Using an artificial intelligence model to detect and localize visible clinically significant prostate cancer in prostate magnetic resonance imaging: a multicenter external validation study
Introduction
Prostate multiparametric magnetic resonance imaging (mpMRI) is a non-invasive examination used for the detection and localization of clinically significant prostate cancer (csPCa). It aims to increase the positive rate of biopsies and reduce unnecessary biopsies (1). Multiple studies (1-3) have advocated for the use of mpMRI prior to biopsy to identify high-risk patients and pinpoint target areas for subsequent biopsy. However, work efficiency needs to be enhanced and variation in prostate mpMRI interpretations needs to be minimized to meet the escalating demand for MRI-based diagnosis (4).
The Prostate Imaging Reporting and Data System (PI-RADS) (5-8) is continuously updated to standardize prostate mpMRI interpretation. However, it still demonstrates some degree of variability (9,10). Intra-reader agreement ranges from 60% to 74%, while inter-reader agreement falls below 50% (9). Such variation has resulted in discrepancies in the diagnostic efficacy of image-guided targeted biopsies, with csPCa detection rates varying by up to 40% for PI-RADS 5 lesions (11). Further, the steep learning curve poses challenges for practitioners, and mpMRI reporting necessitates a high level of expertise (12,13).
The integration of deep-learning models and mpMRI has shown promise in providing automated and scalable assistance in identifying biopsy candidates and guiding biopsy sampling (14). Previous studies have reported that the performance of prostate artificial intelligence (AI) models has been encouraging in specific data sets; however, the generalization and clinical applicability of these models have not been extensively investigated. AI models need to be validated in external cohorts before any consideration is given to their clinical deployment (15).
In this multicenter study, we developed an AI model and validated it in an external data set to evaluate its diagnostic efficacy in detecting and localizing csPCa. We present this article in accordance with the TRIPOD reporting checklist (available at https://qims.amegroups.com/article/view/10.21037/qims-23-791/rc).
Methods
This retrospective study was approved by the Institutional Review Board (IRB) of Peking University First Hospital (IRB number: 2021060). The requirement of individual consent for this retrospective analysis was waived. The study was conducted in accordance with the Declaration of Helsinki (as revised in 2013).
Data inclusion
Figure 1 shows the data enrollment process. In total, 2,221 consecutive prostate MR images acquired between January 2014 and December 2019 were retrospectively collected from 16 MR scanners in four hospitals (Hospital 1, Hospital 2, Hospital 3, and Hospital 4) to develop an AI model for csPCa segmentation. Further, 580 consecutive prostate MR images acquired between January 2020 and November 2021 were retrospectively collected from 14 MR scanners in three hospitals (Hospital 1, Hospital 2, and Hospital 3) to establish an external validation data set. Importantly, the images used in the development data set for the AI model and the external validation data set were mutually exclusive and adhered to the same inclusion and exclusion criteria.
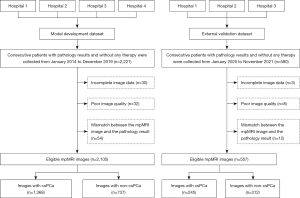
To be eligible for inclusion in the study, patients had to meet the following inclusion criteria: (I) have undergone mpMRI prior to biopsy with a clinical suspicion of prostate cancer (PCa) due to an elevated serum prostate-specific antigen (PSA) level, abnormal findings during a digital rectal examination, and/or abnormal transrectal ultrasound results; (II) have undergone an image-guided biopsy, transurethral prostatectomy, or radical prostatectomy within one month of the MRI examination and received pathological confirmation; (III) have not undergone any PCa-related treatment prior to the examination; and (IV) have tested negative for PCa during biopsy and showed no potential signs of PCa during clinical follow-up for over 1 year. Clinical information, including age, total PSA levels in serum, and pathology results, was collected for all patients.
Patients were excluded from the study if they met any of the following exclusion criteria: (I) they had incomplete image data; (II) the quality of the image was poor; and/or (III) there were inconsistencies between the MRI image and the pathology results, such as variations in tumor location, MRI-invisible csPCa, and imaging that indicated csPCa but pathology results that confirmed its absence.
MR scanning protocols
Prostate MRI images were acquired using 16 different MR scanners for the model development data set and 14 different MR scanners for the external validation data set. The imaging setup involved the use of body coils as transmit coils and phased array coils as receiver coils, but no use of endorectal coils. The MRI sequences included T1-weighted imaging, T2-weighted imaging (T2WI), diffusion-weighted imaging (DWI), and apparent diffusion coefficient (ADC) maps. The acquisition of a dynamic contrast-enhanced sequence was not mandatory. For the DWI, a diffusion-weighted single-shot gradient echo planar imaging sequence was used. For the T2WI, a T2-weighted fast spin echo sequence at both 3.0 T and 1.5 T was used. The ADC maps were generated from the DWI sequence using high and low b-values. Detailed information regarding the MR scanning protocols for both the model development data set and the external validation data set is presented in Table 1.
Table 1
Parameters | Model development data set | External validation data set | ||||
---|---|---|---|---|---|---|
Training (N=1,681) | Validation (N=212) | Test (N=212) | P | Overall (N=557) | ||
DWI/ADC | ||||||
Model name | 0.87 | |||||
Achieva | 52 (3.1) | 5 (2.4) | 4 (1.9) | 13 (2.3) | ||
Aera | 217 (12.9) | 32 (15.1) | 22 (10.4) | 22 (3.9) | ||
Amira | 1 (0.1) | 1 (0.5) | 0 (0.0) | 0 (0.0) | ||
DISCOVERY MR750 | 844 (50.2) | 112 (52.8) | 109 (51.4) | 243 (43.6) | ||
DISCOVERY MR750w | 75 (4.5) | 13 (6.1) | 7 (3.3) | 44 (7.9) | ||
Ingenia | 107 (6.4) | 8 (3.8) | 19 (9.0) | 45 (8.1) | ||
Ingenia CX | 1 (0.1) | 0 (0.0) | 0 (0.0) | 1 (0.2) | ||
Multiva | 24 (1.4) | 2 (0.9) | 4 (1.9) | 3 (0.5) | ||
Prisma | 29 (1.7) | 2 (0.9) | 4 (1.9) | 20 (3.6) | ||
SIGNA EXCITE | 36 (2.1) | 2 (0.9) | 4 (1.9) | 0 (0.0) | ||
Signa HDxt | 3 (0.2) | 0 (0.0) | 0 (0.0) | 1 (0.2) | ||
SIGNA Premier | 1 (0.1) | 0 (0.0) | 0 (0.0) | 0 (0.0) | ||
Skyra | 46 (2.7) | 6 (2.8) | 8 (3.8) | 25 (4.5) | ||
TrioTim | 114 (6.8) | 17 (8.0) | 18 (8.5) | 108 (19.4) | ||
uMR 790 | 87 (5.2) | 8 (3.8) | 7 (3.3) | 6 (1.1) | ||
Verio | 44 (2.6) | 4 (1.9) | 6 (2.8) | 25 (4.5) | ||
MAGNETOM_ESSENZA | 0 (0.0) | 0 (0.0) | 0 (0.0) | 1 (0.2) | ||
Magnetic field | 0.38 | |||||
1.5 T | 254 (15.1) | 36 (17.0) | 26 (12.3) | 27 (4.8) | ||
3.0 T | 1,427 (84.9) | 176 (83.0) | 186 (87.7) | 530 (95.2) | ||
B value (s/mm2) | 1,400 [1,400, 1,400] | 1,400 [1,400, 1,400] | 1,400 [1,400, 1,400] | 0.70 | 1,400 [1,400, 1,400] | |
Slice thickness (mm) | 4.00 [4.00, 4.00] | 4.00 [4.00, 4.00] | 4.00 [4.00, 4.00] | 0.96 | 4.0 [3.0, 5.0] | |
Slice spacing (mm) | 4.00 [4.00, 4.50] | 4.00 [4.00, 4.50] | 4.00 [4.00, 4.50] | 0.87 | 4.0 [3.0, 6.5] | |
Repetition time (ms) | 3,000 [2,640, 4,380] | 2,930 [2,640, 4,110] | 2,910 [2,640, 4,130] | 0.76 | 2,671 [2,000, 6,759] | |
Echo time (ms) | 61.3 [59.7, 63.8] | 61.2 [60.0, 63.7] | 61.3 [59.7, 63.5] | 0.97 | 61 [51, 93] | |
Field of view (mm) | 240 [220, 250] | 240 [220, 250] | 240 [220, 250] | 0.34 | 240 [220, 250] | |
Flip angle (°) | 90 [90, 90] | 90 [90, 90] | 90 [90, 90] | 0.81 | 90 [90, 90] | |
T2WI | ||||||
Slice thickness (mm) | – | – | – | – | 4.0 [3.5, 4.0] | |
Slice spacing (mm) | – | – | – | – | 4.0 [4.0, 4.0] | |
Repetition time (ms) | – | – | – | – | 3,340 [3,000, 4,000] | |
Echo time (ms) | – | – | – | – | 95 [87, 106] | |
Field of view (mm) | – | – | – | – | 240 [200, 240] | |
Flip angle (°) | – | – | – | – | 90 [90, 90] |
The quantitative variables are presented as the median [Q1, Q3] for the non-normalized data. The categorical variables are presented as n (%). MR, magnetic resonance; DWI, diffusion-weighted imaging; ADC, apparent diffusion coefficient; T2WI, T2-weighted imaging.
Patient classification and localization of csPCa
All the patients included in this study underwent a transrectal ultrasonography-guided systematic biopsy using either 12- or 6-core needles, as well as a cognitive-targeted biopsy. The process of the systematic and cognitive-targeted biopsy is described in detail in Appendix 1. The pathology results from both biopsy approaches were combined (16). Sextants showing the highest grade assigned by either the systematic biopsy or the cognitive-targeted biopsy were categorized based on the International Society of Urological Pathology (ISUP) grade, while all other sextants were graded based on the systematic biopsy results. Some patients in this study subsequently underwent transurethral prostatectomy or radical prostatectomy. When obtaining the final comprehensive pathological diagnosis, priority was given to the pathology results from radical prostatectomy over those from transurethral prostatectomy, with the combined biopsy results ranked last. The ISUP grading system was used to classify patients as non-csPCa (the no-cancer or ISUP group 1) or csPCa (ISUP group 2). Patients with negative PCa biopsies were classified as negative if they had an additional follow-up period of at least one year and no evidence of underlying PCa.
All the cases included in this study underwent a retrospective review and were delineated based on the comprehensive pathological diagnosis results of each lesion by two uro-radiologists (Zhaonan Sun and Xiaoying Wang, who had 5 and 30 years of experience in prostate MRI interpretation, respectively) in consensus. The open-source software ITK-SNAP (version 3.8.0; available at http://www.itksnap.org) was used to annotate the tumor foci.
Sextant areas were automatically generated using the prostate sextant location model (Appendix 2). Subsequently, these sextant areas were classified as cancerous or non-cancerous based on the presence or absence of cancer within each sextant. For instance, if the ground-truth segmentation overlapped with a sextant, it was categorized as a cancerous area.
AI model development
In this study, we employed an end-to-end AI model comprising the following four components: (I) MRI sequence classification; (II) prostate gland segmentation and measurement (17); (III) prostate zonal anatomy segmentation; and (IV) csPCa foci segmentation and measurement (18). These models were executed automatically in sequence. Based on the identified suspicious areas, various parameters, including the number of suspicious lesions, three-dimensional (3D) diameter, volume, sextant location, and average ADC value, were calculated and automatically incorporated into the PI-RADS structured report (19). The primary focus of this study was the csPCa foci segmentation model, which provided a binary output. Detailed information about the other AI models can be found in Appendix 2.
Preprocessing
The DWI, ADC maps, and T2WI were registered through rigid transformation using the coordinate information stored in the Digital Imaging and Communications in Medicine (DICOM) image headers. For the automatic pre-segmentation of the prostate gland region, a model (17) previously developed at our institution was employed; detailed information about the latest updated model can be found in Appendix 2. All the prostate areas were standardized and cropped to a size of 64×64×64 (x, y, z), with pixel intensity normalized to the range of [0, 1]. To augment the training set, random rotations (within a range of 10 degrees), random noise, and parallel translations within the range of [(–0.1, 0.1); (–0.1, 0.1)] pixels were applied.
Deep learning
The collected data were divided randomly into the following three data sets: a training data set (comprising 80% of the data); a validation data set (comprising 10% of the data); and an internal test data set (comprising10% of the data). The AI model used a combination of DWI and ADC maps as input for the PCa segmentation (18). A cascade 3D U-Net framework (20) was employed for the segmentation process. The training processes were conducted using the NVIDIA Tesla P100 16G GPU. The algorithm was implemented using Python 3.6, PyTorch 0.4.1, OpenCV 3.4.0.12, Numpy 1.16.2, and SimpleITK 1.2.0. Taking into consideration aspects such as computational efficiency, generalization, training stability, and data set size, a batch size of 60 was employed during training, and 1,000 epochs were conducted to train the networks. The optimization algorithm employed was the Adaptive Moment Estimation (Adam) gradient descent, which had a learning rate of 0.0001, and the binary cross-entropy loss function was minimized.
Post-processing
Based on the current capabilities of mpMRI, csPCa volumes greater than or equal to 0.5 cc can feasibly be detected (7). However, the current use of AI models for csPCa diagnosis is often associated with a high rate of false positive (FP) results (21-23). To address the potential impact of the very small, predicted tumor foci, an output threshold of 0.5 cc was adopted. It is important to emphasize that this post-processing step was only performed on the test and external validation data sets and was not performed on the training and validation sets. The rationale for adopting this approach during the training phase was to enhance the training efficiency and enable the model to learn from a diverse range of cancerous voxels in which a volume threshold was deliberately omitted.
Evaluation of the AI prediction results
At the lesion level, each connected domain predicted by the AI model was regarded as a predicted lesion. For each patient, we focused on studying the four largest lesions. To assess the performance of the AI model, we compared the predicted lesions with the reference standard. The spatial overlap between the predicted lesion and the reference standard was measured using the mean dice similarity coefficient (DSC). If a lesion overlapped with the reference standard, it was classified as a true positive (TP) lesion. Conversely, if an AI-predicted lesion did not overlap with the reference standard, it was classified as an FP lesion. Similarly, if a reference lesion did not overlap with any AI-predicted lesion, it was classified as a false negative (FN) lesion.
At the sextant level, the right-upper, right-middle, right-lower, left-upper, left-middle, and left-lower regions of the prostate gland were independently examined. The presence of lesion overlap within a sextant was defined as a positive finding. Thus, if a sextant overlapped with both the reference lesion and AI-predicted lesion, it was classified as a TP sextant. If a sextant only overlapped with the reference lesion and not the AI-predicted lesion, it was classified as a FN sextant. Conversely, if a sextant only overlapped with the AI-predicted lesion and not the reference lesion, it was classified as a FP sextant. Finally, if a sextant did not overlap with either the reference lesion or the AI-predicted lesion, it was classified as a true negative (TN) sextant.
At the patient level, a patient with any TP sextant was classified as a TP case. A patient with all TN sextants was classified as a TN case. A patient with TN and FP sextants, without any TP sextants, was classified as a FP case. Similarly, a patient with TN and FN sextants without any TP sextants was classified as a FN case.
To assess the ability of the AI model to detect lesions, we calculated the sensitivity at the lesion level. At the sextant level, we evaluated sensitivity, specificity, and accuracy to assess the ability of the AI model to localize lesions, comparing these metrics across the six sextants. Additionally, sensitivity, specificity, and accuracy were calculated at the patient level to evaluate the ability of the AI model to identify biopsy candidates. The accuracy of the AI model at the patient level was also examined; the tumor number, volume, and average ADC value were considered contributing factors.
Statistical analysis
The statistical analyses were conducted using the R software (version 4.2.0). For the normalized data, the quantitative variables are presented as the mean (standard deviation). For the non-normalized data, the quantitative variables are presented as the median (Q1, Q3). The categorical variables are reported as numbers (percentages). The Wilcoxon test was used to compare the quantitative variables, and the chi-square test was used to compare the categorical variables. Segmentation metrics, including the DSC, Jaccard Coefficient (JACARD), volume similarity (VS), Hausdorff distance (HD), and average distance (AD), were calculated and compared by an analysis of variance.
A free-response receiver operating characteristic (FROC) analysis was conducted to assess the lesion detection accuracy of the AI model. A Bland-Altman analysis was used to evaluate the measured values of PCa. All the statistical tests were two-tailed, and a significance level of 5% was applied.
Results
Clinical characteristics
The model development data set comprised 2,105 patients, of whom 1,368 had a confirmed diagnosis of PCa and 737 did not have PCa. For the external validation, the data set comprised 557 patients, of whom 245 had a confirmed diagnosis of PCa and 312 did not have PCa. The clinical characteristics of these patients are presented in Table 2.
Table 2
Parameters | Model development data set | External validation data set | P value | |||
---|---|---|---|---|---|---|
Negative (N=737) | Positive (N=1,368) | Negative (N=312) | Positive (N=245) | |||
Age (years) | 64.5 (60, 69) | 69.0 (63.0, 77.0) | 65.5 (60.0, 71.0) | 70.0 (64.0, 76.0) | 0.23 | |
tPSA value | 7.5 (6.2, 8.6) | 16.2 (10.6, 32.5) | 8.22 (5.07, 18.8) | 12.1 (6.87, 33.2) | <0.001 | |
Final ISUP group | <0.001 | |||||
Biopsy-NoPCa | 698 (94.7) | 0 (0.0) | 299 (95.8) | 0 (0.0) | ||
1 | 39 (5.3) | 0 (0.0) | 13 (4.2) | 0 (0.0) | ||
2 | 0 (0.0) | 628 (45.9) | 0 (0.0) | 101 (41.2) | ||
3 | 0 (0.0) | 208 (15.2) | 0 (0.0) | 40 (16.3) | ||
4 | 0 (0.0) | 164 (12.0) | 0 (0.0) | 37 (15.1) | ||
5 | 0 (0.0) | 232 (17.0) | 0 (0.0) | 46 (18.8) | ||
Positive | 0 (0.0) | 136 (9.9) | 0 (0.0) | 21 (8.6) | ||
ISUP source | <0.001 | |||||
Biopsy | 701 (95.1) | 808 (59.1) | 307 (98.4) | 137 (55.9) | ||
TURP | 36 (48.9) | 54 (3.9) | 5 (1.6) | 9 (3.7) | ||
RP | 0 (0.0) | 506 (37.0) | 0 (0.0) | 99 (40.4) | ||
Per-patient PI-RADS category | <0.001 | |||||
PI-RADS 1–2 | 492 (66.8) | 112 (8.2) | 232 (74.3) | 17 (6.9) | ||
PI-RADS 3 | 194 (26.3) | 364 (26.6) | 67 (21.5) | 61 (24.9) | ||
PI-RADS 4 | 26 (3.5) | 263 (19.2) | 9 (2.9) | 43 (17.6) | ||
PI-RADS 5 | 25 (3.4) | 629 (46.0) | 4 (1.3) | 124 (50.6) | ||
Number of lesions | <0.001 | |||||
0 | 737 (100.0) | 0 (0.0) | 312 (100.0) | 0 (0.0) | ||
1 | 0 (0.0) | 596 (43.6) | 0 (0.0) | 140 (57.1) | ||
2 | 0 (0.0) | 340 (24.9) | 0 (0.0) | 47 (19.2) | ||
3 | 0 (0.0) | 168 (12.3) | 0 (0.0) | 23 (9.4) | ||
4 | 0 (0.0) | 98 (7.2) | 0 (0.0) | 35 (14.3) | ||
>4 | 0 (0.0) | 166 (12.1) | 0 (0.0) | 0 (0.0) | ||
Hospital | <0.001 | |||||
Hospital 1 | 641 (87.0) | 930 (68.0) | 263 (84.3) | 69 (28.2) | ||
Hospital 2 | 74 (10.0) | 229 (16.7) | 21 (6.7) | 67 (27.3) | ||
Hospital 3 | 9 (1.2) | 186 (13.6) | 28 (9.0) | 109 (44.5) | ||
Hospital 4 | 13 (1.8) | 23 (1.7) | 0 (0.0) | 0 (0.0) |
The quantitative variables are presented as the median (Q1, Q3) for the non-normalized data. The categorical variables are presented as n (%). tPSA, total prostate-specific antigen; ISUP, Pathological International Society of Urological Pathology; TURP, transurethral resection of the prostate; RP, radical prostatectomy; PI-RADS, Prostate Imaging Reporting and Data System.
Segmentation metrics and quantitative evaluation
In the test set of the model development data set, the model exhibited proficient performance in lesion segmentation, achieving a DSC value of 0.80 (0.61, 0.86). The DSC, JACRD, VS, HD, and AD values of the different data sets are displayed in Table 3. Further, Table 4 and Figure 2 present the results of the Bland-Altman analysis for the measured values of PCa foci, including the right-left (RL) diameter, anteroposterior (AP) diameter, superoinferior (SI) diameter, volume, and ADC value. The Bland-Altman analysis used radiologist-annotated results as the reference standard to assess the consistency of the AI measurements with these reference standards. These results demonstrated a high level of agreement in the predicted volumes, 3D diameters, and ADC values of the PCa lesions compared to the reference standard. The differences observed between the majority of the AI results and the reference standard were minimal and fell within the 95% limit of agreement.
Table 3
Parameters | Training (N=1,681) | Validation (N=212) | Test (N=212) | P value |
---|---|---|---|---|
DSC | 0.93 (0.91, 0.95) | 0.81 (0.70, 0.87) | 0.80 (0.61, 0.86) | <0.001 |
JACARD | 0.87 (0.83, 0.90) | 0.68 (0.53, 0.77) | 0.67 (0.44, 0.76) | <0.001 |
VS | 0.99 (0.98, 1.00) | 0.90 (0.80, 0.95) | 0.87 (0.73, 0.95) | <0.001 |
HD | 5.63 (2.50, 12.4) | 9.94 (6.76, 18.0) | 12.5 (7.91, 20.4) | <0.001 |
AD | 0.08 (0.05, 0.18) | 0.40 (0.18, 1.11) | 0.49 (0.23, 1.67) | <0.001 |
The quantitative variables are presented as the median (Q1, Q3) for the non-normalized data. DSC, dice similarity coefficient; JACARD, Jaccard Coefficient; VS, volumetric similarity; HD, Hausdorff distance; AD, average distance.
Table 4
Parameters | RL diameter | AP diameter | SI diameter | Volume | ADC value |
---|---|---|---|---|---|
Bias | –0.464 | –0.431 | –0.526 | –1.306 | –0.464 |
BiasUpperCI | –0.394 | –0.37 | –0.464 | –1.053 | –0.394 |
BiasLowerCI | –0.533 | –0.492 | –0.588 | –1.56 | –0.533 |
BiasStdDev | 1.63 | 1.425 | 1.453 | 5.926 | 1.63 |
BiasSEM | 0.036 | 0.031 | 0.032 | 0.129 | 0.036 |
LOA_SEM | 0.061 | 0.053 | 0.054 | 0.221 | 0.061 |
UpperLOA | 2.73 | 2.361 | 2.322 | 10.309 | 2.73 |
UpperLOA_upperCI | 2.85 | 2.465 | 2.428 | 10.742 | 2.85 |
UpperLOA_lowerCI | 2.611 | 2.257 | 2.216 | 9.876 | 2.611 |
LowerLOA | –3.657 | –3.223 | –3.374 | –12.922 | –3.657 |
LowerLOA_upperCI | –3.538 | –3.119 | –3.267 | –12.489 | –3.538 |
LowerLOA_lowerCI | –3.777 | –3.327 | –3.48 | –13.355 | –3.777 |
Regression.fixed.slope | 0.15 | 0.12 | 0.12 | –0.02 | 0.15 |
Regression.fixed.intercept | –0.83 | –0.71 | –0.82 | –1.1 | –0.83 |
RL, right-left; AP, anteroposterior; SI, superoinferior; ADC, apparent diffusion coefficient; CI, confidence interval; LOA, limits of agreement; SEM, standard error of mean.
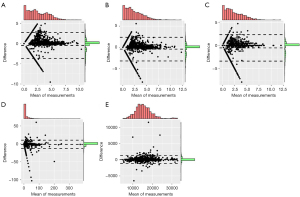
Lesion-level performance in the external validation data set
In total, 434 locations of PCa lesions were annotated by the radiologists and used as the reference standard for evaluating the AI model. Additionally, the AI model identified an additional 96 locations, resulting in a total of 530 locations for analysis. The AI model had a sensitivity of 0.654 and a positive predictive value of 0.747 at the lesion level. The AI-predicted results at the lesion level are depicted in line 3 of Figure 3. The AI model correctly identified 284 cancer foci in 231 patients, yielding a lesion-level sensitivity of 65.4%. The prediction results at the lesion level on the MR images are illustrated in Figure 4. The results of the FROC analysis of the lesion detection ability of the AI model are shown in Figure 5. By illustrating the trade-off between the sensitivity and FP results per patient, this curve visually presents the performance characteristics of the algorithm.
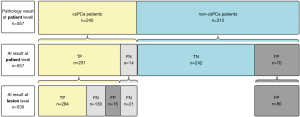
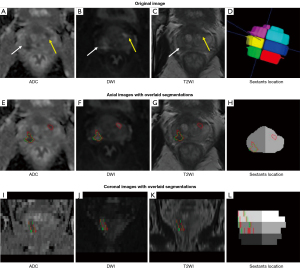
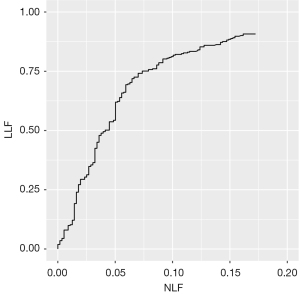
Sextant-level performance in the external validation data set
Of the 3,342 sextant areas evaluated in the 557 patients, the AI model diagnosed 745 TP, 136 FN, 2,175 TN, and 286 FP sextants. As a result, the overall sensitivity, specificity, and accuracy of the AI model at the sextant level were 0.846, 0.884, and 0.874, respectively (Table 5). In relation to each specific type of sextant (i.e., right-superior, right-middle, right-inferior, left-middle, and left-inferior), the sensitivity ranged from 0.772 to 0.902 (P=0.01), the specificity ranged from 0.851 to 0.915 (P=0.02), and the accuracy ranged from 0.858 to 0.894 (P=0.34) (Figure 6).
Table 5
Parameters | Patient level | Sextant level | Lesion level |
---|---|---|---|
Accuracy | 0.849 (0.849, 0.850) | 0.874 (0.874, 0.874) | – |
Sensitivity | 0.943 (0.914, 0.972) | 0.846 (0.822, 0.869) | 0.654 (0.608, 0.699) |
Specificity | 0.776 (0.729, 0.822) | 0.884 (0.871, 0.896) | * |
Positive predictive value | 0.767 (0.720, 0.815) | 0.723 (0.695, 0.750) | 0.747 (0.701, 0.790) |
Negative predictive value | 0.945 (0.917, 0.973) | 0.941 (0.932, 0.951) | * |
*, due to the absence of TN findings for lesion detection, calculating the specificity and negative predictive value was infeasible at the lesion level. The data presented in the table represent various metrics with their corresponding values and their respective 95% confidence intervals. TN, true negative.
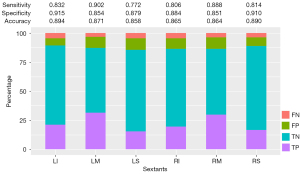
Patient-level performance in the external validation data set
At the patient level, the AI model correctly detected that 231 of 245 patients had csPCa, and 242 of 312 patients did not have csPCa (Figure 3). Thus, the sensitivity, specificity, and accuracy for the detection of csPCa patients were 0.943, 0.776, and 0.849, respectively (Table 5).
Table 6 and Figure 7 present the factors that influenced the accuracy of the AI model at the patient level. The AI model accurately predicted the presence of csPCa in a higher proportion of csPCa patients (231/245, 0.943) than non-csPCa patients (242/312, 0.776) (P<0.001) (Figure 7A). The lesion number and tumor volume (24) were greater in the correctly diagnosed patients than the incorrectly diagnosed patients [0 (0, 1) vs. 0 (0, 0); 0.00 (0.00, 0.00) vs. 0.00 (0.00, 2.36) cm3, both P<0.001] (Figure 7B,7C). Additionally, among the positive patients, those with lower average ADC values were more accurately diagnosed than those with higher average ADC values [0.750 (0.643, 0.866) ×10−3 mm2/s vs. 0.884 (0.765, 0.966)×10−3 mm2/s, P=0.011] (Figure 7D). The statistical analysis indicated that with the exception of the hospital variable (P=0.04), there were no significant differences in the accuracy of the AI model among the different magnetic fields, vendors of MR scanners, or PCa locations (all P>0.05).
Table 6
Parameters | Overall, 557 (100.0) | Error (n=84) | Right (n=473) | P value |
---|---|---|---|---|
Patient diagnosis (%) | <0.001 | |||
Negative | 312 (56.0) | 70 (83.3) | 242 (51.2) | |
Positive | 245 (44.0) | 14 (16.7) | 231 (48.8) | |
Lesion number | 0.00 (0.00, 1.00) | 0.00 (0.00, 0.00) | 0.00 (0.00, 1.00) | <0.001 |
Tumor volume (cm3) | 0.00 (0.00, 1.87) | 0.00 (0.00, 0.00) | 0.00 (0.00, 23.55) | <0.001 |
Average ADC value (10–3 mm2/s) | 0.753 (0.643, 0.875) | 0.884 (0.765, 0.966) | 0.750 (0.643, 0.866) | 0.011 |
Hospital | 0.042 | |||
Hospital 1 | 332 (59.6) | 52 (61.9) | 280 (59.2) | |
Hospital 2 | 88 (15.8) | 19 (22.6) | 69 (14.6) | |
Hospital 3 | 137 (24.6) | 13 (15.5) | 124 (26.2) | |
Magnetic field | 0.999 | |||
1.5 T | 27 (4.8) | 4 (4.8) | 23 (4.9) | |
3.0 T | 530 (95.2) | 80 (95.2) | 450 (95.1) | |
Vendor | 0.364 | |||
GE | 288 (51.7) | 50 (59.5) | 238 (50.3) | |
PHILIPS | 62 (11.1) | 8 (9.5) | 54 (11.4) | |
SIEMENS | 201 (36.1) | 26 (31.0) | 175 (37.0) | |
UIH | 6 (1.1) | 0 (0.0) | 6 (1.3) | |
PCa location | 0.512 | |||
PZ | 32 (5.7) | 3 (21.4) | 29 (12.6) | |
TZ | 188 (33.8) | 2 (14.3) | 23 (10.0) | |
PZ + TZ | 25 (4.5) | 9 (64.3) | 179 (77.4) |
The quantitative variables are presented as the median (Q1, Q3) for the non-normalized data. The categorical variables are presented as n (%). AI, artificial intelligence; ADC, apparent diffusion coefficient; PCa, prostate cancer; PZ, peripheral zone; TZ, transition zone.
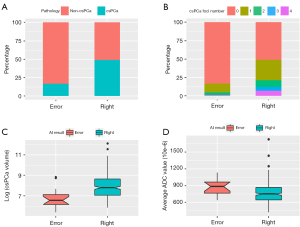
Discussion
Before any AI model can be used to assist radiologists to manage the increase in imaging volumes, it must undergo external evaluation. Thus, this study sought to demonstrate that an AI model could accurately detect and localize suspicious findings on prostate MRI. A diverse multicenter data set was employed for the external validation of our PCa AI model. The correlation between model efficacy and disease characteristics was investigated to promote the transparent validation and facilitate the clinical translation of our findings.
The meta-analysis (25) showed that the model had a pooled sensitivity of 0.89 and a specificity of 0.73 in diagnosing patients with csPCa using PI-RADS Version 2. In relation to sextant location, a previous study reported that radiologists had a sensitivity of 0.55 to 0.67 and a specificity of 0.68 to 0.80 (26). In our study, the sensitivity and specificity of the AI model were 0.943 and 0.776 at the patient level, and 0.846 and 0.884 at the sextant level, respectively. It is crucial to compare the results of the AI model with those of the radiologists. The AI model provides a binary classification of positive and negative results. Radiologists use the PI-RADS scoring system to assess the likelihood of PCa during image interpretation. Since the PI-RADS scores provided by radiologists are subjective evaluations, there may be inconsistencies between different observers and even within the same observer. We have previously conducted research on this very issue, comparing the diagnostic accuracy, diagnostic confidence, and diagnostic time of radiologists when using AI versus not using AI. As the results of this previous study have already been published (18), we elected not to include this content in the current manuscript to avoid duplicate publication. It is crucial to note that our AI model performed well at both the patient and sextant levels, effectively fulfilling the two main objectives of identifying biopsy candidates and providing accurate guidance for targeted biopsies. Our AI model had an average sensitivity of 94.3% in detecting index lesions but only 65.4% in detecting all lesions; thus, the lesion-level sensitivity of the model needs to be further enhanced. The use of PIRADS V2 enabled radiologists to achieve a sensitivity of 91% in identifying index PCa lesions and a sensitivity of 63% in detecting all lesions (27). The sensitivity of the AI model at the lesion level is comparatively lower; however, it remains consistent with the diagnostic performance currently achieved by medical professionals using PIRADS V2. The per-sextant sensitivity was much higher than the per-lesion sensitivity. The observed difference between the per-sextant sensitivity and per-lesion sensitivity can be attributed to the inherent complexity of lesion detection and the manner in which lesions are distributed within the sextants. The lower per-lesion sensitivity arises from the potential occurrence of missed diagnoses at the lesion level, which led to a decrease in the model’s overall sensitivity for detecting individual lesions. Lesions with larger volumes tend to have a lower rate of missed diagnosis and higher detection accuracy, as their size facilitates easier identification. Consequently, larger lesions often span multiple sextants, resulting in the correct diagnosis of more sextants.
Recently, numerous studies have presented compelling evidence in this domain (28). The commercially available software provided at https://fuse-ai.de/prostatecarcinoma-ai has a comparable level of reliability to that of radiologists in detecting carcinomas, with a patient-level sensitivity of 0.86, which, in contrast, is inferior to our model’s sensitivity value of 0.94. Several researchers (18,26,29) have sought to evaluate the efficacy of the U-Net network for PCa detection at various levels, including the lesion, sextant, and patient levels. However, these studies did not examine the effect of different cancer foci on the diagnostic efficiency of the models. Zhong et al. (30) employed a training set (comprising data from 110 prostate patients) and a test set (comprising data from 30 patients) to assess the effectiveness of their model, and found that the transfer learning-based model exhibited superior efficacy in diagnosing PCa than the deep-learning model without transfer learning. However, it should be noted that their analysis was limited to the lesion level. Further, Cao et al. (31) trained a FocalNet model to autonomously identify prostate lesions. Their model achieved a confidence score that yielded outcomes on par with those achieved by experienced radiologists but was specifically limited to high-sensitivity or high-specificity scenarios. These models were trained and validated on private data sets, which are often homogeneous and lack external validation with data from other institutions and scanners produced by different manufacturers. Table S8 provides an overview of the key differences between our study and recent previous studies using deep learning in prostate MRI.
As we enter a new phase in the application of AI to prostate mpMRI, our goal is to prioritize transparent validation and clinical translation. Previous studies have primarily focused on reporting the performance metrics of AI models, such as sensitivity, specificity, and areas under the curve, but have often failed to offer interpretations of these values. Such interpretations are crucial in enhancing radiologists’ confidence in the results and facilitating the clinical implementation of AI models. In our research, we examined the effects of inherent characteristics of PCa on the performance of the AI model. In our analysis, we observed a substantial discrepancy in the AI-predicted accuracy between patients with csPCa and those without csPCa. Specifically, our model’s accuracy for csPCa patients was significantly higher than that for non-csPCa patients. Thus, the AI model’s enhanced ability to detect csPCa could be leveraged to mitigate FN results and unnecessary biopsies. However, it is important to emphasize that further validation at a higher level is required to establish the association between the AI models’ diagnostic results and their clinical value. Thus, future studies with prospective cohorts of patients with long-term follow-up periods need to be conducted to validate these results. Other findings indicate that patients with a greater number of lesions, a larger tumor volume, and lower average ADC values tend to receive a more accurate diagnosis from the AI model. These trends align with the performance of radiologists. Using the PI-RADS Version 2 system, radiologists detected PCa in 12–33%, 22–70%, and 72–91% of lesions with PI-RADS scores of 3, 4, and 5, respectively (32,33).
Recent studies have shown the favorable performance of architectures that integrate two cascaded networks: a first model that segments or performs a crop around the prostate area, and a second binary model that segments the PCa lesion. In the study conducted by Yang et al. (34), convolutional neural networks (CNNs) were employed to crop square regions encompassing the entirety of the prostate area. Subsequently, their proposed co-trained CNNs were fed with pairs of aligned ADC and T2WI squares. Expanding on this work, Wang et al. (35) and Zhu et al. (29) adapted the workflow to create an end-to-end trainable deep neural network comprising two sub-networks. The first sub-network was responsible for prostate detection and ADC-T2WI registration, while the second sub-network was a dual-path multimodal CNN that generated a classification score for csPCa and non-csPCa. In the study conducted by Saha et al. (36), a preparatory network called anisotropic 3D U-Net was employed to generate deterministic or probabilistic zonal segmentation maps. These maps were subsequently fused in a second CNN that produced a probability map for csPCa. Despite the additional weights and more intensive training process introduced by the two-step workflow, it has proven to be an effective method. Certain attention mechanisms have demonstrated improved segmentation performance in prostate imaging tasks. Rundo et al. (37) employed a “squeeze & excitation” (SE) module for prostate zonal segmentation on diverse MRI data sets, and reported a 1.4–2.9% increase in the DSC compared to the U-Net baseline, specifically for peripheral zone segmentation, when evaluating their multi-source model across different data sets. Zhang et al. (38) introduced combined channel-attention (inspired by the SE module) and position-attention layers, and achieved a DSC that surpassed their baseline by 1.8% for prostate lesion segmentation on T2WI. Additionally, Saha et al. (36) improved the sensitivity by 4.34% by incorporating the aforementioned SE model and grid-attention gates mechanisms into a 3D UNet++ (39) backbone architecture for binary PCa segmentation.
At the lesion level, 530 lesions were analyzed, of which 53.6% (284/530) lesions were correctly diagnosed (TP lesions) by the AI model, 28.3% (150/530) lesions were missed (FN lesions), and 18.1% (96/530) additional lesions were over detected (FP lesions). Despite some misdiagnosed lesions, the overall accuracy of the AI model at the lesion level was similar to the results of the imaging interpretation by radiologists. In previous studies, the FP rates of radiologists for lesions with PI-RADS scores ≥3 have varied from 32% to 50% (40). Additionally, the FN rate of radiologists may reach 12% for high-grade cancers during screening, and may be as high as 34% in men undergoing radical prostatectomy (1,41). There might be other reasons for the high prevalence of FN and FP lesions in our study. In terms of the FN lesions, our study methodology may have falsely increased the FN rate. One limitation of our study is that when multiple lesions were detected by the AI model in a case, only the four largest lesions detected by the AI model were studied. Because PI-RADS recommends reporting no more than four lesions in structured reporting, we followed this rule to simulate a real clinical scenario. Thus, if AI detects more than four TP lesions in a patient, the extra lesions would be considered FN lesions. In terms of the FP lesions, the imperfect match between the reference standard annotation and the real pathology might have increased the FP rate. In this study, the diagnoses of 79.7% of the patients were pathologically proven by image-guided biopsy. In comparison to radical prostatectomy specimens, image-guided biopsy pathology results may miss some lesions (16). When radiologists outline the reference area of csPCa foci on MR images according to the biopsy pathology results, they may miss lesions or underestimate the extent of the lesions (42). Conversely, if the missed lesions were detected by the AI model and considered FPs, the lesions may be correctly detected.
In addition to the above-mentioned methodological limitations, our study had a number of other limitations. First, the retrospective study design and unbalanced data prevented a robust assessment of the clinical impact of the model. Ideally, AI models should be deployed in prospective randomized studies to test their performance. Second, if there were mismatches between the MR images and the pathology data, the data were not analyzed. The reference standard was based on image-guided biopsy, and the result obtained by using whole-mount step section pathology as the reference standard was more credible than image-guided biopsy pathology. Third, poor image quality data were excluded; however, we intend to address this in future clinical applications. Finally, there is a lack of research on radiologists interacting with AI models. In the future, we plan to invite radiologists with varying levels of experience to interpret mpMRI reports with the assistance of AI models to determine whether AI models add value in real clinical settings.
Conclusions
In the external validation, the AI model achieved acceptable accuracy for the detection and localization of csPCa at the patient level and the sextant level. However, the sensitivity at the lesion level should be improved for future clinical application.
Acknowledgments
Funding: This work was supported by
Footnote
Reporting Checklist: The authors have completed the TRIPOD reporting checklist. Available at https://qims.amegroups.com/article/view/10.21037/qims-23-791/rc
Conflicts of Interest: All authors have completed the ICMJE uniform disclosure form (available at https://qims.amegroups.com/article/view/10.21037/qims-23-791/coif). P.W. and Xiangpeng Wang were employees of Beijing Smart Tree Medical Technology Co., Ltd., and had no financial or other conflicts with respect to this study. The other authors have no conflicts of interest to declare.
Ethical Statement:
Open Access Statement: This is an Open Access article distributed in accordance with the Creative Commons Attribution-NonCommercial-NoDerivs 4.0 International License (CC BY-NC-ND 4.0), which permits the non-commercial replication and distribution of the article with the strict proviso that no changes or edits are made and the original work is properly cited (including links to both the formal publication through the relevant DOI and the license). See: https://creativecommons.org/licenses/by-nc-nd/4.0/.
References
- Ahmed HU, El-Shater Bosaily A, Brown LC, Gabe R, Kaplan R, Parmar MK, Collaco-Moraes Y, Ward K, Hindley RG, Freeman A, Kirkham AP, Oldroyd R, Parker C, Emberton MPROMIS study group. Diagnostic accuracy of multi-parametric MRI and TRUS biopsy in prostate cancer (PROMIS): a paired validating confirmatory study. Lancet 2017;389:815-22. [Crossref] [PubMed]
- Ahdoot M, Wilbur AR, Reese SE, Lebastchi AH, Mehralivand S, Gomella PT, Bloom J, Gurram S, Siddiqui M, Pinsky P, Parnes H, Linehan WM, Merino M, Choyke PL, Shih JH, Turkbey B, Wood BJ, Pinto PA. MRI-Targeted, Systematic, and Combined Biopsy for Prostate Cancer Diagnosis. N Engl J Med 2020;382:917-28. [Crossref] [PubMed]
- Kasivisvanathan V, Rannikko AS, Borghi M, Panebianco V, Mynderse LA, Vaarala MH, et al. MRI-Targeted or Standard Biopsy for Prostate-Cancer Diagnosis. N Engl J Med 2018;378:1767-77. [Crossref] [PubMed]
- van der Leest M, Cornel E, Israël B, Hendriks R, Padhani AR, Hoogenboom M, Zamecnik P, Bakker D, Setiasti AY, Veltman J, van den Hout H, van der Lelij H, van Oort I, Klaver S, Debruyne F, Sedelaar M, Hannink G, Rovers M, Hulsbergen-van de Kaa C, Barentsz JO. Head-to-head Comparison of Transrectal Ultrasound-guided Prostate Biopsy Versus Multiparametric Prostate Resonance Imaging with Subsequent Magnetic Resonance-guided Biopsy in Biopsy-naïve Men with Elevated Prostate-specific Antigen: A Large Prospective Multicenter Clinical Study. Eur Urol 2019;75:570-8. [Crossref] [PubMed]
- Barentsz JO, Richenberg J, Clements R, Choyke P, Verma S, Villeirs G, Rouviere O, Logager V, Fütterer JJEuropean Society of Urogenital Radiology. ESUR prostate MR guidelines 2012. Eur Radiol 2012;22:746-57. [Crossref] [PubMed]
- Vargas HA, Hötker AM, Goldman DA, Moskowitz CS, Gondo T, Matsumoto K, Ehdaie B, Woo S, Fine SW, Reuter VE, Sala E, Hricak H. Updated prostate imaging reporting and data system (PIRADS v2) recommendations for the detection of clinically significant prostate cancer using multiparametric MRI: critical evaluation using whole-mount pathology as standard of reference. Eur Radiol 2016;26:1606-12. [Crossref] [PubMed]
- Weinreb JC, Barentsz JO, Choyke PL, Cornud F, Haider MA, Macura KJ, Margolis D, Schnall MD, Shtern F, Tempany CM, Thoeny HC, Verma S. PI-RADS Prostate Imaging - Reporting and Data System: 2015, Version 2. Eur Urol 2016;69:16-40. [Crossref] [PubMed]
- Turkbey B, Rosenkrantz AB, Haider MA, Padhani AR, Villeirs G, Macura KJ, Tempany CM, Choyke PL, Cornud F, Margolis DJ, Thoeny HC, Verma S, Barentsz J, Weinreb JC. Prostate Imaging Reporting and Data System Version 2.1: 2019 Update of Prostate Imaging Reporting and Data System Version 2. Eur Urol 2019;76:340-51. [Crossref] [PubMed]
- Smith CP, Harmon SA, Barrett T, Bittencourt LK, Law YM, Shebel H, An JY, Czarniecki M, Mehralivand S, Coskun M, Wood BJ, Pinto PA, Shih JH, Choyke PL, Turkbey B. Intra- and interreader reproducibility of PI-RADSv2: A multireader study. J Magn Reson Imaging 2019;49:1694-703. [Crossref] [PubMed]
- Girometti R, Giannarini G, Greco F, Isola M, Cereser L, Como G, Sioletic S, Pizzolitto S, Crestani A, Ficarra V, Zuiani C. Interreader agreement of PI-RADS v. 2 in assessing prostate cancer with multiparametric MRI: A study using whole-mount histology as the standard of reference. J Magn Reson Imaging 2019;49:546-55. [Crossref] [PubMed]
- Sonn GA, Fan RE, Ghanouni P, Wang NN, Brooks JD, Loening AM, Daniel BL, To'o KJ, Thong AE, Leppert JT. Prostate Magnetic Resonance Imaging Interpretation Varies Substantially Across Radiologists. Eur Urol Focus 2019;5:592-9. [Crossref] [PubMed]
- Stabile A, Giganti F, Kasivisvanathan V, Giannarini G, Moore CM, Padhani AR, Panebianco V, Rosenkrantz AB, Salomon G, Turkbey B, Villeirs G, Barentsz JO. Factors Influencing Variability in the Performance of Multiparametric Magnetic Resonance Imaging in Detecting Clinically Significant Prostate Cancer: A Systematic Literature Review. Eur Urol Oncol 2020;3:145-67. [Crossref] [PubMed]
- Ruprecht O, Weisser P, Bodelle B, Ackermann H, Vogl TJ. MRI of the prostate: interobserver agreement compared with histopathologic outcome after radical prostatectomy. Eur J Radiol 2012;81:456-60. [Crossref] [PubMed]
- Wildeboer RR, van Sloun RJG, Wijkstra H, Mischi M. Artificial intelligence in multiparametric prostate cancer imaging with focus on deep-learning methods. Comput Methods Programs Biomed 2020;189:105316. [Crossref] [PubMed]
- Suarez-Ibarrola R, Sigle A, Eklund M, Eberli D, Miernik A, Benndorf M, Bamberg F, Gratzke C. Artificial Intelligence in Magnetic Resonance Imaging-based Prostate Cancer Diagnosis: Where Do We Stand in 2021? Eur Urol Focus 2022;8:409-17. [Crossref] [PubMed]
- Radtke JP, Schwab C, Wolf MB, Freitag MT, Alt CD, Kesch C, Popeneciu IV, Huettenbrink C, Gasch C, Klein T, Bonekamp D, Duensing S, Roth W, Schueler S, Stock C, Schlemmer HP, Roethke M, Hohenfellner M, Hadaschik BA. Multiparametric Magnetic Resonance Imaging (MRI) and MRI-Transrectal Ultrasound Fusion Biopsy for Index Tumor Detection: Correlation with Radical Prostatectomy Specimen. Eur Urol 2016;70:846-53. [Crossref] [PubMed]
- Zhu Y, Wei R, Gao G, Ding L, Zhang X, Wang X, Zhang J. Fully automatic segmentation on prostate MR images based on cascaded fully convolution network. J Magn Reson Imaging 2019;49:1149-56. [Crossref] [PubMed]
- Sun Z, Wu P, Cui Y, Liu X, Wang K, Gao G, Wang H, Zhang X, Wang X. Deep-Learning Models for Detection and Localization of Visible Clinically Significant Prostate Cancer on Multi-Parametric MRI. J Magn Reson Imaging 2023;58:1067-81. [Crossref] [PubMed]
- Zhu L, Gao G, Liu Y, Han C, Liu J, Zhang X, Wang X. Feasibility of integrating computer-aided diagnosis with structured reports of prostate multiparametric MRI. Clin Imaging 2020;60:123-30. [Crossref] [PubMed]
- Çiçek Ö, Abdulkadir A, Lienkamp SS, Brox T, Ronneberger O. editors. 3D U-Net: Learning Dense Volumetric Segmentation from Sparse Annotation. Medical Image Computing and Computer-Assisted Intervention – MICCAI 2016. Cham: Springer International Publishing; 2016.
- Padhani AR, Turkbey B. Detecting Prostate Cancer with Deep Learning for MRI: A Small Step Forward. Radiology 2019;293:618-9. [Crossref] [PubMed]
- Gaur S, Lay N, Harmon SA, Doddakashi S, Mehralivand S, Argun B, et al. Can computer-aided diagnosis assist in the identification of prostate cancer on prostate MRI? a multi-center, multi-reader investigation. Oncotarget 2018;9:33804-17. [Crossref] [PubMed]
- Giannini V, Mazzetti S, Armando E, Carabalona S, Russo F, Giacobbe A, Muto G, Regge D. Multiparametric magnetic resonance imaging of the prostate with computer-aided detection: experienced observer performance study. Eur Radiol 2017;27:4200-8. [Crossref] [PubMed]
- Mayer R, Simone CB 2nd, Turkbey B, Choyke P. Algorithms applied to spatially registered multi-parametric MRI for prostate tumor volume measurement. Quant Imaging Med Surg 2021;11:119-32. [Crossref] [PubMed]
- Woo S, Suh CH, Kim SY, Cho JY, Kim SH. Diagnostic Performance of Prostate Imaging Reporting and Data System Version 2 for Detection of Prostate Cancer: A Systematic Review and Diagnostic Meta-analysis. Eur Urol 2017;72:177-88. [Crossref] [PubMed]
- Schelb P, Kohl S, Radtke JP, Wiesenfarth M, Kickingereder P, Bickelhaupt S, Kuder TA, Stenzinger A, Hohenfellner M, Schlemmer HP, Maier-Hein KH, Bonekamp D. Classification of Cancer at Prostate MRI: Deep Learning versus Clinical PI-RADS Assessment. Radiology 2019;293:607-17. [Crossref] [PubMed]
- Greer MD, Brown AM, Shih JH, Summers RM, Marko J, Law YM, Sankineni S, George AK, Merino MJ, Pinto PA, Choyke PL, Turkbey B. Accuracy and agreement of PIRADSv2 for prostate cancer mpMRI: A multireader study. J Magn Reson Imaging 2017;45:579-85. [Crossref] [PubMed]
- Wang Y, Wang W, Yi N, Jiang L, Yin X, Zhou W, Wang L. Detection of intermediate- and high-risk prostate cancer with biparametric magnetic resonance imaging: a systematic review and meta-analysis. Quant Imaging Med Surg 2023;13:2791-806. [Crossref] [PubMed]
- Zhu L, Gao G, Zhu Y, Han C, Liu X, Li D, Liu W, Wang X, Zhang J, Zhang X, Wang X. Fully automated detection and localization of clinically significant prostate cancer on MR images using a cascaded convolutional neural network. Front Oncol 2022;12:958065. [Crossref] [PubMed]
- Zhong X, Cao R, Shakeri S, Scalzo F, Lee Y, Enzmann DR, Wu HH, Raman SS, Sung K. Deep transfer learning-based prostate cancer classification using 3 Tesla multi-parametric MRI. Abdom Radiol (NY) 2019;44:2030-9. [Crossref] [PubMed]
- Cao R, Zhong X, Afshari S, Felker E, Suvannarerg V, Tubtawee T, Vangala S, Scalzo F, Raman S, Sung K. Performance of Deep Learning and Genitourinary Radiologists in Detection of Prostate Cancer Using 3-T Multiparametric Magnetic Resonance Imaging. J Magn Reson Imaging 2021;54:474-83. [Crossref] [PubMed]
- Mehralivand S, Bednarova S, Shih JH, Mertan FV, Gaur S, Merino MJ, Wood BJ, Pinto PA, Choyke PL, Turkbey B. Prospective Evaluation of PI-RADS™ Version 2 Using the International Society of Urological Pathology Prostate Cancer Grade Group System. J Urol 2017;198:583-90. [Crossref] [PubMed]
- Greer MD, Shih JH, Lay N, Barrett T, Kayat Bittencourt L, Borofsky S, Kabakus IM, Law YM, Marko J, Shebel H, Mertan FV, Merino MJ, Wood BJ, Pinto PA, Summers RM, Choyke PL, Turkbey B. Validation of the Dominant Sequence Paradigm and Role of Dynamic Contrast-enhanced Imaging in PI-RADS Version 2. Radiology 2017;285:859-69. [Crossref] [PubMed]
- Yang X, Liu C, Wang Z, Yang J, Min HL, Wang L, Cheng KT. Co-trained convolutional neural networks for automated detection of prostate cancer in multi-parametric MRI. Med Image Anal 2017;42:212-27. [Crossref] [PubMed]
- Wang Z, Liu C, Cheng D, Wang L, Yang X, Cheng KT. Automated Detection of Clinically Significant Prostate Cancer in mp-MRI Images Based on an End-to-End Deep Neural Network. IEEE Trans Med Imaging 2018;37:1127-39. [Crossref] [PubMed]
- Saha A, Hosseinzadeh M, Huisman H. End-to-end prostate cancer detection in bpMRI via 3D CNNs: Effects of attention mechanisms, clinical priori and decoupled false positive reduction. Med Image Anal 2021;73:102155. [Crossref] [PubMed]
- Rundo L, Han C, Nagano Y, Zhang J, Hataya R, Militello C, Tangherloni A, Nobile MS, Ferretti C, Besozzi D. USE-Net: Incorporating Squeeze-and-Excitation blocks into U-Net for prostate zonal segmentation of multi-institutional MRI data sets. Neurocomputing 2019;365:31-43.
- Zhang G, Wang W, Yang D, Luo J, He P, Wang Y, Luo Y, Zhao B, Lu J. A bi-attention adversarial network for prostate cancer segmentation. IEEE Access 2019;7:131448-58.
- Zhou Z, Siddiquee MMR, Tajbakhsh N, Liang J. UNet++: Redesigning Skip Connections to Exploit Multiscale Features in Image Segmentation. IEEE Trans Med Imaging 2020;39:1856-67. [Crossref] [PubMed]
- Stolk TT, de Jong IJ, Kwee TC, Luiting HB, Mahesh SVK, Doornweerd BHJ, Willemse PM, Yakar D. False positives in PIRADS (V2) 3, 4, and 5 lesions: relationship with reader experience and zonal location. Abdom Radiol (NY) 2019;44:1044-51. [Crossref] [PubMed]
- Johnson DC, Raman SS, Mirak SA, Kwan L, Bajgiran AM, Hsu W, Maehara CK, Ahuja P, Faiena I, Pooli A, Salmasi A, Sisk A, Felker ER, Lu DSK, Reiter RE. Detection of Individual Prostate Cancer Foci via Multiparametric Magnetic Resonance Imaging. Eur Urol 2019;75:712-20. [Crossref] [PubMed]
- Priester A, Natarajan S, Khoshnoodi P, Margolis DJ, Raman SS, Reiter RE, Huang J, Grundfest W, Marks LS. Magnetic Resonance Imaging Underestimation of Prostate Cancer Geometry: Use of Patient Specific Molds to Correlate Images with Whole Mount Pathology. J Urol 2017;197:320-6. [Crossref] [PubMed]