Brain connectome-based imaging markers for identifiable signature of migraine with and without aura
Introduction
Migraine is a neurological disorder that directly affects over 1 billion people worldwide (1). According to the aura symptoms that are fully reversible, migraine is classified as either migraine with aura (MwA) or migraine without aura (MwoA) (2). Based on the headache attack frequency, migraine can be classified as episodic migraine and chronic migraine (3,4). Fulfillments of the diagnostic criteria for MwoA and/or for MwA are still listed as the priority in the definition of episodic migraine and chronic migraine (5). Around 30% of migraineurs experience a wide spectrum of aura symptoms, including visual, sensory, speech and/or language, motor, brainstem, and retinal symptoms that precede the headache phase (known as the prodromal phase) (2). Patients who experience MwA have higher ischemic stroke and cardiovascular disease risk than those who experience MwoA (6,7). Thus, aura-specific therapy may help to reduce the risk of aura-related vascular events. Either to terminate headache attack or to prevent the next headache attack from happening, migraine aura subtyping based on an understanding of the mechanism of the disease is preferred (8). Cortical spreading depression (CSD) has represented the prominent theory for migraine aura. CSD is characterized by propagating depolarization neurons and glia with a breakdown of normal ionic gradient that translates into neurologic symptoms (9). It is suggested that CSD may activate and sensitize the trigeminovascular pathway which plays a key role in mediating migraine pain attack (5,9). However, it is also argued that CSD can exist in migraine patients but in a silent state, leading to no aura manifestation (10). The subtyping of migraine patients into MwA and MwoA based on aura symptoms may be questionable. Therefore, solid evidence other than aura symptom manifestation for migraine subtyping, as well as for further understanding the heterogeneity of migraine aura and its underlying mechanism, is still needed.
Brain structural connectivity (SC) and functional connectivity (FC) alterations derived from neuroimaging have been reported to provide substantial perceptions of pathophysiology of migraine and may identify the potential MwA discriminative feature (11-18). Brain connectivity variations of migraine patients have been interpreted as associated with CSD and trigeminovascular pathway theories, which are widely recognized as possible pathophysiological mechanisms for migraine (13,19-21). Increased brain FC and hyper-excitability have been detected in MwA relative to MwoA, implying a higher cortical responsiveness in MwA (16). Reduced brain FC has also been found in MwA, which may present a compensatory response to aura dysfunction (12). Brain areas involved in both increased and decreased FC in migraines vary across studies, including the insula and thalamus which play key roles in pain processing and modulation, and default mode network (DMN) and frontoparietal network (FPN) which are responsible for cognitive function, emotion, and decision making, among other roles (13,19,20,22-25). Discrepancies may arise due to heterogeneous clinical presentations, the state of the migraine attack, and the network interactions and compensatory processes. A robust method of FC assessment with reliable validation of the involved areas is still required.
The results of brain white matter (WM) variation representing the brain SC in previous migraine studies have been inconsistent (17,26,27). In a follow-up study spanning 9 years, whole-brain WM difference was not detected between migraine and control cases by using magnetic resonance (MR) dual echo T2-weighted imaging or by fluid-attenuated inversion recovery (FLAIR) imaging, but focal WM alteration was detected in migraine cases (26). Another study reported no significant WM microstructural difference between MwA and MwoA (27). Meanwhile, differences of WM alterations between chronic and episodic migraine have also shown inconsistency across studies (27). However, higher and lower SC involving subcortical and cortical regions associated with pain processing and brain excitability have been identified in chronic and episodic migraine (17). The brain SC alterations in patients MwA still need further study.
Machine learning (ML) approaches characterized with sophisticated algorithms allow the evaluation and validation of the diagnostic value of brain connectivity alteration in migraine. In a resting-state functional MR imaging (rs-fMRI) study using regional functional correlation strength (RFCS) index based on a resting-state approach, Yang et al. applied deep learning models to classify MwA, MwoA, and healthy controls (HC), achieving an accuracy over 98%, but the sample size of MwA was only 15 (28). Another study also identified and validated brain functional connectome-based markers with diagnostic value in a group of 116 patients with MwoA by ML approaches that achieved an accuracy of 92.9%, but no MwA patients were evaluated (29). A study applied a ML to extract WM trajectory derived from diffusion tensor imaging (DTI) as a SC feature to serve as the imaging marker to classify migraine patients who do or do not respond to the treatment (14). In another study, diffusion indexes for WM integrity [fractional anisotropy (FA), mean diffusivity, radial diffusivity, and axial diffusivity] derived from DTI were extracted as image features for migraine diagnosis by using various ML methods, but the sample size of migraine was small and migraine subtype patients were not investigated specifically (30). The contribution of ML methods in discerning MRI biomarkers of significant diagnostic utility has been highlighted (29-31). Therefore, investigation of ML approaches to capture whole-brain SC and FC features showing identifiable signatures of MwA and MwoA in larger sample size remains warranted.
The hypothesis of this study is that whole-brain SC and FC difference could be found between MwA and MwoA by DTI and rs-fMRI. Based on the whole-brain SC and FC alterations, imaging features could be identified using an all-relevant feature selection procedure via ML. These connectivity features might show identifiable power to classify migraine into MwA and MwoA. We present this article in accordance with the TRIPOD reporting checklist (available at https://qims.amegroups.com/article/view/10.21037/qims-23-827/rc).
Methods
Participants and clinical assessment
The study was conducted in accordance with the Declaration of Helsinki (as revised in 2013). The study was approved by the Human Research Ethics Committee of the Nanjing First Hospital, and comprehensive explanations of the procedures were provided to all participants before securing their written informed consent for inclusion in the study. Similar to our previous study, patients were consecutively recruited from the neurological wards in our hospital (from July 2020 to May 2022) (32). Based on the guidelines outlined in the 3rd version of the International Classification of Headache Disorders (ICHD-3) (33), 97 patients were diagnosed with migraine. The diagnostic criteria of MwA were then used to classify migraine patients into MwoA and MwA groups (33). Patients with probable migraine, additional neurological disease other than migraine, severe head injury, brain vascular disease, hydrocephalus, drug abuse, under treatment with potential effects on the central nervous system, clinically diagnosed depression or anxiety, obvious abnormal findings on brain imaging, or other major medical illness were excluded from this study. To mitigate the impact of hormonal fluctuations on cortical excitability, female participants in the mid-cycle phase were included, whereas those who were pregnant or breastfeeding were excluded from the study. To validate the imaging markers potentially derived from the aforementioned migraine patients, we also assembled an external testing cohort from the neurological wards of our affiliated hospital branch. The identical inclusion and exclusion criteria for migraine, MwA, and MwoA, along with the consistent clinical assessments employed to select patients for the initial training dataset, were replicated for enrolling individuals into the external testing sample. According to self-report, all patients with migraine were right-handers. They completed a neuropsychological assessment including the Self-Rating Anxiety Scale (SAS; normal: 20–44; mild to moderate anxiety: 45–59; severe anxiety: 60–74; extreme anxiety: ≥75) (34), Self-Rating Depression Scale (SDS; normal: 20–44; mild depression: 45–59; moderate depression: 60–69; severe depression: ≥70) (35), Montreal Cognitive Assessment (MoCA; normal: ≥26, mild cognitive impairment: 18–25, moderate cognitive impairment: 10–17; severe cognitive impairment <10) (36), Headache Impact Test-6 (HIT-6; little or no impact: ≤49; some impact: 50–55; substantial impact: 56–59; and severe impact: 60–78) (37), and Migraine Disability Assessment Score (MIDAS; little or no disability: 0–5; mild disability: 6–10; moderate disability: 11–20; severe disability: ≥21) (38).
HC participants with no or infrequent tension-type headache (less than one attack per month) who were matched to patients in terms of age, sex, and education were also enrolled in our study. They were recruited from the local population and had no personal or family history of migraine or any other kinds of headache. Those who received any treatment with potential effects on the central nervous system were also excluded.
Kolmogorov-Smirnov tests were applied to check the normality and homogeneity of age, gender, education, disease duration, migraine frequency, HIT-6, MIDAS, and MoCA score between MwA and MwoA patients. Fisher’s test for gender and two-tailed t-tests for continuous variables were then conducted in the comparisons between groups.
MR image acquisition and preprocessing
MR examinations were conducted using two 3.0 Tesla MRI scanners: Ingenia (Philips Medical Systems, Best, Netherlands) for the training sample, uMR 780 (United Imaging Healthcare, Shanghai, China) and for the testing sample. All patients underwent MR scanning while in the headache interictal state and used no preventive treatment. All participants were scanned with almost the same protocol including high-resolution three-dimensional (3D) fast-echo T1-weighted MRI [resolution 1×1×1 mm3, repetition time/echo time (TR/TE) =8.1/3.7 mm, scan time about 4 min 20 s], echo-planar diffusion-weighted imaging (DWI; 60 weighted directions and two b0 images, b=1,000 s/mm2, resolution 2×2×2 mm3, TE/TR =80 ms/8,300 ms, scan time about 7 min 32 s), and rs-fMRI images (echo-planar imaging, resolution 2.75×2.75×4 mm3, TR/TE =2,000 ms/30 ms, 230 volumes, awake and eyes closed, scan time about 7 min 49 s). Based on visual inspections of structural MR images, patients with brain tumor, brain vascular disease, hydrocephalus, or obvious WM hyperintensity were excluded from this study.
The DTI data was preprocessed by using the toolbox of Pipeline for Analyzing braiN Diffusion imAges (PANDA) in MATLAB R2018a (MathWorks, Natick, MA, USA) for fully automated processing of brain diffusion images, which was also used in our previous paper (39,40). The major procedure included removing the skull and cropping the gap, correcting motion and eddy current distortions, and calculating diffusion tensor metrics using the DTIFit command of FSL (University of Oxford, Oxford, UK). The obtained FA images were subjected to linear co-registration within their original space to align with the respective T1-weighted images (T1WI). These T1WI were then non-linearly normalized to conform to the Montreal Neurological Institute (MNI) standard space, utilizing the ICBM152 template and the Advanced Normalization Tools (ANTs). The preprocessing of rs-fMRI data was conducted with the toolbox of GRETNA in MATLAB R2018a (41). Its main steps were as follows: (I) removing the first five time points; (II) slice-timing correction; (III) rigid-body motion correction; (IV) linear co-registration between the T1-weighted structural and functional images; (V) segmentation using the Diffeomorphic Anatomical Registration Through Exponentiated Lie Algebra (DARTEL) algorithm; (VI) normalization; (VII) spatial smoothing using a Gaussian kernel of full width at half maximum (FWHM) of 6 mm; (VIII) temporal detrending; (IX) regressing out nuisance covariates including the 24 motion parameters, WM signal, and cerebrospinal fluid signal; and (X) band-pass temporal filtering (0.01–0.10 Hz).
Region of interest-based MR image comparisons and the connectivity features extraction
Based on the Automated Anatomical Labeling (AAL) atlas, Brainnetome Atlas (42) segmented the standard brain (in the MNI space) into 210 cortical and 36 sub-cortical sub-regions and thus provided a more elaborate framework for whole-brain connectome analysis in humans. The connectivity features were defined as the SC and FC strength calculated from the DTI and rs-fMRI data, respectively. After the segmentation, the SC strength between two arbitrary nodes was computed as the average FA value of all the tracts through the corresponding two nodes after the tractography as described previously (43). Specifically, subject-specific deterministic tractography was executed within the native space of the tracts using the fiber assignment by continuous tracking (FACT) algorithm, accessible through the Diffusion Toolkit (https://www.trackvis.org/dtk/). For each seed, which consisted of eight seeds per voxel, a streamline was initiated. The tracking was halted under the following conditions: when the streamline reached a voxel with an FA value less than 0.2, exceeded the boundaries of the brain mask, or took a trajectory with an angle sharper than 45°. The mean time series of each segmented sub-region were extracted from the preprocessed rs-fMRI data. The pairwise FC strength was then estimated by calculating the Pearson’s correlation coefficients of the time series and transforming the coefficients into z-scores with Fisher’s r-to-z transformation. After the calculation, we extracted a total of 60,270 connectivity features.
Imaging feature selection and random forest classifier construction
Imaging feature selection and random forest classifier construction were simultaneously conducted only in the training group. In assessing the classifiers for distinguishing between MwA and MwoA patients, we conducted 100 repetitions of 10-fold cross-validation. This yielded a cumulative count of 1,000 training-validation iterations. To select connectome-based features with significant discriminative power for MwA identification, we put all connectivity features into an all-relevant feature selection procedure within cross-validation loops using the random forest algorithm (Figure S1) (40,44). The random forest classifiers were constructed using the randomForest package in MATLAB R2018a. Permutation test (permuted 1,000 times) was applied to define the features with significantly higher selection frequency than random values as MwA-related selections. The statistical results were corrected for multiple comparisons using the false discovery rate (FDR) method for the corresponding P value. Utilizing the features that were identified, the final random forest classifier, derived from the training group, was subsequently assessed using the external testing sample.
Results
Demographic characteristics and clinical assessment
The training sample comprised 88 migraine patients, including 56 individuals with MwoA and 32 individuals with MwA. Among the MwA patients, 22 presented with visual or retinal symptoms, eight with sensory symptoms, four with speech and/or language symptoms, and one with motor symptoms (Figure 1). An additional 32 migraine patients (eight MwA) were included as an external testing sample. Both of these groups exhibited a comparable distribution of MwoA and MwA patients (P=0.28 Fisher’s test). A total of 49 individuals were enrolled as HCs. In accordance with our previous study, a total of 169 participants were included in our study (32). The demographic characteristics and clinical assessment of all migraine patients are summarized in Table 1. Analysis demonstrated that data pertaining to age, gender, education, disease duration, migraine frequency, HIT-6, MIDAS, and MoCA score were normally distributed and showed homogeneity after Kolmogorov-Smirnov tests. No notable discrepancies were observed in age, gender, education, disease duration, migraine frequency, HIT-6, MIDAS, and MoCA score between MwA and MwoA patients, after performing Fisher’s test for gender and two-tailed t-tests for continuous variables. The MwA group showed higher headache severity score, SAS, and SDS compared to the MwoA group (all P<0.01). There were no notable distinctions in terms of age, gender, disease duration, migraine frequency, and clinical rating scales between patients in the training and testing samples, except for education and MoCA score (both P<0.01).
Table 1
Parameters | Training sample | Patients | |||||
---|---|---|---|---|---|---|---|
MwA (n=32) | MwoA (n=56) | P value† | Training (n=88) | Testing (n=32) | P value† | ||
Age (years) | 35.4±12.1 | 37.0±8.9 | 0.48 | 36.4±10.1 | 37.2±8.6 | 0.70 | |
Gender (M/F) | 7/25 | 11/45 | 0.79¶ | 18/70 | 7/23 | 0.80¶ | |
Education (years) | 13.4±3.7 | 14.0±3.2 | 0.48 | 13.8±3.4 | 15.5±1.6 | <0.01 | |
Duration (years) | 11.8±8.7 | 14.4±8.8 | 0.19 | 13.4±8.8 | 13.1±8.9 | 0.87 | |
Frequency (days per month) | 4.1±4.0 | 5.4±6.6 | 0.35 | 4.8±5.7 | 5.2±5.6 | 0.74 | |
Headache severity score | 6.0±1.4 | 4.3±1.2 | <0.01 | 5.0±1.5 | 4.6±1.0 | 0.18 | |
HIT-6ǁ | 60.5±7.7 | 59.6±7.3 | 0.61 | 59.9±7.4 | 61.4±6.3 | 0.32 | |
MIDASǁ | 19.2±20.0 | 17.5±21.8 | 0.75 | 18.2±20.9 | 17.2±14.1 | 0.81 | |
MoCAǁ | 25.8±3.1 | 25.7±3.2 | 0.97 | 25.7±3.2 | 29.6±0.8 | <0.01 | |
SASǁ | 52.4±5.2 | 43.6±7.0 | <0.01 | 46.8±9.6 | 49.0±13.5 | 0.33 | |
SDSǁ | 47.2±7.1 | 39.0±6.0 | <0.01 | 42.0±8.7 | 45.6±12.0 | 0.08 |
Values are represented as the mean ± standard deviation, except for the gender distribution. †, unless otherwise indicated, P values were calculated with two-tailed t-tests; ¶, the P values were obtained using Fisher’s tests; ǁ, part of the table content has been adapted from the previous publication of Fu et al. (32) under the Creative Commons Attribution 4.0 International (CC BY) license. MwA, migraine with aura; MwoA, migraine without aura; M, male; F, female; HIT-6, Headache Impact Test-6; MIDAS, Migraine Disability Assessment Score; MoCA, Montreal Cognitive Assessment; SAS, Self-Rating Anxiety Scale; SDS, Self-Rating Depression Scale.
The performance of random forest classifiers
The accuracy and Cohen’s kappa coefficient of the 1,000 random forest classifiers under the 100 runs of 10-fold cross-validation were 82.6%±8.5% and 0.56±0.12, during the all-relevant feature selection procedure. The corresponding sensitivity and specificity were 79.3% [95% confidence interval (CI): 66.2–92.4%] and 85.4% (95% CI: 69.7–100.0%), respectively.
Significantly relevant brain connectivity features
In the construction of the random forest classifiers discriminating between MwA and MwoA patients, eight brain connectivity alterations were identified to exhibit significant relevance to the classification by the permutation test and thus considered the imaging features to discriminate MwA and MwoA patients (Table 2).
Table 2
Feature description | Selection frequency (%)† | MwA | MwoA | HC |
---|---|---|---|---|
MFG-thalamus FC | 92.1 | 0.24±0.26 | 0.41±0.28 | 0.57±0.34 |
PoG-amygdala FC | 90.4 | 0.44±0.17 | 0.19±0.20 | 0.05±0.17 |
STG-insula FC | 89.6 | 0.36±0.17 | 0.15±0.30 | 0.17±0.25 |
PoG-insula SC | 87.2 | 0.15±0.22 | 0.32±0.21 | 0.47±0.14 |
STG-PhG FC | 85.4 | 0.26±0.20 | 0.12±0.27 | 0.10±0.24 |
ITG-LOcC FC | 84.1 | 0.44±0.19 | 0.19±0.34 | 0.17±0.22 |
MFG-PhG FC | 81.5 | 0.23±0.20 | 0.08±0.27 | 0.06±0.24 |
Precuneus-LOcC SC | 78.2 | 0.30±0.14 | 0.36±0.07 | 0.37±0.04 |
Values are represented as the mean ± standard deviation, except for the selection frequency. †, defined as the number of iterations in which the feature was selected divided by the total number of iterations performed. MwA, migraine with aura; MwoA, migraine without aura; HC, healthy controls; MFG, middle frontal gyrus; FC, functional connectivity; PoG, postcentral gyrus; STG, superior temporal gyrus; SC, structural connectivity; PhG, parahippocampal gyrus; ITG, inferior temporal gyrus; LOcC, lateral occipital cortex.
After using the Kolmogorov-Smirnov tests to check the normality, these 8 brain connectivity alterations demonstrated significant distinctions between MwA and MwoA patients, as well as between MwA patients and HC: decreased SC between postcentral gyrus (PoG) and insula, between precuneus and lateral occipital cortex (LOcC); decreased FC between middle frontal gyrus (MFG) and thalamus; increased FC between MFG and parahippocampal gyrus (PhG), between superior temporal gyrus (STG) and insula, between STG and PhG, between inferior temporal gyrus (ITG) and LOcC, as well as between PoG and amygdala (two-tailed t-tests; all P<0.01, after FDR correction; Figure 2). There were no significant differences in the precuneus-LOcC SC, MFG-PhG FC, STG-insula FC, STG-PhG FC, and ITG-LOcC between MwoA and HC (Figure 2).
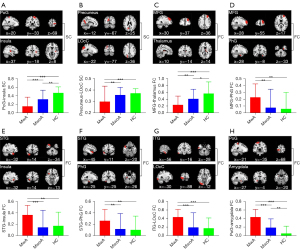
Some of these relevant features were significantly correlated with clinical rating scales in all patients (Pearson’s correlation analysis; all P<0.01, after FDR correction; Figure 3), including the PoG-insula SC with MIDAS (r=−0.35, P=0.003), PoG-insula SC with SAS (r=−0.33, P=0.006), MFG-thalamus FC with headache severity score (r=−0.38, P<0.001) and HIT-6 scores (r=−0.36, P=0.002), STG-insula FC with SAS (r=0.32, P=0.008), and PoG-amygdala FC with SDS (r=0.47, P<0.001).
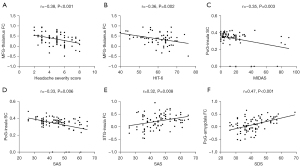
Based on the current sample size of 32 MwA and 56 MwoA patients and 49 HCs, the powers of the significant groupwise differences of the eight relevant features and their significant correlations with the clinical scale scores were all larger than 0.80, using the scripts of sampsizepwr and binofit in MATLAB R2018a.
The predictive ability of the random forest classifier in the testing group
A total of eight potential brain connectivity features of migraine aura were identified after the feature selection procedure in the training group (88 patients), and they showed no significant differences between training and testing groups, as determined by two-tailed t-tests. All P values remained above 0.05 even after correction for FDR. On basis of them, the random forest classifier constructed from the training group achieved an accuracy of 78.1% in the testing group (32 patients, eight MwA) to discriminate MwA patients from the MwoA.
Discussion
This study applied an all-relevant feature selection approach integrated into cross-validation loops to identify whole-brain connectome-based imaging features that contribute to classifying migraine into MwA and MwoA in a data-driven manner. The results indicated that eight brain FC and SC strength alterations have the identifiable power to distinguish MwA. Based on these connectivity imaging features, both the accuracy and the consistency of the random forest classifier constructed with the training sample of 88 migraine patients were close to 80%.
The all-relevant feature selection algorithm we used has been applied in previous studies to select multiple image features as biomarkers to help clinical diagnosis and patient subtyping, and has been shown to obtain satisfying accuracy and consistency (40,44). The brain FC and SC alterations of MwA could serve as connectome-based imaging markers to distinguish MwA with moderate accuracy of 82.6%. Validation of the eight connectome-based imaging markers derived from the 88-patient training sample utilizing an independent cohort of migraine patients scanned on a separate MRI system revealed a predictive accuracy for MwA of 78.1%. Collectively, these whole-brain connectome-based imaging markers are reliable in identifying MwA, and this finding is replicable across an independent sample cohort and another scanner system.
The whole-brain connectome-based features in our results demonstrated a complex whole-brain connectivity pattern of MwA and MwoA. The eight brain connectivity imaging features contain two weakened SC, five increased FC, and one decreased FC. These results were consistent with our previous study showing the co-existence of hypo- and hyper-brain cerebral blood flow in MwA and MwoA (32). The decreased SC changes involved insula, PoG, precuneus, and LOcC, which are important nodes of salience network, nociceptive pathway, DMN, and visual networks, respectively, in MwA patients. According to the results of the current study, a lower structural network involving anatomical compartments including frontotemporal areas and visual areas was detected in episodic and chronic migraine patients (45). The five increased FC-involved nodes are important components of salience network (insula), DMN (MFG), limbic system (amygdala), nociception/anti-nociception in pain processing (PoG), and visual network (LOcC, ITG, STG, and PhG). These areas have also been reported as involved in stronger FC of MwA in previous studies (12,20,24,25). In a magnetoencephalography study, higher FC in MwA than that in MwoA also appeared, which implied higher response and hyperactivity of the brain in MwA (16). This suggested that the pathophysiological hyper-connected brain networks may associate with aura phenomenon (visual, cognitive, motor, and somatosensory processing, etc.). Decreased FC involving the thalamus and MFG has also been reported in different FC studies (12,46). This could be due to the compensatory phenomenon of trigeminovascular pathway modulation in headache attack and post-attack processing.
It is noteworthy that in our results, brain areas involved in increased FC were also involved in decreased FC and SC, for example, MFG was involved in the increased FC of MFG-thalamus and decreased FC of MFG-PhG; insula, PoG, and precuneus were involved in increased FC and decreased SC. Some of our investigated SC or FC alterations-associated areas have also shown FC or SC alterations, respectively, in previous studies (12,14,16,47). A previous study reported a disrupted structural connecting pathway involving the medial prefrontal cortex in MwoA (14). The decreased SC in our results overlaid previous reduced FC in salience and visual networks in MwA (12). In our study, decreased FC involving the thalamus was observed, whereas a previous study reported increased SC involving the thalamus in MwA (47). The heterogeneity of the results mentioned above could be attributed to the difference of the methods. Instead of selecting specific seeds of brain areas to establish and evaluate the connectivity, we applied the whole-brain areas connection analysis. Also, a magnetoencephalography study demonstrated that the brain FC of migraine could be impaired in both low- and high-frequency ranges (16), which may vary and shift along the stimulation of cortex. Thus, the heterogeneity of the cortex activity of our patients during MR scan could also have affected the results. Moreover, the brain metabolism alterations, which were found in the thalamocortical pathway, visual areas, and frontotemporal lobe in migraine patients by previous MR spectroscopy (MRS) and 18F-fluorodeoxyglucose-positron emission tomography (18F-FDG-PET) studies (48,49), may also influence the brain connectivity.
Among the eight varied brain connectivity features with identifiable power to recognize MwA, three recognized MwoA from HC, which may correspond to the postulated pathophysiologic mechanism of migraine initiation and modulation. Compared to HC, MowA showed decreased SC involving the PoG and insula and decreased FC involving the PoG and amygdala, which represent impaired somatosensory area processing, perceived somatic sensation, and dysfunction of cortico-thalamic circuit contributing to trigeminal nociception processing migraine attacks and the migraine mediating trigemino-thalamo-cortical pathway (8,9,19,50). The increased FC involving the PoG and amygdala in our MwoA group was also reported previously, which might attribute to the pain modulation role of the amygdala (9,51). The strength of FC alterations in our MwA group (except FC of MFG-thalamus) was higher than those in the MwoA and HC groups, which may correspond to the theory that CSD may activate the trigeminovascular pathway leading to the more significant alterations in the MwA group (2). Despite the possibility of a CSD silencing phenomena in MwA (10), the 8 imaging markers in our results might provide more information to help to discriminate MwA from MwoA. It has also been demonstrated that the invoked inflammatory molecular reaction leading to glial activation may initiate the CSD process (8). Our connectivity results involved brain areas (visual cortex, insula, and PoG) that play important roles in pain processing and modulation. These brain areas also showed increased uptake of (11C) PBR28 in MwA in previous study (52), which implied possible increased glial activation in the neuro-inflammation process in patients. Both the increased functional activity and increased uptake of (11C) PBR28 results provide possible explanations of glial activation that may initiate the CSD process. The altered permeability of the blood-brain barrier possibly induced by the neuro-inflammation in the amygdala of migraine patients has also been reported (53). Combining our results with neuro-inflammation imaging and vascular permeability studies provides a possible link between CSD and glial activation to further understand the complicated pain modulation mechanism of migraine.
In our results, correlations between the brain connectivity changes and clinical assessments implied the disease progress and may explain symptom manifestation. The absolute correlation coefficient r values were from 0.32 to 0.47, which were significant, but weakly so. These correlations involved key anatomical structures (i.e., MFG, thalamus, insula, STG, amygdala, PoG, shown in Figure 3) in the trigemino-thalamo-cortical pathway for pain processing and modulation, and in the visual network responsible for the visual aura (8). It showed that the lower the FC of MFG-thalamus was, the worse the headache severity and headache impact (HIT-6) the patients would experience. Also, the greater the disruption of the SC of PoG-insula was, the worse the disability (MIDAS) and anxiety (SAS) the migraine patients would experience. Plus, the stronger FC of STG-insula and PoG-amygdala is, the more anxiety (SAS) and depression (SDS) the migraine patients would feel. Similar to our results, functional MRI signal alterations, and relevant molecular and metabolism changes of these areas were also found to be correlated to clinical characteristics in migraine. Alternated thalamus functional activity was detected in a 44-migraine patient cohort and showed a positive correlation with the disease duration (54). A study showed that decreased FC involving PoG was negatively correlated with disease duration in MwoA (55). A negative correlation between insula functional activity and pain-related behavior was also reported in the non-menstrual MwoA (55). An upregulated inflammatory state and increased metabolism of insula in migraine were found previously, which were associated with headache frequency and disease duration, respectively (49,52). The altered directional connectivity of the amygdala was found to be negatively correlated to disease duration in MwoA (51). Meanwhile, lower fractional plasma volume of the amygdala showed a correlation to the intensity of headache attack in migraine (53).
There are several limitations in our study. First, the preprocessing of DTI data did not include the correction of B0 field inhomogeneity using the top-up command of FSL and the denoising based on Marchenko-Pastur principal component analysis. Second, the sample size of MwA in the external testing sample was relatively small. The education and MoCA were significantly different between patients in the training and testing sample, which possibly affected our results. We did not differentiate aura symptoms of our MwA group to specifically classify typical aura migraine, brainstem aura migraine, hemiplegic migraine, or retinal migraine, which may relate to the specific pathophysiological theory of aura. The clinical information of patients, such as whether they had been in a stable clinical situation at least the 3 months before the MRI acquisition or if they experienced headache attacks before or after the MR scan, were not recorded. Although no headache frequency difference between MwA and MwoA was observed in the training sample or between the training sample and external sample, headache frequency should be considered in future research, to eliminate bias in the results. The extraction of only eight features may be insufficient to detect all the possible patterns of change between MwA and MwoA. Although patients with clinically diagnosed depression and anxiety were excluded, the SAS and SDS results of patients with MwA and those with MwoA in the training sample were at the range of normal [20–44] or mild level [45–59] and MwA patients showed higher SDS and SDS results than MwoA patients, which may have led to some bias in the results. More restricted consideration of the effects of depression and anxiety on the results should be considered in the further study. A larger sample size of external testing set of MwA with more specific subtyping is needed in further study to validate our whole-brain connectome-based imaging markers.
Conclusions
Our results showed the coexistence of stronger and weaker brain connectivity in MwA and MwoA comparing to HC. The whole-brain connectivity features in our results may serve as connectome-based imaging markers for MwA identification. The alterations of SC and FC strength provide possible evidence for further understanding the heterogeneity and mechanism of MwA, which may facilitate patient-specific decision-making.
Acknowledgments
Funding: This work was supported by
Footnote
Reporting Checklist: The authors have completed the TRIPOD reporting checklist. Available at https://qims.amegroups.com/article/view/10.21037/qims-23-827/rc
Conflicts of Interest: All authors have completed the ICMJE uniform disclosure form (available at https://qims.amegroups.com/article/view/10.21037/qims-23-827/coif). The authors have no conflicts of interest to declare.
Ethical Statement: The authors are accountable for all aspects of the work in ensuring that questions related to the accuracy or integrity of any part of the work are appropriately investigated and resolved. The study was conducted in accordance with the Declaration of Helsinki (as revised in 2013). The study was approved by the Human Research Ethics Committee of the Nanjing First Hospital and written informed consent was provided by the participants.
Open Access Statement: This is an Open Access article distributed in accordance with the Creative Commons Attribution-NonCommercial-NoDerivs 4.0 International License (CC BY-NC-ND 4.0), which permits the non-commercial replication and distribution of the article with the strict proviso that no changes or edits are made and the original work is properly cited (including links to both the formal publication through the relevant DOI and the license). See: https://creativecommons.org/licenses/by-nc-nd/4.0/.
References
- Ashina M, Katsarava Z, Do TP, Buse DC, Pozo-Rosich P, Özge A, Krymchantowski AV, Lebedeva ER, Ravishankar K, Yu S, Sacco S, Ashina S, Younis S, Steiner TJ, Lipton RB. Migraine: epidemiology and systems of care. Lancet 2021;397:1485-95. [Crossref] [PubMed]
- Migraine Ashina M. N Engl J Med 2020;383:1866-76. [Crossref] [PubMed]
- Torres-Ferrús M, Quintana M, Fernandez-Morales J, Alvarez-Sabin J, Pozo-Rosich P. When does chronic migraine strike? A clinical comparison of migraine according to the headache days suffered per month. Cephalalgia 2017;37:104-13. [Crossref] [PubMed]
- Chalmer MA, Hansen TF, Lebedeva ER, Dodick DW, Lipton RB, Olesen J. Proposed new diagnostic criteria for chronic migraine. Cephalalgia 2020;40:399-406. [Crossref] [PubMed]
- Goadsby PJ, Evers S. International Classification of Headache Disorders - ICHD-4 alpha. Cephalalgia 2020;40:887-8. [Crossref] [PubMed]
- Chen D, Willis-Parker M, Lundberg GP. Migraine headache: Is it only a neurological disorder? Links between migraine and cardiovascular disorders. Trends Cardiovasc Med 2020;30:424-30. [Crossref] [PubMed]
- Yemisci M, Eikermann-Haerter K. Aura and Stroke: relationship and what we have learnt from preclinical models. J Headache Pain 2019;20:63. [Crossref] [PubMed]
- Burstein R, Noseda R, Borsook D. Migraine: multiple processes, complex pathophysiology. J Neurosci 2015;35:6619-29. [Crossref] [PubMed]
- Goadsby PJ, Holland PR, Martins-Oliveira M, Hoffmann J, Schankin C, Akerman S. Pathophysiology of Migraine: A Disorder of Sensory Processing. Physiol Rev 2017;97:553-622. [Crossref] [PubMed]
- Lai J, Dilli E. Migraine Aura: Updates in Pathophysiology and Management. Curr Neurol Neurosci Rep 2020;20:17. [Crossref] [PubMed]
- Nyholt DR, Borsook D, Griffiths LR. Migrainomics - identifying brain and genetic markers of migraine. Nat Rev Neurol 2017;13:725-41. [Crossref] [PubMed]
- Niddam DM, Lai KL, Fuh JL, Chuang CY, Chen WT, Wang SJ. Reduced functional connectivity between salience and visual networks in migraine with aura. Cephalalgia 2016;36:53-66. [Crossref] [PubMed]
- Coppola G, Di Renzo A, Tinelli E, Lepre C, Di Lorenzo C, Di Lorenzo G, Scapeccia M, Parisi V, Serrao M, Colonnese C, Schoenen J, Pierelli F. Thalamo-cortical network activity between migraine attacks: Insights from MRI-based microstructural and functional resting-state network correlation analysis. J Headache Pain 2016;17:100. [Crossref] [PubMed]
- Liu J, Mu J, Chen T, Zhang M, Tian J. White matter tract microstructure of the mPFC-amygdala predicts interindividual differences in placebo response related to treatment in migraine patients. Hum Brain Mapp 2019;40:284-92. [Crossref] [PubMed]
- Park S, Lee DA, Lee HJ, Shin KJ, Park KM. Brain networks in migraine with and without aura: An exploratory arterial spin labeling MRI study. Acta Neurol Scand 2022;145:208-14. [Crossref] [PubMed]
- Wu D, Zhou Y, Xiang J, Tang L, Liu H, Huang S, Wu T, Chen Q, Wang X. Multi-frequency analysis of brain connectivity networks in migraineurs: a magnetoencephalography study. J Headache Pain 2016;17:38. [Crossref] [PubMed]
- Planchuelo-Gómez Á, García-Azorín D, Guerrero ÁL, Aja-Fernández S, Rodríguez M, de Luis-García R. Structural connectivity alterations in chronic and episodic migraine: A diffusion magnetic resonance imaging connectomics study. Cephalalgia 2020;40:367-83. [Crossref] [PubMed]
- Mu J, Chen T, Quan S, Wang C, Zhao L, Liu J. Neuroimaging features of whole-brain functional connectivity predict attack frequency of migraine. Hum Brain Mapp 2020;41:984-93. [Crossref] [PubMed]
- Lim M, Jassar H, Kim DJ, Nascimento TD, DaSilva AF. Differential alteration of fMRI signal variability in the ascending trigeminal somatosensory and pain modulatory pathways in migraine. J Headache Pain 2021;22:4. [Crossref] [PubMed]
- Silvestro M, Tessitore A, Di Nardo F, Scotto di Clemente F, Trojsi F, Cirillo M, Esposito F, Tedeschi G, Russo A. Functional connectivity changes in complex migraine aura: beyond the visual network. Eur J Neurol 2022;29:295-304. [Crossref] [PubMed]
- Coppola G, Parisi V, Di Renzo A, Pierelli F. Cortical pain processing in migraine. J Neural Transm (Vienna) 2020;127:551-66. [Crossref] [PubMed]
- Lee MJ, Park BY, Cho S, Park H, Kim ST, Chung CS. Dynamic functional connectivity of the migraine brain: a resting-state functional magnetic resonance imaging study. Pain 2019;160:2776-86. [Crossref] [PubMed]
- Coppola G, Di Renzo A, Tinelli E, Di Lorenzo C, Scapeccia M, Parisi V, Serrao M, Evangelista M, Ambrosini A, Colonnese C, Schoenen J, Pierelli F. Resting state connectivity between default mode network and insula encodes acute migraine headache. Cephalalgia 2018;38:846-54. [Crossref] [PubMed]
- Gollion C, Lerebours F, Nemmi F, Arribarat G, Bonneville F, Larrue V, Péran P. Insular functional connectivity in migraine with aura. J Headache Pain 2022;23:106. [Crossref] [PubMed]
- Lo Buono V, Bonanno L, Corallo F, Pisani LR, Lo Presti R, Grugno R, Di Lorenzo G, Bramanti P, Marino S. Functional connectivity and cognitive impairment in migraine with and without aura. J Headache Pain 2017;18:72. [Crossref] [PubMed]
- Arkink EB, Palm-Meinders IH, Koppen H, Milles J, van Lew B, Launer LJ, Hofman PAM, Terwindt GM, van Buchem MA, Ferrari MD, Kruit MC. Microstructural white matter changes preceding white matter hyperintensities in migraine. Neurology 2019;93:e688-94. [Crossref] [PubMed]
- Rahimi R, Dolatshahi M, Abbasi-Feijani F, Momtazmanesh S, Cattarinussi G, Aarabi MH, Pini L. Microstructural white matter alterations associated with migraine headaches: a systematic review of diffusion tensor imaging studies. Brain Imaging Behav 2022;16:2375-401. [Crossref] [PubMed]
- Yang H, Zhang J, Liu Q, Wang Y. Multimodal MRI-based classification of migraine: using deep learning convolutional neural network. Biomed Eng Online 2018;17:138. [Crossref] [PubMed]
- Tu Y, Zeng F, Lan L, Li Z, Maleki N, Liu B, Chen J, Wang C, Park J, Lang C, Yujie G, Liu M, Fu Z, Zhang Z, Liang F, Kong J. An fMRI-based neural marker for migraine without aura. Neurology 2020;94:e741-51. [Crossref] [PubMed]
- Garcia-Chimeno Y, Garcia-Zapirain B, Gomez-Beldarrain M, Fernandez-Ruanova B, Garcia-Monco JC. Automatic migraine classification via feature selection committee and machine learning techniques over imaging and questionnaire data. BMC Med Inform Decis Mak 2017;17:38. [Crossref] [PubMed]
- Rocca MA, Harrer JU, Filippi M. Are machine learning approaches the future to study patients with migraine? Neurology 2020;94:291-2. [Crossref] [PubMed]
- Fu T, Liu L, Huang X, Zhang D, Gao Y, Yin X, Lin H, Dai Y, Wu X. Cerebral blood flow alterations in migraine patients with and without aura: An arterial spin labeling study. J Headache Pain 2022;23:131. [Crossref] [PubMed]
- The International Classification of Headache Disorders, 3rd edition (beta version). Cephalalgia 2013;33:629-808.
- Zung WW. A rating instrument for anxiety disorders. Psychosomatics 1971;12:371-9. [Crossref] [PubMed]
- Zung WW. A self-rating depression scale. Arch Gen Psychiatry 1965;12:63-70. [Crossref] [PubMed]
- Nasreddine ZS, Phillips NA, Bédirian V, Charbonneau S, Whitehead V, Collin I, Cummings JL, Chertkow H. The Montreal Cognitive Assessment, MoCA: a brief screening tool for mild cognitive impairment. J Am Geriatr Soc 2005;53:695-9. [Crossref] [PubMed]
- Kosinski M, Bayliss MS, Bjorner JB, Ware JE Jr, Garber WH, Batenhorst A, Cady R, Dahlöf CG, Dowson A, Tepper S. A six-item short-form survey for measuring headache impact: the HIT-6. Qual Life Res 2003;12:963-74. [Crossref] [PubMed]
- Stewart WF, Lipton RB, Dowson AJ, Sawyer J. Development and testing of the Migraine Disability Assessment (MIDAS) Questionnaire to assess headache-related disability. Neurology 2001;56:S20-8. [Crossref] [PubMed]
- Cui Z, Zhong S, Xu P, He Y, Gong G. PANDA: a pipeline toolbox for analyzing brain diffusion images. Front Hum Neurosci 2013;7:42. [Crossref] [PubMed]
- Lin H, Liu Z, Yan W, Zhang D, Liu J, Xu B, Li W, Zhang Q, Cai X. Brain connectivity markers in advanced Parkinson's disease for predicting mild cognitive impairment. Eur Radiol 2021;31:9324-34. [Crossref] [PubMed]
- Wang J, Wang X, Xia M, Liao X, Evans A, He Y. GRETNA: a graph theoretical network analysis toolbox for imaging connectomics. Front Hum Neurosci 2015;9:386. [Crossref] [PubMed]
- Fan L, Li H, Zhuo J, Zhang Y, Wang J, Chen L, Yang Z, Chu C, Xie S, Laird AR, Fox PT, Eickhoff SB, Yu C, Jiang T. The Human Brainnetome Atlas: A New Brain Atlas Based on Connectional Architecture. Cereb Cortex 2016;26:3508-26. [Crossref] [PubMed]
- Lin H, Na P, Zhang D, Liu J, Cai X, Li W. Brain connectivity markers for the identification of effective contacts in subthalamic nucleus deep brain stimulation. Hum Brain Mapp 2020;41:2028-36. [Crossref] [PubMed]
- Lin H, Cai X, Zhang D, Liu J, Na P, Li W. Functional connectivity markers of depression in advanced Parkinson's disease. Neuroimage Clin 2020;25:102130. [Crossref] [PubMed]
- Michels L, Koirala N, Groppa S, Luechinger R, Gantenbein AR, Sandor PS, Kollias S, Riederer F, Muthuraman M. Structural brain network characteristics in patients with episodic and chronic migraine. J Headache Pain 2021;22:8. [Crossref] [PubMed]
- Karsan N, Bose PR, O'Daly O, Zelaya FO, Goadsby PJ. Alterations in Functional Connectivity During Different Phases of the Triggered Migraine Attack. Headache 2020;60:1244-58. [Crossref] [PubMed]
- Coppola G, Di Renzo A, Tinelli E, Petolicchio B, Parisi V, Serrao M, Porcaro C, Fiorelli M, Caramia F, Schoenen J, Di Piero V, Pierelli F. Thalamo-cortical networks in subtypes of migraine with aura patients. J Headache Pain 2021;22:58. [Crossref] [PubMed]
- Lai KL, Niddam DM. Brain Metabolism and Structure in Chronic Migraine. Curr Pain Headache Rep 2020;24:69. [Crossref] [PubMed]
- Torres-Ferrus M, Pareto D, Gallardo VJ, Cuberas-Borrós G, Alpuente A, Caronna E, Vila-Balló A, Lorenzo-Bosquet C, Castell-Conesa J, Rovira A, Pozo-Rosich P. Cortical metabolic and structural differences in patients with chronic migraine. An exploratory (18)FDG-PET and MRI study. J Headache Pain 2021;22:75. [Crossref] [PubMed]
- Mehnert J, May A. Functional and structural alterations in the migraine cerebellum. J Cereb Blood Flow Metab 2019;39:730-9. [Crossref] [PubMed]
- Huang X, Zhang D, Wang P, Mao C, Miao Z, Liu C, Xu C, Yin X, Wu X. Altered amygdala effective connectivity in migraine without aura: evidence from resting-state fMRI with Granger causality analysis. J Headache Pain 2021;22:25. [Crossref] [PubMed]
- Albrecht DS, Mainero C, Ichijo E, Ward N, Granziera C, Zürcher NR, Akeju O, Bonnier G, Price J, Hooker JM, Napadow V, Loggia ML, Hadjikhani N. Imaging of neuroinflammation in migraine with aura: A [11C]PBR28 PET/MRI study. Neurology 2019;92:e2038-50.
- Kim YS, Kim M, Choi SH, You SH, Yoo RE, Kang KM, Yun TJ, Lee ST, Moon J, Shin YW. Altered Vascular Permeability in Migraine-associated Brain Regions: Evaluation with Dynamic Contrast-enhanced MRI. Radiology 2019;292:713-20. [Crossref] [PubMed]
- Kim YE, Kim MK, Suh SI, Kim JH. Altered trigeminothalamic spontaneous low-frequency oscillations in migraine without aura: a resting-state fMRI study. BMC Neurol 2021;21:342. [Crossref] [PubMed]
- Zhang Y, Xu T, Wang Z, Li D, Du J, Wen Y, Zhao Y, Liao H, Liang F, Zhao L. Differences in topological properties of functional brain networks between menstrually-related and non-menstrual migraine without aura. Brain Imaging Behav 2021;15:1450-9. [Crossref] [PubMed]