Opportunistic screening for osteoporosis using enhanced images based on dual-energy computed tomography material decomposition: a comparison with quantitative computed tomography
Introduction
Osteoporosis, which has been described as a silent disease, is a metabolic bone disorder characterized by a decrease in the mass of normally calcified bone tissue per unit volume and the microarchitectural deterioration of bone tissue, resulting in increased bone fragility and fracture risk (1-3). The disease prevalence is 25.41% for women and 15.33% for men in the over-50-year age group and increases with age (4). Unfortunately, a host of eligible patients do not undergo bone mineral density (BMD) measurement or receive treatment to reduce fracture risk (5). Monitoring BMD is particularly recommended for older adults and patients with malignant tumors, as osteoporosis reduces bone stability and increases the risk of fracture (6). However, the cost of osteoporosis imposes a significant economic burden worldwide (7), and regular detection of BMD using X-ray causes additional radiation. Early detection and treatment of osteoporosis can help prevent osteoporosis-related fractures (8), which is crucial for ensuring patient’s good prognosis and quality of life. Quantitative computed tomography (QCT) is a technique that uses advanced computer algorithms to process and analyze computed tomography (CT) attenuation information and measure BMD with high precision and accuracy. It can measure BMD from multiple angles and at different levels based on the 3-dimensional spatial bone tissue distribution and is highly sensitive to subtle changes in osteoporosis (9-11). However, QCT is underutilized due to the need for dedicated measurement equipment, additional radiation exposure, and its high economic costs.
With the development of CT scanning technology, tens of millions of scans are being performed worldwide each year, generating a massive amount of imaging data (12,13). Retrospective bone densitometry based on this existing repository of data has received extensive attention (14), as it avoids additional specialized examinations and does not involve additional radiation or high cost. Previous studies have shown that the CT value of the L1 vertebral trabecula can be used for diagnosing osteoporosis with a threshold of 110 Hounsfield units (HU), with a more than 90% specificity (15,16), but the CT value can be affected by scanning parameters and contrast media (17). Dual-energy CT (DECT) is capable of DECT imaging with rapid tube voltage switching between 80 and 140 kVp (<0.5 ms), providing both conventional anatomical structure information and energy spectral data (18). Booz et al. (19) reported that phantomless volumetric DECT yielded a higher accuracy in assessing BMD and diagnosing osteoporosis compared to traditional CT value measurement. Koch et al. (8) demonstrated that a dual-source DECT material decomposition technique provided more accurate volumetric BMD assessment using a standardized spine phantom at a lower radiation dose. Thus, opportunistic screening for osteoporosis is feasible using the material decomposition technique (20). However, due to the interference of iodine intake in enhanced CT, BMD assessment with DECT is largely limited to unenhanced scans, and a large amount of contrast-enhanced DECT data have not been utilized. The accuracy of BMD assessment using the material decomposition technique based on contrast-enhanced DECT has not been reported. Therefore, the purpose of this study was to investigate the feasibility of opportunistic screening for osteoporosis using the material decomposition technique based on contrast-enhanced DECT images. We present this article in accordance with the STARD reporting checklist (available at https://qims.amegroups.com/article/view/10.21037/qims-23-855/rc).
Methods
This prospective exploratory study was conducted in accordance with the Declaration of Helsinki (as revised in 2013) and approved by the Ethics Committee of First Affiliated Hospital of Dalian Medical University. All patients provided signed informed consent.
Patient population
This study enrolled 518 consecutive patients who underwent total abdominal non-contrast and contrast-enhanced CT scans for clinical indications at our institution from June 2021 to June 2022. The purpose of the scans was not BMD measurement. Patients with bone metastases from malignant tumors (n=24), L1–L3 vertebral fractures (n=55), spinal tumors (n=14), metal implants in the L1–L3 vertebrae (n=27), and hematological diseases (n=43) were excluded. Additionally, patients with contraindications for CT enhancement, such as allergies to iodine contrast media (n=7) and patients with large motion artifacts (n=2) due to poor coordination were also excluded. Ultimately, 346 patients were enrolled in the study. The flowchart of patient enrollment is shown in Figure 1.
Image acquisition
All DECT scans were acquired using a 256-row fast-switching DECT scanner (Revolution CT, GE HealthCare, Chicago, IL, USA). Unenhanced scans were performed with a fixed tube voltage of 120 kVp and smart milliampere (mA) (noise index: 10), following a conventional scanning protocol. Contrast-enhanced scans were performed in gemstone spectral imaging (GSI) mode with fast tube voltage switching between 80 and 140 kVp and a tube current of 400 mA. Other scanning parameters, including detector coverage, rotation speed, pitch, scanning slice thickness, and slice interval, were kept constant at 80 mm, 0.5 s/r, 0.992:1, and 5 mm, respectively. The nonionic contrast media (ioversol; 320 mg/mL, Jiangsu Hengrui Pharmaceutical Co., Ltd., Lianyungang, China) was applied to contrast-enhanced CT scanning. According to the clinical situation, the amount of contrast media was determined by the patient’s body weight and was administered at 500 mgI/kg with a flow rate of 3–4 mL/s. When the contrast injection was completed, 30 mL of saline was injected at the same flow rate. The SmartPrep function was used with a region of interest (ROI) placed on the abdominal aorta and a trigger threshold of 180 HU. Arterial phase scans were started 5.9 s after triggering. The portal vein phase and delayed phase were scanned at 30 and 90 s after the scanning of the arterial phase, respectively. The scanning range extended from the superior margin of the diaphragm to the inferior margin of the pubic symphysis. Additionally, the QCT BMD Model 4 Asynchronous CT Calibration Phantom (Mindways Software Inc., Austin, TX, USA) was scanned to ensure the accuracy of BMD measurement.
Image reconstruction and analysis
After scanning, the abdominal unenhanced scan images were reconstructed with a reconstructed slice thickness and interval of 1.25 mm, a display field of view of 50 cm, adaptive statistical iterative reconstruction-Veo (ASIR-V) of 50%, and the standard kernel. The reconstructed images were transmitted to the QCT Pro workstation (Model 4 QCT pro v. 6.1, Mindways Software, Inc.). The DECT material decomposition images in the arterial, portal venous, and delayed phases were also reconstructed with a thickness and interval of 1.25 mm with 50% ASIR-V before being transferred to the Advantage Workstation 4.7 (AW 4.7; GE HealthCare). DECT uses sequential switching between 80 and 140 kVp to acquire data at high and low energy levels. By exploiting the differential X-ray absorption characteristics of specific substances, two different types of materials are used to represent the attenuation of specific tissues, enabling quantitative analysis of substances. DECT material separation technique offers multiple base materials such as calcium (Ca), hydroxyapatite (HAP), iodine, water, fat, and iron. Through the selection of any two base materials and completion of base-material imaging, effective material separation and relative quantification of the target substance can be achieved. In this study, the material decomposition images were generated using both HAP and iodine (HAP-iodine) and Ca and iodine (Ca-iodine) as base-material pairs on the AW 4.7 (GE HealthCare). The HAP density was measured on the HAP-iodine images, and Ca density was measured on the Ca-iodine images.
Volumetric BMD and base-material pair measurements
For volumetric BMD measurements of unenhanced images on the QCT Pro workstation, the volume of interest (VOI) was manually defined. The VOI size was approximately two-thirds that of the centrum, the VOI depth was set at 9 mm, and the VOI location was in the middle of the vertebral body, with bone islands, the vertebral venous plexus, and osteosclerosis artifact areas being avoided. The average BMD of the L1–L3 vertebral bodies was calculated to evaluate bone status. According to the diagnostic criteria (21), a BMD of less than 80 mg/cm3 indicated osteoporosis, a BMD between 80 and 120 mg/cm3 indicated osteopenia, and a BMD greater than 120 mg/cm3 indicated normal bone mass.
For DECT data measurement, HAP-iodine and Ca-iodine material decomposition images for the arterial, portal venous, and delayed phases were generated using the GSI Viewer module on the AW 4.7. An ROI was placed in the cancellous bone area in the middle of the vertebral body. There were relatively uniform densities and no abnormal density artifacts, with an area of approximately 230 mm2, while areas such as the vertebral venous plexus and bone islands were avoided. The copy and paste functions were used to ensure consistent ROI locations for each phase. Measurements at each vertebra were repeated at the upper and lower image slices and averaged for subsequent analysis (Figure 2).

To analyze the intra- and interobserver agreement, 100 individuals were selected at random and independently measured for BMD, HAP-iodine, and Ca-iodine density values by two blinded radiologists (with 6 and 10 years of clinical experience in musculoskeletal diagnosis, respectively). Following an interval of 3 months, repeat measurements were conducted by less experienced doctors in order to evaluate the intraobserver agreement. If the agreement was good, the remaining individuals were measured by less experienced doctors under the supervision of experienced doctors.
Statistical analysis
The data were analyzed using SPSS software version 24.0 (IBM Corp., Armonk, NY, USA) and MedCalc software version 20.2 (MedCalc Software Ltd., Ostend, Belgium). The normality of data was tested using the Kolmogorov-Smirnov test, and continuous data are presented as the mean ± standard deviation. The intra- and interobserver agreements were analyzed using the intraclass correlation coefficient (ICC) (≤0.40, fair; 0.41–0.60, moderate; 0.61–0.80, good; ≥0.81; excellent). One-way analysis of variance (ANOVA) was employed to compare differences in HAP-iodine and Ca-iodine densities of the L1–L3 vertebral bodies among the three enhanced phases. Pearson correlation analysis was used to assess the relationship between the iodine intake and HAP or Ca densities on enhanced phases and between the BMD and HAP or Ca densities. Furthermore, linear regression was used for quantitative evaluation. The root mean square error (RMSE) of HAP-iodine and Ca-iodine linear equations was calculated. Bland-Altman analysis was conducted to assess the agreement between calculated BMD derived from DECT (BMD-DECT) and reference BMD derived from QCT (BMD-QCT). Receiver operating characteristic (ROC) analysis was performed to evaluate the efficacy of HAP-iodine and Ca-iodine densities in assessing BMD. The DeLong test was used to assess the diagnostic performance of HAP and Ca density measurements for osteoporosis and osteopenia. A two-sided P value of less than 0.05 was considered statistically significant.
Results
Patient population
The population enrolled 346 consecutive patients, with 216 males (age range, 40–87 years; mean age 63.53±8.59 years) and 130 females (age range, 32–86 years; mean age 62.63±9.78 years). According to the clinical diagnostic criteria, 82 patients had osteoporosis, 154 patients had osteopenia, and 110 patients had a normal BMD (Table 1).
Table 1
Characteristics | Values |
---|---|
Age (years), mean ± SD [range] | 63.20±9.04 [32–87] |
Gender, n (%) | |
Female | 130 (37.57) |
Male | 216 (62.43) |
Height (cm), mean ± SD | 168.42±7.71 |
BMI (kg/m2), mean ± SD | 23.17±3.27 |
Bone status, n | |
Osteoporosis | 82 |
Osteopenia | 154 |
Normal BMD | 110 |
SD, standard deviation; BMI, body mass index; BMD, bone mineral density.
The difference in HAP-iodine and Ca-iodine of the L1–L3 vertebrae and their relationship with contrast media intake
The BMD, HAP-iodine density, and Ca-iodine density value measurements showed excellent interobserver and intraobserver agreements (Table 2). The HAP-iodine and Ca-iodine density values of the L1–L3 vertebral bodies were normally distributed in the triphasic enhanced CT, and the differences were not statistically significant (F=0.001–0.049; P>0.05) (Table 3). Pearson correlation analysis showed that there was no correlation between the HAP-iodine or Ca-iodine densities and contrast media intake in the three contrast-enhanced phases (r=0.090–0.104; all P>0.05). Detailed results for this section are presented in Table 4. Additionally, the results showed significant differences in HAP-iodine and Ca-iodine densities in the L1–L3 vertebral bodies, demonstrating a gradually decreasing trend (Table 5).
Table 2
Contrast-enhanced CT | Intraobserver agreement | Interobserver agreement | |||||
---|---|---|---|---|---|---|---|
L1 | L2 | L3 | L1 | L2 | L3 | ||
Arterial phase | |||||||
HAP-iodine | 0.982 | 0.980 | 0.985 | 0.985 | 0.990 | 0.992 | |
Ca-iodine | 0.983 | 0.981 | 0.985 | 0.985 | 0.990 | 0.992 | |
Portal venous phase | |||||||
HAP-iodine | 0.983 | 0.983 | 0.984 | 0.984 | 0.990 | 0.991 | |
Ca-iodine | 0.983 | 0.983 | 0.984 | 0.984 | 0.990 | 0.991 | |
Delayed phase | |||||||
HAP-iodine | 0.925 | 0.983 | 0.985 | 0.981 | 0.991 | 0.993 | |
Ca-iodine | 0.934 | 0.983 | 0.985 | 0.944 | 0.991 | 0.993 | |
BMD | 0.992 | 0.995 | 0.994 | 0.993 | 0.996 | 0.995 |
BMD, bone mineral density; HAP, hydroxyapatite; Ca, calcium.
Table 3
Contrast-enhanced CT | HAP-iodine (2 mg/cm3) | Ca-iodine (2 mg/cm3) | |||||
---|---|---|---|---|---|---|---|
L1 | L2 | L3 | L1 | L2 | L3 | ||
Arterial phase | 688.62±17.53 | 685.49±16.83 | 680.62±17.03 | 841.05±21.40 | 837.24±20.47 | 831.24±20.76 | |
Portal venous phase | 688.59±17.48 | 685.52±16.78 | 680.63±17.02 | 840.81±21.38 | 837.13±20.39 | 831.16±20.73 | |
Delayed phase | 688.61±17.49 | 685.52±16.83 | 680.56±16.98 | 840.92±21.44 | 837.10±20.41 | 830.78±20.76 | |
F value | 0.001 | 0.001 | 0.002 | 0.011 | 0.004 | 0.049 | |
P value | 0.999 | 0.999 | 0.998 | 0.989 | 0.996 | 0.952 |
The values are continuous data and are presented as the mean ± standard deviation. The F value is from one-way analysis of variance. CT, computed tomography; HAP, hydroxyapatite; Ca, calcium.
Table 4
Contrast-enhanced CT | Density value | L1 | L2 | L3 | |||||
---|---|---|---|---|---|---|---|---|---|
r | P | r | P | r | P | ||||
Arterial phase | HAP-iodine | 0.092 | 0.088 | 0.096 | 0.076 | 0.092 | 0.087 | ||
Ca-iodine | 0.090 | 0.094 | 0.091 | 0.090 | 0.090 | 0.094 | |||
Portal venous phase | HAP-iodine | 0.096 | 0.075 | 0.095 | 0.079 | 0.090 | 0.096 | ||
Ca-iodine | 0.094 | 0.079 | 0.093 | 0.084 | 0.093 | 0.086 | |||
Delayed phase | HAP-iodine | 0.098 | 0.068 | 0.094 | 0.082 | 0.092 | 0.088 | ||
Ca-iodine | 0.100 | 0.063 | 0.099 | 0.066 | 0.104 | 0.053 |
HAP, hydroxyapatite; Ca, calcium; CT, computed tomography.
Table 5
Measurement | L1 | L2 | L3 | F | P |
---|---|---|---|---|---|
BMD (mg/cm3) | 111.45±38.08 | 106.53±37.83 | 99.18±37.63 | 9.211 | <0.001 |
HAP-iodine (2 mg/cm3) | 688.61±17.49 | 685.51±16.80 | 680.60±17.00 | 19.27 | <0.001 |
Ca-iodine (2 mg/cm3) | 840.92±21.38 | 837.16±20.40 | 831.06±20.60 | 19.829 | <0.001 |
Data conform to a normal distribution and are presented as the mean ± standard deviation. BMD, bone mineral density; HAP, hydroxyapatite; Ca, calcium.
The relationship and equation of linear regression between HAP-iodine, Ca-iodine, and BMD
Strong positive correlations were found between the HAP-iodine and Ca-iodine densities and BMD (HAP-BMD: r=0.9472, R2=0.8973; Ca-BMD: r=0.9470, R2=0.8968; all P<0.001). The linear regression equation is shown in Figure 3. The overall RMSEs of the HAP-iodine and Ca-iodine linear regressions were 8.01 and 8.10 mg/cm3, respectively. In addition, the Bland-Altman plots exhibited a high agreement between BMD-DECT and BMD-QCT, as shown in Figure 4.
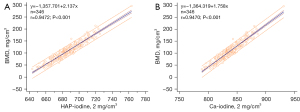
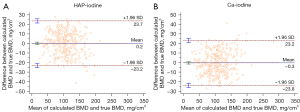
Performance of HAP-iodine and Ca-iodine for bone status assessment
For the diagnosis of osteoporosis, the area under the curve (AUC) values of using HAP-iodine density and Ca-iodine density were 0.963 [95% confidence interval (CI): 0.937–0.980] and 0.964 (95% CI: 0.939–0.981), respectively. The cutoff values of HAP-iodine and Ca-iodine were 679.29 (2 mg/cm3) and 829.56 (2 mg/cm3), respectively; the sensitivity was 100% for both, and the specificity was 83.02% and 82.64%, respectively.
For the diagnosis of osteopenia, the AUC of using HAP-iodine density and Ca-iodine density was 0.951 (95% CI: 0.917–0.973) and 0.950 (95% CI: 0.916–0.973), respectively. As illustrated in Figure 5, the HAP-iodine and Ca-iodine cutoff values were 694.82 (2 mg/cm3) and 847.23 (2 mg/cm3), respectively; the sensitivity was 98.70% and 95.45%, respectively; and the specificity was 77.27% and 79.09%, respectively.
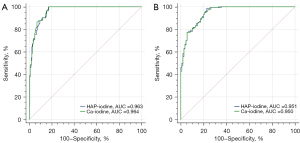
There was no significant difference in the AUC values for diagnosing osteoporosis and osteopenia between the use of HAP and Ca density measurements according to the DeLong test (P=0.1770, 0.3683).
Discussion
In this study, we explored the feasibility of opportunistic screening for osteoporosis using enhanced CT based on material decomposition technique in fast-switching DECT. We found that there were no statistically significant differences in HAP-iodine and Ca-iodine density values of L1–L3 vertebral bodies among the triphasic enhanced CT. Strong positive correlations were found between HAP-iodine and BMD (r=0.9472), and between Ca-iodine and BMD (r=0.9470). Furthermore, Bland-Altman plots also indicated good agreement between the calculated BMD-DECT and reference BMD-QCT. The HAP (on HAP-iodine images) and Ca (on Ca-iodine images) density measurements generated through the material decomposition technique in DECT have good diagnostic performances in assessing BMD and diagnosing osteoporosis.
In clinical practice, many patients with malignant diseases require regular contrast-enhanced CT scans for disease follow-up, staging, and efficacy evaluation. There is an increasing importance being placed on using existing imaging to achieve the opportunistic diagnosis of osteoporosis, as it can reduce financial burden, minimize, radiation exposure, and improve clinical work efficiency. Some studies have been conducted to this end. Pickhardt et al. (16) demonstrated that an L1 vertebral CT attenuation threshold of 110 HU had a specificity of over 90% for diagnosing osteoporosis in 1,867 patients. Buenger et al. (22) found a strong correlation between CT value and BMD and established a linear equation for transforming CT values to BMD. However, the CT attenuation value of CT can easily be affected by scanning parameters such as tube voltage (23) and trabecular bone composition in the vertebral body (24). Wang et al. (25) compared the differences between BMD measured with QCT and the density values of base-material pairs including HAP-water and HAP-fat based on DECT. The results indicated that the BMD obtained with the 2 methods were highly correlated and not affected by radiation dose.
However, due to the interference of contrast media in patients undergoing enhanced CT scanning, only a few studies have evaluated the effect of contrast media on BMD measurement. Pompe et al. (26) compared the CT value differences of the L1 vertebra between unenhanced and enhanced CT scans. The results demonstrated that CT values of enhanced CT were significantly higher than those of unenhanced CT. When a CT threshold is used to determine an osteoporosis diagnosis, there is a false negative rate of 7% to 25%. Roski et al. (14) discovered that all BMD values obtained from enhanced scans were higher than those from standard BMD. For the iodine concentration in the arterial and venous phases of the blood vessels, these authors employed a conversion equation. The resulting translated BMD showed good agreement with the standard BMD. In another study, a third-generation dual-source DECT was used to evaluate BMD via specialist postprocessing software based on material decomposition. There was no significant difference found between the enhanced portal venous phase and the unenhanced CT (27).
For assessing BMD using existing enhanced data, the influence of iodine contrast agents has to be excluded, which was the aim of this study. To date, how accurately the material decomposition algorithm based on fast-switching DECT measures BMD on enhanced CT remains unclear. The main components of the vertebral body include large amounts of bone minerals (Ca and HAP) as well as other components such as red bone marrow, yellow bone marrow (mainly fat), collagen, and water (28). Ca typically refers to elemental Ca, which does not contain other ions or elements, while HAP is a specific Ca phosphate compound with a stoichiometric formula of Ca10(PO4)6(OH)2, which is composed of Ca, phosphorous, and hydroxyl ions. HAP is the main inorganic component of natural bone tissue (29). Changes in bone density depend not only on Ca content, but also on the content and distribution of other organic and inorganic components within the bone. HAP is thought to better reflect BMD information. However, the inquiry into whether the relative content of Ca obtained through the application of material separation techniques is capable of effectively reflecting bone density information represents an issue worth investigating. Therefore, in this study, the HAP (on HAP-iodine images) and Ca (on Ca-iodine images) density values were used to assess the feasibility of opportunistic screening for osteoporosis on enhanced CT. We enrolled patients who underwent both abdominal unenhanced and contrast-enhanced scans. During the unenhanced scan, a QCT examination and standard clinical CT examination were combined to obtain images for BMD assessment and image diagnostics. Fast-switching DECT is capable of material characterization and decomposition based on attenuation coefficient, which is conducive to the selective extraction of iodine contrast media and quantitative evaluation (30). The material decomposition technique combines two different base materials to produce an X-ray attenuation equivalent to the target material for approximately estimating the concentration of the material (31,32). Of note, it can only reflect the relative content and trend of a certain material and not its true content. The more similar the base material pair is to the target substance, the more accurately the measurement of its content will be (33). The results of this study showed that there was no significant difference in the density values of HAP-iodine and those of Ca-iodine among the triphasic enhanced CT. Meanwhile, there was no correlation between HAP-iodine, Ca-iodine, or contrast media intake in the triphasic enhanced CT. The density values of HAP-iodine and Ca-iodine were not affected by the administration of iodine contrast media, which is a significant indicator effect.
Strong positive correlations were found between HAP-iodine or Ca-iodine density values and BMD measured with QCT. Consequently, we established two simple linear regression equations and calculated the RMSE. The RMSE is a more appropriate measure for assessing line regression equations. Rühling et al. (34) used deep learning networks to correct for the effect of contrast agents in enhanced scans. Their RMSE in the venous phase was 9.45 mg/mL, and the RMSE in the arterial phase was 3.98 mg/mL, indicating high accuracy. Sollmann et al. obtained opportunistic BMD data from routine CT scans, with a root mean square coefficient of variation of 14.4% when compared to specialized QCT (35). They used deep learning networks to calibrate BMD. In our study, the overall RMSEs of the HAP-iodine and Ca-iodine linear regressions were 8.01 and 8.10 mg/cm3, respectively, which are within an acceptable error range. We used only the relative Ca and HAP content obtained from the enhanced images with material decomposition technique and then used a simple linear equation to reflect the relationship between this and the specialized BMD, which provided higher cost-effectiveness. Given that this study had a small sample size, obtaining an accurate estimation of precision error would have required a considerable number of repeated measurements or a large number of patients to achieve a reasonable margin of error. Therefore, Bland-Altman analysis was used to assess the agreement. The Bland-Altman plots exhibited a high agreement between BMD-DECT and BMD-QCT, which is consistent with the result of Sollmann et al. (35).
The ROC curve analysis revealed that the AUC values in diagnosing osteoporosis for HAP-iodine and Ca-iodine were greater than 0.95 and the difference between the two density measurements was not statistically significant when tested using the DeLong test. We speculate that this may be due to the fact that HAP and Ca constitute the majority of the vertebral bodies’ composition. Since HAP contains 10 Ca atoms, we can determine the concentration of another material if we know the concentration of Ca or HAP, and this may explain the lower density of HAP-iodine compared to that of Ca-iodine. Zhou et al. (32) demonstrated in vivo that fast-switching DECT can accurately evaluate phantomless BMD, and Ca-fat and HAP-fat have similar and optimal predictive abilities, which is consistent with our findings. Wang et al. (36) also reported a highly positive correlation between BMD assessment derived from new fast-switching DECT and QCT (R2=0.912) and found that the relative error of the new fast-switching DECT was smaller than that of QCT. In addition, the consensus for applying QCT recommends collecting two whole vertebral bodies in the T12–L3 range, typically the L1–L2 vertebral bodies (37,38). We made a more expansive choice of the L1–L3 vertebral bodies for this study. The middle region of the lumbar spine, or the L3 vertebra, can accurately and completely reflect changes in the bone mass of the lumbar spines and even the whole body. When the L1–L2 vertebrae are injured, adjacent vertebrae may be used as replacements.
Furthermore, we found that BMD values gradually decreased from L1 to L3, which is consistent with the study of Rühling et al. (39). Hagen et al. employed virtual non-Ca technique to analyze the attenuation information of vertebral bone marrow. The findings indicated a progressive elevation in the attenuation information of bone marrow from the T11 to L1 vertebrae (40). Osteoporosis is characterized by a reduction in BMD and the accumulation of adipose tissue in the bone marrow. There was a negative correlation between bone marrow fat content and BMD in our study. The bone density content varies among different anatomical regions and is closely related to the nutritional supply of bone from the red and yellow marrow. A physiological distribution pattern of adult bone marrow may already exist at the age of 25 years. As age increases, red bone marrow is gradually replaced by yellow bone marrow (adipose tissue).
Some limitations of the study should be acknowledged. First, we only used a single contrast media protocol and did not investigate how different contrast media protocols affect the material decomposition algorithm. Nevertheless, the stability of HAP-iodine and Ca-iodine in triphasic enhanced CT indicates that the above parameters should not affect the material decomposition algorithm. Second, we employed a single-center study design, and our findings may only apply to a specific vendor’s CT system. Material decomposition algorithms based on a variety of parameters should be investigated further.
Conclusions
The HAP (on HAP-iodine images) and Ca (on Ca-iodine images) density measurements generated through the material decomposition technique in DECT had good diagnostic performance in assessing BMD, which offers a new perspective for the opportunistic screening of osteoporosis on contrast-enhanced CT.
Acknowledgments
The authors thank Dr. Jianying Li (CT Research Center, GE Healthcare, Dalian, China) for proofreading the manuscript and providing suggestions.
Funding: None.
Footnote
Reporting Checklist: The authors have completed the STARD reporting checklist. Available at https://qims.amegroups.com/article/view/10.21037/qims-23-855/rc
Conflicts of Interest: All authors have completed the ICMJE uniform disclosure form (available at https://qims.amegroups.com/article/view/10.21037/qims-23-855/coif). The authors have no conflicts of interest to declare.
Ethical Statement: The authors are accountable for all aspects of the work in ensuring that questions related to the accuracy or integrity of any part of the work are appropriately investigated and resolved. This study was conducted in accordance with the Declaration of Helsinki (as revised in 2013) and was approved by the Ethics Committee of First Affiliated Hospital of Dalian Medical University. All patients provided signed informed consent.
Open Access Statement: This is an Open Access article distributed in accordance with the Creative Commons Attribution-NonCommercial-NoDerivs 4.0 International License (CC BY-NC-ND 4.0), which permits the non-commercial replication and distribution of the article with the strict proviso that no changes or edits are made and the original work is properly cited (including links to both the formal publication through the relevant DOI and the license). See: https://creativecommons.org/licenses/by-nc-nd/4.0/.
References
- Gudmundsson HT, Hansen KE, Halldorsson BV, Ludviksson BR, Gudbjornsson B. Clinical decision support system for the management of osteoporosis compared to NOGG guidelines and an osteology specialist: a validation pilot study. BMC Med Inform Decis Mak 2019;19:27. [Crossref] [PubMed]
- Rashki Kemmak A, Rezapour A, Jahangiri R, Nikjoo S, Farabi H, Soleimanpour S. Economic burden of osteoporosis in the world: A systematic review. Med J Islam Repub Iran 2020;34:154. [Crossref] [PubMed]
- Pennestrì F, Corbetta S, Favero V, Banfi G. Fragility Fracture Prevention-Implementing a Fracture Liaison Service in a High Volume Orthopedic Hospital. Int J Environ Res Public Health 2019;16:4902. [Crossref] [PubMed]
- Chen P, Li Z, Hu Y. Prevalence of osteoporosis in China: a meta-analysis and systematic review. BMC Public Health 2016;16:1039. [Crossref] [PubMed]
- Leslie WD, Giangregorio LM, Yogendran M, Azimaee M, Morin S, Metge C, Caetano P, Lix LM. A population-based analysis of the post-fracture care gap 1996-2008: the situation is not improving. Osteoporos Int 2012;23:1623-9. [Crossref] [PubMed]
- Pasco JA, Seeman E, Henry MJ, Merriman EN, Nicholson GC, Kotowicz MA. The population burden of fractures originates in women with osteopenia, not osteoporosis. Osteoporos Int 2006;17:1404-9. [Crossref] [PubMed]
- Hernlund E, Svedbom A, Ivergård M, Compston J, Cooper C, Stenmark J, McCloskey EV, Jönsson B, Kanis JA. Osteoporosis in the European Union: medical management, epidemiology and economic burden. A report prepared in collaboration with the International Osteoporosis Foundation (IOF) and the European Federation of Pharmaceutical Industry Associations (EFPIA). Arch Osteoporos 2013;8:136. [Crossref] [PubMed]
- Koch V, Hokamp NG, Albrecht MH, Gruenewald LD, Yel I, Borggrefe J, et al. Accuracy and precision of volumetric bone mineral density assessment using dual-source dual-energy versus quantitative CT: a phantom study. Eur Radiol Exp 2021;5:43. [Crossref] [PubMed]
- Löffler MT, Jacob A, Valentinitsch A, Rienmüller A, Zimmer C, Ryang YM, Baum T, Kirschke JS. Improved prediction of incident vertebral fractures using opportunistic QCT compared to DXA. Eur Radiol 2019;29:4980-9. [Crossref] [PubMed]
- Li N, Li XM, Xu L, Sun WJ, Cheng XG, Tian W. Comparison of QCT and DXA: Osteoporosis Detection Rates in Postmenopausal Women. Int J Endocrinol 2013;2013:895474. [Crossref] [PubMed]
- Shuhart CR, Yeap SS, Anderson PA, Jankowski LG, Lewiecki EM, Morse LR, Rosen HN, Weber DR, Zemel BS, Shepherd JA. Executive Summary of the 2019 ISCD Position Development Conference on Monitoring Treatment, DXA Cross-calibration and Least Significant Change, Spinal Cord Injury, Peri-prosthetic and Orthopedic Bone Health, Transgender Medicine, and Pediatrics. J Clin Densitom 2019;22:453-71. [Crossref] [PubMed]
- Bosch de Basea M, Salotti JA, Pearce MS, Muchart J, Riera L, Barber I, Pedraza S, Pardina M, Capdevila A, Espinosa A, Cardis E. Trends and patterns in the use of computed tomography in children and young adults in Catalonia - results from the EPI-CT study. Pediatr Radiol 2016;46:119-29. [Crossref] [PubMed]
- Areeckal AS, Kocher M, S SD. Current and Emerging Diagnostic Imaging-Based Techniques for Assessment of Osteoporosis and Fracture Risk. IEEE Rev Biomed Eng 2019;12:254-68. [Crossref] [PubMed]
- Roski F, Hammel J, Mei K, Haller B, Baum T, Kirschke JS, Pfeiffer D, Woertler K, Pfeiffer F, Noël PB, Gersing AS, Schwaiger BJ. Opportunistic osteoporosis screening: contrast-enhanced dual-layer spectral CT provides accurate measurements of vertebral bone mineral density. Eur Radiol 2021;31:3147-55. [Crossref] [PubMed]
- Li YL, Wong KH, Law MW, Fang BX, Lau VW, Vardhanabuti VV, Lee VK, Cheng AK, Ho WY, Lam WW. Opportunistic screening for osteoporosis in abdominal computed tomography for Chinese population. Arch Osteoporos 2018;13:76. [Crossref] [PubMed]
- Pickhardt PJ, Pooler BD, Lauder T, del Rio AM, Bruce RJ, Binkley N. Opportunistic screening for osteoporosis using abdominal computed tomography scans obtained for other indications. Ann Intern Med 2013;158:588-95. [Crossref] [PubMed]
- Pickhardt PJ, Lauder T, Pooler BD, Muñoz Del Rio A, Rosas H, Bruce RJ, Binkley N. Effect of IV contrast on lumbar trabecular attenuation at routine abdominal CT: correlation with DXA and implications for opportunistic osteoporosis screening. Osteoporos Int 2016;27:147-52. [Crossref] [PubMed]
- Abdullayev N, Neuhaus VF, Bratke G, Voss S, Große Hokamp N, Hellmich M, Krug B, Maintz D, Borggrefe J. Effects of Contrast Enhancement on In-Body Calibrated Phantomless Bone Mineral Density Measurements in Computed Tomography. J Clin Densitom 2018;21:360-6. [Crossref] [PubMed]
- Booz C, Noeske J, Albrecht MH, Lenga L, Martin SS, Yel I, Huizinga NA, Vogl TJ, Wichmann JL. Diagnostic accuracy of quantitative dual-energy CT-based bone mineral density assessment in comparison to Hounsfield unit measurements using dual x-ray absorptiometry as standard of reference. Eur J Radiol 2020;132:109321. [Crossref] [PubMed]
- Li X, Li X, Li J, Jiao X, Jia X, Zhang X, Fan G, Yang J, Guo J. The accuracy of bone mineral density measurement using dual-energy spectral CT and quantitative CT: a comparative phantom study. Clin Radiol 2020;75:320.e9-320.e15. [Crossref] [PubMed]
- American College of Radiology 2018. ACR–SPR–SSR practice parameter for the performance of musculoskeletal quantitative computed tomography (QCT). American College of Radiology, Reston. Available online: https://www.acr.org/-/media/ACR/Files/Practice-Parameters/QCT.pdf
- Buenger F, Eckardt N, Sakr Y, Senft C, Schwarz F. Correlation of Bone Density Values of Quantitative Computed Tomography and Hounsfield Units Measured in Native Computed Tomography in 902 Vertebral Bodies. World Neurosurg 2021;151:e599-606. [Crossref] [PubMed]
- Garner HW, Paturzo MM, Gaudier G, Pickhardt PJ, Wessell DE. Variation in Attenuation in L1 Trabecular Bone at Different Tube Voltages: Caution Is Warranted When Screening for Osteoporosis With the Use of Opportunistic CT. AJR Am J Roentgenol 2017;208:165-70. [Crossref] [PubMed]
- Mazess RB. Errors in measuring trabecular bone by computed tomography due to marrow and bone composition. Calcif Tissue Int 1983;35:148-52. [Crossref] [PubMed]
- Wang M, Wu Y, Zhou Y, Dong J, Hu S, Hou P, Gao J. Application of Dual-Energy Spectral Computed Tomography in Bone Mineral Density Measurement: Phantom and Clinical Research. Int J Gen Med 2022;15:6887-96. [Crossref] [PubMed]
- Pompe E, Willemink MJ, Dijkhuis GR, Verhaar HJ, Mohamed Hoesein FA, de Jong PA. Intravenous contrast injection significantly affects bone mineral density measured on CT. Eur Radiol 2015;25:283-9. [Crossref] [PubMed]
- Koch V, Albrecht MH, Gruenewald LD, Yel I, Eichler K, Gruber-Rouh T, Hammerstingl RM, Burck I, Wichmann JL, Alizadeh LS, Vogl TJ, Lenga L, Wesarg S, Martin SS, Mader C, Dimitrova M, D'Angelo T, Booz C. Impact of Intravenously Injected Contrast Agent on Bone Mineral Density Measurement in Dual-Source Dual-Energy CT. Acad Radiol 2022;29:880-7. [Crossref] [PubMed]
- Gruenewald LD, Koch V, Martin SS, Yel I, Eichler K, Gruber-Rouh T, Lenga L, Wichmann JL, Alizadeh LS, Albrecht MH, Mader C, Huizinga NA, D'Angelo T, Mazziotti S, Wesarg S, Vogl TJ, Booz C. Diagnostic accuracy of quantitative dual-energy CT-based volumetric bone mineral density assessment for the prediction of osteoporosis-associated fractures. Eur Radiol 2022;32:3076-84. [Crossref] [PubMed]
- Sinulingga K, Sirait M, Siregar N, Abdullah H. Synthesis and characterizations of natural limestone-derived nano-hydroxyapatite (HAp): a comparison study of different metals doped HAps on antibacterial activity. RSC Adv 2021;11:15896-904. [Crossref] [PubMed]
- Xu Y, Yan B, Zhang J, Chen J, Zeng L, Wang L. Image Decomposition Algorithm for Dual-Energy Computed Tomography via Fully Convolutional Network. Comput Math Methods Med 2018;2018:2527516. [Crossref] [PubMed]
- Shinohara Y, Sasaki F, Ohmura T, Itoh T, Endo T, Kinoshita T. Evaluation of lumbar intervertebral disc degeneration using dual energy CT virtual non-calcium imaging. Eur J Radiol 2020;124:108817. [Crossref] [PubMed]
- Zhou S, Zhu L, You T, Li P, Shen H, He Y, Gao H, Yan L, He Z, Guo Y, Zhang Y, Zhang K. In vivo quantification of bone mineral density of lumbar vertebrae using fast kVp switching dual-energy CT: correlation with quantitative computed tomography. Quant Imaging Med Surg 2021;11:341-50. [Crossref] [PubMed]
- Diekhoff T, Engelhard N, Fuchs M, Pumberger M, Putzier M, Mews J, Makowski M, Hamm B, Hermann KA. Single-source dual-energy computed tomography for the assessment of bone marrow oedema in vertebral compression fractures: a prospective diagnostic accuracy study. Eur Radiol 2019;29:31-9. [Crossref] [PubMed]
- Rühling S, Navarro F, Sekuboyina A, El Husseini M, Baum T, Menze B, Braren R, Zimmer C, Kirschke JS. Automated detection of the contrast phase in MDCT by an artificial neural network improves the accuracy of opportunistic bone mineral density measurements. Eur Radiol 2022;32:1465-74. [Crossref] [PubMed]
- Sollmann N, Löffler MT, El Husseini M, Sekuboyina A, Dieckmeyer M, Rühling S, Zimmer C, Menze B, Joseph GB, Baum T, Kirschke JS. Automated Opportunistic Osteoporosis Screening in Routine Computed Tomography of the Spine: Comparison With Dedicated Quantitative CT. J Bone Miner Res 2022;37:1287-96. [Crossref] [PubMed]
- Wang M, Wu Y, Zhou Y, Dong J, Hou P, Gao J. The new fast kilovoltage-switching dual-energy computed tomography for measuring bone mineral density. Quant Imaging Med Surg 2023;13:801-11. [Crossref] [PubMed]
- Engelke K, Adams JE, Armbrecht G, Augat P, Bogado CE, Bouxsein ML, Felsenberg D, Ito M, Prevrhal S, Hans DB, Lewiecki EM. Clinical use of quantitative computed tomography and peripheral quantitative computed tomography in the management of osteoporosis in adults: the 2007 ISCD Official Positions. J Clin Densitom 2008;11:123-62. [Crossref] [PubMed]
- Shepherd JA, Schousboe JT, Broy SB, Engelke K, Leslie WD. Executive Summary of the 2015 ISCD Position Development Conference on Advanced Measures From DXA and QCT: Fracture Prediction Beyond BMD. J Clin Densitom 2015;18:274-86. [Crossref] [PubMed]
- Rühling S, Scharr A, Sollmann N, Wostrack M, Löffler MT, Menze B, Sekuboyina A, El Husseini M, Braren R, Zimmer C, Kirschke JS. Proposed diagnostic volumetric bone mineral density thresholds for osteoporosis and osteopenia at the cervicothoracic spine in correlation to the lumbar spine. Eur Radiol 2022;32:6207-14. [Crossref] [PubMed]
- Hagen F, Fritz J, Mair A, Horger M, Bongers MN. Dual-Energy Computed Tomography-Based Quantitative Bone Marrow Imaging in Non-Hematooncological Subjects: Associations with Age, Gender and Other Variables. J Clin Med 2022;11:4094. [Crossref] [PubMed]