Validation of an established TW3 artificial intelligence bone age assessment system: a prospective, multicenter, confirmatory study
Introduction
The short staffing of pediatricians has long been a significant issue in China’s medical community. The “White Paper of Pediatric Resources in China” reveals a current manpower shortage of over 200,000 pediatricians (1). A study conducted from 2015 to 2016, covering Chinese pediatricians in 54,214 hospitals across all 31 provinces of mainland China, unveiled an uneven distribution of skilled pediatricians (2). Pediatric diagnosis and treatment resources are concentrated in children’s specialized hospitals and in the pediatrics departments of some comprehensive tertiary hospitals, showing that pediatrics is relatively weak at the grassroots level (3). Pediatricians, on average, had a low level of education, with approximately 32% having completed only 3 years of junior college training after high school (1). One area where this shortage is particularly noticeable is in the field of bone age assessment (BAA). BAA is a time-consuming process that requires the expertise of experienced pediatric endocrinologists or radiologists to yield reliable results. It is closely linked to factors such as height velocity, menarche, and muscle mass, rather than chronological age. BAA plays a vital role in pediatric radiology and is a critical factor in assessing children’s growth and development (4). The accurate assessment of bone age is crucial in diagnosing and treating endocrinological and growth disorders in children. Proper radiographic assessment of bone age is of utmost importance when making decisions regarding the type and timing of operative interventions in pediatric orthopedics. A consistent assessment, free from artificial errors, is essential for accurately evaluating the progression of certain diseases or the effectiveness of specific treatments.
Traditionally, trained radiologists or pediatricians visually examine X-rays of the hand and wrist to estimate the age of a child’s bones. The estimation relies on the predictable changes in ossification centers over time (5). Standardized methods for assessing skeleton maturity include Tanner-Whitehouse’s third edition (TW3) (6,7), last updated in 2001. TW3 computes scores for radius, ulna, and short bones (RUS), along with the carpal series bones, where each major bone in the hand contributes to the total score. However, this process can be time-consuming and susceptible to inter-observer variability, potentially resulting in inconsistencies in diagnosis and treatment planning (8).
Artificial intelligence (AI) improved by convolutional neural network (CNN) has the potential to play a pivotal role in enhancing BAA in China (9,10). By harnessing deep learning algorithms and computer vision techniques, AI systems can rapidly and accurately analyze X-ray images of pediatric patients, providing estimates of their bone age (11,12). This not only saves time and money but also reduces the risk of errors and variability typically associated with manual assessment. Moreover, the availability of extensive datasets containing BAA, including those from diverse populations, can be leveraged to train AI systems. This, in turn, can help address the problem of the uneven distribution of skilled pediatricians across China (13). By decreasing the chances of misdiagnosis, AI has the potential to curtail the necessity for unnecessary testing and interventions.
Previously, we developed an AI-BA system using a deep learning algorithm based on a dataset of 9,059 clinical radiographs of the left hand collected between January 2012 and December 2016 (14). The TW3-AI model demonstrated high consistency with the reviewers’ overall estimates, achieving a root mean square error (RMSE) of 0.5 years in the analysis. However, there are some shortcomings in our previous study that need addressing. The system, upon which our study is based, lacks a gold standard for comparison. Consequently, evaluating the system’s performance has proven to be challenging, and reducing variations among reviewers and assessing its accuracy and efficacy in comparison have been difficult. Moreover, the previous system had not undergone comprehensive evaluation in various working environments. This limitation stems from the fact that the dataset used to train and validate the system originated from a single center. This could potentially impact its generalizability and real-world applicability. In this article, we aimed to carry out prospective multicenter research with the introduction of gold standards to compare the accuracy and consistency in TW3-AI and reviewers, and finally to prove the feasibility of the BAA system in hospitals of different areas.
Therefore, in this subsequent multicenter study involving nine different medical centers, we conducted this prospective study and consecutively recruited 973 patients between August and December 2020. We utilized mean absolute error (MAE) and RMSE to measure the applicability of TW3-AI system, and accuracy to showcase the superiority of TW3-AI. Additionally, we employed the Kappa test and Bland-Altman (B-A) plot between AI and reviewers to establish robust evidence regarding the effectiveness and generalizability of the TW3-AI system in real-world clinical settings. By evaluating bone age radiographs with both AI model and professional reviewers across nine hospitals, our aim is to demonstrate that AI’s capabilities are comparable to human expertise and hold significant potential as a driving force for future medical advancements. We present this article in accordance with the STARD reporting checklist (available at https://qims.amegroups.com/article/view/10.21037/qims-23-715/rc).
Methods
Sample size estimation
The determination of the sample size is based on research hypotheses and estimates of software measurement accuracy, calculated according to statistical principles. In this study, we set the equivalence threshold for the software measurement results of the experimental group compared to the gold standard at ±1.0 years, with a two-sided significance level of 0.05 and a power of 90%. In the sample size calculation process, we conservatively estimated the difference between the experimental group’s measurement results and the gold standard to be ±0.8 years, with a standard deviation of 1. Using the PASS16 software, we computed the required sample size as 216 cases.
The age range of bone age radiographs intended for inclusion in this study spans from 1 to 18 years. We categorized these radiographs into three age groups based on their real ages: 1–6, 7–12, and 13–18 years. To meet the statistical analysis requirements for each age group, a minimum of 216 participants is needed in each category. Furthermore, considering the practical application of children’s BAA, we expanded the overall sample size to 990 cases. This expansion ensures a comprehensive representation of bone age radiographs across all age groups and facilitates subgroup analyses for different age ranges. Initially, a total of 973 radiographs were enrolled in the study, but 229 (23.5%) radiographs were subsequently excluded due to a lack of essential physiological information and metadata.
Data collection
In this study, we recruited a total of nine hospitals based on specific criteria, including geographical diversity, expertise in the field, and a substantial caseload. The study was conducted in accordance with the Declaration of Helsinki (as revised in 2013). Ethical approval was granted by the Children’s Hospital of Zhejiang University School of Medicine and is on file at all the participating centers. All participating hospitals were informed and agreed with the study. Informed consent was taken from all the patients. And for patients under 18, informed consent was obtained from the patients’ legal guardians. The substantial caseload managed by each hospital is displayed in Table 1, with a total of 973 radiographs enrolled in the study. The radiographs included in the study were selected from the patients who heading to the endocrinology and were all consecutive case series who met the inclusion criteria. The inclusion criteria comprised children and young adults aged 1 to 20 years who underwent a physical examination between August and December 2020. Collected information included radiographs, sex, chronological age, manufacturer of the imaging instrument, and clinical diagnosis. The ethnicity of the population was not collected or assessed in our study. Exclusion criteria included clinical radiographic evidence of fracture or surgeries around the palms and wrists, as well as poor image quality, such as inappropriately positioned palms and images containing splints. Exclusion criteria also included data missing on physical information. We conducted a thorough evaluation of the radiographs and excluded 229 (23.5%) of them from the analysis due to the lack of physiological information and metadata. Finally, 744 left-hand radiographs were filtrated from nine different children’s hospitals between August and December 2020, comprising 378 boys and 366 girls. The distribution of subjects is depicted in Figure 1. The number of patients over 7 years old seeking BAA increased due to the emphasis on upcoming puberty. All images were anonymized and saved in Digital Imaging and Communications in Medicine (DICOM) format, and subsequently stored in the Picture Archiving and Communication Systems (PACS). The full flow diagram is shown in Figure 2.
Table 1
Name of each hospital | Numbers of provided radiographs |
---|---|
Children’s Hospital of Zhejiang University School of Medicine | 200 |
Children’s Hospital of Fudan University | 50 |
The First Affiliated Hospital of Sun Yat-sen University | 123 |
Xi’an Children’s Hospital Affiliated to Xi’an Jiaotong University | 200 |
Tianjin Medical University General Hospital | 89 |
Children’s Hospital of Chongqing Medical University | 150 |
Shenzhen Children’s Hospital | 105 |
The Second Affiliated Hospital of Nanchang University | 25 |
Tongji Hospital of Tongji Medical College of Huazhong University of Science and Technology | 31 |
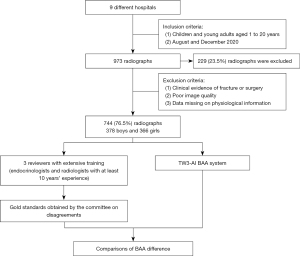
Scanning model
The radiograph was taken with the participant in a seated position, with their left hand (non-dominant hand) placed flat using the standard hand scanning mode. If the non-dominant hand was injured or unavailable for assessment, the use of the dominant hand was permitted as a suitable alternative. The X-ray beam was focused perpendicular to the third metacarpal head, at a focal distance of 85 cm. The left hand was positioned with the palm facing down, close to the dark box. The middle finger axis was aligned in a straight line with the forearm axis, and the five fingers were naturally separated, with the thumb at an approximately 30-degree angle to the palm. The middle finger axis was in a straight line with the forearm axis, held horizontally, and the elbow was bent at a 90-degree angle. The hand occupied approximately 30% of the entire picture, with about 2–3 cm of the ulna and distal radius backbone included in the radiograph. Acquisition parameters were configured to minimize X-ray exposure dose (1.6–20 mAs, 55–65 kV).
Data annotations
The radiograph annotation team comprised over 30 professional endocrinologists and radiologists from nine different children’s hospitals. These team members were required to be at least senior attending physicians with a minimum of 10 years of experience in interpreting X-ray radiographs of children and assessing bone age. Upon joining the program, these reviewers underwent standardized training in the properly use of the annotation system. All reviewers underwent comprehensive training and successfully passed an examination to demonstrate their ability to annotate accurately. During the annotation process, each radiograph image was evaluated using the TW3 scoring method. This evaluation took place on the online annotation platform, involving three reviewers, including at least one endocrinologist and one radiologist. The radius, ulna, metacarpals, and phalanges in the first, third and fifth digits of the hand were assessed using the TW3-RUS method. Additionally, the series of carpals was assessed using the TW3-Carpal method, with each of the 20 bones categorized into 8 or 9 stages. The stage was subsequently converted into a score. Ultimately, a total score was computed and converted into the bone age. The assessment of bone age was carried out independently by three professional reviewers using the TW3 method as described earlier. When two or three of these reviewers reached a consensus on the ossification center judgment for a particular bone, the average value was considered as the gold standard. In cases where a consensus on scores could not be reached, the image was submitted to a committee of experts for additional validation and determination. This committee consisted of three experts, namely Fu, Huang, and Wu, selected for their expertise, qualifications, and an average of 20 years of prior experience in BAA. The committee would engage in a discussion regarding the radiograph, evaluate the image, and ultimately provide the result as the gold standard.
Model implementation
The data pre-processing of collected radiographs and the model implementation have been extensively explained by Zhou et al. in 2020 (14). In the current study, no alterations or improvements were made to the AI system. We used the same version of the AI system described in the referenced paper without any modification. To construct the model, we gathered 9,059 clinical radiographs of the left hand between January 2012 and December 2016 in our hospital. Among them, 8,005/9,059 (88%) samples were treated as the training set for model implementation, 804/9,059 (9%) samples were set aside as the validation set for parameters optimization, and the remaining 250/9,059 (3%) samples were used to verify the accuracy and reliability of the model. The average processing time for the TW3-AI model was 1.5±0.2 s, significantly shorter than the average time (525.6±55.5 s) required for endocrinologists or radiologists to assess bone age using the TW3 rule.
In our research paper, we presented a comprehensive multi-stage neural network framework for BAA. First, we performed preprocessing and adjusted the bone image to meet the required input parameters (Figure 3A). Next, we utilized a Feature Pyramid Objective Detection Convolutional Neural Network (FPN-CNN) to identify key points on the epiphyseal and carpal bones (Figure 3B,3C). Subsequently, we selected regions of interest (ROIs) for evaluation candidates and preserved their relationships for further analysis (Figure 3D). Finally, we transformed the ROI information into a fully connected graph, which was then fed into a Graph Convolutional Neural Network (GCN) for maturity level assessment using the TW3-RUS and TW3-Carpal methods (Figure 3E,3F). This multi-stage approach enhances the accuracy and reliability of BAA, providing a valuable tool for clinical practice.
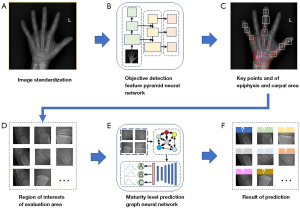
Statistical analyses
The data were analyzed using the R statistical software (version 4.1.1). The model’s overall performance was evaluated by comparing the RMSE and MAE. RMSE was calculated as the square root of the sum of the squared differences between paired values, while MAE was determined as the average of these paired differences. The 95% confidence intervals of MAE were calculated according to age groups and instrument groups (Tables S1-S4). Accuracy was defined as the percentage of the age gap between the observers’ predictions and the gold standard within 1 year. A mean paired inter-observer difference was calculated for the gold standard pair to compare the performance of both human reviewers and AI. Individual bone agreements were assessed using Fleiss’ kappa statistics for each reviewer and the AI system. B-A plots were generated to illustrate the consistency between AI assessments and the gold standard, as well as between the reviewer’s assessments and the gold standard, categorized by different examining parts and age groups. The critical difference was calculated by the distance between the line of 95% confidence interval between the two observers’ differences and the mean difference line. Statistical significance was assessed using paired t-tests for comparing mean values and F-tests for comparing variances (i.e., RMSE). A value with a P value <0.05 was considered statistically significant.
Results
The overall diagnostic performance of the TW3-AI model
When evaluating the performance of the TW3-AI system and human reviewers in estimating bone age, we considered the MAE, accuracy, and RMSE values (Table 2). The RMSE, which signifies the degree of deviation between the estimated bone age and the gold standard, decreased, indicating a higher level of agreement between the estimates and the gold standard.
Table 2
Group | Sample size | MAE | RMSE | Accuracy (%) | ||||||||
---|---|---|---|---|---|---|---|---|---|---|---|---|
MAE of reviewer-gold | MAE of AI-gold |
P value | RMSE of reviewer-gold | RMSE of AI-gold |
P value | Reviewer accuracy |
AI accuracy | |||||
TW3-RUS | ||||||||||||
Age (year) | ||||||||||||
1–6 | 172 | 0.01 | −0.02 | <0.001 | 0.43 | 0.51 | <0.001 | 95.99 | 95.36 | |||
7–12 | 367 | −0.01 | −0.06 | <0.001 | 0.59 | 0.55 | 0.64 | 90.37 | 94.44 | |||
13–18 | 178 | 0.02 | −0.15 | <0.001 | 0.53 | 0.51 | 0.22 | 92.53 | 93.36 | |||
19–20 | 27 | −0.04 | −0.14 | <0.001 | 0.25 | 0.27 | 0.45 | 98.15 | 100 | |||
Gender | ||||||||||||
Male | 378 | 0.03 | −0.05 | <0.001 | 0.54 | 0.54 | 0.05 | 92.21 | 92.86 | |||
Female | 366 | −0.03 | −0.10 | <0.001 | 0.53 | 0.50 | 0.22 | 92.49 | 96.29 | |||
All | 744 | 0.00 | −0.072 | <0.001 | 0.54 | 0.52 | 0.02 | 92.34 | 94.55 | |||
TW3-Carpel | ||||||||||||
Age (year) | ||||||||||||
1–6 | 172 | 0.05 | −0.17 | <0.001 | 0.38 | 0.43 | <0.001 | 97.05 | 95.99 | |||
7–12 | 367 | 0.03 | −0.07 | <0.001 | 0.79 | 0.82 | <0.001 | 83.52 | 80.56 | |||
13–18 | 178 | −0.08 | −0.35 | <0.001 | 1.02 | 1.09 | <0.001 | 69.29 | 68.46 | |||
19–20 | 27 | −0.27 | −0.94 | <0.001 | 0.83 | 1.38 | <0.001 | 72.22 | 46.3 | |||
Gender | ||||||||||||
Male | 378 | 0.03 | −0.12 | <0.001 | 0.79 | 0.82 | <0.001 | 82.25 | 82.25 | |||
Female | 366 | −0.03 | −0.24 | <0.001 | 0.77 | 0.87 | <0.001 | 83.8 | 78.44 | |||
All | 744 | 0.00 | −0.18 | <0.001 | 0.78 | 0.85 | <0.001 | 83.01 | 80.38 |
MAE, mean absolute error; RMSE, root mean square error; AI, artificial intelligence; TW3, Tanner-Whitehouse 3; RUS, radius, ulna and short bones.
The RMSE of the AI model in TW3-RUS was 0.52 years, which was lower than that of reviewers at 0.54 years (P=0.02). This demonstrates that the TW3-AI-RUS model performed better than manual assessments in BAA. The overall accuracy of TW3-AI and reviewers using the TW3-RUS method were 94.55% and 92.34%, respectively, indicating the model’s reliability and excellence in accuracy. On the other hand, the RMSE of the AI model in TW3-Carpal was slightly higher than that of reviewers (0.85 vs. 0.78 years, P<0.001). Additionally, the overall accuracy of the AI model in TW3-Carpal was slightly lower than the reviewers (80.38% vs. 83.01%).
The BAA of the TW3-AI system and human reviewers was conducted, and the results demonstrated excellent consistency across different genders. Specifically, in boys, the RMSE of the AI model and reviewers in TW3-RUS were 0.54 and 0.54 years (P=0.05), respectively. For girls, the RMSE values for the AI model and reviewers in TW3-RUS were 0.50 and 0.53 (P=0.22), respectively. The accuracy rates achieved by the TW3-AI-RUS system and human reviewers were comparable, with the AI achieving an accuracy of 92.86% and 96.29% in boys and girls, while human reviewers achieved an accuracy of 92.21% and 92.49% in boys and girls, respectively. When evaluating carpal bones, the RMSE values for the AI model and reviewers were 0.82 and 0.79 (P<0.001) in boys, and 0.87 and 0.77 (P<0.001) in girls. The accuracy remained consistent at 82.25% in boys, while in girls, the AI achieved an accuracy of 78.44%, and reviewers achieved 83.8%.
The consistence of TW3-AI in BAA among different age groups
When age stratification was applied, no significant differences in RMSE were observed between the AI model and reviewers in TW3-RUS among patients aged over 7 years old, with P values of 0.64, 0.22, and 0.45 for patients aged between 7–12, 13–18, and 19–20 years old, respectively. However, among patients between 1 and 6 years old, the RMSE of TW3-AI was higher than that of reviewers (P<0.001). For the TW3-RUS method, the accuracy of AI model was higher than reviewers among the patients over 7 years old (94.44% vs. 90.37% in 7–12 years group, 93.36% vs. 92.53% in 13–18 years old, 100% vs. 98.15% in 19–20 years old), while lower in patients between 1–6 years old (95.36% vs. 95.99%). These data indicate that the accuracy and consistency of TW3-AI-RUS in BAA were better in patients over 7 years old than in younger ones.
When assessing Carpal bones, the RMSE of TW3-AI was higher than that of reviewers in all the age groups (P<0.001). In the TW3-Carpal system, the accuracy of both AI model and reviewers was lower compared to that in the TW3-RUS system for patients over 7 years old (80.56% vs. 83.52% in 7–12 years group, 68.46% vs. 69.29% in 13–18 years old, 46.3% vs. 72.22% in 19–20 years old). However, in children between 1 and 6 years old, the accuracy of both AI model and reviewers was higher than that in the TW3-RUS system (95.99% by model and 97.05% by reviewers in Carpal vs. 95.36% by model and 95.99% by reviewers in RUS). These findings suggest that the accuracy and consistency of TW3-AI-Carpal in BAA were better in children under 7 years old than older individuals.
The B-A plot (Figure 4 for TW3-RUS and Figure 5 for TW3-Carpal) illustrates the agreement between the AI model, the reviewers, and the gold standard, demonstrating a high level of concordance between the model and reviewers (Figure 4A and Figure 5A). In the TW3-RUS model, most paired-BAA differences were less than 1 year, and the points representing the differences predominantly fell within the 95% confidence interval of agreements compared to the reviewers’ assessments. In the TW3-Carpal, both the AI and reviewers exhibited similar performance, although for patients over 11 years old, there was a slight increase in the proportion of points outside the 95% confidence interval. To assess the impact of age on BAA, the subjects were categorized into different age groups, and subsequent BA analysis was conducted (Figures 6,7). Notably, the 19–20 years old groups were not included due to the limited sample size. The results revealed that both AI and reviewers exhibited greater consistency in BAA among younger children. Furthermore, the TW3-RUS method showed closer alignment with the gold standard compared to TW3-Carpal when assessing the bone age of children aged 7 to 18 years old.
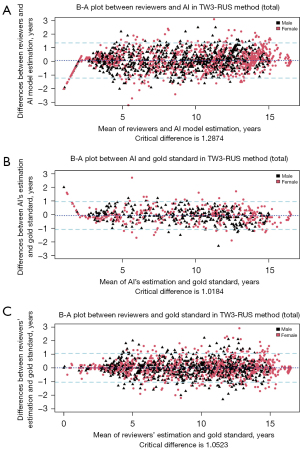
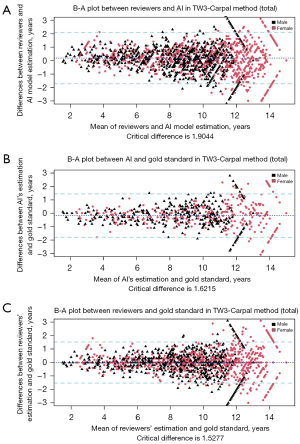

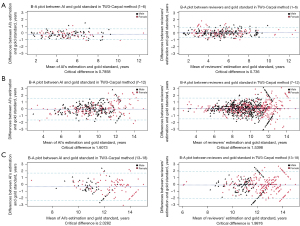
Effects of different sources of radiographs on TW3-AI in BAA
To examine the stability and accuracy of the TW3-AI system, we analyzed the RMSE of TW3-AI-RUS and TW3-AI-Carpal using radiographs acquired from various imaging instruments across different medical centers. The results were presented in Tables 3,4. In the RUS system, the RMSE of AI model did not show statistical significance compared to the reviewers when using most instruments, including Canon Incorporated Company (P=0.43), Ge Healthcare (P=0.55), Kodak (P=0.73), Philips Medical (P=0.47), and Siemens (P=0.61). This demonstrates the AI system’s suitability for clinical use in assessing radiographs from various instruments. While TW3-AI’s BAA in TW3-Carpal may be slightly less accurate than that of the reviewers, it still falls within the acceptable clinical range. Notably, among all manufacturers, Canon Incorporated Company exhibited a significant advantage in BAA accuracy for both TW3-RUS and TW3-Carpal methods, achieving 100% and 85.83%, respectively.
Table 3
Manufacturer | Sample size | MAE | RMSE | Accuracy (%) | |||||||
---|---|---|---|---|---|---|---|---|---|---|---|
MAE of reviewer-gold | MAE of AI-gold |
P value | RMSE reviewer-gold |
RMSE of AI-gold | P value | RUS reviewer accuracy | RUS AI accuracy | ||||
Canon Inc. | 43 | 0.07 | −0.02 | <0.001 | 0.51 | 0.46 | 0.43 | 92.91 | 100 | ||
Carestream Health | 107 | −0.05 | −0.16 | <0.001 | 0.52 | 0.56 | 0.01 | 92.95 | 94.97 | ||
GE HealthCare | 63 | 0.06 | −0.04 | <0.001 | 0.60 | 0.58 | 0.55 | 90.56 | 90.56 | ||
Kodiak | 173 | −0.03 | −0.08 | <0.001 | 0.57 | 0.55 | 0.73 | 90.89 | 93.52 | ||
Philips Medical | 195 | 0.02 | −0.02 | <0.001 | 0.51 | 0.49 | 0.47 | 92.98 | 94.88 | ||
Siemens | 96 | 0.02 | −0.10 | <0.001 | 0.53 | 0.48 | 0.61 | 92.62 | 95.57 |
BAA; bone age assessment; TW3, Tanner-Whitehouse 3; RUS, radius, ulna and short bones; MAE, mean absolute error; RMSE, root mean square error; AI, artificial intelligence.
Table 4
Manufacturer | Sample size | MAE | RMSE | Accuracy (%) | |||||||
---|---|---|---|---|---|---|---|---|---|---|---|
MAE of reviewer-gold | MAE of AI-gold |
P value | RMSE of reviewer-gold | RMSE of AI-gold | P value | Carpel reviewer accuracy | Carpel AI accuracy | ||||
Canon Inc. | 43 | −0.09 | −0.05 | <0.001 | 0.81 | 0.92 | <0.001 | 85.04 | 85.83 | ||
Carestream Health | 107 | −0.08 | −0.13 | <0.001 | 0.79 | 0.79 | <0.001 | 82.21 | 82.21 | ||
GE HealthCare | 63 | 0.06 | 0.09 | <0.001 | 0.63 | 0.67 | <0.001 | 88.33 | 86.11 | ||
Kodiak | 173 | −0.02 | −0.03 | <0.001 | 0.84 | 0.88 | <0.001 | 80.77 | 77.33 | ||
Philips Medical | 195 | 0.02 | −0.44 | <0.001 | 0.77 | 0.95 | <0.001 | 81.97 | 78.56 | ||
Siemens | 96 | 0.03 | −0.28 | <0.001 | 0.80 | 0.79 | <0.001 | 83.39 | 81.55 |
BAA, bone age assessment; TW3, Tanner-Whitehouse 3; MAE, mean absolute error; RMSE, root mean square error; AI, artificial intelligence.
The optimized TW3-AI model showed less variability in BAA
Kappa tests were employed to assess the concordance between AI and reviewers in the evaluation of each specific bone. The interpretation of kappa suggests that a range of 0.41–0.60 signifies moderate agreement, 0.60–0.80 indicates substantial agreement, and 0.81–1.00 represents nearly perfect agreement. As illustrated in Figure 8, experienced reviewers’ interpretations seldom exhibited divergent opinions. The overall consistency of AI showed moderate agreement (Table S5), falling within the medically acceptable range and aligning with results from our prior study (14).
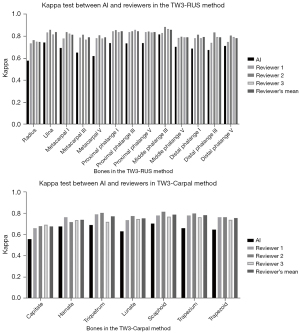
In our previous study, interpretations exhibited the greatest variability in male capitate and hamate bones, as well as female capitate and trapezoid bones in TW3-Carpal. Additionally, the bones with the highest estimation variation in TW3-RUS were the male’s first distal and fifth middle phalanx, as well as the female’s third middle and fifth middle phalanx, all with kappa values below 0.6. However, in this multicenter study employing the optimized TW3-AI system, we observed an increase in kappa values for the detection of the hamate (kappa =0.68) and trapezoid (kappa =0.65) in TW3-Carpal, as well as the first distal (kappa =0.69), third middle (kappa =0.81), and fifth middle (kappa =0.70) in TW3-RUS. Nonetheless, there were still some challenges in detecting the capitate (kappa =0.56) in TW3-Carpal and the radius (kappa =0.58) in TW3-RUS, indicating moderate agreement. The introduction of the gold standard and standardized photography criterion contributed to reducing the variation of BAA but still encountered difficulties in consistently distinguishing some specific bones, such as the capitate.
Discussion
In this multicenter validation study of the previously established TW3-AI BAA system, we have showcased its remarkable expertise and consistency across multiple centers, diverse instruments, various age groups, and geographical regions. These results strongly affirm the reliability and potential applicability of the AI system for children and adolescents in diverse settings. While the optimized TW3-AI model exhibited reduced variability in BAA, it still encountered challenges in consistently distinguishing specific bones, such as the capitate.
In preparation for this investigation, we established rigorous criteria to standardize the radiographic procedure. A prior research delved into the behavior of deep CNN bone age algorithms when presented with inappropriate data inputs, both in radiological and non-radiological domains. This research highlighted the AI’s inability to distinguish inappropriate data inputs (15). Therefore, the adoption of a uniform radiographic method in this multicenter study played a crucial role in minimizing errors in radiograph interpretation and ensuring the accuracy of BAA while reducing potential sources of interference (16). Additionally, the overall high quality of the radiographs collected from multiple centers, the standardized training provided to each reviewer, and the incorporation of a gold standard in this investigation all contributed to the reduction of inter-reviewer variation.
The RMSE for TW3-RUS and TW3-Carpal by AI l was 0.52 and 0.84 years, respectively, with the accuracy of 94.55% and 80.38% when compared to the gold standard. These indicate that the model is still some distance from perfection. It is important to consider that the AI’s training database originated from a single hospital, and past quality control assessments by reviewers may not have been precise, resulting in the current inevitable inter-reviewer variations. In this regard, when compared to BoneXpert (17), a well-established BAA system founded on data from four different studies in Denmark, our study implies the imperative requirement of continuous optimization of model training to improve the accuracy and consistency of BAA.
Additionally, it is worth noting that the computer version of this model can scan and integrate all available information from radiographs, whereas human reviewers primarily assess grades by observing specific bone features. Because the relative importance assigned to various features on the radiograph may not be identical, the bone age results from the two methods may exhibit substantial differences (18).
As the results indicate, TW3-AI demonstrates substantial stability across different age groups, especially in the assessment of RUS series bones (Figure 6). However, in Figure 7, TW3-Carpal exhibits less consistency in the assessment of bone aged 7 to 12 and 13 to 16 years. This is because, as growth progresses, some carpals may overlap with others, and by age 13 years in boys and 11 years in girls, the carpals have reached full maturity and cease further development (19). Carpals, characterized by less prominent morphological changes in hand and wrist bones during puberty, pose challenges for both AI and reviewers in identification (20). During this stage, RUS features become the predominant parameters for depicting bone maturity, spanning from 10 years old to the adult stage.
While our AI system demonstrates excellence in BAA in a multicenter system, there are still some limitations, notably the relatively small dataset. Therefore, obtaining more data is essential to enhance the system’s reliability. Additionally, the included data were primarily collected from the hospitals in the southern regions, and it remains unclear whether data from northern regions would yield similar results. Given the sampled cities are predominantly first-tier and second-tier cities, further research is required to ascertain the universality of our findings among rural children. In our forthcoming research endeavors, we intend to place more emphasis on the correlation between the characteristic signs of some common endocrinological diseases and specific abnormal bone age. This presents a challenge for AI in terms of identification and diagnosis. For example, we may choose to focus on the delayed puberty groups to investigate whether AI can properly assess the maturation level of each bone.
Our BAA model, powered by deep learning algorithms, stands as a splendid example of how computer vision and AI can be effectively integrated into clinical practice. It is undeniable that AI systems and deep learning algorithms are poised to become increasingly prevalent, serving as valuable aids in daily clinical diagnosis and treatment across various organs, pathologies, and image modalities. There are numerous remarkable instances in diverse complex domains, including cardiovascular diseases, oncological diseases, dermatology, and more, all of which significantly contribute to enhancing the capabilities of general practitioners and streamlining the decision-making process (21,22). However, it is important to acknowledge that challenges persist in the form of a high number of false positive or non-relevant AI-based findings when employing AI software platforms for specific medical diagnoses (23). First and foremost, it is worth noting that the AI model relies solely on the standalone computer version for medical image analysis, lacking the support of clinical data. Incorporating clinical data, along with the patient’s medical history and relevant diagnostic inquiries, is undoubtedly poised to make the AI system more targeted and efficient. In addition, if the AI system could compare the new image with the patient’s previous imaging studies, it could yield valuable insights for risk assessment, customized pre-and post-treatment evaluation, longitudinal follow-up, and significantly alleviate the burden on clinicians (24).
Conclusions
In summary, this TW3-AI system conducted by our team was validated in this multicenter study, which can efficiently simplify the complexity of the TW3 method while maintaining nearly the same level of accuracy. Our study opted for the TW3 method, rather than the more convenient yet less detailed Greulich and Pyle (GP) method, with the goal of validating its suitability for Chinese children and young adults across diverse working environments. Consequently, this TW3-AI BAA system holds significant promise for clinical expansion in China. It has the capacity to alleviate the workload of Chinese clinicians by providing a convenient means to interpret and accurately process radiographs from various types of instruments in different regions.
Acknowledgments
We are deeply grateful to all the children who participated in our study program. We are incredibly grateful to Rahim Ullah (Department of Endocrinology, Children’s Hospital of Zhejiang University School of Medicine, National Clinical Research Center for Child Health, Hangzhou, China) who provided language editing and proofreading assistance.
Funding: This work received financial support from
Footnote
Reporting Checklist: The authors have completed the STARD reporting checklist. Available at https://qims.amegroups.com/article/view/10.21037/qims-23-715/rc
Conflicts of Interest: All authors have completed the ICMJE uniform disclosure form (available at https://qims.amegroups.com/article/view/10.21037/qims-23-715/coif). JF is an employee of Beijing Deepwise & League of PHD Technology Co., Ltd., in charge of developing the AI model. The other authors have no conflicts of interest to declare.
Ethical Statement: The authors are accountable for all aspects of the work in ensuring that questions related to the accuracy or integrity of any part of the work are appropriately investigated and resolved. The study was conducted in accordance with the Declaration of Helsinki (as revised in 2013). Ethical approval was granted by the Children’s Hospital of Zhejiang University School of Medicine and is on file at all the participating centers. All participating hospitals were informed and agreed with the study. Informed consent was taken from all the patients. And for patients under 18, informed consent was obtained from the patients’ legal guardians.
Open Access Statement: This is an Open Access article distributed in accordance with the Creative Commons Attribution-NonCommercial-NoDerivs 4.0 International License (CC BY-NC-ND 4.0), which permits the non-commercial replication and distribution of the article with the strict proviso that no changes or edits are made and the original work is properly cited (including links to both the formal publication through the relevant DOI and the license). See: https://creativecommons.org/licenses/by-nc-nd/4.0/.
References
- Wu Q, Zhao L, Ye XC. Shortage of healthcare professionals in China. BMJ 2016;354:i4860. [Crossref] [PubMed]
- Zhang Y, Huang L, Zhou X, Zhang X, Ke Z, Wang Z, et al. Characteristics and Workload of Pediatricians in China. Pediatrics 2019;144:e20183532. [Crossref] [PubMed]
- Wang H, Liang L, Du C, Wu Y. Implementation of Online Hospitals and Factors Influencing the Adoption of Mobile Medical Services in China: Cross-Sectional Survey Study. JMIR Mhealth Uhealth 2021;9:e25960. [Crossref] [PubMed]
- Matsuoka H, Sato K, Sugihara S, Murata M. Bone maturation reflects the secular trend in growth. Horm Res 1999;52:125-30. [Crossref] [PubMed]
- Zerin JM, Hernandez RJ. Approach to skeletal maturation. Hand Clin 1991;7:53-62.
- Creo AL, Schwenk WF 2nd. Bone Age: A Handy Tool for Pediatric Providers. Pediatrics 2017;140:e20171486. [Crossref] [PubMed]
- Cavallo F, Mohn A, Chiarelli F, Giannini C. Evaluation of Bone Age in Children: A Mini-Review. Front Pediatr 2021;9:580314. [Crossref] [PubMed]
- Gao C, Qian Q, Li Y, Xing X, He X, Lin M, Ding Z. A comparative study of three bone age assessment methods on Chinese preschool-aged children. Front Pediatr 2022;10:976565. [Crossref] [PubMed]
- Anwar SM, Majid M, Qayyum A, Awais M, Alnowami M, Khan MK. Medical Image Analysis using Convolutional Neural Networks: A Review. J Med Syst 2018;42:226. [Crossref] [PubMed]
- Iglesias LL, Bellón PS, Del Barrio AP, Fernández-Miranda PM, González DR, Vega JA, Mandly AAG, Blanco JAP. A primer on deep learning and convolutional neural networks for clinicians. Insights Imaging 2021;12:117. [Crossref] [PubMed]
- Hosny A, Parmar C, Quackenbush J, Schwartz LH, Aerts HJWL. Artificial intelligence in radiology. Nat Rev Cancer 2018;18:500-10. [Crossref] [PubMed]
- Huang Z, Li Q, Lu J, Feng J, Hu J, Chen P. Recent Advances in Medical Image Processing. Acta Cytol 2021;65:310-23. [Crossref] [PubMed]
- Zhang Y, Zhu W, Li K, Yan D, Liu H, Bai J, Liu F, Cheng X, Wu T. SMANet: multi-region ensemble of convolutional neural network model for skeletal maturity assessment. Quant Imaging Med Surg 2022;12:3556-68. [Crossref] [PubMed]
- Zhou XL, Wang EG, Lin Q, Dong GP, Wu W, Huang K, Lai C, Yu G, Zhou HC, Ma XH, Jia X, Shi L, Zheng YS, Liu LX, Ha D, Ni H, Yang J, Fu JF. Diagnostic performance of convolutional neural network-based Tanner-Whitehouse 3 bone age assessment system. Quant Imaging Med Surg 2020;10:657-67. [Crossref] [PubMed]
- Yi PH, Arun A, Hafezi-Nejad N, Choy G, Sair HI, Hui FK, Fritz J. Can AI distinguish a bone radiograph from photos of flowers or cars? Evaluation of bone age deep learning model on inappropriate data inputs. Skeletal Radiol 2022;51:401-6. [Crossref] [PubMed]
- Gao Y, Zhu T, Xu X. Bone age assessment based on deep convolution neural network incorporated with segmentation. Int J Comput Assist Radiol Surg 2020;15:1951-62. [Crossref] [PubMed]
- Thodberg HH, Kreiborg S, Juul A, Pedersen KD. The BoneXpert method for automated determination of skeletal maturity. IEEE Trans Med Imaging 2009;28:52-66. [Crossref] [PubMed]
- Liu J, Qi J, Liu Z, Ning Q, Luo X. Automatic bone age assessment based on intelligent algorithms and comparison with TW3 method. Comput Med Imaging Graph 2008;32:678-84. [Crossref] [PubMed]
- Hsieh CW, Jong TL, Tiu CM. Bone age estimation based on phalanx information with fuzzy constrain of carpals. Med Biol Eng Comput 2007;45:283-95. [Crossref] [PubMed]
- Ahn KS, Bae B, Jang WY, Lee JH, Oh S, Kim BH, Lee SW, Jung HW, Lee JW, Sung J, Jung KH, Kang CH, Lee SH. Assessment of rapidly advancing bone age during puberty on elbow radiographs using a deep neural network model. Eur Radiol 2021;31:8947-55. [Crossref] [PubMed]
- Olveres J, González G, Torres F, Moreno-Tagle JC, Carbajal-Degante E, Valencia-Rodríguez A, Méndez-Sánchez N, Escalante-Ramírez B. What is new in computer vision and artificial intelligence in medical image analysis applications. Quant Imaging Med Surg 2021;11:3830-53. [Crossref] [PubMed]
- Greco F, Mallio CA. Artificial intelligence and abdominal adipose tissue analysis: a literature review. Quant Imaging Med Surg 2021;11:4461-74. [Crossref] [PubMed]
- Rueckel J, Sperl JI, Kaestle S, Hoppe BF, Fink N, Rudolph J, Schwarze V, Geyer T, Strobl FF, Ricke J, Ingrisch M, Sabel BO. Reduction of missed thoracic findings in emergency whole-body computed tomography using artificial intelligence assistance. Quant Imaging Med Surg 2021;11:2486-98. [Crossref] [PubMed]
- Mallio CA, Quattrocchi CC, Beomonte Zobel B, Parizel PM. Artificial intelligence, chest radiographs, and radiology trainees: a powerful combination to enhance the future of radiologists? Quant Imaging Med Surg 2021;11:2204-7. [Crossref] [PubMed]