.18 | 0.218‡ | 0.75±0.17 | 0.96±0.23 | 0.001**† | 0.80±0.21 | 0.73±0.17 | 0.282† | 0.82±0.17 | 0.69±0.21 | 0.010**‡ | |||
MKmax | 1.48±0.35 | 1.50±0.32 | 0.597† | 1.45±0.30 | 1.67±0.43 | 0.044**† | 1.59±0.35 | 1.38±0.26 | 0.004**† | 1.45±0.31 | 1.46±0.33 | 0.769† | |||
MDmean (×10−3 mm2/s) | 1.03±0.18 | 1.05±0.15 | 0.848† | 1.04±0.17 | 1.05±0.20 | 0.996† | 1.00±0.18 | 1.08±0.17 | 0.161† | 1.03±0.12 | 1.06±0.22 | 0.650† | |||
MDmin (×10−3 mm2/s) | 0.55±0.14 | 0.54±0.13 | 0.718‡ | 0.56±0.14 | 0.52±0.11 | 0.303‡ | 0.47±0.10 | 0.61±0.14 | <0.001**‡ | 0.55±0.13 | 0.55±0.16 | 0.888‡ |
Data are presented as mean ± standard deviation. **, indicates the comparison with statistical significance; †, comparisons were performed by Mann-Whitney U test; ‡, comparisons were performed by independent t-test. APT, amide proton transfer; DWI, diffusion-weighted imaging; LVSI, lymphovascular space invasion; SCC, squamous cell carcinoma; AC, adenocarcinoma; SI, signal intensity; ADC, apparent diffusion coefficient; D, pure molecular diffusion; D*, pseudo-diffusion; f, perfusion fraction; MK, mean kurtosis; MD, mean diffusivity.
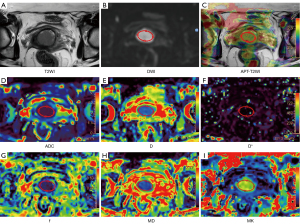
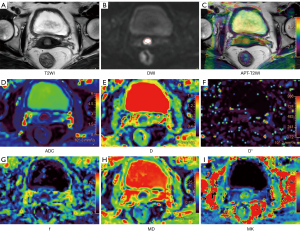
Univariate and multivariate logistic regression analyses
Univariate logistic analysis revealed that APT SImax, APT SImin, MKmean, and MKmax were predictors for the histological subtype of cervical cancer, and APT SImean, APT SImax, ADCmin, Dmin, MDmin, and MKmax were predictors for the histological grade of cervical cancer. For APT metrics, multivariate logistic analysis showed that APT SImax was the independent predictor for both subtype and grade of cervical cancer, and APT SImin was the independent predictor for tumor subtype. For DWI metrics, only MKmean was an independent predictor for the histological subtype, and MDmin was an independent predictor for the histological grade of cervical cancer (Table 4).
Table 4
Parameters | SCC and AC (univariate analyses) |
SCC and AC (multivariable analyses) |
High- and low-grade (univariate analyses) |
High- and low-grade (multivariable analyses) |
|||||||
---|---|---|---|---|---|---|---|---|---|---|---|
OR (95% CI) | P value | OR (95% CI) | P value | OR (95% CI) | P value | OR (95% CI) | P value | ||||
APT SImean (%) | 1.129 (0.676–1.884)* | 0.644 | – | – | 2.085 (1.062–4.095)* | 0.033** | 1.949 (0.664–5.720)* | 0.225 | |||
APT SImax (%) | 1.966 (1.156–3.344)* | 0.013** | 2.347 (1.090–5.053)* | 0.029** | 2.532 (1.329–4.822)* | 0.005** | 2.885 (1.028–8.096)* | 0.044** | |||
APT SImin (%) | 0.432 (0.236–0.791)* | 0.006** | 0.352 (0.142–0.874)* | 0.024** | 0.796 (0.471–1.344)* | 0.393 | – | – | |||
ADCmean (×10−3 mm2/s) | 0.957 (0.548–1.673)* | 0.878 | – | – | 0.572 (0.288–1.137)* | 0.111 | – | – | |||
ADCmin (×10−3 mm2/s) | 0.702 (0.400–1.232)* | 0.217 | – | – | 0.424 (0.221–0.815)* | 0.010** | 2.002 (0.392–10.220)* | 0.404 | |||
Dmean (×10−3 mm2/s) | 0.937 (0.546–1.609)* | 0.814 | – | – | 0.550 (0.293–1.033)* | 0.063 | – | – | |||
Dmin (×10−3 mm2/s) | 0.693 (0.407–1.181)* | 0.177 | – | – | 0.462 (0.254–0.840)* | 0.011** | 1.641 (0.403–6.678)* | 0.489 | |||
D*mean (×10−3 mm2/s) | 0.686 (0.381–1.237)* | 0.210 | – | – | 1.085 (0.663–1.774)* | 0.746 | – | – | |||
fmean | 1.538 (0.910–2.598)* | 0.108 | – | – | 1.049 (0.659–1.669)* | 0.841 | – | – | |||
MKmean | 3.797 (1.833–7.862)* | <0.001** | 6.523 (2.081–20.441)* | 0.001** | 1.495 (0.833–2.683)* | 0.178 | – | – | |||
MKmax | 1.815 (1.072–3.071)* | 0.026** | 0.921 (0.376–2.258)* | 0.858 | 2.168 (1.144–4.109)* | 0.018** | 3.856 (0.991–15.008)* | 0.052 | |||
MDmean (×10−3 mm2/s) | 1.062 (0.616–1.830)* | 0.829 | – | – | 0.613 (0.344–1.093)* | 0.097 | – | – | |||
MDmin (×10−3 mm2/s) | 0.748 (0.432–1.296)* | 0.301 | – | – | 0.269 (0.127–0.571)* | 0.001** | 0.155 (0.036–0.662)* | 0.012** |
All factors with P<0.05 in univariate analyses were included in multivariate regression analyses. *, OR for per 1 standard deviation; **, indicates the logistic regression analyses with statistical significance. SCC, squamous cell carcinoma; AC, adenocarcinoma; OR, odds ratio; CI, confidence interval; APT SI, amide proton transfer signal intensity; ADC, apparent diffusion coefficient; D, pure molecular diffusion; D*, pseudo-diffusion; f, perfusion fraction; MK, mean kurtosis; MD, mean diffusivity.
Diagnostic performance of APT and DWI metrics
Table 5 and Figure 4 summarize the results of ROC curve analysis for significantly different metrics. The AUC for MKmean to predict LVSI was 0.689. The AUCs for MKmean, APT SImin, APT SImax, and MKmax to differentiate SCC and AC were 0.760, 0.708, 0.691, and 0.661, respectively. For distinguishing between high-grade and low-grade tumor, MDmin achieved the highest AUC (0.810), followed by ADCmin (0.740), APT SImax (0.731), Dmin (0.727), MKmax (0.712), APT SImean (0.693), and Dmean (0.652).
Table 5
Category | Threshold | AUC (95% CI) | Sensitivity | Specificity | P value |
---|---|---|---|---|---|
SCC vs. AC | |||||
APT SImax (%) | 4.91 | 0.691 (0.584–0.786) | 81.2% | 51.4% | 0.008 |
APT SImin (%) | −0.65 | 0.708 (0.601–0.800) | 62.5% | 80.6% | 0.005 |
MKmean | 0.91 | 0.760 (0.657–0.844) | 68.7% | 88.9% | <0.001 |
MKmax | 1.54 | 0.661 (0.553–0.759) | 56.2% | 73.6% | 0.042 |
Combined diagnosis† | NA | 0.908 (0.827–0.959) | 87.5% | 83.3% | <0.001 |
Tumor grade: high vs. low | |||||
APT SImean (%) | 2.81 | 0.693 (0.566–0.803) | 75.0% | 66.7% | 0.006 |
APT SImax (%) | 5.40 | 0.731 (0.606–0.834) | 64.3% | 75.0% | <0.001 |
ADCmin (×10−3 mm2/s) | 0.52 | 0.740 (0.615–0.841) | 75.0% | 69.4% | <0.001 |
Dmean (×10−3 mm2/s) | 0.70 | 0.652 (0.522–0.767) | 67.9% | 63.9% | 0.031 |
Dmin (×10−3 mm2/s) | 0.48 | 0.727 (0.601–0.831) | 92.9% | 47.2% | <0.001 |
MKmax | 1.52 | 0.712 (0.585–0.818) | 60.7% | 86.1% | 0.002 |
MDmin (×10−3 mm2/s) | 0.61 | 0.810 (0.693–0.897) | 100.0% | 52.8% | 0.002 |
Combined diagnosis‡ | NA | 0.903 (0.803–0.963) | 78.6% | 88.9% | <0.001 |
LVSI (+) vs. (−) | |||||
MKmean | 0.77 | 0.689 (0.547–0.809) | 55.6% | 76.9% | 0.010 |
†, the combined diagnosis represents APT SImax + APT SImin + MKmean + MKmax; ‡, the combined diagnosis represents APT SImean + APT SImax + ADCmin + MKmax + MDmin. ROC, receiver operating characteristic; APT, amide proton transfer; DWI, diffusion-weighted imaging; AUC, area under the curve; CI, confidence interval; SCC, squamous cell carcinoma; AC, adenocarcinoma; SI, signal intensity; MK, mean kurtosis; ADC, apparent diffusion coefficient; D, pure molecular diffusion; MD, mean diffusivity; LVSI, lymphovascular space invasion.

Additive value of APT imaging to DWI for differentiating tumor subtypes and grades
For distinction of SCC and AC, 15 combinations of prediction models were designed using MKmean, APT SImin, APT SImax, and MKmax. The combination of all four metrics revealed the highest AUC (0.908), which was significantly higher than that of any single metric (all P<0.05). For prediction of high-grade cervical cancer, 127 combinations of prediction models were designed using APT SImean, APT SImax, ADCmin, Dmean, Dmin, MDmin, and MKmax. The combination of APT SImean, APT SImax, ADCmin, MKmax, and MDmin revealed the highest AUC (0.903), which was significantly higher than that of any single metric (all P<0.05).
Discussion
In this study, we explored the value of multi-model DWI and APT imaging in the prediction of clinical-pathological factors in patients with cervical cancer. Our results demonstrated that both APT imaging and multi-model DWI can be used to identify the histological subtype and grade of cervical cancer. Multiparametric analysis showed that adding APT to DWI can significantly improve the diagnostic performance with an AUC of 0.908 to predict tumor subtype, and an AUC of 0.903 to predict histological grade. In addition, higher MKmean were observed in LVSI-positive tumors.
By focusing on amide protons, APT imaging has been used to visualize endogenous mobile proteins and peptides, which indirectly reflects changes of the internal environment (13). We observed a significantly higher APT SImax and a significantly lower APT SImin in AC than SCC. Cervical AC originates from endocervical cells that have abundant glandular structures and strong secretion function. This may result in increased concentrations of macromolecules in tumor tissue and consequently increased APT SImax. The APT contrast is also affected by tissue pH. Decreased APT SImin in AC may indicate the different local pH changes of the two microenvironments. However, APT SImean was unable to distinguish between SCC and AC in this study. It could be that the heterogeneity of both SCC and AC affected the endogenous mobile proteins and tissue pH in a similar extent as depicted by APT SImean. Meng et al. found that the APT SI of the SCC was significantly lower than that of AC by using a 2D EPI sequence (19). The inconsistent results may be due to the differences in the study population, sequence acquisition method, and ROI placement. To the best of our knowledge, this is the first report on the application of maximum and minimum values of APT SI in cervical cancer. Previous studies were performed at a single slice containing the maximum cross-sectional tumor area (9,19,20). In the present study, APT imaging was acquired using a 3D TSE sequence, which allowed the measurement of maximum and minimum values of APT SI based on volumetric coverage. 3D-TSE-APT imaging could provide geometrically undistorted images with high quality, and reduced lipid artifact (24).
This study also found that the APT SImean and APT SImax of the high-grade tumor were generally higher than those of the low-grade tumor, which was consistent with previous studies (9,16,18,19,25). Choi et al. confirmed that high-grade gliomas had higher concentrations of mobile proteins and peptides than low-grade gliomas (25). Li et al. stated that APT SI was positively correlated with the SCC grades (9). The pathological features of high-grade cervical cancer include a higher cell density, greater nuclear atypia, and more microscopic necrosis than low-grade cervical cancer. The increased number of tumor cells which contain more proteins and peptides may account for the increase in the APT SI in high-grade tumor (9,18,25,26). Nuclear atypia has been reported to cause an interaction between the hydrophobic cell membrane and macromolecule substance and promote the release of proteins and peptides (16,19). Necrosis may be the third possible factor of increased APT SI in high-grade tumor. Togao et al. found that the diffuse gliomas with microscopic necrosis showed higher APT SI than those without necrosis (27). The highly concentrated proteins and peptides in the extracellular space, such as microscopic necrosis or fluid collection in the microcystic space, may result in higher APT SI (27,28). Different tumor stages or different LVSI status, however, may not alter the overall macromolecular concentration or structure of the whole tumor, and the corresponding APT metrics showed no significant difference in our study.
Mono-exponential DWI, IVIM, and DKI have been widely reported to provide insight into typing and grading of cervical cancer (11,29,30). However, most studies only utilized one or two diffusion models. Our study processed three diffusion models through a single scan to predict the adverse histological features. We found that MK metrics showed significant differences between patients with different subtypes, grades and LVSI status. Moreover, diffusion metrics (ADCmin, Dmean, Dmin, and MDmin) showed significant differences between high-grade and low-grade tumor, and no DWI metrics showed significant differences between FIGO stages. These results are partially consistent with previous studies. Winfield et al. reported that perfusion metrics (D*, f), and MK were significantly different between cervical SCC and AC, and diffusion coefficients (ADC, D, and MD) were significantly different between tumor grades (11). Wang et al. found that MD and ADC exhibited significant differences between histological subtypes and FIGO stages of cervical cancer, but not with tumor grades (29). Another study demonstrated that ADC, D, D*, and f were significantly different among the 3 grades of cervical cancer (30). These mixed results could be related to a variety of reasons, such as the reproducibility of DWI metrics, the choice of b values, and different research subjects. According to a previous study, the reproducibility of IVIM metrics, especially D* and f, was significantly lower than that of ADC calculated from the mono-exponential model (31). Different b values might also cause a bias in DWI metrics. Rosenkrantz et al. suggested acquiring more than 3 b values for body DKI analysis, including at least 2 b values both above and below 1,000 s/mm2 to allow robust estimates of MK (32). In the study of Winfield et al., the highest b value was 800 s/mm2, which may not have been sufficiently high enough to capture the non-Gaussian behavior (11). Perucho et al. suggested setting at least 6 b values for reliable estimation of IVIM metrics, with at least 2 b values greater than 200 s/mm2 to estimate D and at least 2 b values less than 200 s/mm2 to calculate D* and f (33). In this study, 11 b values were acquired, among which 9 b values (0, 10, 20, 50, 100, 200, 400, 800, and 1,200 s/mm2) were selected for IVIM fitting and 8 b values (0, 100, 200, 400, 800, 1,200, 1,600, and 2,000 s/mm2) were selected for DKI fitting. The sets of b values were sufficient to estimate IVIM and DKI metrics according to the previous studies (32,33). Additionally, our study had a high proportion of SCC, whereas other studies had a more balanced distribution of SCC and AC cases (11,29); this could have influenced our results. Therefore, further studies with large samples and multiple centers are needed to confirm the role of different DWI metrics in cervical cancer evaluation.
We have also applied multivariable analysis to the identification of SCC and AC and the identification of high- and low-grade cervical cancer. Our results revealed that among APT and DWI metrics, APT SImax, APT SImin, and MKmean were the independent predictors for tumor subtype. APT SImax and MDmin were the independent predictors for the grade of cervical cancer. The combined diagnostic model (APT SImax + APT SImin + MKmean + MKmax) revealed additional value in comparison to any individual metric for distinguishing between SCC and AC with an AUC of 0.908. The combination of APT and DWI metrics also significantly improved the diagnostic performance of high-grade cervical cancer than that of individual metrics with an AUC of 0.903. The results of additional value of APT to DWI were basically consistent with the conclusions of Choi et al. and Meng et al. (25,34). Choi et al. found that APT imaging added value to the ADC for discriminating high-grade from low-grade gliomas (25). Meng et al. demonstrated that the AUC of the combination of D, MK, and APT SI was significantly higher than that of any single metric for risk stratification in early-stage endometrial carcinoma (34). These findings support the hypothesis that APT imaging complement the multi-model DWI for cervical cancer typing and grading, when the APT signal reflects a different aspect of the tumor microenvironment in comparison to the DWI metrics, namely the protein and peptide concentrations.
In addition to the benefit to cervical cancer patients from quantitative MR parameters, the practicability of APT imaging in the clinical real-world setting should also be considered. 3D APT MRI in our study required a scan time of about 6 minutes. Although the fast-imaging acquisition technique (3D TSE readout) has been used to accelerate the sequence, the use of multiple radio-frequency (RF) saturation frequencies (to correct for the B0 inhomogeneity) and multiple acquisitions (to increase the signal-to-noise ratio) extend the scan time (35). A next step in APT sequence may focus on the development of a fast 3D acquisition technique by combining some novel undersampling acquisition and reconstruction approaches (including keyhole, compressed sensing, and deep learning). It is known that quantification of APT signal is sensitive to accuracy of saturation pulse (B1), B0 inhomogeneity, and lipid artifacts (36). We used the APT imaging protocol that employed the multi-transmit RF system for the specific-frequency saturation with amplitude of 2 µT and duration of 2 seconds with sufficient B1 homogeneity. Additionally, B0 homogeneity correction was performed and the Spectral Attenuation with Inversion Recovery module was used to suppress strong lipid artifacts in the pelvic APT imaging. A previous study evaluated the image quality and clinical feasibility of 3D APT MRI for cervical cancer and showed the excellent agreement in both image quality assessment and APT values measurement (37). Lee et al. found that APT MRI showed high repeatability under the condition of the same acquisition protocol and the same MRI machine (38). However, little is known about the repeatability of APT signal measurement in different platforms. In this respect, it is encouraging to see that the recent 8th International Workshop on chemical exchange saturation transfer (CEST) Imaging [2020] provided consensus recommendations for APT imaging of brain tumors involving the three main MRI vendors. They indicated that APT imaging methods would be optimized and standardized separately for other body regions in a few years (39).
Several limitations must be considered when interpreting the present results. First, the sample size of AC patients was small due to the relatively low incidence (40). Some rare subtypes of cervical cancer such as adenosquamous carcinoma and neuroendocrine carcinomas were excluded. Our observations need to be validated in multicenter studies with maximum AC cases and other rare subtypes. Second, we only evaluated the relationship between the imaging metrics and traditional prognostic factors of cervical cancer. Several recent studies have clarified the importance of the molecular status of the tumor (40). We should conduct further studies to investigate the potential associations between imaging metrics and some molecular prognostic factors of cervical cancer (41,42). Third, the histological reference of some cases recruited in our study was based on biopsy samples; therefore, although the results are promising especially for tumor subtype characterization, further investigation with larger study cohorts confirmed by surgical histopathology should be conducted to validate our preliminary study in the future.
Conclusions
In conclusion, our findings indicate that 3D-APT imaging and multi-model DWI are useful for cervical cancer typing and grading. DKI-derived MKmean may provide helpful information to assist in the prediction of LVSI. In combination with multi-model DWI, 3D-APT showed the potential to significantly improve the performance in differentiating tumor subtypes and grades.
Acknowledgments
Funding: This study was supported by
Footnote
Reporting Checklist: The authors have completed the STARD reporting checklist. Available at https://qims.amegroups.com/article/view/10.21037/qims-23-324/rc
Conflicts of Interest: All authors have completed the ICMJE uniform disclosure form (available at https://qims.amegroups.com/article/view/10.21037/qims-23-324/coif). LL, an MR collaborating scientist from Philips Healthcare, provided technical support under the Philips collaboration regulations and had no financial or other conflicts for this study. The other authors have no conflicts of interest to declare.
Ethical Statement: The authors are accountable for all aspects of the work in ensuring that questions related to the accuracy or integrity of any part of the work are appropriately investigated and resolved. The study was conducted in accordance with the Declaration of Helsinki (as revised in 2013). The study was approved by the institutional ethics board of The First Affiliated Hospital of Zhengzhou University, and all patients provided written informed consent prior to enrollment in the study.
Open Access Statement: This is an Open Access article distributed in accordance with the Creative Commons Attribution-NonCommercial-NoDerivs 4.0 International License (CC BY-NC-ND 4.0), which permits the non-commercial replication and distribution of the article with the strict proviso that no changes or edits are made and the original work is properly cited (including links to both the formal publication through the relevant DOI and the license). See: https://creativecommons.org/licenses/by-nc-nd/4.0/.
References
- Bewley S. HPV vaccination and cervical cancer screening. Lancet 2022;399:1939. [Crossref] [PubMed]
- Park JY, Chong GO, Park JY, Chung D, Lee YH, Lee HJ, Hong DG, Han HS, Lee YS. Tumor budding in cervical cancer as a prognostic factor and its possible role as an additional intermediate-risk factor. Gynecol Oncol 2020;159:157-63. [Crossref] [PubMed]
- Hutchcraft ML, Smith B, McLaughlin EM, Hade EM, Backes FJ, O'Malley DM, Cohn DE, Fowler JM, Copeland LJ, Salani R. Conization pathologic features as a predictor of intermediate and high risk features on radical hysterectomy specimens in early stage cervical cancer. Gynecol Oncol 2019;153:255-8. [Crossref] [PubMed]
- Sedlis A, Bundy BN, Rotman MZ, Lentz SS, Muderspach LI, Zaino RJ. A randomized trial of pelvic radiation therapy versus no further therapy in selected patients with stage IB carcinoma of the cervix after radical hysterectomy and pelvic lymphadenectomy: A Gynecologic Oncology Group Study. Gynecol Oncol 1999;73:177-83. [Crossref] [PubMed]
- Lee SI, Atri M. 2018 FIGO Staging System for Uterine Cervical Cancer: Enter Cross-sectional Imaging. Radiology 2019;292:15-24. [Crossref] [PubMed]
- Manganaro L, Lakhman Y, Bharwani N, Gui B, Gigli S, Vinci V, Rizzo S, Kido A, Cunha TM, Sala E, Rockall A, Forstner R, Nougaret S. Staging, recurrence and follow-up of uterine cervical cancer using MRI: Updated Guidelines of the European Society of Urogenital Radiology after revised FIGO staging 2018. Eur Radiol 2021;31:7802-16. [Crossref] [PubMed]
- Sakala MD, Shampain KL, Wasnik AP. Advances in MR Imaging of the Female Pelvis. Magn Reson Imaging Clin N Am 2020;28:415-31. [Crossref] [PubMed]
- Lee J, Kim CK, Gu KW, Park W. Value of blood oxygenation level-dependent MRI for predicting clinical outcomes in uterine cervical cancer treated with concurrent chemoradiotherapy. Eur Radiol 2019;29:6256-65. [Crossref] [PubMed]
- Li B, Sun H, Zhang S, Wang X, Guo Q. Amide proton transfer imaging to evaluate the grading of squamous cell carcinoma of the cervix: A comparative study using (18) F FDG PET. J Magn Reson Imaging 2019;50:261-8. [Crossref] [PubMed]
- Le Bihan D, Breton E, Lallemand D, Aubin ML, Vignaud J, Laval-Jeantet M. Separation of diffusion and perfusion in intravoxel incoherent motion MR imaging. Radiology 1988;168:497-505. [Crossref] [PubMed]
- Winfield JM, Orton MR, Collins DJ, Ind TE, Attygalle A, Hazell S, Morgan VA, deSouza NM. Separation of type and grade in cervical tumours using non-mono-exponential models of diffusion-weighted MRI. Eur Radiol 2017;27:627-36. [Crossref] [PubMed]
- Song J, Lu Y, Wang X, Peng W, Lin W, Hou Z, Yan Z. A comparative study of four diffusion-weighted imaging models in the diagnosis of cervical cancer. Acta Radiol 2022;63:536-44. [Crossref] [PubMed]
- Zhou J, Payen JF, Wilson DA, Traystman RJ, van Zijl PC. Using the amide proton signals of intracellular proteins and peptides to detect pH effects in MRI. Nat Med 2003;9:1085-90. [Crossref] [PubMed]
- Su C, Li S, Chen X, Liu C, Shaghaghi M, Jiang J, Zhang S, Qin Y, Cai K. Predicting cancer malignancy and proliferation in glioma patients: intra-subject inter-metabolite correlation analyses using MRI and MRSI contrast scans. Quant Imaging Med Surg 2021;11:2721-32. [Crossref] [PubMed]
- Jia G, Abaza R, Williams JD, Zynger DL, Zhou J, Shah ZK, Patel M, Sammet S, Wei L, Bahnson RR, Knopp MV. Amide proton transfer MR imaging of prostate cancer: a preliminary study. J Magn Reson Imaging 2011;33:647-54. [Crossref] [PubMed]
- Fu F, Meng N, Huang Z, Sun J, Wang X, Shang J, Fang T, Feng P, Wang K, Han D, Wang M. Identification of histological features of endometrioid adenocarcinoma based on amide proton transfer-weighted imaging and multimodel diffusion-weighted imaging. Quant Imaging Med Surg 2022;12:1311-23. [Crossref] [PubMed]
- Meng N, Wang XJ, Sun J, Huang L, Wang Z, Wang KY, Wang J, Han DM, Wang MY. Comparative Study of Amide Proton Transfer-Weighted Imaging and Intravoxel Incoherent Motion Imaging in Breast Cancer Diagnosis and Evaluation. J Magn Reson Imaging 2020;52:1175-86. [Crossref] [PubMed]
- Wang HJ, Cai Q, Huang YP, Li MQ, Wen ZH, Lin YY, Ouyang LY, Qian L, Guo Y. Amide Proton Transfer-weighted MRI in Predicting Histologic Grade of Bladder Cancer. Radiology 2022;305:127-34. [Crossref] [PubMed]
- Meng N, Wang X, Sun J, Han D, Ma X, Wang K, Wang M. Application of the amide proton transfer-weighted imaging and diffusion kurtosis imaging in the study of cervical cancer. Eur Radiol 2020;30:5758-67. [Crossref] [PubMed]
- Hou M, Song K, Ren J, Wang K, Guo J, Niu Y, Li Z, Han D. Comparative analysis of the value of amide proton transfer-weighted imaging and diffusion kurtosis imaging in evaluating the histological grade of cervical squamous carcinoma. BMC Cancer 2022;22:87. [Crossref] [PubMed]
- Zhu H, Jones CK, van Zijl PC, Barker PB, Zhou J. Fast 3D chemical exchange saturation transfer (CEST) imaging of the human brain. Magn Reson Med 2010;64:638-44. [Crossref] [PubMed]
- Ghosh A, Yekeler E, Dalal D, Holroyd A, States L. Whole-tumour apparent diffusion coefficient (ADC) histogram analysis to identify MYCN-amplification in neuroblastomas: preliminary results. Eur Radiol 2022;32:8453-62. [Crossref] [PubMed]
- Zhuo Z, Qu L, Zhang P, Duan Y, Cheng D, Xu X, Sun T, Ding J, Xie C, Liu X, Haller S, Barkhof F, Zhang L, Liu Y. Prediction of H3K27M-mutant brainstem glioma by amide proton transfer-weighted imaging and its derived radiomics. Eur J Nucl Med Mol Imaging 2021;48:4426-36. [Crossref] [PubMed]
- Chen W, Li L, Yan Z, Hu S, Feng J, Liu G, Liu B, Liu X. Three-dimension amide proton transfer MRI of rectal adenocarcinoma: correlation with pathologic prognostic factors and comparison with diffusion kurtosis imaging. Eur Radiol 2021;31:3286-96. [Crossref] [PubMed]
- Choi YS, Ahn SS, Lee SK, Chang JH, Kang SG, Kim SH, Zhou J. Amide proton transfer imaging to discriminate between low- and high-grade gliomas: added value to apparent diffusion coefficient and relative cerebral blood volume. Eur Radiol 2017;27:3181-9. [Crossref] [PubMed]
- Lin Y, Luo X, Yu L, Zhang Y, Zhou J, Jiang Y, Zhang C, Zhang J, Li C, Chen M. Amide proton transfer-weighted MRI for predicting histological grade of hepatocellular carcinoma: comparison with diffusion-weighted imaging. Quant Imaging Med Surg 2019;9:1641-51. [Crossref] [PubMed]
- Togao O, Yoshiura T, Keupp J, Hiwatashi A, Yamashita K, Kikuchi K, Suzuki Y, Suzuki SO, Iwaki T, Hata N, Mizoguchi M, Yoshimoto K, Sagiyama K, Takahashi M, Honda H. Amide proton transfer imaging of adult diffuse gliomas: correlation with histopathological grades. Neuro Oncol 2014;16:441-8. [Crossref] [PubMed]
- Togao O, Hiwatashi A, Yamashita K, Kikuchi K, Keupp J, Yoshimoto K, Kuga D, Yoneyama M, Suzuki SO, Iwaki T, Takahashi M, Iihara K, Honda H. Grading diffuse gliomas without intense contrast enhancement by amide proton transfer MR imaging: comparisons with diffusion- and perfusion-weighted imaging. Eur Radiol 2017;27:578-88. [Crossref] [PubMed]
- Wang M, Perucho JAU, Chan Q, Sun J, Ip P, Tse KY, Lee EYP. Diffusion Kurtosis Imaging in the Assessment of Cervical Carcinoma. Acad Radiol 2020;27:e94-101. [Crossref] [PubMed]
- Shi B, Dong JN, Zhang LX, Li CP, Gao F, Li NY, Wang CB, Fang X, Wang PP. A Combination Analysis of IVIM-DWI Biomarkers and T2WI-Based Texture Features for Tumor Differentiation Grade of Cervical Squamous Cell Carcinoma. Contrast Media Mol Imaging 2022;2022:2837905. [Crossref] [PubMed]
- Andreou A, Koh DM, Collins DJ, Blackledge M, Wallace T, Leach MO, Orton MR. Measurement reproducibility of perfusion fraction and pseudodiffusion coefficient derived by intravoxel incoherent motion diffusion-weighted MR imaging in normal liver and metastases. Eur Radiol 2013;23:428-34. [Crossref] [PubMed]
- Rosenkrantz AB, Padhani AR, Chenevert TL, Koh DM, De Keyzer F, Taouli B, Le Bihan D. Body diffusion kurtosis imaging: Basic principles, applications, and considerations for clinical practice. J Magn Reson Imaging 2015;42:1190-202. [Crossref] [PubMed]
- Perucho JAU, Chang HCC, Vardhanabhuti V, Wang M, Becker AS, Wurnig MC, Lee EYP. B-Value Optimization in the Estimation of Intravoxel Incoherent Motion Parameters in Patients with Cervical Cancer. Korean J Radiol 2020;21:218-27. [Crossref] [PubMed]
- Meng N, Fang T, Feng P, Huang Z, Sun J, Wang X, Shang J, Wang K, Han D, Wang M. Amide Proton Transfer-Weighted Imaging and Multiple Models Diffusion-Weighted Imaging Facilitates Preoperative Risk Stratification of Early-Stage Endometrial Carcinoma. J Magn Reson Imaging 2021;54:1200-11. [Crossref] [PubMed]
- Jones CK, Schlosser MJ, van Zijl PC, Pomper MG, Golay X, Zhou J. Amide proton transfer imaging of human brain tumors at 3T. Magn Reson Med 2006;56:585-92. [Crossref] [PubMed]
- Zhou J, Heo HY, Knutsson L, van Zijl PCM, Jiang S. APT-weighted MRI: Techniques, current neuro applications, and challenging issues. J Magn Reson Imaging 2019;50:347-64. [Crossref] [PubMed]
- He YL, Li Y, Lin CY, Qi YF, Wang X, Zhou HL, Yang JJ, Xiang Y, Xue HD, Jin ZY. Three-dimensional turbo-spin-echo amide proton transfer-weighted mri for cervical cancer: A preliminary study. J Magn Reson Imaging 2019;50:1318-25. [Crossref] [PubMed]
- Lee JB, Park JE, Jung SC, Jo Y, Kim D, Kim HS, Choi CG, Kim SJ, Kang DW. Repeatability of amide proton transfer-weighted signals in the brain according to clinical condition and anatomical location. Eur Radiol 2020;30:346-56. [Crossref] [PubMed]
- Zhou J, Zaiss M, Knutsson L, Sun PZ, Ahn SS, Aime S, et al. Review and consensus recommendations on clinical APT-weighted imaging approaches at 3T: Application to brain tumors. Magn Reson Med 2022;88:546-74. [Crossref] [PubMed]
- Cohen PA, Jhingran A, Oaknin A, Denny L. Cervical cancer. Lancet 2019;393:169-82. [Crossref] [PubMed]
- Meyer HJ, Hamerla G, Höhn AK, Surov A. Whole Lesion Histogram Analysis Derived From Morphological MRI Sequences Might be Able to Predict EGFR- and Her2-Expression in Cervical Cancer. Acad Radiol 2019;26:e208-15. [Crossref] [PubMed]
- Fleischmann M, Chatzikonstantinou G, Fokas E, Wichmann J, Christiansen H, Strebhardt K, Rödel C, Tselis N, Rödel F. Molecular Markers to Predict Prognosis and Treatment Response in Uterine Cervical Cancer. Cancers (Basel) 2021;13:5748. [Crossref] [PubMed]