Radiomics for predicting survival in patients with locally advanced rectal cancer: a systematic review and meta-analysis
Introduction
Colorectal cancer (CRC) is the third most common and second deadliest cancer worldwide (1). Over one-third of CRCs are located in the rectum, and more than 70% of cases are diagnosed as locally advanced rectal cancer (LARC). Total mesorectal excision (TME) after neoadjuvant chemoradiotherapy (nCRT) has become the standard treatment for patients with LARC (2). This therapeutic strategy has reduced the local recurrence rate of rectal cancer patients, but the 5-year survival rates remain low. Therefore, to improve the long-term prognosis of patients with LARC, it is crucial that adverse prognostic factors are accurately identified (3).
Tumor-node-metastasis (TNM) staging is a key part of prognostic assessment and risk stratification, but it lacks precision (4,5). In the current TNM staging system, the inclusion of tumor deposits (TDs) within nodal staging has given rise to worldwide discussions (6-8). Other significant prognostic factors, such as circumferential resection margin (CRM) and extramural vascular invasion (EMVI), are prone to subjective factors, making prognosis prediction less reliable (9,10). As a result, a more accurate survival estimation that considers each patient’s unique circumstances is needed.
The growing field of radiomics has the potential to provide noninvasive imaging biomarkers for tumor aggressiveness that may be utilized preoperatively to guide treatment decisions. Radiomics involves the extraction of high-throughput features from conventional images to build high-dimensional datasets, which are then mined for features related to molecular tumor typing, treatment response, and clinical outcomes to promote accurate tumor diagnosis (11). Mounting evidence suggests that radiomics could play an important role in evaluating tumor development and progression in various types of cancers. A recent meta-analysis indicated that radiomics shows good prognostic performance in patients with nasopharyngeal carcinoma (12). Another meta-analysis supported a similar conclusion that radiomics-based models offered modest prognostic capabilities for predicting survival in non-small cell lung cancer (13). Recent studies have suggested a potential prognostic role of radiomics in LARC patients as well (14-18). Therefore, the purpose of this study was to analyze the current status of radiomics studies used to predict survival outcomes in patients with LARC and to evaluate the quality of radiomics studies by using the radiomics quality score (RQS) tool, the Transparent Reporting of a Multivariable Prediction Model for Individual Prognosis or Diagnosis (TRIPOD) statement, the Image Biomarkers Standardization Initiative (IBSI) guideline, and the Prediction Model Risk of Bias Assessment Tool (PROBAST) (19-22). In addition, quantitative analysis was used to assess the role of radiomics in predicting disease-free survival (DFS) and overall survival (OS) outcomes in patients with LARC. We present this article in accordance with the PRISMA reporting checklist (available at https://qims.amegroups.com/article/view/10.21037/qims-23-692/rc) (23).
Methods
Protocol and registration
The review protocol was registered on the Prospective Register of Systematic Reviews (PROSPERO; https://www.crd.york.ac.uk; registration number CRD42022342859).
Search strategy
A comprehensive search of the Cochrane, Embase, Medline, and Web of Science databases was conducted for studies published between 1 January 2012 and 30 June 2022. The search terms mainly included “rectal neoplasms”, “rectal cancer”, “radiomics”, “texture”, “prognosis”, and “survival”. The list of retrieved references was manually searched to identify additional eligible studies. Table S1 provides a full description of the search strategy.
Study selection
Studies were selected based on the following criteria: (I) patients had pathologically confirmed rectal cancer; (II) imaging was assessed using radiomics; (III) the main survival outcome was reported as DFS and/or OS; and (IV) hazard ratio (HR) values based on radiomics models were reported.
Studies were excluded based on the following criteria: (I) reviews, editorials, and conference summaries; (II) tumors other than rectal cancer; and (III) insufficient survival data for estimating performance measurement indices. Eligible studies were selected by two reviewers (Feng Y and Tong T) individually.
Data extraction
Data extraction and further statistical analysis were performed by two reviewers independently (Feng Y and Tong T). If there was a disagreement, the two reviewers discussed or reassessed the issue and reached a consensus. The following data were extracted: (I) study information: authors, publication year, country, median follow-up time, and study design (prospective or retrospective); (II) cohort information: number of overall participants, mean age or age range, sex, tumor stages, and treatment protocols; (III) information on radiomic models: imaging modality, software, segmentation, feature selection, and numbers and categories of radiomic features; and (IV) clinical outcomes and HRs with 95% confidence intervals (95% CIs).
Quality assessment
The included studies’ methodology was evaluated using the RQS (19,24), which comprises 16 items assessing crucial aspects of radiomics study methodology. The scoring of the specific RQS items was based on a previous report (19). In addition, the reporting completeness of the included prediction models was determined using the TRIPOD statement (20). Several modifications needed to be made to the TRIPOD statement before it could be utilized in radiomics studies, as it had originally been designed for clinical prediction models. Items 21 and 22 related to funding and supplemental materials were excluded. In addition, when calculating overall adherence rates, “if completed” or “if relevant” items (5c, 11, and 14b) and validation items (10c, 10e, 12, 13c, 17, and 19a) were excluded from both the numerator and denominator, as reported previously (25-29). The IBSI guideline provides a comprehensive and unified reporting checklist for radiomics studies (21). Since many items in the IBSI checklist overlap with those in the RQS or TRIPOD checklists, we included only the items relevant to image pre-processing steps, as indicated in Table S2. Finally, the bias risk in the included studies was assessed using the PROBAST, which assesses bias risk in four domains (participants, predictors, outcomes, and analysis) and applicability in three domains (participants, predictors, and outcomes) (22). Based on a comprehensive evaluation, the included studies were categorized into three groups: high, low, and unclear risk of bias (ROB) and applicability. The quality assessment was performed independently by two reviewers (Feng Y and Gong J). If a disagreement occurred, a final decision was made with the assistance of a third reviewer (Tong T). The mean score, percentage of the ideal RQS score, detailed checklist of the TRIPOD statement adherence rate of IBSI, and rate of ROB were calculated and recorded.
Meta-analysis
The HR is a common metric for evaluating time-to-event data. Therefore, the HRs and 95% CIs of the radiomics models regarding DFS and/or OS were extracted for further meta-analysis. When HRs were not recorded, calculations were performed using Engauge Digitizer (Version 12.1; http://markummitchell.github.io/engauge-digitizer/) based on Kaplan-Meier curves. The forest plot figures presented the pooled HR and its 95% CI. When significant heterogeneity was observed, a random-effects model was used; otherwise, a fixed-effects model was used (30). Cochran’s Q test and Higgins I2 statistic were employed to assess heterogeneity. An I2 value of ≤25% indicated insignificant heterogeneity, whereas an I2 of >25% to ≤50% indicated low heterogeneity, I2>50% to ≤75% indicated moderate heterogeneity, and I2>75% signified significant heterogeneity (31). Subgroup analysis was applied to explore the origin of heterogeneity. For results containing more than ten studies, publication bias was assessed using a funnel plot and Egger’s test, as <10 studies could lead to bias in the interpretation of the funnel plot (32). A 2-sided P<0.05 was considered statistically significant. All these data analysis processes were performed by using the statistical software R (version 4.1.0; R Foundation for Statistical Computing, Vienna, Austria).
Results
Literature search
A flowchart of the research selection procedure is shown in Figure 1. A total of 358 studies were identified during the first search process cycle. In the end, 232 studies remained after removal of all duplicates. When abstracts and titles were considered, 215 studies were excluded. After reviewing each manuscript in detail, we eliminated an additional two articles because they lacked survival data. Finally, only 15 studies met the criteria for statistical analysis (14-16,18,33-43).
Study characteristics
A total of 15 studies, including 2,151 patients overall, that had applied radiomics methods to predict patient survival status were selected in our review. All the studies were retrospective. Only 1 study was from multiple centers (36), and the others were all from a single center. In addition, 8 of the studies established both development and validation sets (15,16,33-36,38,39), whereas the other 7 established only development sets (14,18,37,40-43). The number of patients included in the studies ranged from 48 to 411. In addition, the mean/median age ranged from 52.8 to 67, and the median follow-up time ranged from 27.2 to
60 months. All participants underwent nCRT. Other clinical characteristics are summarized in Table 1.
Table 1
First author | Year | Country | Study design | Single center | Number of patients (frequency) | Age (years) | Stage | Treatment | Outcome | Median follow-up (months) | |||||
---|---|---|---|---|---|---|---|---|---|---|---|---|---|---|---|
All | D | V | D | V | DFS | OS | |||||||||
Meng (34) | 2018 | China | R | Yes | 108 | 54 | 54 | 53.9±11.5* | 55.7±10.5* | II, III | nCRT + TME | DFS | 34.5 [11, 45]† | NA | |
Wang (38) | 2019 | China | R | Yes | 411 | 370 | 41 | NR | III | nCRT + TME | DFS, OS | NR | NR | ||
Cui (33) | 2021 | China | R | Yes | 186 | 131 | 53 | 54.2±10.4* | 52.8±11.4* | II, III | nCRT + TME + AC | DFS, OS | 43 [29, 43]† | NA | |
Tibermacine (36) | 2021 | France | R | No | 146 | 98 | 48 | 60 [21, 88]† | 58 [37, 78]† | II, III | nCRT + TME | DFS | 60 [21, 77]† | NA | |
Chiloiro (14) | 2022 | Italy | R | Yes | 48 | NA | NA | All: 62 [39, 87]† | II, III | nCRT + TME | DFS | 31 [4, 47]† | NA | ||
Chuanji (15) | 2022 | China | R | Yes | 206 | 146 | 60 | 59.7±11.52* | 58.42±12.06* | NR | TME + nCRT | OS | NA | 39 [1, 55]† | |
Cui (16) | 2022 | China | R | Yes | 234 | 164 | 70 | 58.10±9.64* | 55.81±10.86* | II, III | nCRT + TME + AC | DFS | 42 [6, 60]† | NA | |
Nie (35) | 2022 | China | R | Yes | 165 | 114 | 51 | All: 67±13* | II, III | nCRT + TME | OS | NA | 60–121§ | ||
Wang (37) | 2022 | China | R | Yes | 191 | NA | NA | All: 63 [28, 85]† | II, III | nCRT + TME | DFS, OS | 60 | 60 | ||
Meng (39) | 2018 [2] | China | R | Yes | 51 | 36 | 15 | All: 55±12* | II, III | nCRT + TME | DFS | NR | NA | ||
Bang (18) | 2016 | Korea | R | Yes | 74 | NA | NA | All: 58.8 [28, 82]† | II, III | nCRT + TME | DFS | 27.2 [10, 36]† | NA | ||
Chee (40) | 2017 | Korea | R | Yes | 95 | NA | NA | All: 61.1 [36, 85]† | II, III | nCRT | DFS | 54 [28, 75]† | NA | ||
Jalil (42) | 2017 | England | R | Yes | 56 | NA | NA | All: 64±8.8* | II, III | nCRT + TME | DFS, OS | 47.2±18.2* | 47.2±18.2* | ||
Lovinfosse (43) | 2018 | Belgium | R | Yes | 86 | NA | NA | All: 66±11* | II, III | nCRT + TME | DFS, OS | 41 [5, 75]† | 41 [5, 75]† | ||
Hotta (41) | 2021 | Japan | R | Yes | 94 | NA | NA | All: 65.3±12.4* | II, III | nCRT + TME | OS | NA | 41.7 [30.5, 60.4]† |
*, mean ± standard deviation; †, median [interquartile range]; §, range (from minimum to maximum). D, development set; V, validation set; DFS, disease-free survival; OS, overall survival; R, retrospective study; NA, not available; NR, not reported; nCRT, neoadjuvant chemoradiotherapy; TME, total mesorectal excision; AC, adjuvant chemotherapy.
Radiomics model metrics
Table 2 provides a summary of the radiomics model metrics of the included studies. In terms of imaging modalities, 9 (60.0%) studies used magnetic resonance imaging (MRI) (14-16,33-36,39,42), 3 (20.0%) used computed tomography (CT) (37,38,40), and 3 (20.0%) used positron emission tomography/computed tomography (PET/CT) (18,41,43). In MRI-based research, 8 studies employed T2-weighted imaging (T2WI), several employed mixed sequences, such as contrast T1-weighted imaging (T1WI), diffusion-weighted imaging (DWI), apparent diffusion coefficient (ADC) maps, and dynamic contrast enhanced MRI (DCE-MRI), and 1 employed true fast imaging with steady state precession (TrueFISP) (14). There were a variety of feature extraction and selection approaches. A total of 13 studies (86.7%) employed the 3-dimensional (3D) region of interest (ROI) segmentation method. Manual segmentation was performed in all studies. Furthermore, 11/15 (73.3%) studies were associated with first-order statistics (FOS), 10/15 (66.7%) with gray-level co-occurrence matrix (GLCM), and 8/15 (53.3%) with gray-level run-length matrix (GLRLM). Other higher-order features, such as gray-level size zone matrix (GLSZM) and neighboring gray-tone difference matrix (NGTDM), were rare, occupying 4/15 (26.7%) and 3/15 (20.0%) studies, respectively.
Table 2
First author | Year | Imaging modality | Segmentation | Feature extraction and selection | |||||||
---|---|---|---|---|---|---|---|---|---|---|---|
Software | ROI | Methods | Reviewers | Software | Method | Number of selected features | Categories of selected features | ||||
Meng (34) | 2018 | MRI (T2) | ITK-SNAP | 3D | Manual | 2 | MatLab | LASSO-COX | 8 | FOS + GLCM + GLRLM | |
Wang (38) | 2019 | NCCT | MIM | 3D | Manual | 2 | In-house Software | Test-retest, contour-recontour | 21 | GLCM + GLRLM | |
Cui (33) | 2021 | MRI (T2, cTI, ADC) | ITK-SNAP | 3D | Manual | 1 | PyRadiomics | RF, COX | 4 | GLRLM + GLSZM | |
Tibermacine (36) | 2021 | MRI (T2) | 3D slicer | 2D + 3D | Manual | 1 | PyRadiomics | RF | 9 | FOS + GLRLM + GLSZM + NGTDM + GLDM + GLCM | |
Chiloiro (14) | 2022 | MRI (TrueFISP) | Eclipse | 3D | Manual | 1 | MODDICOM | WMW test | 2 | FOS | |
Chuanji (15) | 2022 | MRI (T2) | ITK-SNAP | 3D | Manual | 2 | PyRadiomics | LASSO | 10 | FOS + GLCM + GLRLM + GLSZM + NGTDM | |
Cui (16) | 2022 | MRI (T2, cTI,DWI) | ITK-SNAP | 3D | Manual | 2 | PyRadiomics | Correlation-based, stability-based analysis | 6 | FOS + GLCM + GLRLM + GLSZM | |
Nie (35) | 2022 | MRI (T2, T1, DWI, DCE-MRI) | ITK-SNAP | 3D | Manual | 2 | NR | LASSO | 8 | FOS + GLCM + GLRLM | |
Wang (37) | 2022 | CECT | NR | 3D | Manual | 1 | PyRadiomics | LASSO-COX | 12 | FOS + Shape + GLCM + GLDM | |
Meng (39) | 2018 [2] | MRI (T2, cT1) | ITK-SNAP | 3D | Manual | 1 | NR | LASSO-COX | 12 | FOS + GLCM + GLRLM | |
Bang (18) | 2016 | PET/CT | MaZda | 3D | Manual | 1 | MaZda | COX | 1 | Absolute gradient | |
Chee (40) | 2017 | CT | NR | 2D | Manual | 2 | NR | Spearman’s rank correlation coefficient | 3 | FOS | |
Jalil (42) | 2017 | MRI (T2) | NR | 2D | Manual | 1 | TexRAD | COX | 2 | FOS | |
Lovinfosse (43) | 2018 | PET/CT | FLAB | 3D | Manual | 1 | Python | COX | 3 | FOS + GLCM + NGTDM | |
Hotta (41) | 2021 | PET/CT | NR | 3D | Manual | 2 | LIFEx | COX | 1 | GLCM |
ROI, region of interest; MRI, magnetic resonance imaging; T2, T2-weighted imaging; NCCT, non-contrast computed tomography; cT1, contrast enhanced T1-weighted imaging; ADC, apparent diffusion coefficient; TrueFISP, true fast imaging with steady state precession; T1, T1-weighted imaging; DWI, diffusion-weighted imaging; DCE-MRI, dynamic contrast-enhanced MRI; CECT, contrast-enhanced computed tomography; PET/CT, positron emission tomography/computed tomography; NR, not reported; 3D, 3-dimensional; 2D, 2-dimensional; LASSO, least absolute shrinkage and selection operator; RF, random forest; WMW test, Wilcoxon-Mann-Whitney test; FOS, first-order statistic; GLCM, gray-level co-occurrence matrix; GLRLM, gray-level run-length matrix; GLSZM, gray-level size zone matrix; NGTDM, neighboring gray-tone difference matrix.
Quality assessment of the radiomics models based on RQS score
In the 15 selected studies, the overall percentage of the total RQS score was 21.5% (Figure 2A). Among the six key domains, domain 5 performed the worst, with no significant high level of evidence, including prospective study and cost-effectiveness analysis. The second lowest score compared to the ideal score was observed in domain 6, with a mean of 3.3%, which meant that only two studies made code and data publicly available. Domain 1, domain 3, and domain 4 performed similarly, with mean scores of 33.3%, 36.7%, and 38.7%, respectively.
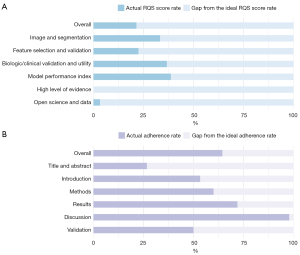
The details of the assessment of a total of 16 items for RQS are recorded in Table 3 and Table S3. The mean ± standard deviation [SD; median, range] of the total RQS score was 7.73±4.61 [8, 2–14]. In domain 1, all studies followed a well-documented image protocol. Some 53.3% of the studies were completed with multiple segmentations by different physicians or software. Only one study evaluated the feature robustness of CT scanners, and only one collected image of individuals at additional time points. In domain 2, all studies performed feature reduction or adjustment to decrease the risk of overfitting. Validation in seven studies was missing, seven studies were based on a dataset from the same institute, and only one was employed on another independent dataset. In domain 3, 13 studies reported the correlation between radiomics and non-radiomics features, 6 studies compared radiomics to “the gold standard”, and 4 investigated potential clinical utility. However, no studies detected and discussed biological correlates with radiomics. In domain 4, to reduce the risk of overly optimistic reporting, 11 studies analyzed the effects of the cutoff values on the model performance. In addition, 10 studies reported discrimination statistics of radiomics models, 3 of which applied bootstrapping or cross-validation. Only 4 studies reported calibration statistics. In domain 5, none of the studies provided the highest level of evidence or reported on the cost-effectiveness of the clinical application.
Table 3
16 items according to 6 key domains (N=15) | Total score range | Mean score | Percentage of ideal score (%) |
---|---|---|---|
Total 16 items | −8 to 36 | 7.73 | 21.5 |
Domain 1: protocol quality and stability in image and segmentation | 0 to 5 | 1.67 | 33.3 |
Image protocol quality | 0 to 2 | 1.00 | 50.0 |
Multiple segmentation | 0 to 1 | 0.53 | 53.3 |
Phantom study on all scanners | 0 to 1 | 0.07 | 6.7 |
Imaging at multiple time points | 0 to 1 | 0.07 | 6.7 |
Domain 2: feature selection and validation | −8 to 8 | 1.80 | 22.5 |
Feature reduction or adjustment for multiple testing | −3 to 3 | 3.00 | 100.0 |
Validation | −5 to 5 | −1.20 | 0 |
Domain 3: biologic/clinical validation and utility | 0 to 6 | 2.20 | 36.7 |
Multivariate analysis with non-radiomics features | 0 to 1 | 0.87 | 86.7 |
Detect and discuss biologic correlates | 0 to 1 | 0.00 | 0.0 |
Comparison to gold standard | 0 to 2 | 0.80 | 40.0 |
Potential clinical utility | 0 to 2 | 0.53 | 26.7 |
Domain 4: model performance index | 0 to 5 | 1.93 | 38.7 |
Cut-off analysis | 0 to 1 | 0.73 | 73.3 |
Discrimination statistics | 0 to 2 | 0.87 | 43.3 |
Calibration statistics | 0 to 2 | 0.33 | 16.7 |
Domain 5: high level of evidence | 0 to 8 | 0.00 | 0.0 |
Prospective study registered in a trial database | 0 to 7 | 0.00 | 0.0 |
Cost-effective analysis | 0 to 2 | 0.00 | 0.0 |
Domain 6: open science and data | 0 to 4 | 0.13 | 3.3 |
Open science and data | 0 to 4 | 0.13 | 3.3 |
RQS, radiomics quality score.
Quality assessment of prognosis studies based on the TRIPOD checklist
In 26 out of 35 items in the TRIPOD checklist (Figure 2B, Table 4), excluding “if relevant”, “if done”, and “validation” items, the mean number of adhered items was 16.7±3.8 (SD; range, 15–21), with an overall adherence rate of 64.4% (251/390). None of the studies satisfied the items of title (item 1), blindness in assessments (items 6b and 7b), missing data (item 9), and model recalibration in statistical analysis methods and results (items 10e and 17). The completeness of reporting individual TRIPOD items is shown in Table 4.
Table 4
35 selected items (N=15) | Adherence rate, n (%) |
---|---|
Overall | 251/390 (64.4) |
Title and Abstract | 8/30 (26.7) |
1. Title—identify developing/validating a model, target population, and the outcome | 0 (0.0) |
2. Abstract—provide a summary of objectives, study design, setting, participants, sample size, predictors, outcome, statistical analysis, results, and conclusions | 8 (53.3) |
Introduction | 16/30 (53.3) |
3a. Background—explain the medical context and rationale for developing/validating the model | 14 (93.3) |
3b. Objective—specify the objectives, including whether the study describes the development/validation of the model or both | 2 (13.3) |
Methods | 117/195 (60.0) |
4a. Source of data—describe the study design or source of data (randomized trial, cohort, or registry data) | 15 (100.0) |
4b. Source of data—specify the key dates | 14 (93.3) |
5a. Participants—specify key elements of the study setting including number and location of centers | 14 (93.3) |
5b. Participants—describe eligibility criteria for participants (inclusion and exclusion criteria) | 13 (86.7) |
5c. Participants—give details of treatment received, if relevant | NA |
6a. Outcome—clearly define the outcome, including how and when assessed | 15 (100.0) |
6b. Outcome—report any actions to blind assessment of the outcome | 0 (0.0) |
7a. Predictors—clearly define all predictors, including how and when assessed | 15 (100.0) |
7b. Predictors—report any actions to blind assessment of predictors for the outcome and other predictors | 0 (0.0) |
8. Sample size—explain how the study size was attained | 2 (13.3) |
9. Missing data—describe how missing data were handled with details of any imputation method | 0 (0.0) |
10a. Statistical analysis methods—describe how predictors were handled | 15 (100.0) |
10b. Statistical analysis methods—specify type of model, all model-building procedures (any predictor selection), and method for internal validation | 9 (60.0) |
10d. Statistical analysis methods—specify all measures used to assess model performance and if relevant, to compare multiple models (discrimination and calibration) | 5 (33.3) |
11. Risk groups—provide details on how risk groups were created, if conducted (N=7) | NA |
Results | 66/90 (73.3) |
13a. Participants—describe the flow of participants, including the number of participants with and without the outcome. A diagram may be helpful | 14 (93.3) |
13b. Participants—describe the characteristics of the participants, including the number of participants with missing data for predictors and outcome | 12 (80.0) |
14a. Model development—specify the number of participants and outcome events in each analysis | 15 (100.0) |
14b. Model development—report the unadjusted association between each candidate predictor and outcome, if done (N=9) | NA |
15a. Model specification—present the full prediction model to allow predictions for individuals (regression coefficients, intercept) | 8 (53.3) |
15b. Model specification—explain how to the use the prediction model (nomogram, calculator, etc.) | 7 (46.7) |
16. Model performance—report performance measures (with confidence intervals) for the prediction model | 10 (66.7) |
Discussion | 44/45 (97.8) |
18. Limitations—discuss any limitations of the study | 15 (100.0) |
19b. Interpretation—give an overall interpretation of the results | 15 (100.0) |
20. Implications—discuss the potential clinical use of the model and implications for future research | 14 (93.3) |
For validation (N=8) | 24/48 (50.0) |
10c. Statistical analysis methods—describe how the predictions were calculated | 6 (75.0) |
10e. Statistical analysis methods—describe any model updating (recalibration), if conducted | 0 (0.0) |
12. Development vs. validation—Identify any differences from the development data in setting, eligibility criteria, outcome, and predictors | 8 (100.0) |
13c. Participants (for validation)—show a comparison with the development data of the distribution of important variables | 8 (100.0) |
17. Model updating—report the results from any model updating, if performed | 0 (0.0) |
19a. Interpretation (for validation)—discuss the results with reference to performance in the development data and any other validation data | 2 (25.0) |
TRIPOD, Transparent Reporting of a Multivariable Prediction Model for Individual Prognosis or Diagnosis; NA, not available.
Quality assessment of the radiomics models based on IBSI guideline
Table 5 presents the pre-processing steps carried out in the included studies, following the IBSI guidelines, with an overall adherence rate of 51.4% (54/105). Intensity normalization and image interpolation were the most frequently conducted pre-processing steps, both at 53.3%. Image filtering was conducted in seven studies, accounting for 46.7% of the total. Grey-level discretization was carried out in six studies, comprising 18.8% of the sample. In addition, robustness assessment of imaging biomarkers was performed in 6 studies, making up 40.0% of the total. Among the software packages used for radiomics feature extraction, only PyRadiomics (https://www.radiomics.io/pyradiomics.html) conforms to the IBSI guidelines, which was utilized in 33.3% of the articles. Lastly, the segmentation method employed during delineation was exclusively manual tracing. None of the included studies utilized fully automatic or semi-automatic methods for segmentation.
Table 5
Pre-processing performed | Number of studies (adherence rate, %) |
---|---|
Total | 54 (51.4) |
Intensity normalization | 8 (53.3) |
Segmentation method | 15 (100.0) |
Image interpolation | 8 (53.3) |
Grey-level discretization | 5 (33.3) |
Image filter | 7 (46.7) |
Extraction software | 5 (33.3) |
Robustness assessment | 6 (40.0) |
IBSI, Image Biomarkers Standardization Initiative.
Quality assessment of the radiomics models based on PROBAST tool
The analysis of ROB and applicability is presented in Figure 3. The overall ROB was unclear in 6 studies and high in 9 studies (Figure 3A). Within the ROB assessment, high bias was identified in the “analysis” domain for 93.3% of the studies, contrasting with low bias observed in the “results” domain (73.3%). Concerning overall applicability (Figure 3B), 12 studies displayed low concern, 3 studies had unclear concern, and additional details are provided in the Table S4.
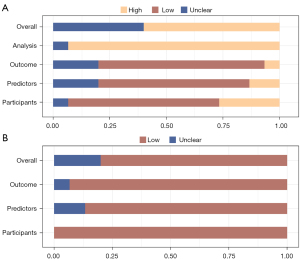
Meta-analysis results for DFS
The association between radiomic features and DFS was evaluated in 12/15 (80%) studies, and all of them showed a significant association between radiomic features and DFS. Furthermore, 10 studies with a total of 1,492 patients provided HR values, which were then extracted for further meta-analysis. The pooled HR for DFS was 3.14 (95% CI: 2.12–4.64), and Cochran’s Q test (P=0.02) and Higgins’ I2 test (56%) showed moderate heterogeneity among the included studies (Figure 4A). A further subgroup analysis based on the imaging modality found significant results in the MRI, CT, and PET/CT subgroups (Figure 4B, MRI: HR =3.34, 95% CI: 2.10–5.32; CT: HR =2.10, 95% CI: 1.11–3.98; PET/CT: HR =10.30, 95% CI: 2.90–36.53). Visual inspection of the funnel plot and Egger’s test (P=0.398) showed no publication bias (Figure S1).
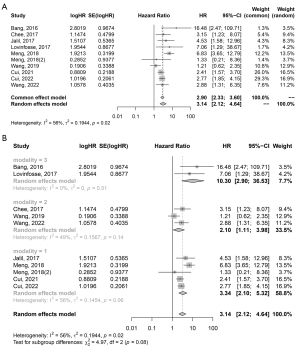
Meta-analysis results for OS
The association between radiomic features and OS was evaluated in 8/15 (53.3%) studies, and all of them showed a significant association between radiomic features and OS. In addition, 7 of these studies, with a combined total of 1,230 patients, provided HR values, which were then extracted for further meta-analysis. The pooled HR for OS was 3.36 (95% CI: 1.74–6.49), and Cochran’s Q test (P=0.01) and Higgins’ I2 test (63%) showed moderate heterogeneity among the included studies (Figure 5A). A further subgroup analysis based on the imaging modality found significant results in the MRI and PET/CT subgroups (Figure 5B, MRI: HR =6.98, 95% CI: 3.24–15.02; PET/CT: HR =3.90, 95% CI: 1.71–8.89).
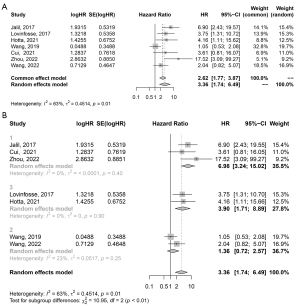
Discussion
To the best of our knowledge, this is the first study to perform both a systematic review and a meta-analysis regarding radiomics prediction value on survival outcomes in LARC patients undergoing nCRT. This systematic review combined the outcomes of 2,151 LARC patients from 15 individual studies and extracted the HR values for further meta-analysis, which showed that radiomics based on the primary LARC lesion, depicting intratumor heterogeneity, played a promising role in LARC prognosis prediction.
Radiomics is a novel, noninvasive, and potential tool to extract quantitative features from medical images, which could convert images into mineable data for subsequent analysis. In particular, radiomics has been shown to reveal tumor heterogeneity, which is associated with prognosis in LARC patients. Our meta-analyses indicated that radiomics based on the primary LARC lesion significantly predicted poor DFS (HR =3.14, 95% CI: 2.12–4.64, P<0.01) and OS (HR =3.36, 95% CI: 1.74–6.49, P<0.01). The results showed that the radiomics model may be an independent and noninvasive predictive biomarker, allowing us to stratify patients into low- and high-risk groups and identify those who may truly benefit from treatment and achieve long-term survival by mining medical image data to reflect tumor heterogeneity. Similar conclusions have been reached in previous meta-analyses regarding the prognosis of non-small cell lung cancer, esophageal cancer, pancreatic ductal adenocarcinoma, and ovarian cancer (44-47). In addition, deep learning is a machine learning algorithm based on neural networks, providing an alternative to traditional manual radiomics (48). It alleviates the model’s reliance on accurate tumor segmentation and feature definition, thereby enhancing feature consistency and reproducibility while reducing the workload associated with data management. However, its current application in the literature remains limited (49-52), possibly due to its substantial demand for training data and lack of interpretability in models (53). In the future, the integration of radiomics with deep learning could lead to the creation of a new frontier in personalized medical imaging, resulting in the development of higher-performance models.
Generally, radiomics data contain first-, second-, and higher-order statistics (11). In our review, we summarized the radiomics features associated with survival (Table 2). The results were similar to those of Schurink et al., who found that simpler features (e.g., first-order, shape, GLCM, and GLRLM) showed overall good reproducibility, whereas higher-order features (e.g., GLSZM and NGTDM) were poorly reproducible (54). These results also aligned with those of previous studies (55-57). In addition, Gao et al. selected nine studies for the meta-analysis, which indicated that first-order entropy was reported multiple times in the studies on prognosis prediction and showed a significant pooled HR of 1.66 (95% CI: 1.18–2.34) in pancreatic ductal adenocarcinoma patients (46). Although these studies preliminarily demonstrate seemingly good reproducibility, the reproducibility of simpler radiomics features is still insufficient compared with the measurement of carcinoembryonic antigen (CEA), carbohydrate antigen 19-9 (CA19-9), and other tumor markers, which has been one of the most important challenges in radiomics for years. However, the IBSI guideline, which was an IBSI instigated by Zwanenburg et al., aims to improve the standardization of imaging protocols and results reporting; thus, strict compliance may improve reproducibility (21). In addition, the inclusion of higher-order features in radiomics models may be a major cause of poor reproducibility, but no relevant studies have shown how much it affects reproducibility, thus future research should focus on higher-order features.
For radiomics quality, different tools were utilized in this analysis to provide an in-depth and all-encompassing evaluation of the included studies. We found that the quality of radiomics studies for prognosis assessment in LARC patients was insufficient. The overall mean RQS score in our study was 7.73±4.61 (21.5% of the ideal score), which was consistent with those of other systematic reviews (12,25,29,58), and the most problematic issues were similar. The included studies performed the worst in domain 5 (item prospective study and cost-effective analysis), with the actual score being 0% of the ideal RQS score (Figure 2, Table 3). In the era of evidence-based medicine, radiomics, as the basis of promising noninvasive imaging markers, must first be prospectively validated in clinical populations before it can be used in the clinic, and then the utility of radiomics in comparison to other accessible biomarkers needs to be evaluated through a cost-effectiveness analysis. However, most radiomic studies are proof-of-concept studies, and no prospective trials on prognosis prediction in LARC have been initialized. Therefore, it is essential to consider prospective trials and cost-effectiveness analyses in the design of future radiomics studies.
In addition, the mean RQS score on item validation of domain 2 was only 0.56 because most of the included studies used the single-center internal validation cohort and received a score of 2, yet the rest did not use the validation cohort and received a score of –5. The RQS score assigns a –5 if validation is missing, a 2 if validation is based on the same institute’s dataset, a 3 if validation is based on another institute’s dataset, a 4 if validation is based on two datasets from two different institutes or validates previously published features, and a 5 if validation is based on datasets from 3 or more different institutes. As a result of the current poor scores, a multicenter validation set or validation of the previous radiomics features will be required in the future to improve the estimated quality of radiomics. Federated multicenter data studies can increase sample size and data diversity, thus improving the generalization of models. However, for reasons such as medical data privacy and security, it is difficult to centralize data in one place for centralized machine learning. Therefore, how to combine multicenter data to build radiomics models without sharing private patient data is also one of the future research priorities. Federated learning techniques may be one solution to address this issue.
Furthermore, there were certain other prevalent issues. The insufficiency of phantom study, test-retest, cutoff, and open science and data were repeatedly addressed. Although the performance was good in terms of image protocol quality, multiple segmentation, feature reduction, multivariate analysis with non-radiomics features, and discrimination statistics, of the six domains, only domain 4 exceeded 50% in the percentage of the ideal score. According to the TRIPOD checklist, the keywords “development” and “validation” were seldom ever used in the titles, abstracts, or objectives. The vast majority of studies lack blinded assessment, processing of missing data, and sample size determination. However, the large number of features compared to the number of patients makes sample size calculations virtually impossible. Therefore, considering the specificity of radiomics features, a reasonable sample size determination standard designed specifically for radiomics must be developed.
This systematic review has some limitations. First, there was moderate heterogeneity among the included studies in the HR values for DFS and OS. Although we performed subgroup analyses, the sample sizes may be too small to draw reliable conclusions from the group analyses. Second, the main limitation of our study is that the study designs of the published studies were all retrospective in design. Some patients may be lost to follow-up, which might affect the accuracy of prognosis prediction. Third, because of the limited study numbers, visual inspection of the funnel plot and Egger’s test for studies predicting OS were not employed. Fourth, due to overlapping with RQS and TRIPOD, we focused on evaluating the pre-processing steps based on the IBSI guidelines. In future research, it would be beneficial to integrate these checklists to establish universally accepted methods and reporting standards. Finally, only radiomics-based prognostic models that were not integrated with other clinical factors were evaluated because these factors varied greatly between trials and were unsuitable for pooled values.
Conclusions
In conclusion, the primary tumor lesion-based radiomics model performed promisingly in LARC prognosis prediction. However, the overall methodological quality of radiomics studies was low and the adherence to the TRIPOD statement was moderate. Future radiomics research should put a greater focus on enhancing methodological quality and considering the influence of higher-order features on reproducibility in radiomics.
Acknowledgments
Funding: None.
Footnote
Reporting Checklist: The authors have completed the PRISMA reporting checklist. Available at https://qims.amegroups.com/article/view/10.21037/qims-23-692/rc
Conflicts of Interest: All authors have completed the ICMJE uniform disclosure form (available at https://qims.amegroups.com/article/view/10.21037/qims-23-692/coif). The authors have no conflicts of interest to declare.
Ethical Statement: The authors are accountable for all aspects of the work in ensuring that questions related to the accuracy or integrity of any part of the work are appropriately investigated and resolved.
Open Access Statement: This is an Open Access article distributed in accordance with the Creative Commons Attribution-NonCommercial-NoDerivs 4.0 International License (CC BY-NC-ND 4.0), which permits the non-commercial replication and distribution of the article with the strict proviso that no changes or edits are made and the original work is properly cited (including links to both the formal publication through the relevant DOI and the license). See: https://creativecommons.org/licenses/by-nc-nd/4.0/.
References
- Sung H, Ferlay J, Siegel RL, Laversanne M, Soerjomataram I, Jemal A, Bray F. Global Cancer Statistics 2020: GLOBOCAN Estimates of Incidence and Mortality Worldwide for 36 Cancers in 185 Countries. CA Cancer J Clin 2021;71:209-49. [Crossref] [PubMed]
- Benson AB, Venook AP, Al-Hawary MM, Cederquist L, Chen YJ, Ciombor KK, et al. Rectal Cancer, Version 2.2018, NCCN Clinical Practice Guidelines in Oncology. J Natl Compr Canc Netw 2018;16:874-901. [Crossref] [PubMed]
- Meng Y, Wan L, Ye F, Zhang C, Zou S, Zhao X, Xu K, Zhang H, Zhou C. MRI morphologic and clinicopathologic characteristics for predicting outcomes in patients with locally advanced rectal cancer. Abdom Radiol (NY) 2019;44:3652-63. [Crossref] [PubMed]
- Valentini V, van Stiphout RG, Lammering G, Gambacorta MA, Barba MC, Bebenek M, Bonnetain F, Bosset JF, Bujko K, Cionini L, Gerard JP, Rödel C, Sainato A, Sauer R, Minsky BD, Collette L, Lambin P. Nomograms for predicting local recurrence, distant metastases, and overall survival for patients with locally advanced rectal cancer on the basis of European randomized clinical trials. J Clin Oncol 2011;29:3163-72. [Crossref] [PubMed]
- Babaei M, Jansen L, Balavarca Y, Sjövall A, Bos A, van de Velde T, Moreau M, Liberale G, Gonçalves AF, Bento MJ, Ulrich CM, Schrotz-King P, Lemmens V, Glimelius B, Brenner H. Neoadjuvant Therapy in Rectal Cancer Patients With Clinical Stage II to III Across European Countries: Variations and Outcomes. Clin Colorectal Cancer 2018;17:e129-42. [Crossref] [PubMed]
- Dekker JW, Peeters KC, Putter H, Vahrmeijer AL, van de Velde CJ. Metastatic lymph node ratio in stage III rectal cancer; prognostic significance in addition to the 7th edition of the TNM classification. Eur J Surg Oncol 2010;36:1180-6.
- Yagi R, Shimada Y, Kameyama H, Tajima Y, Okamura T, Sakata J, Kobayashi T, Kosugi SI, Wakai T, Nogami H, Maruyama S, Takii Y, Kawasaki T, Honma KI. Clinical significance of extramural tumor deposits in the lateral pelvic lymph node area in low rectal cancer: a retrospective study at two institutions. Ann Surg Oncol 2016;23:552-8. [Crossref] [PubMed]
- Nagtegaal ID, Quirke P, Schmoll HJ. Has the new TNM classification for colorectal cancer improved care? Nat Rev Clin Oncol 2011;9:119-23. [Crossref] [PubMed]
- Taylor FG, Quirke P, Heald RJ, Moran BJ, Blomqvist L, Swift IR, Sebag-Montefiore D, Tekkis P, Brown GMagnetic Resonance Imaging in Rectal Cancer European Equivalence Study Study Group. Preoperative magnetic resonance imaging assessment of circumferential resection margin predicts disease-free survival and local recurrence: 5-year follow-up results of the MERCURY study. J Clin Oncol 2014;32:34-43. [Crossref] [PubMed]
- Zech CJ. MRI of Extramural Venous Invasion in Rectal Cancer: A New Marker for Patient Prognosis? Radiology 2018;289:686-7. [Crossref] [PubMed]
- Gillies RJ, Kinahan PE, Hricak H. Radiomics: Images Are More than Pictures, They Are Data. Radiology 2016;278:563-77. [Crossref] [PubMed]
- Lee S, Choi Y, Seo MK, Jang J, Shin NY, Ahn KJ, Kim BS. Magnetic Resonance Imaging-Based Radiomics for the Prediction of Progression-Free Survival in Patients with Nasopharyngeal Carcinoma: A Systematic Review and Meta-Analysis. Cancers (Basel) 2022;14:653. [Crossref] [PubMed]
- Kothari G, Korte J, Lehrer EJ, Zaorsky NG, Lazarakis S, Kron T, Hardcastle N, Siva S. A systematic review and meta-analysis of the prognostic value of radiomics based models in non-small cell lung cancer treated with curative radiotherapy. Radiother Oncol 2021;155:188-203. [Crossref] [PubMed]
- Chiloiro G, Boldrini L, Preziosi F, Cusumano D, Yadav P, Romano A, Placidi L, Lenkowicz J, Dinapoli N, Bassetti MF, Gambacorta MA, Valentini V. A Predictive Model of 2yDFS During MR-Guided RT Neoadjuvant Chemoradiotherapy in Locally Advanced Rectal Cancer Patients. Front Oncol 2022;12:831712. [Crossref] [PubMed]
- Chuanji Z, Zheng W, Shaolv L, Linghou M, Yixin L, Xinhui L, Ling L, Yunjing T, Shilai Z, Shaozhou M, Boyang Z. Comparative study of radiomics, tumor morphology, and clinicopathological factors in predicting overall survival of patients with rectal cancer before surgery. Transl Oncol 2022;18:101352. [Crossref] [PubMed]
- Cui Y, Wang G, Ren J, Hou L, Li D, Wen Q, Xi Y, Yang X. Radiomics Features at Multiparametric MRI Predict Disease-Free Survival in Patients With Locally Advanced Rectal Cancer. Acad Radiol 2022;29:e128-38. [Crossref] [PubMed]
- Wei FZ, Mei SW, Chen JN, Wang ZJ, Shen HY, Li J, Zhao FQ, Liu Z, Liu Q. Nomograms and risk score models for predicting survival in rectal cancer patients with neoadjuvant therapy. World J Gastroenterol 2020;26:6638-57. [Crossref] [PubMed]
- Bang JI, Ha S, Kang SB, Lee KW, Lee HS, Kim JS, Oh HK, Lee HY, Kim SE. Prediction of neoadjuvant radiation chemotherapy response and survival using pretreatment [(18)F]FDG PET/CT scans in locally advanced rectal cancer. Eur J Nucl Med Mol Imaging 2016;43:422-31.
- Lambin P, Leijenaar RTH, Deist TM, Peerlings J, de Jong EEC, van Timmeren J, Sanduleanu S, Larue RTHM, Even AJG, Jochems A, van Wijk Y, Woodruff H, van Soest J, Lustberg T, Roelofs E, van Elmpt W, Dekker A, Mottaghy FM, Wildberger JE, Walsh S. Radiomics: the bridge between medical imaging and personalized medicine. Nat Rev Clin Oncol 2017;14:749-62. [Crossref] [PubMed]
- Collins GS, Reitsma JB, Altman DG, Moons KG. Transparent reporting of a multivariable prediction model for individual prognosis or diagnosis (TRIPOD): the TRIPOD statement. Br J Surg 2015;102:148-58.
- Zwanenburg A, Vallières M, Abdalah MA, Aerts HJWL, Andrearczyk V, Apte A, et al. The Image Biomarker Standardization Initiative: Standardized Quantitative Radiomics for High-Throughput Image-based Phenotyping. Radiology 2020;295:328-38. [Crossref] [PubMed]
- Wolff RF, Moons KGM, Riley RD, Whiting PF, Westwood M, Collins GS, Reitsma JB, Kleijnen J, Mallett S. PROBAST Group. PROBAST: A Tool to Assess the Risk of Bias and Applicability of Prediction Model Studies. Ann Intern Med 2019;170:51-8. [Crossref] [PubMed]
- Page MJ, McKenzie JE, Bossuyt PM, Boutron I, Hoffmann TC, Mulrow CD, et al. The PRISMA 2020 statement: an updated guideline for reporting systematic reviews. Syst Rev 2021;10:89. [Crossref] [PubMed]
- Sanduleanu S, Woodruff HC, de Jong EEC, van Timmeren JE, Jochems A, Dubois L, Lambin P. Tracking tumor biology with radiomics: A systematic review utilizing a radiomics quality score. Radiother Oncol 2018;127:349-60. [Crossref] [PubMed]
- Lee S, Han K, Suh YJ. Quality assessment of radiomics research in cardiac CT: a systematic review. Eur Radiol 2022;32:3458-68. [Crossref] [PubMed]
- Park CJ, Park YW, Ahn SS, Kim D, Kim EH, Kang SG, Chang JH, Kim SH, Lee SK. Quality of Radiomics Research on Brain Metastasis: A Roadmap to Promote Clinical Translation. Korean J Radiol 2022;23:77-88. [Crossref] [PubMed]
- Park JE, Kim D, Kim HS, Park SY, Kim JY, Cho SJ, Shin JH, Kim JH. Quality of science and reporting of radiomics in oncologic studies: room for improvement according to radiomics quality score and TRIPOD statement. Eur Radiol 2020;30:523-36. [Crossref] [PubMed]
- Won SY, Park YW, Ahn SS, Moon JH, Kim EH, Kang SG, Chang JH, Kim SH, Lee SK. Quality assessment of meningioma radiomics studies: Bridging the gap between exploratory research and clinical applications. Eur J Radiol 2021;138:109673. [Crossref] [PubMed]
- Zhong J, Hu Y, Ge X, Xing Y, Ding D, Zhang G, Zhang H, Yang Q, Yao W. A systematic review of radiomics in chondrosarcoma: assessment of study quality and clinical value needs handy tools. Eur Radiol 2023;33:1433-44. [Crossref] [PubMed]
- DerSimonian R, Laird N. Meta-analysis in clinical trials. Control Clin Trials 1986;7:177-88. [Crossref] [PubMed]
- Higgins JP, Thompson SG, Deeks JJ, Altman DG. Measuring inconsistency in meta-analyses. BMJ 2003;327:557-60. [Crossref] [PubMed]
- Lau J, Ioannidis JP, Terrin N, Schmid CH, Olkin I. The case of the misleading funnel plot. BMJ 2006;333:597-600. [Crossref] [PubMed]
- Cui Y, Yang W, Ren J, Li D, Du X, Zhang J, Yang X. Prognostic value of multiparametric MRI-based radiomics model: Potential role for chemotherapeutic benefits in locally advanced rectal cancer. Radiother Oncol 2021;154:161-9. [Crossref] [PubMed]
- Meng Y, Zhang Y, Dong D, Li C, Liang X, Zhang C, Wan L, Zhao X, Xu K, Zhou C, Tian J, Zhang H. Novel radiomic signature as a prognostic biomarker for locally advanced rectal cancer. J Magn Reson Imaging 2018; Epub ahead of print. [Crossref]
- Nie K, Hu P, Zheng J, Zhang Y, Yang P, Jabbour SK, Yue N, Dong X, Xu S, Shen B, Niu T, Hu X, Cai X, Sun J. Incremental Value of Radiomics in 5-Year Overall Survival Prediction for Stage II-III Rectal Cancer. Front Oncol 2022;12:779030. [Crossref] [PubMed]
- Tibermacine H, Rouanet P, Sbarra M, Forghani R, Reinhold C, Nougaret SGRECCAR Study Group. Radiomics modelling in rectal cancer to predict disease-free survival: evaluation of different approaches. Br J Surg 2021;108:1243-50. [Crossref] [PubMed]
- Wang F, Tan BF, Poh SS, Siow TR, Lim FLWT, Yip CSP, Wang MLC, Nei W, Tan HQ. Predicting outcomes for locally advanced rectal cancer treated with neoadjuvant chemoradiation with CT-based radiomics. Sci Rep 2022;12:6167. [Crossref] [PubMed]
- Wang J, Shen L, Zhong H, Zhou Z, Hu P, Gan J, Luo R, Hu W, Zhang Z. Radiomics features on radiotherapy treatment planning CT can predict patient survival in locally advanced rectal cancer patients. Sci Rep 2019;9:15346. [Crossref] [PubMed]
- Meng Y, Zhang Y, Zhang C, Wan L, Zhang H, Dong D, Zhao X, Xu K, Li C, Zhou C. To compare the predictive value of the radiomics signature extrated from MRI plain or enhancement imaging for the survival of rectal cancer. Chinese Journal of Radiology 2018;52:349-55.
- Chee CG, Kim YH, Lee KH, Lee YJ, Park JH, Lee HS, Ahn S, Kim B. CT texture analysis in patients with locally advanced rectal cancer treated with neoadjuvant chemoradiotherapy: A potential imaging biomarker for treatment response and prognosis. PLoS One 2017;12:e0182883. [Crossref] [PubMed]
- Hotta M, Minamimoto R, Gohda Y, Miwa K, Otani K, Kiyomatsu T, Yano H. Prognostic value of (18)F-FDG PET/CT with texture analysis in patients with rectal cancer treated by surgery. Ann Nucl Med 2021;35:843-52. [Crossref] [PubMed]
- Jalil O, Afaq A, Ganeshan B, Patel UB, Boone D, Endozo R, Groves A, Sizer B, Arulampalam T. Magnetic resonance based texture parameters as potential imaging biomarkers for predicting long-term survival in locally advanced rectal cancer treated by chemoradiotherapy. Colorectal Dis 2017;19:349-62. [Crossref] [PubMed]
- Lovinfosse P, Polus M, Van Daele D, Martinive P, Daenen F, Hatt M, Visvikis D, Koopmansch B, Lambert F, Coimbra C, Seidel L, Albert A, Delvenne P, Hustinx R. FDG PET/CT radiomics for predicting the outcome of locally advanced rectal cancer. Eur J Nucl Med Mol Imaging 2018;45:365-75. [Crossref] [PubMed]
- Chen Q, Zhang L, Mo X, You J, Chen L, Fang J, Wang F, Jin Z, Zhang B, Zhang S. Current status and quality of radiomic studies for predicting immunotherapy response and outcome in patients with non-small cell lung cancer: a systematic review and meta-analysis. Eur J Nucl Med Mol Imaging 2021;49:345-60. [Crossref] [PubMed]
- Rizzo S, Manganaro L, Dolciami M, Gasparri ML, Papadia A, Del Grande F. Computed Tomography Based Radiomics as a Predictor of Survival in Ovarian Cancer Patients: A Systematic Review. Cancers (Basel) 2021;13:573. [Crossref] [PubMed]
- Gao Y, Cheng S, Zhu L, Wang Q, Deng W, Sun Z, Wang S, Xue H. A systematic review of prognosis predictive role of radiomics in pancreatic cancer: heterogeneity markers or statistical tricks? Eur Radiol 2022;32:8443-52. [Crossref] [PubMed]
- Shi Z, Zhang Z, Liu Z, Zhao L, Ye Z, Dekker A, Wee L. Methodological quality of machine learning-based quantitative imaging analysis studies in esophageal cancer: a systematic review of clinical outcome prediction after concurrent chemoradiotherapy. Eur J Nucl Med Mol Imaging 2022;49:2462-81. [Crossref] [PubMed]
- Wainberg M, Merico D, Delong A, Frey BJ. Deep learning in biomedicine. Nat Biotechnol 2018;36:829-38. [Crossref] [PubMed]
- Jiang X, Zhao H, Saldanha OL, Nebelung S, Kuhl C, Amygdalos I, Lang SA, Wu X, Meng X, Truhn D, Kather JN, Ke J. An MRI Deep Learning Model Predicts Outcome in Rectal Cancer. Radiology 2023;307:e222223. [Crossref] [PubMed]
- Liu X, Zhang D, Liu Z, Li Z, Xie P, Sun K, Wei W, Dai W, Tang Z, Ding Y, Cai G, Tong T, Meng X, Tian J. Deep learning radiomics-based prediction of distant metastasis in patients with locally advanced rectal cancer after neoadjuvant chemoradiotherapy: A multicentre study. EBioMedicine 2021;69:103442. [Crossref] [PubMed]
- Yang CH, Chen WC, Chen JB, Huang HC, Chuang LY. Overall mortality risk analysis for rectal cancer using deep learning-based fuzzy systems. Comput Biol Med 2023;157:106706. [Crossref] [PubMed]
- Li J, Zhou Y, Wang P, Zhao H, Wang X, Tang N, Luan K. Deep transfer learning based on magnetic resonance imaging can improve the diagnosis of lymph node metastasis in patients with rectal cancer. Quant Imaging Med Surg 2021;11:2477-85. [Crossref] [PubMed]
- Papadimitroulas P, Brocki L, Christopher Chung N, Marchadour W, Vermet F, Gaubert L, Eleftheriadis V, Plachouris D, Visvikis D, Kagadis GC, Hatt M. Artificial intelligence: Deep learning in oncological radiomics and challenges of interpretability and data harmonization. Phys Med 2021;83:108-21. [Crossref] [PubMed]
- Schurink NW, van Kranen SR, Roberti S, van Griethuysen JJM, Bogveradze N, Castagnoli F, El Khababi N, Bakers FCH, de Bie SH, Bosma GPT, Cappendijk VC, Geenen RWF, Neijenhuis PA, Peterson GM, Veeken CJ, Vliegen RFA, Beets-Tan RGH, Lambregts DMJ. Sources of variation in multicenter rectal MRI data and their effect on radiomics feature reproducibility. Eur Radiol 2022;32:1506-16. [Crossref] [PubMed]
- Haarburger C, Schock J, Truhn D, Weitz P, Mueller-Franzes G, Weninger L, Merhof D. Radiomic Feature Stability Analysis Based on Probabilistic Segmentations. 2020 IEEE 17th International Symposium on Biomedical Imaging (ISBI 2020); 2020:1188-92.
- Lee J, Steinmann A, Ding Y, Lee H, Owens C, Wang J, Yang J, Followill D, Ger R, MacKin D, Court LE. Radiomics feature robustness as measured using an MRI phantom. Sci Rep 2021;11:3973. [Crossref] [PubMed]
- Traverso A, Wee L, Dekker A, Gillies R. Repeatability and Reproducibility of Radiomic Features: A Systematic Review. Int J Radiat Oncol Biol Phys 2018;102:1143-58. [Crossref] [PubMed]
- Jia LL, Zheng QY, Tian JH, He DL, Zhao JX, Zhao LP, Huang G. Artificial intelligence with magnetic resonance imaging for prediction of pathological complete response to neoadjuvant chemoradiotherapy in rectal cancer: A systematic review and meta-analysis. Front Oncol 2022;12:1026216. [Crossref] [PubMed]