Global trend in research of intracranial aneurysm management with artificial intelligence technology: a bibliometric analysis
Introduction
Intracranial aneurysm (IA) is a prevalent cerebrovascular disease, affecting 3% to 7% of the adult population (1,2) and is associated with a potentially fatal subarachnoid hemorrhage (SAH) leading to high mortality and disability rates (3). Despite advancements in IA management, including diagnosis, rupture risk assessment, treatment option selection, and follow-up observation, it remains heavily dependent on individual clinician experience. The multifaceted challenges associated with aneurysm management cannot be resolved with conventional approaches.
Artificial intelligence (AI) is an algorithmic technique that automates intellectual tasks and has demonstrated favorable performance in various medical fields (4). Recently, AI technology has shown promising results in the management of early neurological diseases and is anticipated to provide clinicians with guidance, resulting in higher accuracy and efficacy at all stages of IA management (5,6).
Bibliometric analysis entails the enumeration and statistical analysis of scientific output, encompassing articles, publications, citations, patents, and more complex indicators, to assess the contributions of authors, journals, institutions, or countries and identify trends in recent research directions (7,8). The application of bibliometric analysis has gained increasing popularity among clinical disease researchers. Zhang et al. (9) conducted a bibliometric analysis of 5,406 articles on IAs published between 2012 and 2021, while Lu et al. (10) evaluated the nature, content, and temporal changes of the 100 most cited articles on unruptured aneurysms. By comprehending the origins and designs of IA articles, we can better anticipate the future state of this field. However, to the best of our knowledge, no up-to-date bibliometric analysis study has been published to date on the application of IA management with AI technology (IAMWAIT).
This study aims to perform a bibliometric analysis of articles retrieved from IAMWAIT and reveal the most influential or prolific countries, institutions, journals, authors, co-cited papers, co-cited references, and potential collaborators. Through keyword analysis and reference cluster analysis, the major research directions and frontiers in this field have been detected.
Methods
From January 1, 1900 to July 20, 2023, we conducted a literature search using the Web of Science Core Collection (WoSCC) database. Two researchers screened the publications separately (Fujunhui Zhang and Mirzat Turhon) and the disputes were resolved by a senior researcher (Ying Zhang) after a collaborative discussion.
The bibliometric parameters of publications were extracted, including title, publishing date, country, author, institution, journal, keywords, and references. WPS Office 2022.11.03 (Kingsoft Office, Beijing, China) was used for the analysis of contribution. Scimago Graphica (Version 1.0.25) was used to generate the collaborative map of countries. VOSviewer (Version 1.6.18) was used for network visualizations while CiteSpace (Version 6.2.4) was used for cluster analysis, dual-map overlay of citations, timeline, and strongest citation bursts of references or keywords. R (Version 4.0.3) was used to generate the thematic map and thematic evolution analysis based on the “Bibliometric” package.
The concept of relative research interest (RRI) can be defined as the ratio of the number of publications pertaining to IAMWAIT in a given year to the total number of publications on IA within the WoSCC database for the same period, as outlined in reference (9). Meanwhile, the metric of average paper citations refers to the quotient of the total number of citations received by a given set of articles and the number of articles included in that set. To account for fluctuations in publication trends over time, the gross domestic product (GDP) of each country was calculated by aggregating data from 2006 to 2022. The H-index, which measures the number of publications that have received citation counts of at least H, was determined for the IAMWAIT field during the period spanning from 2006 to 2022, as described in reference (11).
Results
General data
The comprehensive literature search yielded a total of 504 publications, which underwent a rigorous screening process. Among these, 36 publications were excluded for not meeting the inclusion criteria as articles or reviews, while 191 were considered irrelevant due to the broad scope of the search terms. Ultimately, 277 articles were included in the analysis, as depicted in Figure 1.
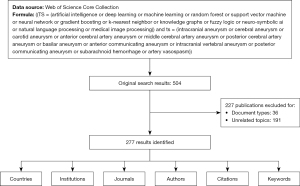
Trends in publications and RRI over time are illustrated in Figure 2. The number of publications showed a notable upward trend, increasing from 7 in the period between 2006 and 2012 to 88 in 2022. By the time of data collection in 2023 (before July 20th), there were 36 publications. Similarly, the RRI witnessed a substantial rise from 0.0008 in the period between 2006 and 2012 to 0.0452 in 2022. The contributions to the IAMWAIT theme emanated from 39 countries, 600 institutions, 1,764 authors, 133 journals, and resulted in 3,197 citations.
Countries
Table 1 displays the distribution of papers, total citation counts, average paper citations, and GDP by country. Presently, China has emerged as the most prolific country, accounting for 118 papers (42.6% of the total) and garnering 825 citations. The United States boasts the highest total citation count, amassing 1,618 citations, and also holds the highest average paper citations, with an average of 19.7 citations per paper. China, the United States, and Australia were the most frequent collaborators in research efforts. International collaborations were notable for both the United States and China, with nine overseas partnerships each (Figure 3A,3B). Notably, both the total number of papers (r=0.8207, P=0.0036) and the total citation count (r=0.9854, P<0.0001) showed positive and significant correlations with the GDP of countries (Figure 3C,3D).
Table 1
Country | Papers | Total citations | Average paper citations (%) | GDP (trillion dollars)* |
---|---|---|---|---|
China | 118 | 825 | 7.0 | 170.9 |
USA | 82 | 1,618 | 19.7 | 305.9 |
Germany | 25 | 264 | 10.6 | 63.9 |
Japan | 21 | 329 | 15.7 | 86.2 |
England | 15 | 94 | 6.3 | 47.3 |
South Korea | 13 | 181 | 13.9 | 23.2 |
Australia | 12 | 113 | 9.4 | 22.1 |
Switzerland | 10 | 70 | 7.0 | 10.4 |
Canada | 7 | 58 | 8.3 | 28.4 |
France | 7 | 28 | 4.0 | 46.4 |
*, GDP was calculated by summing from 2006 to 2022. IAMWAIT, intracranial aneurysm management with artificial intelligence technology; GDP, gross domestic product.
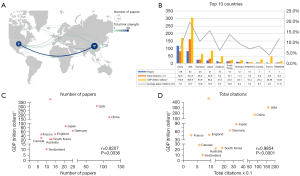
Institutions
Table 2 provides a comprehensive ranking of the top 10 institutions based on their productivity in the IAMWAIT field. Of these institutions, 5 are located in China, 4 in the United States, and 1 in Australia. Capital Medical University emerged as the most productive institution, leading the chart with the highest number of published papers (China, n=17). Following closely were the Chinese Academy of Sciences (China, n=11) and Fudan University (China, n=11). Notably, the University of California System (USA) secured the maximum number of citations, with its publications receiving citations 229 times, and an H-index of 6. It was followed by the State University of New York Suny System (USA) with 200 citations and an H-index of 8, and Harvard University (USA) with 184 citations and an H-index of 7.
Table 2
Rank | Institution | Country | Papers | Citations | Average paper citations | H-index |
---|---|---|---|---|---|---|
1 | Capital Medical University | China | 17 | 123 | 7.24 | 5 |
2 | Chinese Academy of Sciences | China | 11 | 42 | 3.82 | 4 |
3 | Fudan University | China | 11 | 20 | 1.82 | 2 |
4 | State University of New York Suny System | USA | 10 | 200 | 20.00 | 8 |
5 | Macquarie University | Australia | 9 | 108 | 12.00 | 5 |
6 | Shanghai Jiao Tong University | China | 9 | 78 | 8.67 | 3 |
7 | Southern Medical University | China | 9 | 48 | 5.33 | 3 |
8 | The University of California System | USA | 9 | 229 | 25.44 | 6 |
9 | The University of Texas System | USA | 9 | 55 | 6.11 | 5 |
10 | Harvard University | USA | 9 | 184 | 23.00 | 7 |
IAMWAIT, intracranial aneurysm management with artificial intelligence technology.
Journals
Table 3 presents the top 10 journals in the IAMWAIT field based on their publication output. Frontiers in Neurology holds the top position, publishing 19 papers, constituting 6.9% of the total output. The Journal of Interventional Neurosurgery follows with 14 papers (5.1%), and the American Journal of Neuroradiology with 10 papers (3.6%). Among the most cited journals, Stroke stands out with 3,185 citations, followed by the American Journal of Neuroradiology with 269 citations, and European Radiology with 249 citations.
Table 3
Rank | Journal | Papers | Citations | IF | Quartile in category | H-index |
---|---|---|---|---|---|---|
1 | Front Neurol | 19 | 68 | 3.4 | Q2 | 4 |
2 | J Neurointerv Surg | 14 | 121 | 4.8 | Q1 | 5 |
3 | Am J Neruoradiol | 10 | 269 | 3.5 | Q2 | 6 |
4 | Eur Radiol | 10 | 249 | 5.9 | Q1 | 6 |
5 | Int J Comput Assist Radiol Surg | 10 | 68 | 3.0 | Q2 | 4 |
6 | World Neurosurg | 9 | 96 | 2.0 | Q4 | 4 |
7 | Scientific reports | 5 | 74 | 4.6 | Q2 | 3 |
8 | Stroke | 5 | 3,185 | 8.3 | Q1 | 27 |
9 | Acta Neurochir | 4 | 31 | 2.7 | Q2 | 3 |
10 | Comput Methods Programs Biomed | 4 | 14 | 6.1 | Q1 | 2 |
IF, impact factor; IAMWAIT, intracranial aneurysm management with artificial intelligence technology.
The dual-map overlay provides a visual representation of the characteristics of journals within the IAMWAIT field (12). Analysis of citation publications revealed a concentration of works in the neurological discipline, with additional contributions in the fields of medicine, molecular biology, and immunology. Interestingly, the most frequently cited papers were published in journals specializing in psychology, education, society, health, nursing, medicine, molecular biology, and genetics. The majority of cited journals were affiliated with the imaging and neurosurgery fields, with Stroke, American Journal of Neuroradiology, Neurosurgery, Journal of Neurosurgery, Radiology, Lancet, and Journal of Biomechanics having the largest circle size, indicative of their high citation frequency (see Figure 4).
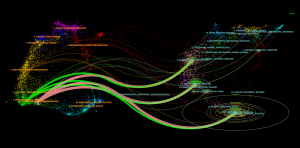
Authors
Table 4 presents the top 10 authors with the highest publication output and the top 10 co-cited authors, with a significant representation from China. The top 10 co-cited authors were identified based on the number of citations accrued within the IAMWAIT field. Duan Chuan-Zhi (n=9 papers), Ou Chubin (n=7 papers), Zhang Xin (n=7 papers), Yang Yunjun (n=7 papers), and Chen Yongchun (n=7 papers) emerged as the most prolific authors.
Table 4
Rank | Author | Country | Institution | Publications |
---|---|---|---|---|
1 | Duan, Chuan-Zhi | China | Southern Medical University | 9 |
2 | Ou, Chubin | China | The First People’s Hospital of Foshan | 7 |
3 | Zhang, Xin | China | Southern Medical University | 7 |
4 | Yang, Yunjun | China | Wenzhou Medical University | 7 |
5 | Chen, Yongchun | China | National Engineering Laboratory of Coal Mine Ecological Environment Protection | 7 |
6 | Lin, Boli | China | Wenzhou Medical University | 6 |
7 | Meng, Hui | USA | State University of New York at Buffalo | 5 |
8 | Li, Youxiang | China | Capital Medical University | 5 |
9 | Snyder Kenneth | USA | State University of New York at Buffalo | 5 |
10 | Zhou, Jiafeng | China | East China Normal University | 5 |
Figure 5 provides a visual representation of the collaborative author network in the IAMWAIT field and the co-cited author network. Notably, Zhang Longjiang, Morgan Michael, and were active during the early stages of the field (i.e., 2020), while Duan Chuan-Zhi, Sun Kaijiang, Feng Xin, and were active during the later stages (i.e., 2022 to 2023).
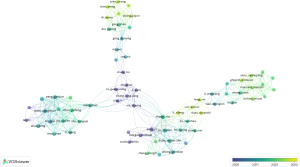
Cited papers and co-cited references
Table 5 presents a comprehensive list of the top 10 most highly cited papers from a total of 277 papers in the IAMWAIT field. These papers were authored by researchers from the USA (n=3), China (n=2), Japan (n=2), Germany (n=1), India (n=1), and Singapore (n=1). Of note, Chilamkurthy et al. (13) published the paper with the highest number of citations (91 times) in the Lancet in 2018, entitled “Deep learning algorithms for detection of critical findings in head CT scans: a retrospective study”. Their study focused on the development of a deep learning model for aiding in the diagnosis of aneurysms on head computed tomography (CT) scans.
Table 5
Rank | Cited papers | Publication type | Country | Citations | IF |
---|---|---|---|---|---|
1 | Chilamkurthy, 2018, Lancet (13) | Article | India | 387 | 168.9 |
2 | Chang, 2018, Am J Neruoradiol (14) | Article | USA | 134 | 3.5 |
3 | Park, 2019, JAMA Netw Open (15) | Article | USA | 107 | 13.8 |
4 | Ye, 2019, Eur Radiol (16) | Article | China | 103 | 5.9 |
5 | Ueda, 2019, Radiology (17) | Article | Japan | 96 | 19.7 |
6 | Nakao, 2018, J Magn Reson Imaging (18) | Article | Japan | 96 | 4.4 |
7 | Ker, 2019, Sensors (Basel) (19) | Article | Singapore | 91 | 3.9 |
8 | Sichtermann, 2019, Am J Neruoradiol (20) | Article | Germany | 69 | 3.5 |
9 | Castro, 2017, Neurology (21) | Article | USA | 58 | 9.9 |
10 | Liu, 2019, Stroke (22) | Article | China | 54 | 8.3 |
IF, impact factor.
The timeline map of the reference clusters identified 9 distinct clusters with modularity Q score and silhouette score values of 0.6158 (>0.3) and 0.8804 (>0.7), respectively. References play a pivotal role in shaping research directions, and cluster analysis of references can effectively highlight the prominent areas of research in published papers. In this study, the clusters pointed to 6 main categories within IAMWAIT, namely, “computer-assisted detection”, “outcome predictors”, “rupture risk”, “three-dimensional reconstruction”, “cerebral vasospasm”, and “biomarkers” (Figure 6A). “Computer-assisted detection” encompassed references focusing on the detection and diagnosis of IAs through AI technology. “Outcome predictors” involved references related to outcome prediction of IAs after treatment, encompassing growth prediction, vasospasm prediction, complications prediction, and others. “Rupture risk” references were centered on the prediction of rupture for unruptured IAs. “Three-dimensional reconstruction” pertained to IA segment and reconstruction references. “Cerebral vasospasm” included keywords related to vasospasm prediction after treatment. Finally, “biomarkers” referred to references in the domain of metabolic fingerprints, gene expression, inflammation, immune microenvironment, and related areas. Notably, references in the “three-dimensional reconstruction” category were the earliest, dating back to 1998. The majority of references were published between 2016 and 2021, particularly in the areas of “computer-assisted detection” and “rupture risk”.
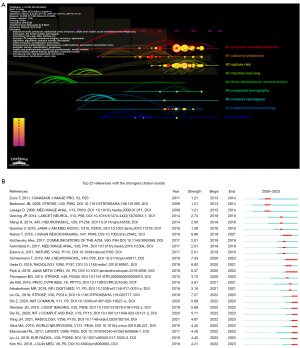
Citations for most of the references occurred between 2013 and 2023 (Figure 6B). Early references with the strongest citation bursts between 2013 and 2014 were Zuva et al. (23), Bederson et al. (24), and Lesage et al. (25), which focused on IA segment and rupture. Conversely, the reference with the highest strength pertained to computer-assisted detection of IAs, published by Nakao et al. (18) in the Journal of Magnetic Resonance Imaging, and experienced a notable surge in citations from 2019 through 2021.
Keywords
The Sankey diagram in Figure 7A illustrates the evolution of keywords over different periods of article publication, divided into five segments: 2006–2017, 2018–2020, 2021, 2022, and 2023. During the period from 2006 to 2017, keywords such as “rupture”, “segmentation”, “subarachnoid hemorrhage”, and “diagnosis” were prominent. From 2018 to 2020, important keywords that emerged included “hemodynamics”, “vasospasm”, and “diagnosis”, among others. In 2021, novel keywords like “symptomatic vasospasm”, “endovascular treatment”, “hemodynamics”, and “growth” gained significance. In 2022, the emergence of “complication”, “subarachnoid hemorrhage”, “vasospasm”, “rupture”, and “segmentation” as key keywords was observed. By 2023, “subarachnoid hemorrhage”, “diagnosis”, and “complication” were the major keywords.
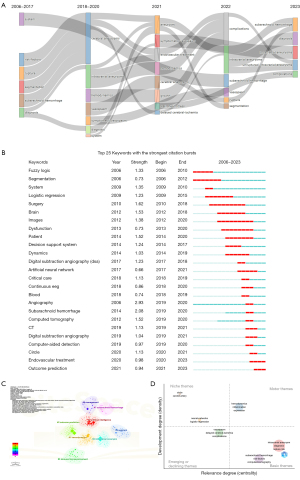
Figure 7B displays the top 25 keywords with the most citations. Notably, “fuzzy logic” and “segment” were among the earliest burst words, dating back to 2006 to 2012. Keywords like “disfunction”, “decision support system”, “dynamics”, and “digital subtraction angiography” emerged during the middle period from 2013 to 2020. More recent burst words from 2019 to 2023 included “subarachnoid hemorrhage”, “computer-aided detection”, and “outcome prediction”, among others.
To provide clarity, the keywords were clustered into eight groups, as shown in Figure 7C, with a modularity Q score and silhouette score of 0.5102 (>0.3) and 0.8104 (>0.7), respectively. The most critical cluster was centered around “artificial intelligence”, followed by clusters focusing on “hemodynamics”, “outcome prediction”, “immune microenvironment”, “segment”, “subarachnoid hemorrhage”, and others. Merged proximal words were excluded for clarity (Table S1), and irrelevant keywords were also excluded (Table S2).
Figure 7D, the thematic map, visually represents the importance and development of themes in IAMWAIT, guiding scientific research themes. Keywords such as “hemodynamics”, “inflammation”, and “expression” were identified as important and well-developed themes. Themes like “diagnosis” and “rupture risk” were important but not as well-developed, while emerging themes in the field included “vasospasm”, “delay cerebral-ischemia”, and “complications”.
Discussion
This study presents a comprehensive bibliometric analysis of the rapidly developing field of IAMWAIT, encompassing 277 papers published until July 20, 2023. Over the years, IAMWAIT has witnessed significant growth, with the number of publications rising from 7 in the period between 2006 and 2012 to 88 in 2022, resulting in a substantial increase in the RRI from 0.11% to 3.59%, indicating a growing interest in this field. China was very active and most involved whereas the United States had the strongest impact in this field. The main research themes, of interest in this field are hemodynamics, rupture risk assessment/prediction, outcome prediction (growth prediction, ischemic or hemorrhage event prediction, vasospasm prediction, etc.), computer-assisted diagnosis, basic research of IA (metabolic fingerprints, gene-expression, inflammation, immune microenvironment, etc.), and IA segment. Among these themes, computer-assisted diagnosis has lasted the longest, and outcome prediction was the latest theme. The most prolific journal in this field is Frontiers in Neurology, while Stroke has the largest number of citations.
Countries
Regarding the geographic distribution of publications and impact in the field of IAMWAIT, China has emerged as a leading contributor, producing the highest number of publications (n=118), with representation from five top prolific institutions and collaborations with nine different countries. Notably, China’s close collaborations with the United States and Australia indicate its significant contributions to the development of AI technology in the medical field, in line with the findings of a previous study (26).
The United States holds the largest world influence in the IAMWAIT field, evident from its highest number of total citations (n=1,618), presence in the top 3 institutions for citations/H index, collaborations with nine countries, and three of the top 10 cited papers, ranked second, third, and ninth, respectively. These findings underscore the strong scientific strength of the United States and its potential for knowledge exchange and collaboration, which aligns with similar observations in bibliometric reports of other fields, such as the treatment of diffuse intrinsic pontine glioma (27) and urological surgery (28).
Furthermore, among the top 10 papers with the highest number of citations, notable contributions come from India, Japan, Singapore, and Germany. Australia, represented by a prolific institution with a high number of citations, also significantly contributed to the development of IAMWIAT. These countries’ presence in the top-cited papers highlights their significant impact in the field and their active participation in driving advancements in AI technology for IA management. The bibliometric analysis reveals a strong positive correlation between the number of papers and GDP, and the number of citations and GDP, suggesting that the economic level of the countries has a significant impact on the application of AI technology. A bibliometric analysis by Wang et al. also found a similar correlation between GDP and moyamoya disease management (29).
Journals
Regarding journal publications, Frontiers in Neurology emerged as the most prolific journal, having published a total of 15 papers (n=19) in the field of IAMWAIT. On the other hand, Stroke demonstrated the highest number of citations, accumulating an impressive total of 3,185 citations. Furthermore, Stroke exhibited the highest H-index and impact factor (IF), indicative of its substantial influence and reputation within the field. The outcomes of this journal analysis offer valuable insights to researchers, guiding them in selecting appropriate journals for disseminating their IAMWAIT-related work. Furthermore, it is pertinent to highlight that among the most highly cited journals in IAMWAIT, several publications dedicated to computing, mathematics, and biomedicine actively contributed to supporting research endeavors related to IAMWAIT. This noteworthy observation underscores the interdisciplinary nature of the field and the active involvement of diverse journals in advancing knowledge and innovations in this area.
Sub-fields of IAMWAIT
Sub-fields of IAMWAIT can be identified through cluster analysis of co-cited references and keywords, revealing the prominent areas of research within this domain. Special attention has been given to highlighting the emerging trends and potential future directions for research within each sub-field. By doing so, we aim to offer a comprehensive outlook for researchers and practitioners, facilitating their endeavors to explore new avenues of investigation and innovation. The primary fields of AI technology applied in IAMWAIT encompass rupture risk assessment/prediction, computer-assisted diagnosis, outcome prediction, hemodynamics, and laboratory research of IAs.
The accurate differentiation of the rupture risk of IAs holds paramount importance due to its potential impact on treatment optimization and improved patient outcomes (5). Over time, the parameters used in rupture risk prediction studies have expanded. Initially, clinical characteristics, aneurysm size, and IA location were utilized to develop AI models (30). Subsequently, studies incorporated morphological parameters such as aspect ratio (AR) and size ratio (SR). For instance, Zhu et al. (6) devised an AI model based on clinical and morphological features to predict rupture risk, surpassing the performance of traditional logistic regression models and the PHASES score. More recently, parameters of hemodynamics and radiomics have been integrated into AI-powered rupture risk prediction models (22,31). In the future, the inclusion of newly proposed parameters is anticipated to further enhance the AI models, providing novel perspectives and improving predictive accuracy. For example, Yang et al. (32) introduced a novel morphological index, the mass moment of inertia, which quantifies the shape irregularity of unruptured IAs and addresses the limitations of previous subjective determinations.
In the context of computer-assisted diagnosis, the application of AI algorithms holds significant promise in reducing radiologists’ reading time and improving diagnostic performance within clinical settings (33). Particularly, AI has shown remarkable potential in automating the segmentation of intracranial arteries and detecting IAs. This theme has garnered substantial attention in the field of IAMWAIT, with half of the top 10 most cited papers focusing on this subject (13,15,17-20). Leveraging extensive medical imaging data, AI algorithms have demonstrated the capacity to enhance the accuracy and efficiency of aneurysm detection and segmentation, providing valuable diagnostic support to medical professionals. Notably, convolutional neural networks (CNNs), a type of AI algorithm, have emerged as a revolutionary tool in computer-assisted diagnosis, exhibiting the potential to expedite processes and improve result consistency (34). However, some limitations have been reported, particularly in simulating blood vessels (35). As a consequence, there is an ongoing need for the creation or updating of novel AI algorithms to address these specific shortcomings. Studies such as those conducted by Lee et al. (36) and Jin et al. (37) have ventured into developing new deep-learning algorithms to overcome these challenges. Moreover, there has been a shift in the focus of research, with an increasing emphasis on time-of-flight MR angiography (TOF-MRA) as a primary non-invasive screening method for IAs, in contrast to the previous concentration on digital subtraction angiography (DSA) (38). Additionally, some studies have extended the application of AI to encompass both diagnosis and rupture prediction, exemplified by the work of Hentschke et al. (39). Furthermore, recent trends in IAMWAIT research indicate greater availability of large annotated datasets, a heightened emphasis on data safety and ethical considerations, and a growing interest in integrating aneurysm detection and segmentation algorithms into clinical workflow systems.
Outcome prediction represents a novel and significant theme in the field of IAMWAIT, playing a crucial role in clinical decision-making. Within outcome prediction, the sub-fields of complication (ischemic or hemorrhage event) prediction, vasospasm prediction after treatment, and growth prediction of unruptured IAs have emerged as areas of particular interest in this study. Notable contributions include Tanioka et al.’s creation of an AI model for predicting delayed cerebral ischemia (40), Kim et al.’s development of an AI-explainable predictive model for vasospasm prediction (41), and Bizjak et al.’s model to predict the growth of untreated IAs based on baseline aneurysm morphology (42). Additionally, some potential sub-fields, such as recurrence prediction for IAs after treatment (43) and effectiveness prediction after endovascular treatment (44), remain underexplored and warrant further investigation in the future.
Hemodynamic simulation, facilitated by AI models of blood flow, proves to be a valuable tool in the management of IAs. Integrating hemodynamic parameters into rupture predictive models, as demonstrated by Yang et al., has shown promise in improving the predictive accuracy of these models (45). Hemodynamic analysis powered by AI has been applied to outcome prediction, rupture prediction, and growth prediction (46-48). Further explorations in this field hold potential, including the use of 4D phase-contrast magnetic resonance imaging (4D pcMRI) as an imaging acquisition method (49) and guiding stent placement during procedures (50). Notably, IA segmentation methods are a necessary step in creating computational fluid dynamics (CFD) models for hemodynamic analysis. Presently, standard procedures for IA segmentation rely on manual segmentation, which may introduce errors and time constraints. The development of AI-powered automated segmentation holds promise as a future direction (51).
Laboratory research of IA, encompassing investigations into metabolic fingerprints, gene expression, inflammation, immune microenvironment, and related aspects, holds promise in providing valuable insights into the inflammatory response associated with IA. Such research can play a pivotal role in advancing diagnosis, outcome prediction, and rupture risk assessment, among other crucial aspects (52-54). By leveraging AI algorithms, laboratory research endeavors can experience significant time savings and increased efficiency, thus accelerating the pace of discoveries in this domain. However, it is pertinent to note that the incorporation of gene expression, metabolic markers, and similar factors into routine clinical examinations has faced criticism, possibly limiting their current instructiveness for IA management in clinical settings. Thus, the endeavor to bridge the gap between research findings and real-world clinical applications becomes a vital direction for future investigations.
This study boasts several noteworthy strengths. Firstly, it is the first bibliometric analysis conducted in the field of IAMWAIT, which has recently gained significant attention and shows great potential. Secondly, to achieve comprehensive and high-quality visualization results, we utilized multiple bibliometric analysis software. Despite these strengths, the study does present certain limitations that warrant acknowledgment. Foremost among them is the utilization of the WoSCC dataset, which, while being the most comprehensive source compatible with VOSviewer and CiteSpace, may not entirely represent the entirety of IAMWAIT. As a result, some literature within the field might not have been fully captured in this analysis. Moreover, the prevalence of certain keywords or co-cited references may have led to potential overrepresentation, potentially resulting in limited coverage of all areas within the diverse domain of IAMWAIT.
Conclusions
The field of IAMWAIT has undergone rapid and significant development in recent years, with AI emerging as a powerful tool capable of addressing various critical aspects, including rupture risk assessment/prediction, computer-assisted diagnosis, outcome prediction, hemodynamics, and laboratory research related to IAs. Looking ahead, there is a pressing need to further explore the potential applications of AI algorithms in additional sub-fields within IAMWAIT, fostering a more comprehensive and versatile approach. Moreover, it is imperative to optimize AI algorithms to align them better with real-world clinical settings, facilitating their seamless integration and practical utility in the management of IA patients. By actively pursuing these research directions, the field can unlock the full potential of AI in IAMWAIT, ushering in a new era of enhanced patient care and clinical outcomes.
Acknowledgments
Funding: This work was supported by
Footnote
Conflicts of Interest: All authors have completed the ICMJE uniform disclosure form (available at https://qims.amegroups.com/article/view/10.21037/qims-23-793/coif). The authors have no conflicts of interest to declare.
Ethical Statement: The authors are accountable for all aspects of the work in ensuring that questions related to the accuracy or integrity of any part of the work are appropriately investigated and resolved.
Open Access Statement: This is an Open Access article distributed in accordance with the Creative Commons Attribution-NonCommercial-NoDerivs 4.0 International License (CC BY-NC-ND 4.0), which permits the non-commercial replication and distribution of the article with the strict proviso that no changes or edits are made and the original work is properly cited (including links to both the formal publication through the relevant DOI and the license). See: https://creativecommons.org/licenses/by-nc-nd/4.0/.
References
- Vlak MH, Algra A, Brandenburg R, Rinkel GJ. Prevalence of unruptured intracranial aneurysms, with emphasis on sex, age, comorbidity, country, and time period: a systematic review and meta-analysis. Lancet Neurol 2011;10:626-36. [Crossref] [PubMed]
- Li MH, Chen SW, Li YD, Chen YC, Cheng YS, Hu DJ, et al. Prevalence of unruptured cerebral aneurysms in Chinese adults aged 35 to 75 years: a cross-sectional study. Ann Intern Med 2013;159:514-21. [Crossref] [PubMed]
- Claassen J, Park S. Spontaneous subarachnoid haemorrhage. Lancet 2022;400:846-62. [Crossref] [PubMed]
- Choi RY, Coyner AS, Kalpathy-Cramer J, Chiang MF, Campbell JP. Introduction to Machine Learning, Neural Networks, and Deep Learning. Transl Vis Sci Technol 2020;9:14. [Crossref] [PubMed]
- Shi Z, Hu B, Schoepf UJ, Savage RH, Dargis DM, Pan CW, Li XL, Ni QQ, Lu GM, Zhang LJ. Artificial Intelligence in the Management of Intracranial Aneurysms: Current Status and Future Perspectives. AJNR Am J Neuroradiol 2020;41:373-9. [Crossref] [PubMed]
- Zhu W, Li W, Tian Z, Zhang Y, Wang K, Zhang Y, Liu J, Yang X. Stability Assessment of Intracranial Aneurysms Using Machine Learning Based on Clinical and Morphological Features. Transl Stroke Res 2020;11:1287-95. [Crossref] [PubMed]
- Kiraz M, Demir E, Özdemir Ö. An international bibliometric study of scientific articles on intracranial aneurysms. Neuroradiol J 2021;34:482-93. [Crossref] [PubMed]
- Wilson M, Sampson M, Barrowman N, Doja A. Bibliometric Analysis of Neurology Articles Published in General Medicine Journals. JAMA Netw Open 2021;4:e215840. [Crossref] [PubMed]
- Zhang Q, Weng L, Li J. The evolution of intracranial aneurysm research from 2012 to 2021: Global productivity and publication trends. Front Neurol 2022;13:953285. [Crossref] [PubMed]
- Lu VM, Chen SH, Young CC, Starke RM. Nature, content and shifts over time of the most impactful unruptured intracranial aneurysms articles: a bibliometric analysis. J Neurointerv Surg 2021;13:177-81. [Crossref] [PubMed]
- Rad AE, Brinjikji W, Cloft HJ, Kallmes DF. The H-index in academic radiology. Acad Radiol 2010;17:817-21. [Crossref] [PubMed]
- Chen C, Leydesdorff L. Patterns of connections and movements in dual‐map overlays: A new method of publication portfolio analysis. J Assoc Inf Sci Technol 2014;65:334-51.
- Chilamkurthy S, Ghosh R, Tanamala S, Biviji M, Campeau NG, Venugopal VK, Mahajan V, Rao P, Warier P. Deep learning algorithms for detection of critical findings in head CT scans: a retrospective study. Lancet 2018;392:2388-96. [Crossref] [PubMed]
- Chang PD, Kuoy E, Grinband J, Weinberg BD, Thompson M, Homo R, Chen J, Abcede H, Shafie M, Sugrue L, Filippi CG, Su MY, Yu W, Hess C, Chow D. Hybrid 3D/2D Convolutional Neural Network for Hemorrhage Evaluation on Head CT. AJNR Am J Neuroradiol 2018;39:1609-16. [Crossref] [PubMed]
- Park A, Chute C, Rajpurkar P, Lou J, Ball RL, Shpanskaya K, et al. Deep Learning-Assisted Diagnosis of Cerebral Aneurysms Using the HeadXNet Model. JAMA Netw Open 2019;2:e195600. [Crossref] [PubMed]
- Ye H, Gao F, Yin Y, Guo D, Zhao P, Lu Y, Wang X, Bai J, Cao K, Song Q, Zhang H, Chen W, Guo X, Xia J. Precise diagnosis of intracranial hemorrhage and subtypes using a three-dimensional joint convolutional and recurrent neural network. Eur Radiol 2019;29:6191-201. [Crossref] [PubMed]
- Ueda D, Yamamoto A, Nishimori M, Shimono T, Doishita S, Shimazaki A, Katayama Y, Fukumoto S, Choppin A, Shimahara Y, Miki Y. Deep Learning for MR Angiography: Automated Detection of Cerebral Aneurysms. Radiology 2019;290:187-94. [Crossref] [PubMed]
- Nakao T, Hanaoka S, Nomura Y, Sato I, Nemoto M, Miki S, Maeda E, Yoshikawa T, Hayashi N, Abe O. Deep neural network-based computer-assisted detection of cerebral aneurysms in MR angiography. J Magn Reson Imaging 2018;47:948-53. [Crossref] [PubMed]
- Ker J, Singh SP, Bai Y, Rao J, Lim T, Wang L. Image Thresholding Improves 3-Dimensional Convolutional Neural Network Diagnosis of Different Acute Brain Hemorrhages on Computed Tomography Scans. Sensors (Basel) 2019;19:2167. [Crossref] [PubMed]
- Sichtermann T, Faron A, Sijben R, Teichert N, Freiherr J, Wiesmann M. Deep Learning-Based Detection of Intracranial Aneurysms in 3D TOF-MRA. AJNR Am J Neuroradiol 2019;40:25-32. [Crossref] [PubMed]
- Castro VM, Dligach D, Finan S, Yu S, Can A, Abd-El-Barr M, Gainer V, Shadick NA, Murphy S, Cai T, Savova G, Weiss ST, Du R. Large-scale identification of patients with cerebral aneurysms using natural language processing. Neurology 2017;88:164-8. [Crossref] [PubMed]
- Liu Q, Jiang P, Jiang Y, Ge H, Li S, Jin H, Li Y. Prediction of Aneurysm Stability Using a Machine Learning Model Based on PyRadiomics-Derived Morphological Features. Stroke 2019;50:2314-21. [Crossref] [PubMed]
- Zuva T, Olugbara OO, Ojo SO, Ngwira S. Image segmentation, available techniques, developments and open issues. Canadian Journal on Image Processing and Computer Vision 2011;2:20-9.
- Bederson JB, Connolly ES Jr, Batjer HH, Dacey RG, Dion JE, Diringer MN, Duldner JE Jr, Harbaugh RE, Patel AB, Rosenwasser RHAmerican Heart Association. Guidelines for the management of aneurysmal subarachnoid hemorrhage: a statement for healthcare professionals from a special writing group of the Stroke Council, American Heart Association. Stroke 2009;40:994-1025. [Crossref] [PubMed]
- Lesage D, Angelini ED, Bloch I, Funka-Lea G. A review of 3D vessel lumen segmentation techniques: models, features and extraction schemes. Med Image Anal 2009;13:819-45. [Crossref] [PubMed]
- He J, Baxter SL, Xu J, Xu J, Zhou X, Zhang K. The practical implementation of artificial intelligence technologies in medicine. Nat Med 2019;25:30-6. [Crossref] [PubMed]
- Lu VM, Power EA, Kerezoudis P, Daniels DJ. The 100 most-cited articles about diffuse intrinsic pontine glioma: a bibliometric analysis. Childs Nerv Syst 2019;35:2339-46. [Crossref] [PubMed]
- He L, Fang H, Wang X, Wang Y, Ge H, Li C, Chen C, Wan Y, He H. The 100 most-cited articles in urological surgery: A bibliometric analysis. Int J Surg 2020;75:74-9. [Crossref] [PubMed]
- Wang C, Kong D, Song H, Liu J, Qi M, Li L. Characterization of Global Research Trends and Prospects on Moyamoya Disease: Bibliometric Analysis. World Neurosurg 2023;173:e329-40. [Crossref] [PubMed]
- Kim HC, Rhim JK, Ahn JH, Park JJ, Moon JU, Hong EP, Kim MR, Kim SG, Lee SH, Jeong JH, Choi SW, Jeon JP. Machine Learning Application for Rupture Risk Assessment in Small-Sized Intracranial Aneurysm. J Clin Med 2019;8:683. [Crossref] [PubMed]
- Chen G, Lu M, Shi Z, Xia S, Ren Y, Liu Z, Liu X, Li Z, Mao L, Li XL, Zhang B, Zhang LJ, Lu GM. Development and validation of machine learning prediction model based on computed tomography angiography-derived hemodynamics for rupture status of intracranial aneurysms: a Chinese multicenter study. Eur Radiol 2020;30:5170-82. [Crossref] [PubMed]
- Miki S, Hayashi N, Masutani Y, Nomura Y, Yoshikawa T, Hanaoka S, Nemoto M, Ohtomo K. Computer-Assisted Detection of Cerebral Aneurysms in MR Angiography in a Routine Image-Reading Environment: Effects on Diagnosis by Radiologists. AJNR Am J Neuroradiol 2016;37:1038-43. [Crossref] [PubMed]
- Yang H, Cho KC, Kim JJ, Kim YB, Oh JH. New morphological parameter for intracranial aneurysms and rupture risk prediction based on artificial neural networks. J Neurointerv Surg 2023;15:e209-15. [Crossref] [PubMed]
- Podgorsak AR, Rava RA, Shiraz Bhurwani MM, Chandra AR, Davies JM, Siddiqui AH, Ionita CN. Automatic radiomic feature extraction using deep learning for angiographic parametric imaging of intracranial aneurysms. J Neurointerv Surg 2020;12:417-21. [Crossref] [PubMed]
- Chen G, Wei X, Lei H, Liqin Y, Yuxin L, Yakang D, Daoying G. Automated computer-assisted detection system for cerebral aneurysms in time-of-flight magnetic resonance angiography using fully convolutional network. Biomed Eng Online 2020;19:38. [Crossref] [PubMed]
- Lee JY, Kim JS, Kim TY, Kim YS. Detection and classification of intracranial haemorrhage on CT images using a novel deep-learning algorithm. Sci Rep 2020;10:20546. [Crossref] [PubMed]
- Jin H, Geng J, Yin Y, Hu M, Yang G, Xiang S, Zhai X, Ji Z, Fan X, Hu P, He C, Qin L, Zhang H. Fully automated intracranial aneurysm detection and segmentation from digital subtraction angiography series using an end-to-end spatiotemporal deep neural network. J Neurointerv Surg 2020;12:1023-7. [Crossref] [PubMed]
- Chen G, Yifang B, Jiajun Z, Dongdong W, Zhiyong Z, Ruoyu D, Bin D, Sirong P, Daoying G, Meng C, Yakang D, Yuxin L. Automated unruptured cerebral aneurysms detection in TOF MR angiography images using dual-channel SE-3D UNet: a multi-center research. Eur Radiol 2023;33:3532-43. [Crossref] [PubMed]
- Hentschke CM, Beuing O, Paukisch H, Scherlach C, Skalej M, Tönnies KD. A system to detect cerebral aneurysms in multimodality angiographic data sets. Med Phys 2014;41:091904. [Crossref] [PubMed]
- Tanioka S, Ishida F, Nakano F, Kawakita F, Kanamaru H, Nakatsuka Y, Nishikawa H, Suzuki H. pSEED group. Machine Learning Analysis of Matricellular Proteins and Clinical Variables for Early Prediction of Delayed Cerebral Ischemia After Aneurysmal Subarachnoid Hemorrhage. Mol Neurobiol 2019;56:7128-35. [Crossref] [PubMed]
- Kim KH, Koo HW, Lee BJ, Sohn MJ. Analysis of risk factors correlated with angiographic vasospasm in patients with aneurysmal subarachnoid hemorrhage using explainable predictive modeling. J Clin Neurosci 2021;91:334-42. [Crossref] [PubMed]
- Bizjak Ž, Pernuš F, Špiclin Ž. Deep Shape Features for Predicting Future Intracranial Aneurysm Growth. Front Physiol 2021;12:644349. [Crossref] [PubMed]
- Lin S, Zou Y, Hu J, Xiang L, Guo L, Lin X, Zou D, Gao X, Liang H, Zou J, Zhao Z, Dai X. Development and assessment of machine learning models for predicting recurrence risk after endovascular treatment in patients with intracranial aneurysms. Neurosurg Rev 2022;45:1521-31. [Crossref] [PubMed]
- Guédon A, Thépenier C, Shotar E, Gabrieli J, Mathon B, Premat K, Lenck S, Degos V, Sourour N, Clarençon F. Predictive score for complete occlusion of intracranial aneurysms treated by flow-diverter stents using machine learning. J Neurointerv Surg 2021;13:341-6. [Crossref] [PubMed]
- Yang H, Cho KC, Kim JJ, Kim JH, Kim YB, Oh JH. Rupture risk prediction of cerebral aneurysms using a novel convolutional neural network-based deep learning model. J Neurointerv Surg 2023;15:200-4. [Crossref] [PubMed]
- Paliwal N, Jaiswal P, Tutino VM, Shallwani H, Davies JM, Siddiqui AH, Rai R, Meng H. Outcome prediction of intracranial aneurysm treatment by flow diverters using machine learning. Neurosurg Focus 2018;45:E7. [Crossref] [PubMed]
- Silva MA, Patel J, Kavouridis V, Gallerani T, Beers A, Chang K, Hoebel KV, Brown J, See AP, Gormley WB, Aziz-Sultan MA, Kalpathy-Cramer J, Arnaout O, Patel NJ. Machine Learning Models can Detect Aneurysm Rupture and Identify Clinical Features Associated with Rupture. World Neurosurg 2019;131:e46-51. [Crossref] [PubMed]
- Hadad S, Mut F, Slawski M, Robertson AM, Cebral JR. Evaluation of predictive models of aneurysm focal growth and bleb development using machine learning techniques. J Neurointerv Surg 2023; Epub ahead of print. [Crossref]
- Karmonik C, Elias SN, Zhang JY, Diaz O, Klucznik RP, Grossman RG, Britz GW. Augmented Reality with Virtual Cerebral Aneurysms: A Feasibility Study. World Neurosurg 2018;119:e617-22. [Crossref] [PubMed]
- Gao H, You W, Lv J, Li Y. Hemodynamic Analysis of Pipeline Embolization Device Stent for Treatment of Giant Intracranial Aneurysm under Unsupervised Learning Algorithm. J Healthc Eng 2022;2022:8509195. [Crossref] [PubMed]
- Rezaeitaleshmahalleh M, Lyu Z, Mu N, Jiang JF. Using convolution neural network-based segmentation for image-based computational fluid dynamics simulations of brain aneurysms: Initial experience in automated model creation. J Mech Med Biol 2023;23: [Crossref]
- Lu T, Liu Z, Guo D, Ma C, Duan L, He Y, Jia R, Guo C, Xing Z, Liu Y, Li T, He Y. Transcriptome-Based Dissection of Intracranial Aneurysms Unveils an "Immuno-Thermal" Microenvironment and Defines a Pathological Feature-Derived Gene Signature for Risk Estimation. Front Immunol 2022;13:878195. [Crossref] [PubMed]
- Poppenberg KE, Tutino VM, Li L, Waqas M, June A, Chaves L, Jiang K, Jarvis JN, Sun Y, Snyder KV, Levy EI, Siddiqui AH, Kolega J, Meng H. Classification models using circulating neutrophil transcripts can detect unruptured intracranial aneurysm. J Transl Med 2020;18:392. [Crossref] [PubMed]
- Xiong Y, Zheng Y, Yan Y, Yao J, Liu H, Shen F, Kong S, Yang S, Yan G, Zhao H, Zhou X, Hu J, Zhou B, Jin T, Shen H, Leng B, Yang P, Liu X. Circulating proteomic panels for risk stratification of intracranial aneurysm and its rupture. EMBO Mol Med 2022;14:e14713. [Crossref] [PubMed]