Clinical application of convolutional neural network for mass analysis on mammograms
Introduction
According to the latest global cancer data released by the International Agency for Research on Cancer (IARC), in 2020, breast cancer surpassed lung cancer as the most commonly diagnosed cancer worldwide and is among the leading causes of cancer-related death in women (1). Mammography is the first-line imaging modality for breast cancer screening and diagnosis, playing a central role in early detection and treatment. The detection of calcifications and masses on a mammogram represent some of the earliest signs of a malignant breast tumor. Calcifications are clearly depicted on mammograms, as they almost completely absorb X-radiation. Masses may be hard to detect if the breast tissue is dense, leading to false negative results.
In recent years, deep learning (DL) has become a research hotspot in the application of artificial intelligence to medical imaging. Convolutional neural networks (CNN), a class of artificial neural network, are commonly used for image processing, with the CNN-based DL method matching or surpassing human intelligence in medical image analysis and diagnosis (2-5).
At present, most studies investigating the application of DL in mammography have been based on image databases from Western countries. However, more Asian women than Western women have dense breasts (6), and it is uncertain whether DL models constructed using mammographic databases in Western countries can be applied to Asian women. In our previous work (7), we constructed a CNN-based DL system for mass analysis using a Chinese mammography database. The model can be used to detect and classify masses in mammographic images. Chinese women have a common characteristic of Asian women in terms of breast density. Therefore, the training set used in this study could be considered to represent Asian women. The aim of this study was to explore the clinical application of the CNN-based DL system as an objective and accurate tool for breast cancer screening and diagnosis in Asian women.
Methods
Participants
The study was conducted in accordance with the provisions of the Declaration of Helsinki (as revised in 2013). The study was approved by the Ethics Committee of Shenzhen People’s Hospital (No. LL-KY-2021624), and the requirement for individual consent for this retrospective analysis was waived. Women with masses detected on a diagnostic mammogram at Shenzhen People’s Hospital between April and December 2019 were eligible for this study. The inclusion criteria were as follows: (I) satisfactory diagnostic image quality; (II) standard mammographic projections: bilateral or unilateral cranio-caudal (CC) projection and internal and mediolateral oblique (MLO) projection; and (III) masses underwent histopathological examination after mammography, or were confirmed benign by other imaging examinations or stable follow-up for 2 years. The exclusion criteria were as follows: (I) poor image quality; (II) breast augmentation with implants or injection of filler; or (III) having received neoadjuvant chemotherapy.
Imaging
All images were collected by digital mammography machines from Siemens Mammoma Inspiration (Siemens, Erlangen, Germany) (anode target: molybdenum/tungsten, filtering material: molybdenum/rhodium), GE Senographe Pristina (GE Healthcare, Chicago, IL, USA) (anode target: molybdenum/rhodium, filtering material: molybdenum/argentum), or Hologic Selenia Dimensions system (Hologic, Marlborough, MA, USA) (anode target: tungsten, filtering material: rhodium/argentum/aluminum). All devices adopted automatic exposure time control and breast compression methods. Cameras selected manual exposure in special cases. CC and MLO projection images were routinely captured. Mammography acquisition complied with the technical standards formulated for the construction and quality control of mammography databases in 2022 by the Mammography Group of the Radiology Branch of the Chinese Medical Association (8). In order to ensure that the model has good generalization ability and good adaptability to images taken by different machine, data-cleaning was applied to the images from various vendors’ machines. All the pixels of images were resized to 448×448 pixels.
DL analysis system
The CNN-based DL analysis system (Mammo-AI-MASS), which was jointly developed by our hospital and Ping An Technology (Shenzhen) Co., Ltd. (Shenzhen, China), was used in this study.
Mammo-AI-MASS includes two models, detection and classification. The detection model (Figure 1) consists of three submodules: the ipsilateral dual-view network (IDVN), bilateral dual-view network (BDVN), and integrated fusion network (IFN). The detection model receives multiple projection images from different views for each patient, and designs high resolution deep detection and segmentation networks for ipsilateral and contralateral images to detect masses. Most women have roughly symmetric breasts in terms of density and texture. This property is well leveraged by radiologists to identify the abnormalities in mammograms. Hinging on a bilateral dual-view, radiologists are able to locate a mass based on its distinct morphologic appearance and relative position compared to its corresponding area in the lateral image. The BDVN submodule was developed to incorporate this diagnostic prior information and facilitate the learning of the symmetry constraint. Nipple locations are required in image registration for MLO views and IDVN. Ipsilateral images provide information on the same breast from two different views. Hence, a mass in the ipsilateral images tends to have similar distances to the nipple and share common appearance traits. This supplies essential knowledge to assist radiologists in making decisions. The IDVN submodule was developed to incorporate this prior diagnostic knowledge.
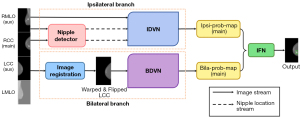
Using the right CC (RCC) image as an example, the IDVN uses the RCC image as the main view and the right MLO image as the auxiliary view, and the BDVN uses the RCC image as the main view and the left CC image as the auxiliary view. Comparison of the left and right breasts allows detection of a suspicious (with mass) area on the main image. Using the nipple detection algorithm combined with the object detection algorithm, the IDVN and BDVN output a probability map of mass location on the RCC. The IFN combines the outputs from the IDVN and the BDVN to generate final mass detection results.
The detected masses are classified using a multi-task DL model (Figure 2). During the training period, a large number of mammography images with benign and malignant masses are input into DenseNet-121, and features of the masses are extracted and classified. The model outputs a score of 0 to 100 (with 0 indicating benign and 100 indicating malignant) to determine the probability that the detected mass is benign or malignant.
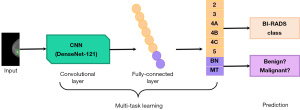
Imaging interpretation
For interpretation, images were independently analyzed by two junior radiologists (A and B, with 2 or 3 years of experience) who were blinded to the previous imaging report, clinical history, and pathological results. As the reference, a senior radiologist A with 20 years of experience in breast imaging analyzed the images after reviewing all patients’ relevant information. Masses were classified in consensus by two other senior radiologists (B and C with 10 or 15 years of respective experience), and images were input into the DL system. Masses without pathological results were confirmed benign by other imaging examinations or stable follow-up for 2 years.
The 2013 American College of Radiology (ACR) BI-RADS version 5 (9), was used for two junior (A and B) and two senior (B and C) radiologists to determine the patient’s breast density, and the morphology, margin, size, density, and BI-RADS category of the masses. BI-RADS categories 4A, 4B, 4C, and 5 require biopsy and were therefore defined as positive, whereas BI-RADS categories 1, 2, and 3 were defined as negative.
Statistical analysis
Statistical analyses were performed using SPSS 26.0 (IBM Corp., Armonk, NY, USA). The sensitivity of mass detection by the junior radiologists and the DL system was calculated as the number of images in which the junior radiologists or the DL system correctly detected a mass among all images with masses. Pearson’s chi-square (χ2) test was used to assess the effects of different factors (breast density; patient age; the morphology, margin, size, and BI-RADS category of the masses) on mass detection by the junior radiologists and the DL system. Area under the receiver operator characteristic (ROC) curves (AUC) and 95% confidence intervals (95% CIs) were used to assess the accuracy, sensitivity, and specificity of mass classification by the junior radiologists, senior radiologists, and the DL system. AUCs were compared with DeLong’s test. A P value <0.05 was considered statistically significant.
Results
Features of breast masses
A total of 324 patients (mean age, 46.07±12.18 years; age range, 22–87 years) with masses detected on a diagnostic mammogram were enrolled in this study (618 masses). Most patients had oval masses [66.0% (214/324) patients, 405 masses], with obscured margins [35.8% (116/324) patients, 224 masses] and equal density [70.7% (229/324) patients, 428 masses] that were BI-RADS 3 or 4A [BI-RADS 3: 30.2% (98/324) patients, 192 masses; BI-RADS 4A: 24.1% (78/324) patients, 138 masses] (Table 1).
Table 1
Feature | Category | Case (%) (n=324) | Number of masses (%) (n=618) |
---|---|---|---|
Morphology | Round | 17 (5.2) | 27 (4.4) |
Oval | 214 (66.0) | 405 (65.5) | |
Irregular | 93 (28.7) | 186 (30.1) | |
Margin | Circumscribed | 79 (24.4) | 146 (23.6) |
Obscured | 116 (35.8) | 224 (36.2) | |
Microlobulated | 20 (6.2) | 39 (6.3) | |
Indistinct | 52 (16.0) | 97 (15.7) | |
Spiculated | 57 (17.6) | 112 (18.1) | |
Density | a* | 5 (1.5) | 9 (1.5) |
b* | 7 (2.2) | 13 (2.1) | |
c* | 229 (70.7) | 428 (69.3) | |
d* | 83 (25.6) | 168 (27.2) | |
BI-RADS | 2 | 15 (4.6) | 27 (4.4) |
3 | 98 (30.2) | 192 (31.1) | |
4A | 78 (24.1) | 138 (22.3) | |
4B | 40 (12.3) | 79 (12.8) | |
4C | 38 (11.7) | 72 (11.7) | |
5 | 55 (17.0) | 110 (17.8) |
*, the letter refers to the BI-RADS guideline classification of breast density: a, fat-containing; b, low density; c, equal density; d, high density. BI-RADS, breast imaging reporting and data system.
Histopathological classification
Among the 618 masses, tissue from 258 masses underwent histopathological examination after diagnostic mammography and 360 masses were confirmed benign by other imaging examinations or stable follow-up for 2 years. Among all the masses with precise pathological results, fibroadenoma (67.9%, 72/106, except for ‘stable follow-up’) and invasive ductal carcinoma (70.4%; 107/152) were the most common negative and positive cases, respectively (Table 2).
Table 2
Pathological type | Number of masses (%) |
---|---|
Negative (n=466) | |
Fibroadenoma | 72 (15.5) |
Hyperplasia | 29 (6.2) |
Dilation of duct | 1 (0.2) |
Epidermoid cyst | 1 (0.2) |
Inflammatory disease | 3 (0.6) |
Stable follow-up | 360 (77.3) |
Positive (n=152) | |
Invasive ductal carcinoma | 107 (70.4) |
Invasive lobular carcinoma | 7 (4.6) |
Ductal carcinoma in situ | 8 (5.3) |
Mucinous carcinoma | 6 (3.9) |
Phyllode tumor | 7 (4.6) |
Intraductal papillary carcinoma | 1 (0.7) |
Intraductal papilloma | 16 (10.5) |
Sensitivity of mass detection
The sensitivity of mass detection on diagnostic mammograms by the junior radiologists [78.0% (482/618) and 84.0% (519/618), respectively] was lower than that of the DL system [86.2% (533/618)]. Breast density significantly affected mass detection by the junior radiologists (both P=0.030) but not by the DL system (P=0.385). A total of 460 masses were detected in breasts identified as c-type. The sensitivity of mass detection in breasts identified as c-type was lower for the junior radiologists [84.8% (390/460) and 77.8% (358/460), respectively] compared to the DL system [86.5% (398/460)]. A total of 97 masses were detected in breasts identified as d-type. The sensitivity of mass detection in breasts identified as d-type was lower for the junior radiologists [75.3% (73/97) and 71.1% (69/97)] compared to the DL system [85.6% (83/97)]. Patients’ age and the morphology, margin, density, and BI-RADS classification of the mass significantly affected mass detection by the junior radiologists and the DL system (Table 3).
Table 3
Variables | Reference | Junior radiologist A | Junior radiologist B | DL system | |
---|---|---|---|---|---|
Breast density | |||||
a* | 14 | 14 | 14 | 10 | |
b* | 47 | 42 | 41 | 42 | |
c* | 460 | 390 | 358 | 398 | |
d* | 97 | 73 | 69 | 83 | |
χ2 | – | 8.982 | 8.955 | 3.043 | |
P value | – | 0.030 | 0.030 | 0.385 | |
Age (years) | |||||
<40 | 167 | 131 | 124 | 130 | |
40–60 | 348 | 293 | 267 | 307 | |
>60 | 103 | 95 | 91 | 96 | |
χ2 | – | 9.032 | 8.125 | 15.282 | |
P value | – | 0.011 | 0.017 | <0.0001 | |
Morphology | |||||
Round | 27 | 20 | 20 | 19 | |
Oval | 405 | 332 | 311 | 329 | |
Irregular | 186 | 167 | 151 | 185 | |
χ2 | – | 7.838 | 1.686 | 41.700 | |
P value | – | 0.020 | 0.430 | <0.0001 | |
Margin | |||||
Circumscribed | 146 | 130 | 124 | 126 | |
Obscured | 224 | 167 | 159 | 165 | |
Microlobulated | 39 | 37 | 36 | 39 | |
Indistinct | 97 | 84 | 68 | 91 | |
Spiculated | 112 | 101 | 95 | 112 | |
χ2 | – | 24.707 | 21.727 | 58.674 | |
P value | – | <0.0001 | <0.0001 | <0.0001 | |
Density | |||||
High density | 168 | 161 | 154 | 167 | |
Equal density | 428 | 340 | 313 | 355 | |
Low density | 13 | 10 | 10 | 6 | |
Fat-containing | 9 | 8 | 5 | 5 | |
χ2 | – | 24.747 | 26.844 | 53.219 | |
P value | – | <0.0001 | <0.0001 | <0.0001 | |
BI-RADS | |||||
2 | 27 | 22 | 17 | 21 | |
3 | 192 | 152 | 146 | 145 | |
4A | 138 | 109 | 106 | 112 | |
4B | 79 | 73 | 65 | 74 | |
4C | 72 | 59 | 59 | 71 | |
5 | 110 | 104 | 89 | 110 | |
χ2 | – | 24.710 | 6.137 | 53.754 | |
P value | – | <0.0001 | 0.293 | <0.0001 |
*, the letter refers to the BI-RADS guideline classification of breast density: a, fat-containing; b, low density; c, equal density; d, high density. DL, deep learning; BI-RADS, breast imaging reporting and data system.
Classification performance
The accuracy, sensitivity, and specificity of the DL system for classifying masses on diagnostic mammograms as negative or positive was higher compared to the junior radiologists, but lower compared to the senior radiologists. The AUC for classifying masses as negative or positive for the DL system was significantly higher compared to those of the junior radiologists, but not significantly different compared to those of the senior radiologists [DL system, 0.697; junior radiologists, 0.612 and 0.620 (P=0.021, 0.019]; senior radiologists, 0.748 (P=0.071) (Table 4, Figure 3).
Table 4
Variables | Reference | Junior radiologist A | Junior radiologist B | Senior radiologist | DL system |
---|---|---|---|---|---|
Negative | 466 | 411 | 407 | 423 | 420 |
Positive | 152 | 114 | 121 | 137 | 126 |
Accuracy (%) | – | 85.0 | 85.4 | 90.6 | 88.3 |
Sensitivity (%) | – | 75.0 | 79.6 | 90.1 | 82.9 |
Specificity (%) | – | 88.2 | 87.3 | 90.8 | 90.1 |
AUC | – | 0.612 | 0.620 | 0.748 | 0.697 |
95% CI | – | 0.542–0.683 | 0.549–0.690 | 0.683–0.812 | 0.630–0.765 |
Z | – | 2.308 | 2.336 | 1.803 | – |
P value | – | 0.021 | 0.019 | 0.071 | – |
DL, deep learning; AUC, the area under the receiver operating characteristic curve; CI, confidence interval.
Discussion
This study investigated the clinical utility of a CNN-based DL system as an objective and accurate tool for breast cancer screening and diagnosis in Asian women. Specifically, Asian women tend to have denser breasts compared to Western women and an earlier age of breast cancer onset. Dense breasts may lead to missed diagnosis or misdiagnosis as dense breast tissue and masses have similar appearances on mammograms (6,10). DL algorithms have shown remarkable advancements in early breast cancer diagnosis, and may be appropriate for analyzing medical imaging of the breast in Asian women.
In the present study, the sensitivity of all mass detection in dense breasts on diagnostic mammograms was lower for the junior radiologists compared to the DL system. As masses can be obscured by dense breast tissue, these data imply that the DL system may have clinical utility in Chinese women with dense breasts, including reducing the influence of radiologist experience and the potential for missed diagnoses (Figure 4).
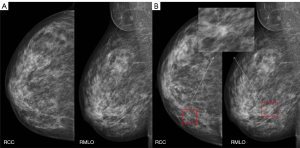
Diagnosis of breast masses on mammography is challenging due to their variation in shape, size, and margins. Malignant breast masses are characterized by irregular morphology, microlobulated, indistinct, and spiculated margins, or high density. In the present study, patients’ age and the shape, margins, density, and BI-RADS classification of the mass significantly affected mass detection by the junior radiologists and the DL system. The sensitivity of the detection of masses with malignant features was higher for the DL system compared to the junior radiologists. These data imply that the DL system can support junior radiologists in clinical decision-making for patients with breast cancer (Figure 5).
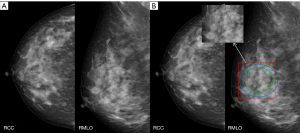
Consistent with our findings, a previous study showed that the breast mass detection rate on digital mammograms of junior radiologists is effectively improved by the use of a mammogram mass detection system based on DL and not affected by features such as breast density, BI-RADS category, morphology, and density of the mass (11). In other studies, a You Only Look Once (YOLO) computer-aided diagnosis (CAD) system based on DL was able to distinguish between benign and malignant masses on digital mammograms with an overall accuracy of 85.52% and successfully identify masses in the pectoralis muscle and dense fibrous glandular tissue (12). A CNN-based DL method improved the diagnosis of breast cancer on mammograms with a diagnostic AUC of 0.898 and 0.862 on two respective mammographic mass datasets (13); transfer learning with a deep convolutional neural network (DCNN)-based system facilitated mass classification on full-field digital mammography (FFDM) and digital breast tomosynthesis (DBT), and DBT outperformed FFDM when combined with transfer learning (14). The dataset used by the DL model constructed in this study was composed entirely of Chinese women, whose breasts had the typical characteristics of Asian women. The model has achieved high diagnostic efficiency in both detection and classification of mass lesions.
Among the positive masses missed or misdiagnosed by the DL system in this study, one intraductal papillary carcinoma was not detected. The patient had a clinical symptom of bloody discharge from the nipple, which is a sign of intraductal papillary lesions (Figure 6). Further, one intraductal papilloma, one benign phyllode tumor and four invasive carcinoma presented as suspicious malignant calcifications, which were classified as BI-RADS 4 or 5 by radiologists. There were three intraductal papilloma, one benign phyllodes tumor, and four invasive carcinoma that presented as asymmetry, which were classified as BI-RADS 4 by radiologists. Mammography is a useful diagnostic tool; however, radiologists should comprehensively analyze imaging combined with a patient’s clinical history when making a diagnosis.
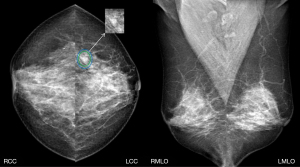
The present study has some limitations. First, this was a single-center, retrospective study with a small sample size; therefore, findings may not be generalizable to clinical practice. Second, the diagnostic performance of the radiologists combined with the DL system was not investigated.
Conclusions
The CNN-based DL system had improved mass detection and classification compared to junior radiologists and was not affected by breast density. This DL system may have clinical utility in women with dense breasts, including reducing the influence of radiologist experience and the potential for missed diagnoses, so as to be beneficial for clinicians to make decision-making recommendations.
Acknowledgments
Funding: This work was supported by
Footnote
Conflicts of Interest: All authors have completed the ICMJE uniform disclosure form (available at https://qims.amegroups.com/article/view/10.21037/qims-23-642/coif). The authors have no conflicts of interest to declare.
Ethical Statement: The authors are accountable for all aspects of the work in ensuring that questions related to the accuracy or integrity of any part of the work are appropriately investigated and resolved. The study was conducted in accordance with the provisions of the Declaration of Helsinki (as revised in 2013). The study was approved by the Ethics Committee of Shenzhen People’s Hospital (No. LL-KY-2021624), and the requirement for individual consent for this retrospective analysis was waived.
Open Access Statement: This is an Open Access article distributed in accordance with the Creative Commons Attribution-NonCommercial-NoDerivs 4.0 International License (CC BY-NC-ND 4.0), which permits the non-commercial replication and distribution of the article with the strict proviso that no changes or edits are made and the original work is properly cited (including links to both the formal publication through the relevant DOI and the license). See: https://creativecommons.org/licenses/by-nc-nd/4.0/.
References
- Sung H, Ferlay J, Siegel RL, Laversanne M, Soerjomataram I, Jemal A, Bray F. Global Cancer Statistics 2020: GLOBOCAN Estimates of Incidence and Mortality Worldwide for 36 Cancers in 185 Countries. CA Cancer J Clin 2021;71:209-49. [Crossref] [PubMed]
- Arevalo J, González FA, Ramos-Pollán R, Oliveira JL, Guevara Lopez MA. Representation learning for mammography mass lesion classification with convolutional neural networks. Comput Methods Programs Biomed 2016;127:248-57. [Crossref] [PubMed]
- Topol EJ. High-performance medicine: the convergence of human and artificial intelligence. Nat Med 2019;25:44-56. [Crossref] [PubMed]
- Zhang C, Zhao J, Niu J, Li D. New convolutional neural network model for screening and diagnosis of mammograms. PLoS One 2020;15:e0237674. [Crossref] [PubMed]
- McKinney SM, Sieniek M, Godbole V, Godwin J, Antropova N, Ashrafian H, et al. International evaluation of an AI system for breast cancer screening. Nature 2020;577:89-94. [Crossref] [PubMed]
- del Carmen MG, Halpern EF, Kopans DB, Moy B, Moore RH, Goss PE, Hughes KS. Mammographic breast density and race. AJR Am J Roentgenol 2007;188:1147-50. [Crossref] [PubMed]
- Yang Z, Cao Z, Zhang Y, Tang Y, Lin X, Ouyang R, Wu M, Han M, Xiao J, Huang L, Wu S, Chang P, Ma J. MommiNet-v2: Mammographic multi-view mass identification networks. Med Image Anal 2021;73:102204. [Crossref] [PubMed]
- Breast Group of Chinese Society of Radiology Chinese Medical Association. Expert consensus on the construction and quality control of mammography datasets. Chinese Journal of Radiology 2022;56:959-66.
- Sickles EA, D’Orsi CJ, Bassett LW, Appleton CM, Berg WA, Burnside ES, Feig SA, Gavenonis SC, Newell MS, Trinh MM (eds.). ACR BI-RADS® atlas Breast Imaging Reporting and Data System. American College of Radiology; 2013.
- Sung H, Ren J, Li J, Pfeiffer RM, Wang Y, Guida JL, Fang Y, Shi J, Zhang K, Li N, Wang S, Wei L, Hu N, Gierach GL, Dai M, Yang XR, He J. Breast cancer risk factors and mammographic density among high-risk women in urban China. NPJ Breast Cancer 2018;4:3. [Crossref] [PubMed]
- Zhang X, Zhang X, Zhang G, Hao S, Li Y. Mammography mass detection system based on deep learning in diagnosis of breast masses. Chinese Journal of Medical Imaging Technology 2019;35:1794-8.
- Al-Masni MA, Al-Antari MA, Park JM, Gi G, Kim TY, Rivera P, Valarezo E, Han SM, Kim TS. Detection and classification of the breast abnormalities in digital mammograms via regional Convolutional Neural Network. Annu Int Conf IEEE Eng Med Biol Soc 2017;2017:1230-3. [Crossref] [PubMed]
- Tsochatzidis L, Koutla P, Costaridou L, Pratikakis I. Integrating segmentation information into CNN for breast cancer diagnosis of mammographic masses. Comput Methods Programs Biomed 2021;200:105913. [Crossref] [PubMed]
- Li X, Qin G, He Q, Sun L, Zeng H, He Z, Chen W, Zhen X, Zhou L. Digital breast tomosynthesis versus digital mammography: integration of image modalities enhances deep learning-based breast mass classification. Eur Radiol 2020;30:778-88. [Crossref] [PubMed]