Quantitative assessment of inflammation and evaluation of spatial heterogeneity for non-alcoholic fatty liver disease in mice based on iron-adjustive T1
Introduction
Non-alcoholic fatty liver disease (NAFLD), including non-alcoholic fatty liver (NAFL), non-alcoholic steatohepatitis (NASH), and NASH-related liver fibrosis, constitutes an increasing burden of chronic liver disease worldwide (1). NASH is characterised by steatosis with inflammation and hepatocellular injury, which progresses to cirrhosis and various liver-related complications (2). Patients with NASH have worse prognoses and higher mortality than those of normal people or patients with NAFL (3). However, NASH is reversible, and its prognosis can be improved by timely intervention (2). Therefore, it is essential to improve the identification of NASH, especially diagnosis methods.
Currently, the assessment of NASH severity relies on invasive liver biopsy, which is associated with sampling bias, underestimation of disease severity, and a risk of complications (4). Therefore, a non-invasive method is required. Serological tests (5), including Cytokeratin 18, were expected to replace the biopsy; but they could not specifically show the details of liver lesions, with unsatisfactory sensitivity and specificity. Magnetic resonance imaging (MRI) is non-invasive and reproducible, providing images of the whole liver. Corrected T1 (cT1), a recently developed MRI technology, can potentially help assess NASH. Studies have shown that T1 relaxation time could reflect the severity of fibrosis and inflammation; however, it is shortened by the presence of iron (6), and iron overload is common in NAFLD (7). Combined with T2*, which reflects the iron content, the shortening effect of iron on T1 is corrected as cT1 (8). Studies have suggested that a higher cT1 value is associated with greater histological inflammation and fibrosis (9,10); nevertheless, the contribution of cT1 in assessing inflammatory activity in NAFLD remains unclear (9,11).
NASH is characterised by spatial heterogeneity (12), which affects the diagnostic properties of liver biopsies and is related to the risk of disease progression (13). Therefore, assessing the spatial heterogeneity of liver lesions in NASH may provide additional information for prognostic assessment and clinical determination. Notably, MRI provides images of the whole liver, allowing the visualisation of heterogeneous lesions. Moreover, histogram analysis, which shows the number of pixels with the same intensity throughout images, has been widely used to assess heterogeneity in tumours to quantify the heterogeneity of diseases (14). However, the inflammatory heterogeneity of NASH using MRI and histograms remains unclear.
Our study aimed to investigate the ability of iron-adjustive T1 (aT1) to diagnose the inflammatory activity in the liver with NAFLD and the effect of fibrosis. Furthermore, we explored the heterogeneity of NAFLD using the histogram characters of aT1. We present this article in accordance with the ARRIVE reporting checklist (available at https://qims.amegroups.com/article/view/10.21037/qims-23-782/rc).
Methods
Animal model
This study was approved by the Independent Ethics Committee of Clinical Research and Animal Trials of the First Affiliated Hospital of Sun Yat-sen University (No. [2021] 862), in compliance with national and institutional guidelines for the care and use of animals. A protocol was prepared before the study without registration. After a week of adaptation to the standard condition, a total of 70 C57BL/6J mice (8-week-old, male, obtained from Jiangsu GemPhaematech Co., Ltd., Nanjing, China) were randomly divided into three groups with the following treatments: (I) NASH group (n=40): mice were fed a methionine and choline deficient (MCD) diet, and 10 mice were selected for MRI scan at each time point of 4, 6, 8, and 10 weeks. The advantage of MCD is inducting of NASH histological features within a short feeding period (15). (II) NASH-associated fibrosis group (n=20): 10 mice were fed the MCD diet for 4 and 6 weeks each, with an additional intraperitoneal injection of 10% CCl4-olive oil solution (at a dose of 2 mL/kg) once a week. Since the MCD alone did not result in enough fibrosis in the pre-experiment, CCl4 was used (16), which was feasible after histological evaluation. (III) Normal control group (n=10): the mice were fed with ordinary diet. Throughout the study period, all mice were housed in the specific pathogen free environment of our laboratory animal centre, with free food and water, an ambient temperature of 22±1 ℃, relative humidity of 50–60%, a light/dark cycle of 12 h/12 h, and lighting starting at 8:00 a.m. daily. Institutional Animal Care and Use Committee (IACUC) guidelines were used to define humane endpoints.
MRI scanning and image analysis
All scans were performed using a 3.0 T MR scanner (Ingenia CX, Philips Healthcare, Best, The Netherlands) with a 3.0-cm-diameter eight-channel animal coil. Mice were fasted for at least 6 h before scanning. After weighing, the mice were anaesthetized by intraperitoneal injection. During respiratory stabilization, all mice were placed in the prone position, and the tail went first. The areas of all scans encompassed the whole liver, including the T2-weighted imaging (T2WI), T1-weighted imaging (T1WI), T1 map, T2* map, and mDIXON. T1 and T2* maps were acquired using the modified Look-Locker inversion recovery and multi-echo gradient echo sequences, respectively. The specific scan parameters are listed in Table 1. aT1 maps were obtained by post-processing combined T1 and T2* maps, which is a similar approach as cT1 (LiverMultiScan), performed in MATLAB (MathWorks, Natick, MA, USA).
Table 1
Sequences | FOV (mm2) | Matrix | TR/TE (ms) | Thickness (mm) | Slice gap (mm) | Slice number |
---|---|---|---|---|---|---|
T2WI | 60×60 | 172×150 | 2,000/90 | 2.0 | 0 | 6 |
T1WI | 60×60 | 100×96 | 500/10 | 2.0 | 0 | 6 |
T1_map | 60×60 | 64×64 | 3.4/1.63 | 2.0 | 0 | 6 |
T2*_map | 60×60 | 64×64 | 27/2.0 | 2.0 | 0 | 6 |
mDIXON_Quant | 120×120 | 64×60 | 8.2/1.45 | 2.0 | 0 | 6 |
FOV, field of view; TR, repetition time; TE, echo time; T2WI, T2-weighted imaging; T1WI, T1-weighted imaging.
The T1, T2*, fat fraction (FF), and aT1 values were measured by outlining the region of interest (ROI) (Figure S1) at the post-processing station. The slice with the maximum area of the left and right liver lobes was found on the transaxial position T1WI, and the ROI was set to exclude the gallbladder, blood vessels, and bile ducts. The ROI was then copied to T1, T2*, and aT1 maps, and the value was recorded, respectively. Each ROI measurement was performed independently by two radiologists without awareness of the pathology scores (with 2 and 10 years of experience in liver MRI diagnosis, respectively). The mean ROI size was 24.53±12.3 mm2. Due to the different sizes of the left and right lobes, the mean ROI size of left lobe was 29.70±1.63 mm2, while the mean ROI size of right lobe was 19.27±1.27 mm2.
To obtain histogram features, the ROIs of the left and right liver lobes were out-lined separately at each layer of aT1 mapping based on ITK-SNAP (www.itksnap.org). The first and last layers of the liver were unlabelled to avoid partial volume effects, and the blood vessels, gallbladder, and liver edges were avoided. Histogram features were extracted using open-source software FAE (https://github.com/salan668/FAE.git), including the 10th percentile, 90th percentile, maximum, minimum, mean, median, entropy, interquartile range, kurtosis, skewness, range, mean absolute deviation, robust mean absolute deviation, root mean squared, uniformity, and variance.
Histology
Within 24 h after scanning, the left and right upper liver lobes of mice were dissected and fixed. Subsequently, paraffin sections across the largest cross section were selected for haematoxylin-eosin and Masson staining for NASH assessment and fibrosis scoring, respectively, and evaluated by a liver pathologist with 20 years of experience without awareness of MRI measurements. We referenced the NAFLD activity score (NAS) proposed by the NASH Clinical Re-search Network (NASH-CRN) (17). NAS ≥4 is mostly recommended as an inclusion criterion in NASH clinical studies (18); hence, we used the same threshold in this study, classifying sections with 0< NAS <4 as NAFL (or mild NAFLD) and sections with NAS ≥4 as NASH (or severe NAFLD). Since most mice liver sections lacked ballooning lesions, lobular inflammation and ballooning lesions were combined for analysis. An additional fibrosis score (F) was staged from 0–4 based on the NASH-CRN (F0: no fibrosis, F1: perisinusoidal or periportal fibrosis, F2: peri-sinusoidal and portal/periportal fibrosis, F3: bridging fibrosis, and F4: cirrhosis) (19).
Statistics analysis
SPSS (SPSS 22.0, SPSS Inc., Chicago, IL, USA), MedCalc (version 15.0, Mariakerke, Belgium), and GraphPad Prism (version 8.0; GraphPad Software, www.graphpad.com) were used for statistical analysis and plotting. We referred to previous results (9) and assumed an AUC of 80% for distinguishing between the NAFL and NASH. We then performed sample size estimation by MedCalc, assuming a null hypothesis of 0.6, a sample size ratio of 1 for the negative/positive group, α=0.05, and 20% modelling failure or accidental death, and the final estimated total sample size was 70.
The intraclass correlation coefficient (ICC) test was used to calculate the inter-observer agreement of MRI measured values. Data conforming to a normal distribution were described as mean ± standard deviation and the rest as median (quartiles). If the normal distribution and homogeneity of variance were satisfied, the difference between the two and multiple groups was compared using an independent samples t-test and analysis of variance (ANOVA), respectively. Otherwise, comparisons were made using Mann-Whitney U test (two groups) or Kruskal-Wallis H-test (multiple groups). Bonferroni correction was used to adjust p value. For correlation analysis, Pearson’s correlation and Spearman’s rank analyses were used for continuous variables conforming to a normal distribution and ordered variables, respectively. Lastly, the receiver operator characteristic (ROC) curve was used to analyse the diagnostic efficacy of the parameters. The evaluation indexes included the area under the curve (AUC), sensitivity, and specificity. Differences between AUCs were identified using the Delong test. Binary logistic regression analysis was used to assess the risk factors. P<0.05 was considered statistically significant.
Results
NASH model and staging
Liver specimens (n=116) from 58 mice were analysed after excluding accidental deaths and poor-quality scanned images (6 cases were missed in NASH group and 18 cases were missed in NASH-associated group). The liver pathology NAS ranged from 0–6, and ballooning lesions were only observed in the NASH with severe fibrosis groups. Fibrosis scores ranged from 0 to 3 without stage 1c fibrosis and cirrhosis (Figure 1). As shown in Figure 2, typical T1, T2*, and aT1 maps in the mice livers changed with the increase in NAS.
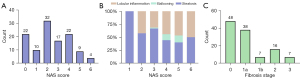
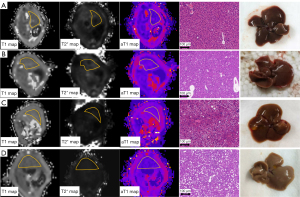
Relationship between MRI measurements and pathology scores
All MRI measurements showed good agreement (ICC ≥0.75) between both observers’ measurements (Table S1). As shown in Figure 3, the aT1 value was highly correlated with the NAS (r=0.747, P<0.001). However, the T1 (r=0.505, P<0.001), T2* (r=−0.614, P<0.001), and FF values (r=0.580, P<0.001) were moderately correlated with the NAS. The T2* (r=−0.666, P<0.001) and aT1 values (r=0.582, P<0.001) were moderately correlated with lobular inflammation and ballooning.
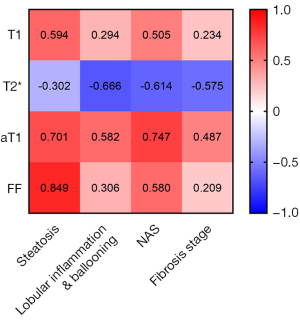
The aT1 and T1 values were moderately (r=0.487, P<0.001) and poorly correlated (r<0.3) with the fibrosis stage. However, since the fibrosis stage and NAS were highly correlated (r=0.699, P<0.001), with the NAS as the control variable, partial correlation analysis showed that the aT1 value was not correlated with the fibrosis stage (r=−0.135, P=0.147) and that the T1 and T2* values were mildly correlated with the fibrosis stage (rT1=−0.274, P=0.003; rT2*=−0.319, P<0.001) (Table S2).
The livers were classified into normal, NAFL, and NASH groups. The differences in the T1, T2*, aT1, and FF values were statistically significant (P<0.05) among the groups (Table 2). However, for all pairwise comparisons between the groups, only the difference in the aT1 values was statistically significant (P<0.001) (Figure 4).
Table 2
MRI measurements | Normal | 0< NAS <4 | NAS ≥4 | F/H | P |
---|---|---|---|---|---|
T1 (ms) | 738.53±9.54 | 770.10±6.70 | 807.14±11.13 | 10.932 | <0.001 |
T2* (ms) | 13.83±0.55 | 12.90 (9.38, 16.22) | 7.60±0.33 | 52.739 | <0.001 |
aT1 (ms) | 783.21±6.03 | 842.65±6.77 | 915.5±11.08 | 43.862 | <0.001 |
FF (%) | 2.59±0.23 | 6.28 (4.52, 9.27) | 6.89 (4.23, 12.03) | 41.033 | <0.001 |
Data are shown as mean ± SD or median (P25, P75). F (F statistic) was calculated using the Analysis of Variance test, and H (H statistic) was calculated using the Kruskal-Wallis test. MRI, magnetic resonance imaging; NAS, non-alcoholic fatty liver disease activity score; aT1, iron-adjustive T1; FF, fat fraction; SD, standard deviation.
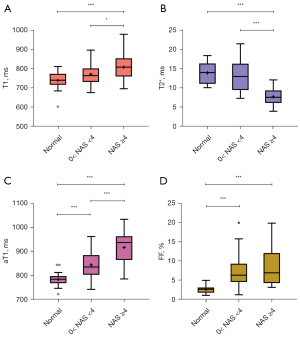
Furthermore, comparison of the T1, T2*, aT1, and FF values among groups with different degrees of fibrosis showed that the difference in the T1 values was not statistically significant (P=0.13), whereas the differences in the T2*, aT1, and FF values among the groups were statistically significant (P<0.05) (Table S3). Further pairwise comparison showed that the difference in the aT1 values of the mild and significant fibrosis groups was not significant (P=0.12) (Figure S2).
ROC curve analysis
To distinguish NAFLD mice from the normal group, the ROC curves of the T1, T2*, aT1, and FF values are shown in Figure 5A. As shown in Table 3, the AUC of aT1 was 0.891 [95% confidence interval (CI): 0.820–0.941], and that of FF was 0.937 (95% CI: 0.877–0.974), both higher than those of the T1 and T2* values (P<0.05). However, the difference between the AUC of aT1 and FF values was not statistically significant (P=0.28).
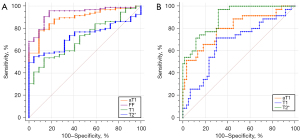
Table 3
MRI measurements | AUC (95% CI) | P | Cut-off value | Sensitivity (%) | Specificity (%) |
---|---|---|---|---|---|
aT1 | 0.891 (0.820–0.941) | <0.0001 | 811.68 ms | 77.89 | 90.91 |
T1 | 0.712 (0.621–0.792) | <0.0001 | 774.86 ms | 53.68 | 86.36 |
T2* | 0.731 (0.641–0.809) | <0.0001 | 10.425 ms | 54.74 | 95.45 |
FF | 0.937 (0.877–0.974) | <0.0001 | 3.07% | 95.79 | 81.82 |
ROC, receiver operator characteristic; FF, fat fraction; aT1, iron-adjustive T1; NAFLD, non-alcoholic fatty liver disease; MRI, magnetic resonance imaging; AUC, area under the curve; 95% CI, 95% confidence interval.
To differentiate the severe NAFLD (NAS ≥4) from mild NAFLD (0< NAS <4), the ROC curves of T1, T2*, and aT1 values are shown in Figure 5B. As shown in Table 4, the AUC of aT1 was 0.802 (95% CI: 0.708–0.877) and that of T2* was 0.897 (95% CI: 0.817–0.950), both higher than that of T1 (aT1 vs. T1, P=0.009; T2* vs. T1, P=0.001); the difference in the AUC between the aT1 and T2* value was not statistically significant (P=0.07).
Table 4
MRI measurements | AUC (95% CI) | P | Cut-off value | Sensitivity (%) | Specificity (%) |
---|---|---|---|---|---|
aT1 | 0.802 (0.708–0.877) | <0.0001 | 860.58 ms | 80.00 | 70.00 |
T1 | 0.670 (0.566–0.763) | 0.004 | 784.33 ms | 71.43 | 70.00 |
T2* | 0.897 (0.817–0.950) | <0.0001 | 10.54 ms | 97.14 | 68.33 |
ROC, receiver operator characteristic; aT1, iron-adjustive T1; NASH, non-alcoholic steatohepatitis; NAFL, non-alcoholic fatty liver; MRI, magnetic resonance imaging; AUC, area under the curve; 95% CI, 95% confidence interval.
Spatial heterogeneity analysis
The pathology score analysis in the left and right lobes of NAFLD mice (NAS >0) showed that the difference in the NAS was statistically significant (P<0.05). On comparing the differences in MRI measurements between the left and right lobes, the difference in aT1 values was not statistically significant in normal mice (Z=1.784, P=0.074); but it was statistically significant in NAFLD mice (Z=6.134, P<0.001) (Table S4).
Comparison of the histogram characteristics of aT1 in the normal and NAFL groups revealed statistically significant differences (P<0.05) in the 10th percentile, 90th percentile, minimum, mean, median, and root mean square, all of which were histogram features reflecting the magnitude of the values (Table S5); the remaining histogram features were not significantly different (P>0.05).
Comparison of the characteristics of aT1 histograms in the NAFL and the NASH groups showed statistically significant differences in the 10th percentile, 90th percentile, maximum, mean, median, and root mean square, reflecting the magnitude of the values. Moreover, entropy, interquartile range, range, and variance, reflecting the heterogeneity of the histogram, were significantly different between both groups (Table 5). However, the other histogram characteristics were not significantly different between both groups. A multifactorial logistic regression equation was constructed incorporating entropy, interquartile range, range, and variance. Only the entropy for NASH was found to be statistically significant [odds ratio (OR) =5.223, 95% CI: 1.449–18.826, P=0.011] (Table S6).
Table 5
Histogram features | 0< NAS <4 | NAS ≥4 | Z | P |
---|---|---|---|---|
10th percentile | 747.92 (718.18, 790.94) | 785.16 (750.15, 835.17) | 2.285 | 0.022* |
90th percentile | 955.28 (897.24, 996.52) | 1,009.64 (954.53, 1,071.18) | 3.367 | 0.001* |
Maximum | 1,077.19 (1,007.77, 1,139.15) | 1,137.27 (1,073.43, 1,208.66) | 2.729 | 0.006* |
Mean | 849.23 (802.29, 895.86) | 895.13 (864.72, 940.91) | 3.315 | 0.001* |
Minimum | 695.93 (649.70, 734.90) | 703.34 (658.93, 747.92) | 0.929 | 0.353 |
Median | 835.54 (800.08, 891.62) | 889.75 (859.83, 938.42) | 3.147 | 0.002* |
Root mean square | 853.41 (806.69, 899.21) | 902.58 (867.39, 943.46) | 3.339 | 0.001* |
Entropy | 2.58 (2.34, 2.81) | 2.75 (2.47, 3.00) | 2.149 | 0.032* |
Interquartile range | 104.42 (82.37, 127.11) | 112.16 (92.68, 152.9) | 1.998 | 0.046* |
Range | 366.30 (320.71, 448.42) | 426.59 (351.21, 493.69) | 2.240 | 0.025* |
Variance | 6,000.17 (4,010.09, 8,203.42) | 6,880.98 (4,838.18, 10,929.53) | 2.141 | 0.032* |
Kurtosis | 2.78 (2.41, 3.45) | 2.84 (2.48, 3.41) | 0.349 | 0.727 |
Mean absolute deviation | 62.95 (51.35, 72.86) | 66.66 (54.63, 86.83) | 1.943 | 0.052 |
Robust mean absolute deviation | 44.55 (36.01, 54.57) | 47.19 (37.96, 65.57) | 1.721 | 0.085 |
Skewness | 0.31 (0.06, 0.55) | 0.23 (−0.03, 0.59) | −0.531 | 0.595 |
Uniformity | 0.19 (0.16, 0.23) | 0.18 (0.14, 0.22) | −1.840 | 0.066 |
Data are shown as median (P25, P75). *, P<0.05. aT1, iron-adjustive T1; NAFL, non-alcoholic fatty liver; NASH, non-alcoholic steatohepatitis; NAS, non-alcoholic fatty liver disease activity score.
Discussion
We used multiparametric MRI to quantify liver lesions in an NAFLD mouse model and found that the aT1 value was highly correlated with the NAS of the liver but not with the degree of fibrosis. aT1 showed high diagnostic efficacy in differentiating normal mice from NAFLD mice and identifying NASH mice with NAS ≥4. Additionally, histogram analysis based on aT1 showed that inflammation was not homogeneous within the liver lobe and that the higher the degree of inflammation, the higher the spatial heterogeneity.
Hepatocyte damage, one of the most important features of NASH, triggers extracellular matrix remodelling and epithelial cell expansion (20), all of which may cause an increase in water content in tissues, consequently increasing the T1 values. However, we observed no difference in the T1 values between normal and NAFL mice, and negative correlation between T1 and fibrosis after NAS adjusting. A possible explanation is that the liver, an important regulatory centre of iron metabolism, stores a large amount of iron, which shortens the T1 relaxation time, consequently affecting the ability to detect inflammation based on T1. Furthermore, we found that the lower the T2* values, the more severe the liver inflammation, which is believed to reflect iron content, especially when steatosis is not severe and inflammation is significant (21). Therefore, it is critical to correct the influence of iron when using T1 relaxation time to detect liver diseases. Previous population-based studies have shown that iron correction is required in 39–54% of patients with NAFLD (9,22). Notably, when assessing NAFLD, the shortening effect of iron on T1 can significantly affect its diagnostic efficacy for inflammation.
Furthermore, our study showed that aT1 values were highly correlated with NAS in NAFLD mice and that they had enough specificity in diagnosing the degree of NAFLD in mice. NAS is closely related to disease progression or regression (23); therefore, an accurate diagnosis of NAFLD activity makes it easier to detect smaller changes, which is particularly essential in trials for the early evaluation of anti-inflammatory treatments. However, the evaluation of NAS relies on biopsy, which often results in a high rate of missed visits, leading to unreliable results or even experimental failure (24). Therefore, finding a non-invasive alternative endpoint may significantly decrease patient dropout rates and enable timely efficacy assessment. The advantage of cT1 in identifying high-risk NAFLD patients (NAS ≥4 and grade of fibrosis ≥2) has been shown in previous studies (10); additionally, it has shown an important role in screening patients with NAFLD suitable for biopsy. We found that aT1 values were highly correlated with NAS, indicating that aT1 values may be a suitable alternative to biopsy and can play a role in monitoring disease changes.
However, the aT1 value did not correlate with the degree of fibrosis. Inflammation and fibrosis often co-exist in a liver with NAFLD, which can increase the T1 relaxation time. However, previous studies have not clarified the relationship between cT1 levels and fibrosis. cT1 values can help differentiate the degree of fibrosis (9), but the confounding effect of inflammation was not excluded in previous studies. Another study (8) showed that the cT1 value was significantly correlated with the Ishak score of liver fibrosis, but the disease categories were not chosen. Moreover, cT1 has been shown to be unable to predict the Kleiner stage of liver fibrosis in patients with NAFLD (11). Our study showed no correlation between the aT1 value and fibrosis stage after controlling for the NAS. Previous studies have shown that increased hepatic iron levels in NAFLD may correlate with the degree of fibrosis (25,26). Although inflammation can also influence the distribution and content of iron, several studies have shown that iron may be more closely related to fibrosis, with hepatic iron being the main determinant of serum ferritin and varying according to the stage of fibrosis in NAFLD (27,28). This may be the reason why aT1 is not affected by mild fibrosis, especially in early NAFLD. When fibrosis is more advanced, the relationship between the degree of fibrosis and iron may be altered due to phenomena such as increased bone marrow iron, occult gastrointestinal bleeding, and burning out of NASH (29). This may be the reason why aT1 was unaffected by fibrosis in this study, at least not by early fibrosis.
On analysing the histogram characteristics of aT1, we found that a higher NAS in NAFLD mice was associated with a higher spatial heterogeneity of lesions, mainly for histogram characteristics reflecting the heterogeneity of the aT1 grey-scale histogram. And among all histogram features reflecting spatial distribution, entropy was a risk factor for NASH. There was no significant difference in the spatial heterogeneity of the liver between the normal and mild NAFLD groups. The present findings are consistent with those of 18F-fluorodeoxyglucose (18F-FDG) positron-emission tomography-computed tomography (PET/CT) in humans in a previous study (30), which showed that K1 values (representing the rate of FDG transport from blood to hepatic tissue) differed between liver segments, and the higher the NAS, the greater the difference in K1 values. Additionally, a study on 18F-FDGal (fluoro-2-deoxy-D-galactose) PET/CT showed higher heterogeneity of liver function in NASH than in NAFL (31). The heterogeneity may be due to differences in blood supply because each liver segment exhibits different uptake patterns (30). Reduced perfusion and dysfunction of the hepatic sinusoids have been reported in the liver with NAFLD (32); the reduced oxygen supply affects oxidation of fatty acids, leading to fat accumulation and hepatocyte damage, exacerbating the hepatic sinusoidal perfusion disorder, and producing a vicious cycle (33). Moreover, a study based on a mathematical model showed that higher heterogeneity of lesions among hepatic units might indicate more severe fibrosis progression in NAFLD (34). Another study of MR elastography also showed that spatial heterogeneity of MRI may reveal spatial patterns of stiffness changes in hepatitis (35). Furthermore, parameters based on MRI image heterogeneity allowed better differential diagnosis of hepatic fibrosis (36). Therefore, future research on histogram parameters of aT1 may help to detect heterogeneity and predict prognosis, especially the entropy. However, further study will be required to investigate the relationship between entropy and prognosis.
This study has some limitations. Firstly, no further analysis of the relationship between aT1 values and the characteristics of NAS (degree of steatosis, lobular inflammation, and ballooning) was performed, and hepatocyte ballooning was rare in our study. The effect of fat content on aT1 was also not further evaluated. However, previous studies have shown an interaction between NAS characteristics and that the relationship between the overall changes in NASH over time is stronger than that between individual NAS features (23,37). Many studies have shown that ballooning is less common in animal models, which may be attributable to differences in species (38). Therefore, the optimal diagnostic threshold values obtained from our animal-model-based exploration may not be directly applicable to humans, the effect of ballooning needs to be corrected before clinical application. Furthermore, severe fibrosis in a small number of mice in this study may have led to underestimation of the effect of fibrosis on aT1 values, but the estimation would not be affected by mild fibrosis, which may be useful for monitoring patients with NASH at an early stage.
Another question is that the sensitivity of aT1 was 77.89% when used to diagnose NAFLD, and a proportion of patients may be missed if aT1 is used alone for screening. A possible solution is to use FF to screen those patients with steatosis, combining with aT1 to further evaluate liver inflammation on the basis of FF.
Conclusions
We found that aT1 could help accurately assess the inflammatory activity of NAFLD mice independently of the degree of fibrosis, which may be useful in evaluating liver inflammation in patients with NAFLD, especially those without severe fibrosis, enabling timely reversal of the disease course. Lastly, we found that lesions in NAFLD were heterogeneously distributed in the liver. Additionally, histogram characteristics of aT1 can reflect the distribution of inflammation, and the higher degree of inflammation, the higher the spatial heterogeneity of aT1, potentially enabling the precise evaluation of NASH.
Acknowledgments
The authors thank Yansong Lin from The First Affiliated Hospital, Sun Yat-sen University for his help in generating animal models.
Funding: This work was supported by
Footnote
Reporting Checklist: The authors have completed the ARRIVE reporting checklist. Available at https://qims.amegroups.com/article/view/10.21037/qims-23-782/rc
Conflicts of Interest: All authors have completed the ICMJE uniform disclosure form (available at https://qims.amegroups.com/article/view/10.21037/qims-23-782/coif). The authors have no conflicts of interest to declare. KD is an employee in the Clinical & Technical Support team of Philips. She has a research partnership with the First Affiliated Hospital, Sun Yat-sen University. In this study, she provided technical support, like the MR scanning, post-processing, etc. The other authors have no conflicts of interest to declare.
Ethical Statement: The authors are accountable for all aspects of the work in ensuring that questions related to the accuracy or integrity of any part of the work are appropriately investigated and resolved. The animal study was approved by the Independent Ethics Committee of Clinical Research and Animal Trials of the First Affiliated Hospital of Sun Yat-sen University (No. [2021] 862), in compliance with national and institutional guidelines for the care and use of animals. A protocol was prepared before the study without registration.
Open Access Statement: This is an Open Access article distributed in accordance with the Creative Commons Attribution-NonCommercial-NoDerivs 4.0 International License (CC BY-NC-ND 4.0), which permits the non-commercial replication and distribution of the article with the strict proviso that no changes or edits are made and the original work is properly cited (including links to both the formal publication through the relevant DOI and the license). See: https://creativecommons.org/licenses/by-nc-nd/4.0/.
References
- Younossi Z, Tacke F, Arrese M, Chander Sharma B, Mostafa I, Bugianesi E, Wai-Sun Wong V, Yilmaz Y, George J, Fan J, Vos MB. Global Perspectives on Nonalcoholic Fatty Liver Disease and Nonalcoholic Steatohepatitis. Hepatology 2019;69:2672-82. [Crossref] [PubMed]
- Anstee QM, Reeves HL, Kotsiliti E, Govaere O, Heikenwalder M. From NASH to HCC: current concepts and future challenges. Nat Rev Gastroenterol Hepatol 2019;16:411-28. [Crossref] [PubMed]
- Sheka AC, Adeyi O, Thompson J, Hameed B, Crawford PA, Ikramuddin S. Nonalcoholic Steatohepatitis: A Review. JAMA 2020;323:1175-83. [Crossref] [PubMed]
- Ratziu V, Charlotte F, Heurtier A, Gombert S, Giral P, Bruckert E, Grimaldi A, Capron F, Poynard TLIDO Study Group. Sampling variability of liver biopsy in nonalcoholic fatty liver disease. Gastroenterology 2005;128:1898-906. [Crossref] [PubMed]
- Masoodi M, Gastaldelli A, Hyötyläinen T, Arretxe E, Alonso C, Gaggini M, Brosnan J, Anstee QM, Millet O, Ortiz P, Mato JM, Dufour JF, Orešič M. Metabolomics and lipidomics in NAFLD: biomarkers and non-invasive diagnostic tests. Nat Rev Gastroenterol Hepatol 2021;18:835-56. [Crossref] [PubMed]
- Hoad CL, Palaniyappan N, Kaye P, Chernova Y, James MW, Costigan C, Austin A, Marciani L, Gowland PA, Guha IN, Francis ST, Aithal GP. A study of T1 relaxation time as a measure of liver fibrosis and the influence of confounding histological factors. NMR Biomed 2015;28:706-14. [Crossref] [PubMed]
- Britton LJ, Subramaniam VN, Crawford DH. Iron and non-alcoholic fatty liver disease. World J Gastroenterol 2016;22:8112-22. [Crossref] [PubMed]
- Banerjee R, Pavlides M, Tunnicliffe EM, Piechnik SK, Sarania N, Philips R, Collier JD, Booth JC, Schneider JE, Wang LM, Delaney DW, Fleming KA, Robson MD, Barnes E, Neubauer S. Multiparametric magnetic resonance for the non-invasive diagnosis of liver disease. J Hepatol 2014;60:69-77. [Crossref] [PubMed]
- Pavlides M, Banerjee R, Tunnicliffe EM, Kelly C, Collier J, Wang LM, Fleming KA, Cobbold JF, Robson MD, Neubauer S, Barnes E. Multiparametric magnetic resonance imaging for the assessment of non-alcoholic fatty liver disease severity. Liver Int 2017;37:1065-73. [Crossref] [PubMed]
- Andersson A, Kelly M, Imajo K, Nakajima A, Fallowfield JA, Hirschfield G, Pavlides M, Sanyal AJ, Noureddin M, Banerjee R, Dennis A, Harrison S. Clinical Utility of Magnetic Resonance Imaging Biomarkers for Identifying Nonalcoholic Steatohepatitis Patients at High Risk of Progression: A Multicenter Pooled Data and Meta-Analysis. Clin Gastroenterol Hepatol 2022;20:2451-2461.e3. [Crossref] [PubMed]
- Eddowes PJ, McDonald N, Davies N, Semple SIK, Kendall TJ, Hodson J, Newsome PN, Flintham RB, Wesolowski R, Blake L, Duarte RV, Kelly CJ, Herlihy AH, Kelly MD, Olliff SP, Hübscher SG, Fallowfield JA, Hirschfield GM. Utility and cost evaluation of multiparametric magnetic resonance imaging for the assessment of non-alcoholic fatty liver disease. Aliment Pharmacol Ther 2018;47:631-44. [Crossref] [PubMed]
- Regev A, Berho M, Jeffers LJ, Milikowski C, Molina EG, Pyrsopoulos NT, Feng ZZ, Reddy KR, Schiff ER. Sampling error and intraobserver variation in liver biopsy in patients with chronic HCV infection. Am J Gastroenterol 2002;97:2614-8. [Crossref] [PubMed]
- Steinman JB, Salomao MA, Pajvani UB. Zonation in NASH - A key paradigm for understanding pathophysiology and clinical outcomes. Liver Int 2021;41:2534-46. [Crossref] [PubMed]
- Just N. Improving tumour heterogeneity MRI assessment with histograms. Br J Cancer 2014;111:2205-13. [Crossref] [PubMed]
- Reimer KC, Wree A, Roderburg C, Tacke F. New drugs for NAFLD: lessons from basic models to the clinic. Hepatol Int 2020;14:8-23. [Crossref] [PubMed]
- Tsuchida T, Lee YA, Fujiwara N, Ybanez M, Allen B, Martins S, Fiel MI, Goossens N, Chou HI, Hoshida Y, Friedman SL. A simple diet- and chemical-induced murine NASH model with rapid progression of steatohepatitis, fibrosis and liver cancer. J Hepatol 2018;69:385-95. [Crossref] [PubMed]
- Kleiner DE, Brunt EM, Van Natta M, Behling C, Contos MJ, Cummings OW, Ferrell LD, Liu YC, Torbenson MS, Unalp-Arida A, Yeh M, McCullough AJ, Sanyal AJNonalcoholic Steatohepatitis Clinical Research Network. Design and validation of a histological scoring system for nonalcoholic fatty liver disease. Hepatology 2005;41:1313-21. [Crossref] [PubMed]
- Sanyal AJ, Brunt EM, Kleiner DE, Kowdley KV, Chalasani N, Lavine JE, Ratziu V, McCullough A. Endpoints and clinical trial design for nonalcoholic steatohepatitis. Hepatology 2011;54:344-53. [Crossref] [PubMed]
- Noureddin M, Truong E, Gornbein JA, Saouaf R, Guindi M, Todo T, Noureddin N, Yang JD, Harrison SA, Alkhouri N. MRI-based (MAST) score accurately identifies patients with NASH and significant fibrosis. J Hepatol 2022;76:781-7. [Crossref] [PubMed]
- Cordero-Espinoza L, Huch M. The balancing act of the liver: tissue regeneration versus fibrosis. J Clin Invest 2018;128:85-96. [Crossref] [PubMed]
- Imajo K, Kessoku T, Honda Y, Hasegawa S, Tomeno W, Ogawa Y, Motosugi U, Saigusa Y, Yoneda M, Kirikoshi H, Yamanaka S, Utsunomiya D, Saito S, Nakajima A. MRI-Based Quantitative R2(*) Mapping at 3 Tesla Reflects Hepatic Iron Overload and Pathogenesis in Nonalcoholic Fatty Liver Disease Patients. J Magn Reson Imaging 2022;55:111-25. [Crossref] [PubMed]
- Milic S, Mikolasevic I, Orlic L, Devcic E, Starcevic-Cizmarevic N, Stimac D, Kapovic M, Ristic S. The Role of Iron and Iron Overload in Chronic Liver Disease. Med Sci Monit 2016;22:2144-51. [Crossref] [PubMed]
- Kleiner DE, Brunt EM, Wilson LA, Behling C, Guy C, Contos M, et al. Association of Histologic Disease Activity With Progression of Nonalcoholic Fatty Liver Disease. JAMA Netw Open 2019;2:e1912565. [Crossref] [PubMed]
- Rowe IA, Parker R. Liver biopsy for the selection of patients with nonalcoholic steatohepatitis for clinical trials. Gastroenterology 2015;148:262. [Crossref] [PubMed]
- Maliken BD, Nelson JE, Klintworth HM, Beauchamp M, Yeh MM, Kowdley KV. Hepatic reticuloendothelial system cell iron deposition is associated with increased apoptosis in nonalcoholic fatty liver disease. Hepatology 2013;57:1806-13. [Crossref] [PubMed]
- Valenti L, Fracanzani AL, Bugianesi E, Dongiovanni P, Galmozzi E, Vanni E, Canavesi E, Lattuada E, Roviaro G, Marchesini G, Fargion S. HFE genotype, parenchymal iron accumulation, and liver fibrosis in patients with nonalcoholic fatty liver disease. Gastroenterology 2010;138:905-12. [Crossref] [PubMed]
- Ryan JD, Armitage AE, Cobbold JF, Banerjee R, Borsani O, Dongiovanni P, Neubauer S, Morovat R, Wang LM, Pasricha SR, Fargion S, Collier J, Barnes E, Drakesmith H, Valenti L, Pavlides M. Hepatic iron is the major determinant of serum ferritin in NAFLD patients. Liver Int 2018;38:164-73. [Crossref] [PubMed]
- Valenti L, Dongiovanni P, Fracanzani AL, Santorelli G, Fatta E, Bertelli C, Taioli E, Fiorelli G, Fargion S. Increased susceptibility to nonalcoholic fatty liver disease in heterozygotes for the mutation responsible for hereditary hemochromatosis. Dig Liver Dis 2003;35:172-8. [Crossref] [PubMed]
- Angulo P, George J, Day CP, Vanni E, Russell L, De la Cruz AC, Liaquat H, Mezzabotta L, Lee E, Bugianesi E. Serum ferritin levels lack diagnostic accuracy for liver fibrosis in patients with nonalcoholic fatty liver disease. Clin Gastroenterol Hepatol 2014;12:1163-1169.e1. [Crossref] [PubMed]
- Sarkar S, Corwin MT, Olson KA, Stewart SL, Liu CH, Badawi RD, Wang G. Pilot Study to Diagnose Nonalcoholic Steatohepatitis With Dynamic (18)F-FDG PET. AJR Am J Roentgenol 2019;212:529-37. [Crossref] [PubMed]
- Eriksen PL, Thomsen KL, Larsen LP, Grønbaek H, Vilstrup H, Sørensen M. Non-alcoholic steatohepatitis, but not simple steatosis, disturbs the functional homogeneity of the liver - a human galactose positron emission tomography study. Aliment Pharmacol Ther 2019;50:84-92. [Crossref] [PubMed]
- McCuskey RS, Ito Y, Robertson GR, McCuskey MK, Perry M, Farrell GC. Hepatic microvascular dysfunction during evolution of dietary steatohepatitis in mice. Hepatology 2004;40:386-93. [Crossref] [PubMed]
- Anavi S, Madar Z, Tirosh O. Non-alcoholic fatty liver disease, to struggle with the strangle: Oxygen availability in fatty livers. Redox Biol 2017;13:386-92. [Crossref] [PubMed]
- Schuppan D, Surabattula R, Wang XY. Determinants of fibrosis progression and regression in NASH. J Hepatol 2018;68:238-50. [Crossref] [PubMed]
- Fried MJ, Warren PM, Drummond GB. Oxygen uptake during rebreathing in a Mapleson A system. Br J Anaesth 1994;72:217-8. [Crossref] [PubMed]
- Veira DM, Butler G, Proulx JG, Poste LM. Utilization of grass silage by cattle: effect of supplementation with different sources and amounts of protein. J Anim Sci 1994;72:1403-8. [Crossref] [PubMed]
- Brunt EM, Kleiner DE, Wilson LA, Sanyal AJ, Neuschwander-Tetri BANonalcoholic Steatohepatitis Clinical Research Network. Improvements in Histologic Features and Diagnosis Associated With Improvement in Fibrosis in Nonalcoholic Steatohepatitis: Results From the Nonalcoholic Steatohepatitis Clinical Research Network Treatment Trials. Hepatology 2019;70:522-31. [Crossref] [PubMed]
- Denk H, Abuja PM, Zatloukal K. Animal models of NAFLD from the pathologist's point of view. Biochim Biophys Acta Mol Basis Dis 2019;1865:929-42. [Crossref] [PubMed]