Development and validation of a deep learning model for multicategory pneumonia classification on chest computed tomography: a multicenter and multireader study
Introduction
Pneumonia, a common form of lung infection, can cause serious mortality and morbidity, especially among children and older adults (1). During the coronavirus disease 2019 (COVID-19) epidemic, more than 766 million people have been affected around the world, and over 6.93 million COVID-19-associated pneumonia deaths were confirmed as of January 2023 (2). The type of pneumonia that emerges depends on the contagious pathogen, such as viral, bacterial, and fungal, etc. Thus, early diagnosis and accurate pneumonia classification are vital for early and effective management and for reducing mortality. In addition, rapid and accurate diagnosis can help direct the isolation protocol and prevent the spread of the disease.
Computed tomography (CT) and chest X-ray are routinely used to diagnose various respiratory conditions, including pneumonia and COVID-19-associated pneumonia (3,4). However, as different types of pneumonia may have similar imaging features, such as consolidation, ground-glass opacity, cavity, and pleural effusions, chest X-ray and CT images can often be inconclusive, and different conditions can be easily confused for one another (1,5).
The deep learning (DL) method is a widely adopted technology with proven effectiveness. It can automatically learn representative features from large datasets of imaging features that are indiscernible to the radiologists on chest CT and can efficiently generate models that produce more accurate results than can human performance in predicting and classifying different diseases, even in task-specific applications (6). A common function of DL in oncology is the differentiation and screening of tumors, such those of the breast, liver, brain, and lungs (7). More recently, DL has also been applied to detect COVID-19 pneumonia and differentiate COVID-19 pneumonia from other viral pneumonia (VP) or community-acquired pneumonia (8,9).
Our study aimed to develop and validate a DL model based on chest CT for accurate classification of VP, bacterial pneumonia (BP), fungal pneumonia (FP), pulmonary tuberculosis (PTB), and no pneumonia (NP) conditions and to assess radiologists’ performance with and without the assistance of the DL method. We present this article in accordance with the TRIPOD reporting checklist (available at https://qims.amegroups.com/article/view/10.21037/qims-23-1097/rc).
Methods
Patient selection
This retrospective study was approved by the Medical Ethics Committee of Shanghai Public Health Clinical Center (No. 2022-S074-02) and was conducted in accordance with the Declaration of Helsinki (as revised in 2013). Informed consent was waived due to the retrospective nature of this research.
A total of 1,776 cases attending five hospitals between September 2019 and June 2023 diagnosed with VP, BP, FP, PTB, and NP were enrolled in this study. The general inclusion criteria for participants were the following: (I) age ≥18 years old; (II) confirmed with VP, FP, or PTB according to microbial, beta-d-glucan and galactomannan, or nucleic acid test; (III) for healthy participants, NP and no pulmonary parenchymal lesions except nodules smaller than 3 mm; (IV) for patients with BP (10), definite etiologic evidence (for example, Streptococcus pneumoniae and Legionella pneumonia) or cases meeting the diagnostic criteria for community-acquired pneumonia, with etiological results with diagnostic value for BP, with no evidence of Mycoplasma pneumonia and Chlamydia pneumonia infection, and with definite effect of antibiotic treatment; (V) patients who received thoracic CT scans before treatment; and (VI) patients with available CT images in Digital Imaging and Communications in Medicine (DICOM) format. The exclusion criteria were the following: (I) patients with other lung diseases (including pneumonia with more than two pathogens or tumors); (II) patients with a history of lung surgery; and (III) cases with poor CT image quality caused by respiratory motion or metal artifacts. After screening, 1,611 cases from two hospitals (from Shanghai, China) were used to develop the DL model and conduct internal testing, while 165 cases from 3 hospitals (2 from Shanghai, China, and 1 from Taiyuan, Shanxi, China) were used as the independent external test set to evaluate the model. Figure 1 shows the study design and the inclusion and exclusion criteria.
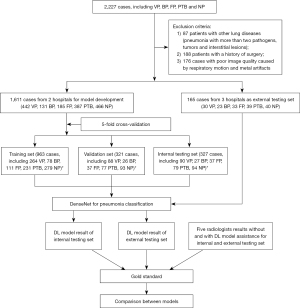
CT scan parameters
CT images of the cases were collected according to the standard chest imaging protocol. Participants were supine, with their arms over their head, and scanning was performed during a breath-hold using three different scanners (Brilliance 64, Philips, Amsterdam, The Netherlands; Aquilion ONE 320, Canon, Tokyo, Japan; uCT760, United Imaging, Shanghai, China). The main acquisition parameters were as follows: tube voltage =80–140 kV, automatic tube current modulation, pitch =1, matrix =512×512, slice thickness =5 mm, and slice interval =5 mm. The number of cases scanned by each CT scanner in each hospital is listed in Table 1.
Table 1
Hospital | Brilliance 64, Philips | Aquilion ONE 320, Canon | uCT760, United Imaging |
---|---|---|---|
Hospital 1 | 542 | 391 | 272 |
Hospital 2 | 101 | 0 | 305 |
Hospital 3 | 60 | 0 | 0 |
Hospital 4 | 13 | 17 | 0 |
Hospital 5 | 32 | 0 | 43 |
CT, computed tomography.
Image preprocessing
In order to solely focus on the lung region in the CT images, a DL segmentation model trained with Visual Basic.Net (VB-Net) (11) was used to segment the left and right lungs before lung disease classification. The image preprocessing flowchart is shown in Figure 2. Briefly, the chest CT images were first input into the segmentation model to quickly and accurately obtain the segmentation mask of the left and right lungs. Then, the bounding box of the lung was calculated based on the lung segmentation results. The lung region was subsequently cut out according to the bounding box, and the image block was rescaled to 128×128×128 pixels to ensure that the image size of the lung area obtained from different images was consistent. Finally, the lung window [window width =1,500 Hounsfield units (HU); window length =−400 HU] was used to normalize the image intensity value to [−1, 1] as the input image of the classification model. The segmentation model achieved a Dice similarity coefficient (DSC) of 0.989±0.004. The VB-Net model provided accurate and reliable automated lung segmentation.
The development of the DL model
This study adopted the DL network DenseNet (12) as the classification model, which has been proven to have good classification performance in medical imaging for other diseases (13-15). As shown in Figure 3, the network structure of DenseNet is mainly composed of four dense blocks and a transition layer. Based on the radical dense connection mechanism in the dense block, DenseNet implements feature reuse, using both low-level and high-level features to connect each layer to the previous layers, with errors being easily propagated to the earlier layers. Furthermore, the earlier layer has direct supervision from the final classification layer, alleviating the problems of gradient vanishing.
A 5-fold cross validation method was applied to verify and analyze the model performance. We randomly split the development data into five subsets while ensuring that the distribution of each classification target in each subfold followed the distribution of the collected data set. Each of the 5 subfolds were sequentially used as the test set, and the remaining 4 folds were used for model development, among which 3 subfolds were used for training and 1 for validation. An illustration of subfold distribution and training-validation-testing (internal) partition is shown in Figure 4. Additional data from 165 cases were collected for independent external testing.
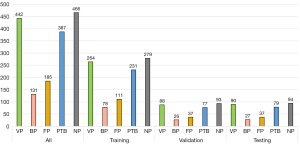
Training setting
The disease classification model was developed based on the PyTorch framework. The training environment consisted of Ubuntu 20.04.1 with 4 NVIDIA Quadro RTX 6000 graphics processing units (GPUs) and CUDA 11.4. In the training process, NP data, PTB data, VP data, BP data, and FP data were respectively labeled as 0, 1, 2, 3, and 4. Based on the image block obtained from preprocessing as training input, the corresponding image label as the training label, and the focal loss (16) were selected as the loss function of network optimization. Adam was used as an optimizer, and its initial learning rate was 1×10−3. We trained the classification model from scratch, set the training epoch to 1,000, calculated the accuracy of the verification set for every 20 epochs, and selected the epoch with the highest accuracy as the final epoch. In addition, different sampling ratios were used proportional to the quantities of five different classes of data to ensure that the same batch could sample the same number of samples for each class during learning. Additionally, random image flipping, rotation, and scaling were used as data augmentation strategies for model training to improve the training network’s robustness.
Radiologist interpretation
Three attending radiologists (with 5, 8, and 8 years of experience in chest CT) and two junior radiologists (both with 2 years of experience in chest CT) reviewed the internal test set of each cross-validation fold (including 327 cases for radiologist 1, 321 cases for radiologist 2, 321 cases for radiologist 3, 321 cases for radiologist 4, and 321 cases for radiologist 5) and the category of pneumonia of each case first without and then with DL model assistance. The radiologists further reviewed the additional data from 165 cases collected for the independent test set first without and then with DL model assistance. All identifying information was removed from the CT studies, which were shuffled and uploaded to 3D Slicer software for interpretation. All radiologists were given information on patient age and sex when reviewing images.
Performance evaluation and statistical analyses
In this study, gender is presented as the male-to-female ratio, and age is presented using the appropriate mean ± standard deviation (SD). The discriminative ability of the DL model was evaluated with precision, recall, F1-score, and weighted F1-average. Weighted F1-average, as a combined metric, was calculated to reduce the imbalanced image distribution in multi-label classification. Receiver operating characteristic (ROC) curves were delineated in each category based on the corresponding probabilities, and the area under the curve (AUC) was subsequently calculated. In addition, 95% confidence intervals (95% CIs) were determined using the adjusted Wald method. DL model performance was compared to radiologist performance with and without DL model assistance. The P values were calculated using the permutation method. Calibration was tested using a calibration plot with bootstraps of 1,000 resamples, which described the degree of fit between actual and DL model-predicted mortality. All statistical analyses were conducted with Python software version: 3.7.0 (Python Software Foundation, Wilmington, DE, USA). A 2-sided P value <0.05 was considered statistically significant. The formulas of F1-score and weighted F1-average were as follows:
Results
Demographic characteristics
A total of 1,611 cases from 2 hospitals were enrolled, comprising 442 VP, 131 BP, 185 FP, 387 PTB, and 466 NP cases. All cases of VP were confirmed by nucleic acid test. All cases of BP met the inclusion criteria. Among the cases of FP, 78 were confirmed by sputum culture, 54 by bronchoalveolar lavage, 32 by serological fungal antigen test, and 21 by lung biopsy. All cases of PTB were confirmed by sputum culture. The mean age was 50.5±17.4 years (range, 18–92 years), and the male-to-female ratio was 1:1.2. For independent testing, 165 cases from 3 hospitals were enrolled, comprising 30 VP, 23 BP, 33 FP, 39 PTB, and 40 NP cases. All cases of VP were confirmed by nucleic acid test. All cases of BP met the inclusion criteria. Among the cases of FP, 9 were confirmed by sputum culture, 12 by bronchoalveolar lavage, 9 by serological fungal antigen test, and 3 by lung biopsy. All cases of PTB were confirmed by sputum culture. The mean age was 44.5±18.9 years (range, 20–90 years), and the male-to-female ratio was 1:1.3.
Evaluation of the DL model and radiologists performance on internal test set
The 5-fold cross-validation average performance of the DL network in the classification of VP, BP, FP, PTB, and NP is summarized in Table 2. For the DL model, the precision of VP, BP, FP, PTB, and NP was 0.946, 0.511, 0.835, 0.845, and 0.978, respectively; the recall was 0.948, 0.511, 0.849, 0.842, and 0.972, respectively; the F1-score was 0.947, 0.511, 0.842, 0.843, and 0.975, respectively; and the weighted F1-average was 0.883. The best F1-score of 0.947 and 0.975 was obtained for VP and NP, respectively. An ideal F1-score of 0.843 and 0.842 was achieved for PTB and FP, respectively. The AUC for identifying VP, BP, FP, PTB, and NP was 0.996, 0.913, 0.963, 0.970, and 0.997, respectively; the sensitivity was 0.948, 0.511, 0.849, 0.842, and 0.972, respectively; and the specificity was 0.980, 0.957, 0.978, 0.951 and 0.991, respectively. The 5-fold cross-validation average classification-related confusion matrix and ROC curve of the testing images are presented in Figure 5A and Figure 5B, respectively. The true positives of VP, BP, FP, PTB, and NP were 94.8% (419/442), 51.1% (67/131), 84.9% (157/185), 84.2% (326/387), and 97.2% (453/466), respectively.
Table 2
Category | Precision | Recall | F1-score | AUC | Weighted F1-average |
---|---|---|---|---|---|
VP | 0.946 (0.925, 0.967) | 0.948 (0.927, 0.969) | 0.947 (0.936, 0.958) | 0.996 (0.993, 0.999) | 0.883 (0.867, 0.898) |
BP | 0.511 (0.426, 0.597) | 0.511 (0.426, 0.597) | 0.511 (0.487, 0.536) | 0.913 (0.899, 0.926) | |
FP | 0.835 (0.782, 0.888) | 0.849 (0.797, 0.900) | 0.842 (0.824, 0.860) | 0.963 (0.953, 0.972) | |
PTB | 0.845 (0.808, 0.881) | 0.842 (0.806, 0.879) | 0.843 (0.826, 0.861) | 0.970 (0.961, 0.978) | |
NP | 0.978 (0.965, 0.992) | 0.972 (0.957, 0.987) | 0.975 (0.968, 0.983) | 0.997 (0.994, 0.999) |
Data in parenthesis are shown as (95% CI). DL, deep learning; AUC, area under the curve; VP, viral pneumonia; BP, bacterial pneumonia; FP, fungal pneumonia; PTB, pulmonary tuberculosis; NP, no pneumonia; CI, confidence interval.
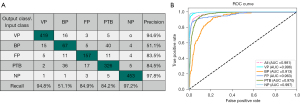
All cases were divided into five parts according to the testing images of 5-fold cross-validation. First, five radiologists (with 2–8 years of experience in thoracic imaging) completed a blind review of the images without the DL model for each part, and then, completed a reevaluation with the DL model. The F1-score of the DL model was much higher than that of the radiologists for VP (0.947 vs. 0.837; ∆=0.110), BP (0.511 vs. 0.356; ∆=0.155), FP (0.842 vs. 0.247; ∆=0.595), PTB (0.843 vs. 0.729; ∆=0.114), and NP (0.975 vs. 0.934; ∆=0.041), as was the weighted F1-average (0.883 vs. 0.732; ∆=0.151). The precision and recall of the DL model for all conditions were higher than those of the radiologists (all P values <0.001), except the recall of BP (0.511 vs. 0.550; ∆=–0.039; P=0.609), which suggested the recall of the DL model for BP was at least not lower than that of the radiologists (Table 3). Assisted by the DL model, the radiologists achieved a higher F1-score for VP (0.922 vs. 0.837; ∆=0.085), BP (0.508 vs. 0.356; ∆=0.152), FP (0.697 vs. 0.247; ∆=0.450), PTB (0.842 vs. 0.729; ∆=0.113), and NP (0.976 vs. 0.934; ∆=0.042) and also a higher weighted F1-average (0.859 vs. 0.732, ∆=0.127). The precision and recall of radiologists with the DL model were all higher than those of the radiologists without the DL model (all P values <0.001), except the recall of BP (P=0.511). The AUC for identifying VP, BP, FP, PTB, and NP was 0.954, 0.762, 0.800, 0.891, and 0.980, respectively (Table 4). Figure 6 shows a calibration plot. This compares the prediction of pneumonia category between the DL model prediction and actual observation. The calibration plot revealed good predictive accuracy of the DL model.
Table 3
Pneumonia category | Index of model performance | Radiologist performance | DL model performance | DL model performance minus radiologist performance | P value |
---|---|---|---|---|---|
VP | Precision | 0.796 (0.760, 0.832) | 0.946 (0.925, 0.967) | 0.150 (0.133, 0.167) | <0.001 |
Recall | 0.882 (0.852, 0.912) | 0.948 (0.927, 0.969) | 0.066 (0.054, 0.078) | <0.001 | |
F1-score | 0.837 (0.819, 0.855) | 0.947 (0.936, 0.958) | 0.110 (0.095, 0.125) | – | |
BP | Precision | 0.263 (0.211, 0.315) | 0.511 (0.426, 0.597) | 0.248 (0.227, 0.269) | <0.001 |
Recall | 0.550 (0.464, 0.635) | 0.511 (0.426, 0.597) | −0.039 (−0.048, −0.030) | 0.609 | |
F1-score | 0.356 (0.332, 0.379) | 0.511 (0.487, 0.536) | 0.155 (0.137, 0.173) | – | |
FP | Precision | 0.432 (0.320, 0.545) | 0.835 (0.782, 0.888) | 0.403 (0.379, 0.427) | <0.001 |
Recall | 0.173 (0.118, 0.227) | 0.849 (0.797, 0.900) | 0.676 (0.653, 0.699) | <0.001 | |
F1-score | 0.247 (0.226, 0.268) | 0.842 (0.824, 0.860) | 0.595 (0.571, 0.619) | – | |
PTB | Precision | 0.779 (0.735, 0.823) | 0.845 (0.808, 0.881) | 0.066 (0.054, 0.078) | <0.001 |
Recall | 0.685 (0.638, 0.731) | 0.842 (0.806, 0.879) | 0.157 (0.139, 0.175) | <0.001 | |
F1-score | 0.729 (0.707, 0.751) | 0.843 (0.826, 0.861) | 0.114 (0.098, 0.130) | – | |
NP | Precision | 0.970 (0.954, 0.986) | 0.978 (0.965, 0.992) | 0.008 (0.004, 0.012) | <0.001 |
Recall | 0.901 (0.874, 0.928) | 0.972 (0.957, 0.987) | 0.071 (0.058, 0.084) | <0.001 | |
F1-score | 0.934 (0.922, 0.946) | 0.975 (0.968, 0.983) | 0.041 (0.031, 0.051) | – | |
All | Weighted F1-average | 0.732 (0.711, 0.754) | 0.883 (0.867, 0.898) | 0.151 (0.134, 0.168) | – |
Data in parenthesis are shown as (95% CI). DL, deep learning; VP, viral pneumonia; BP, bacterial pneumonia; FP, fungal pneumonia; PTB, pulmonary tuberculosis; NP, no pneumonia; CI, confidence interval.
Table 4
Pneumonia category | Index of model performance | Radiologists performance without DL model assistance | Radiologists performance with DL model assistance | Radiologists with DL model assistance minus radiologists without DL model assistance | P value |
---|---|---|---|---|---|
VP | Precision | 0.796 (0.760, 0.832) | 0.896 (0.868, 0.923) | 0.100 (0.085, 0.115) | <0.001 |
Recall | 0.882 (0.852, 0.912) | 0.950 (0.930, 0.971) | 0.068 (0.056, 0.080) | <0.001 | |
F1-score | 0.837 (0.819, 0.855) | 0.922 (0.909, 0.935) | 0.085 (0.071, 0.099) | – | |
BP | Precision | 0.263 (0.211, 0.315) | 0.448 (0.373, 0.522) | 0.185 (0.166, 0.204) | <0.001 |
Recall | 0.550 (0.464, 0.635) | 0.588 (0.503, 0.672) | 0.038 (0.029, 0.047) | 0.511 | |
F1-score | 0.356 (0.332, 0.379) | 0.508 (0.484, 0.533) | 0.152 (0.134, 0.170) | – | |
FP | Precision | 0.432 (0.320, 0.545) | 0.793 (0.727, 0.859) | 0.361 (0.338, 0.384) | <0.001 |
Recall | 0.173 (0.118, 0.227) | 0.622 (0.552, 0.692) | 0.449 (0.425, 0.473) | <0.001 | |
F1-score | 0.247 (0.226, 0.268) | 0.697 (0.675, 0.719) | 0.450 (0.426, 0.474) | – | |
PTB | Precision | 0.779 (0.735, 0.823) | 0.860 (0.825, 0.895) | 0.081 (0.068, 0.094) | <0.001 |
Recall | 0.685 (0.638, 0.731) | 0.824 (0.786, 0.862) | 0.139 (0.122, 0.156) | <0.001 | |
F1-score | 0.729 (0.707, 0.751) | 0.842 (0.824, 0.860) | 0.113 (0.098, 0.128) | – | |
NP | Precision | 0.970 (0.954, 0.986) | 0.989 (0.979, 0.999) | 0.019 (0.012, 0.026) | <0.001 |
Recall | 0.901 (0.874, 0.928) | 0.964 (0.946, 0.981) | 0.063 (0.051, 0.075) | <0.001 | |
F1-score | 0.934 (0.922, 0.946) | 0.976 (0.969, 0.984) | 0.042 (0.032, 0.052) | – | |
All | Weighted F1-average | 0.732 (0.711, 0.754) | 0.859 (0.842, 0.876) | 0.127 (0.111, 0.143) | – |
Data in parenthesis are shown as (95% CI). DL, deep learning; VP, viral pneumonia; BP, bacterial pneumonia; FP, fungal pneumonia; PTB, pulmonary tuberculosis; NP, no pneumonia; CI, confidence interval.
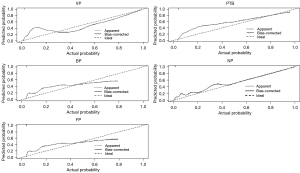
Figures 7,8 show the classification-related confusion matrix and ROC curve of the average results of the five radiologists without and with DL model assistance in the testing images, respectively. Figure 9A-9F show four cases in the test set where the DL model was correct while the radiologists were incorrect.
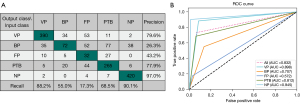
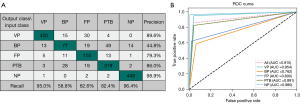
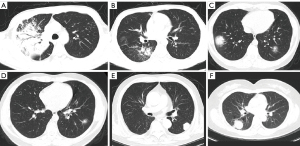
Evaluation of the DL model and radiologists performance on external test set
The performance of the DL model on the external test set for VP, BP, FP, PTB, and NP is summarized in Table 5. For the DL model, the precision for VP, BP, FP, PTB, and NP was 0.933, 0.619, 0.848, 0.795, and 0.952, respectively; the recall was 0.933, 0.565, 0.848, 0.795, and 0.100, respectively; the F1-score was 0.933, 0.591, 0.848, 0.795, and 0.976, respectively; the weighted F1-average was 0.846; the AUC was 0.992, 0.926, 0.949, 0.961, and 0.998, respectively; the sensitivity was 0.933, 0.565, 0.849, 0.795, and 1.000, respectively; and the specificity was 0.985, 0.944, 0.962, 0.937, and 0.984, respectively. The performance of the external test set is comparable to the internal test set. The classification-related confusion matrix and ROC curve of the DL model on the external testing images are displayed in Figure 10A and Figure 10B, respectively.
Table 5
Category | Precision | Recall | F1-score | AUC | Weighted F1-average | Average AUC |
---|---|---|---|---|---|---|
VP | 0.933 (0.893, 0.973) | 0.933 (0.893, 0.973) | 0.933 (0.916, 0.950) | 0.992 (0.986, 0.998) | 0.846 (0.822, 0.871) | 0.967 (0.955, 0.979) |
BP | 0.619 (0.526, 0.712) | 0.565 (0.475, 0.656) | 0.591 (0.557, 0.624) | 0.926 (0.908, 0.944) | ||
FP | 0.848 (0.794, 0.903) | 0.848 (0.794, 0.903) | 0.848 (0.824, 0.873) | 0.949 (0.934, 0.964) | ||
PTB | 0.795 (0.738, 0.852) | 0.795 (0.738, 0.852) | 0.795 (0.767, 0.822) | 0.961 (0.948, 0.974) | ||
NP | 0.952 (0.924, 0.981) | 1.000 (1.000, 1.000) | 0.976 (0.965, 0.986) | 0.998 (0.995, 1.000) |
Data in parenthesis are shown as (95% CI). DL, deep learning; AUC, area under the curve; VP, viral pneumonia; BP, bacterial pneumonia; FP, fungal pneumonia; PTB, pulmonary tuberculosis; NP, no pneumonia; CI, confidence interval.
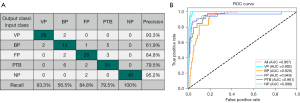
For the radiologists, the F1-score was 0.743, 0.556, 0.541, 0.737, and 0.985 for VP, BP, FP, PTB, and NP, respectively, while the weighted F1-average was 0.734. The DL model achieved a higher F1-score for FP (0.848 vs. 0.541), and the precision for the other three pneumonia conditions was higher (all P values <0.001). With DL model assistance, the radiologists performance for FP was significantly improved (0.778 vs. 0.541), and the precision for the other three pneumonia conditions was also improved (all P values <0.001) (Tables 6,7).
Table 6
Pneumonia category | Index of model performance | Radiologist performance | DL model performance | DL model performance minus radiologist performance | P value |
---|---|---|---|---|---|
VP | Precision | 0.630 (0.565, 0.694) | 0.933 (0.893, 0.973) | 0.303 (0.272, 0.334) | <0.001 |
Recall | 0.907 (0.860, 0.953) | 0.933 (0.893, 0.973) | 0.026 (0.015, 0.037) | 0.523 | |
F1-score | 0.743 (0.713, 0.773) | 0.933 (0.916, 0.950) | 0.190 (0.163, 0.217) | – | |
BP | Precision | 0.511 (0.427, 0.595) | 0.619 (0.526, 0.712) | 0.108 (0.087, 0.129) | <0.001 |
Recall | 0.609 (0.519, 0.698) | 0.565 (0.475, 0.656) | −0.044 (−0.058, −0.030) | 0.551 | |
F1-score | 0.556 (0.522, 0.589) | 0.591 (0.557, 0.624) | 0.035 (0.022, 0.048) | – | |
FP | Precision | 0.767 (0.679, 0.854) | 0.848 (0.794, 0.903) | 0.081 (0.062, 0.100) | <0.001 |
Recall | 0.418 (0.343, 0.493) | 0.848 (0.794, 0.903) | 0.430 (0.396, 0.464) | <0.001 | |
F1-score | 0.541 (0.507, 0.575) | 0.848 (0.824, 0.873) | 0.307 (0.276, 0.338) | – | |
PTB | Precision | 0.764 (0.702, 0.825) | 0.795 (0.738, 0.852) | 0.031 (0.019, 0.043) | <0.001 |
Recall | 0.713 (0.649, 0.776) | 0.795 (0.738, 0.852) | 0.082 (0.063, 0.101) | 0.017 | |
F1-score | 0.737 (0.707, 0.767) | 0.795 (0.767, 0.822) | 0.058 (0.042, 0.074) | – | |
NP | Precision | 0.985 (0.968, 1.000) | 0.952 (0.924, 0.981) | −0.033 (−0.045, −0.021) | 1 |
Recall | 0.985 (0.968, 1.000) | 1.000 (1.000, 1.000) | 0.015 (0.007, 0.023) | 0.25 | |
F1-score | 0.985 (0.977, 0.993) | 0.976 (0.965, 0.986) | −0.009 (−0.015, −0.003) | – | |
All | Weighted F1-average | 0.734 (0.704, 0.764) | 0.846 (0.822, 0.871) | 0.112 (0.009, 0.134) | – |
Data in parenthesis are shown as (95% CI). DL, deep learning; VP, viral pneumonia; BP, bacterial pneumonia; FP, fungal pneumonia; PTB, pulmonary tuberculosis; NP, no pneumonia; CI, confidence interval.
Table 7
Pneumonia category | Index of model performance | Radiologist performance without DL model assistance | Radiologist performance with DL model assistance | Radiologist with DL model assistance minus radiologist without DL model assistance | P value |
---|---|---|---|---|---|
VP | Precision | 0.630 (0.565, 0.694) | 0.788 (0.728, 0.848) | 0.158 (0.133, 0.183) | <0.001 |
Recall | 0.907 (0.860, 0.953) | 0.940 (0.902, 0.978) | 0.033 (0.021, 0.045) | 0.267 | |
F1-score | 0.743 (0.713, 0.773) | 0.857 (0.833, 0.881) | 0.114 (0.092, 0.136) | – | |
BP | Precision | 0.511 (0.427, 0.595) | 0.607 (0.520, 0.693) | 0.096 (0.076, 0.116) | <0.001 |
Recall | 0.609 (0.519, 0.698) | 0.643 (0.556, 0.731) | 0.034 (0.022, 0.046) | 0.585 | |
F1-score | 0.556 (0.522, 0.589) | 0.624 (0.591, 0.658) | 0.068 (0.051, 0.085) | – | |
FP | Precision | 0.767 (0.679, 0.854) | 0.815 (0.753, 0.877) | 0.048 (0.033, 0.063) | <0.001 |
Recall | 0.418 (0.343, 0.493) | 0.745 (0.679, 0.812) | 0.327 (0.295, 0.359) | <0.001 | |
F1-score | 0.541 (0.507, 0.575) | 0.778 (0.750, 0.807) | 0.237 (0.208, 0.266) | – | |
PTB | Precision | 0.764 (0.702, 0.825) | 0.851 (0.798, 0.904) | 0.087 (0.068, 0.106) | <0.001 |
Recall | 0.713 (0.649, 0.776) | 0.759 (0.699, 0.819) | 0.046 (0.032, 0.060) | 0.108 | |
F1-score | 0.737 (0.707, 0.767) | 0.802 (0.775, 0.829) | 0.065 (0.048, 0.082) | – | |
NP | Precision | 0.985 (0.968, 1.000) | 0.990 (0.976, 1.000) | 0.005 (0.000, 0.010) | 1 |
Recall | 0.985 (0.968, 1.000) | 0.985 (0.968, 1.000) | 0.000 (0.000, 0.000) | 1 | |
F1-score | 0.985 (0.977, 0.993) | 0.987 (0.980, 0.995) | 0.002 (−0.001, 0.005) | – | |
All | Weighted F1-average | 0.734 (0.704, 0.764) | 0.828 (0.802, 0.853) | 0.094 (0.074, 0.114) | – |
Data in parenthesis are shown as (95% CI). DL, deep learning; VP, viral pneumonia; BP, bacterial pneumonia; FP, fungal pneumonia; PTB, pulmonary tuberculosis; NP, no pneumonia; CI, confidence interval.
Discussion
In this study, we evaluated the diagnostic ability of DL in discriminating pneumonia caused by different pathogens and assessed radiologists, performance without and with DL model assistance. Different pneumonia conditions, including VP, BP, FP, PTB, and NP, were analyzed. Our results on internal and external test set both yielded excellent values from the DL model for predicting and categorizing pneumonia. Meanwhile, the radiologist achieved a higher performance with DL assistance.
The recent studies on the artificial intelligence (AI)-based differential diagnosis of pneumonia consist mostly of binary classification and mainly report on the discrimination between COVID-19 and other pneumonia types (17-19). Our model attempts to classify four pneumonia categories and normal cases in a single model, and our study showed that AI augmentation significantly improves radiologists’ performance in differentiating types of pneumonia. Bai et al. (20) established and evaluated an AI system for differentiating COVID-19 and other pneumonia on chest CT and assessed radiologist performance with and without AI assistance. The results revealed that the AI model, compared to radiologists, had a higher test accuracy (96% vs. 85%; P<0.001), sensitivity (95% vs. 79%; P<0.001), and specificity (96% vs. 88%; P=0.002), and the radiologists achieved a higher average accuracy with AI assistance. These results are consistent with our study, but Bai et al.’s model is only for binary COVID-19 and other pneumonia classifications. Ibrahim et al. (7) evaluated different DL architectures using public digital chest X-ray and CT datasets with four classes (i.e., normal, COVID-19, pneumonia, and lung cancer) and reported that the VGG (Visual Geometry Group)-19 + convolutional neural network (CNN) model achieved a 0.981 accuracy and a 0.997 AUC based on X-ray and CT images. This study also demonstrated an ideal performance of AI but did not classify pneumonia subtypes in detail. Qi et al. (6) used a CNN-based DL approach for automatically fast-tracking lung tumor lesions and conducting multicategory classification of histological subtypes of lung cancer (including small cell lung cancer, lung adenocarcinoma, and lung squamous cell carcinoma), which attested to the effectiveness of the DL model in multicategory tasks and provided an important reference for our research. Our results also showed that although radiologists’ performance was improved with AI assistance, it still did not surpass—or was even lower than—the lower performance of the DL model. Similar findings have reported in many other areas of AI research (7,21,22), which suggests that the diagnosis of AI should be fully considered in a clinicians’ routine workflow, especially when the treatment is ineffective.
The early diagnosis of FP is challenging in routine clinical work. In our study, the F1-score of radiologists in diagnosing FP was only 0.541 on the external test set, which was much lower than that of the DL model (0.848). However, with DL model assistance, the F1-score increased to 0.778. Recently, Wang et al. (23) used DL to divide pneumonia into three types: BP, FP, and VP. Compared to our model, their model had a similar performance for FP with an F1-score of 0.830 in the test cohort, a superior performance for BP (F1-score: 0.821 vs. 0.591) on the external test set, and similar performance on VP (0.933 vs. 0.895) on the external test set. However, Wang et al. did not enroll PTB and NP cases in their model, thus limiting the spectrum of differential diagnosis. Zhang et al. (24) used DL to divided pneumonia into four types, including BP, FP, VP, and COVID-19, reporting AUCs of 0.989, 0.996, 0.994, and 0.997, respectively. This is similar to our study, but the data volume of FP (n=4) was much smaller than that for other pneumonia types in the testing group, which might have influenced the performance of the model. In addition, the lack of external validation data is also a major limitation of this study.
The precision, recall, and F1-score of our DL model for BP were only between 0.511–0.619 in both the internal and external test set, which were much lower than those of the other categories of the above-mentioned studies. This may due to the small sample size of BP. The diagram of subfold distribution and the training-validation-testing (internal) partition in Figure 4 indicates that there were 78 BP cases for training, 26 for validation, 27 for internal testing, and 23 for external testing. This might have resulted in less attention being paid to this category by the model during training. However, the AUC for BP in our model was high at 0.913 and 0.926, and the specificity was also high at 0.957 and 0.944 in the internal and external test sets, respectively. This suggests our model is able to correctly exclude negative samples from BP, resulting in high specificity and high AUC values. Although the F1-score was low for BP, the AUC and specificity were high, and the results for other categories indicated good performance, which suggests that the overall performance of the model is acceptable.
This study has some limitations which should be mentioned. First, although a large sample was included in this study, the sample size of BP and FP groups was small. However, the weighted F1-average was calculated to reduce the imbalanced image distribution in multi-label classification. Second, as we employed a retrospective design; multicenter prospective studies are needed to verify our findings. Finally, our study only included four categories of pneumonia and did not accurately classify pathogens, which should be addressed in future research.
Conclusions
In conclusion, this DL approach is valuable for distinguishing pneumonia caused by different etiologies and can help radiologists make accurate diagnoses. Thus, this DL model for multicategory classification of pneumonia on chest CT has important potential clinical applications and warrants broader application. Clinicians should take AI results into full consideration within their routine workflow.
Acknowledgments
Funding: This work was supported by
Footnote
Reporting Checklist: The authors have completed the TRIPOD reporting checklist. Available at https://qims.amegroups.com/article/view/10.21037/qims-23-1097/rc
Conflicts of Interest: All authors have completed the ICMJE uniform disclosure form (available at https://qims.amegroups.com/article/view/10.21037/qims-23-1097/coif). YG and Ying Shao are consultants and employees of Shanghai United Imaging Intelligence Co., Ltd. The other authors have no conflicts of interest to declare.
Ethical Statement: The authors are accountable for all aspects of the work in ensuring that questions related to the accuracy or integrity of any part of the work are appropriately investigated and resolved. This retrospective study was approved by the Medical Ethics Committee of Shanghai Public Health Clinical Center (No. 2022-S074-02) and was conducted in accordance with the Declaration of Helsinki (as revised in 2013). The need for informed consent was waived due to the retrospective nature of this research.
Open Access Statement: This is an Open Access article distributed in accordance with the Creative Commons Attribution-NonCommercial-NoDerivs 4.0 International License (CC BY-NC-ND 4.0), which permits the non-commercial replication and distribution of the article with the strict proviso that no changes or edits are made and the original work is properly cited (including links to both the formal publication through the relevant DOI and the license). See: https://creativecommons.org/licenses/by-nc-nd/4.0/.
References
- Grief SN, Loza JK. Guidelines for the Evaluation and Treatment of Pneumonia. Prim Care 2018;45:485-503. [Crossref] [PubMed]
- Available online: https://covid19.who.int/, accessed 20 May 2023.
- Shibly KH, Dey SK, Islam MT, Rahman MM. COVID faster R-CNN: A novel framework to Diagnose Novel Coronavirus Disease (COVID-19) in X-Ray images. Inform Med Unlocked 2020;20:100405. [Crossref] [PubMed]
- Mulrenan C, Rhode K, Fischer BM. A Literature Review on the Use of Artificial Intelligence for the Diagnosis of COVID-19 on CT and Chest X-ray. Diagnostics (Basel) 2022;12:869. [Crossref] [PubMed]
- Elshennawy NM, Ibrahim DM. Deep-Pneumonia Framework Using Deep Learning Models Based on Chest X-Ray Images. Diagnostics (Basel) 2020;10:649. [Crossref] [PubMed]
- Qi J, Deng Z, Sun G, Qian S, Liu L, Xu B. One-step algorithm for fast-track localization and multi-category classification of histological subtypes in lung cancer. Eur J Radiol 2022;154:110443. [Crossref] [PubMed]
- Ibrahim DM, Elshennawy NM, Sarhan AM. Deep-chest: Multi-classification deep learning model for diagnosing COVID-19, pneumonia, and lung cancer chest diseases. Comput Biol Med 2021;132:104348. [Crossref] [PubMed]
- Zeng QQ, Zheng KI, Chen J, Jiang ZH, Tian T, Wang XB, Ma HL, Pan KH, Yang YJ, Chen YP, Zheng MH. Radiomics-based model for accurately distinguishing between severe acute respiratory syndrome associated coronavirus 2 (SARS-CoV-2) and influenza A infected pneumonia. MedComm (2020) 2020;1:240-8. [Crossref] [PubMed]
- Li L, Qin L, Xu Z, Yin Y, Wang X, Kong B, Bai J, Lu Y, Fang Z, Song Q, Cao K, Liu D, Wang G, Xu Q, Fang X, Zhang S, Xia J, Xia J. Using Artificial Intelligence to Detect COVID-19 and Community-acquired Pneumonia Based on Pulmonary CT: Evaluation of the Diagnostic Accuracy. Radiology 2020;296:E65-71. [Crossref] [PubMed]
- Society of Respiratory Diseases, Chinese Medical Association. Guidelines for the diagnosis and treatment of community-acquired pneumonia (one). Clinical Education of General Practice 2007;5:270-2.
- Han M, Zhang Y, Zhou Q, Rong C, Zhan Y, Zhou X, Gao Y. Large-scale evaluation of V-Net for organ segmentation in image guided radiation therapy. Image-Guided Procedures, Robotic Interventions, and Modeling. Medical Imaging 2019.
- Huang G, Liu Z, Maaten LVD, Weinberger KQ. Densely Connected Convolutional Networks. IEEE Conference on Computer Vision and Pattern Recognition; 2017.
- Cheng J, Chen Y, Yu Y, Chiu B. Carotid plaque segmentation from three-dimensional ultrasound images by direct three-dimensional sparse field level-set optimization. Comput Biol Med 2018;94:27-40. [Crossref] [PubMed]
- Wang Y, Zhou C, Chan HP, Hadjiiski LM, Chughtai A, Kazerooni EA. Hybrid U-Net-based deep learning model for volume segmentation of lung nodules in CT images. Med Phys 2022;49:7287-302. [Crossref] [PubMed]
- Li D, Miao H, Jiang Y, Shen Y. A Multi-model Organ Segmentation Method Based on Abdominal Ultrasound Image. 2020 15th IEEE International Conference on Signal Processing (ICSP) IEEE; 2020.
- Lin TY, Goyal P, Girshick R, He K, Dollar P. Focal Loss for Dense Object Detection. IEEE Trans Pattern Anal Mach Intell 2020;42:318-27. [Crossref] [PubMed]
- Ozturk T, Talo M, Yildirim EA, Baloglu UB, Yildirim O, Rajendra Acharya U. Automated detection of COVID-19 cases using deep neural networks with X-ray images. Comput Biol Med 2020;121:103792. [Crossref] [PubMed]
- Ardakani AA, Kanafi AR, Acharya UR, Khadem N, Mohammadi A. Application of deep learning technique to manage COVID-19 in routine clinical practice using CT images: Results of 10 convolutional neural networks. Comput Biol Med 2020;121:103795. [Crossref] [PubMed]
- Yan T, Wong PK, Ren H, Wang H, Wang J, Li Y. Automatic distinction between COVID-19 and common pneumonia using multi-scale convolutional neural network on chest CT scans. Chaos Solitons Fractals 2020;140:110153. [Crossref] [PubMed]
- Bai HX, Wang R, Xiong Z, Hsieh B, Chang K, Halsey K, et al. Artificial Intelligence Augmentation of Radiologist Performance in Distinguishing COVID-19 from Pneumonia of Other Origin at Chest CT. Radiology 2020;296:E156-65. [Crossref] [PubMed]
- Deng J, Zhao M, Li Q, Zhang Y, Ma M, Li C, et al. Implementation of artificial intelligence in the histological assessment of pulmonary subsolid nodules. Transl Lung Cancer Res 2021;10:4574-86. [Crossref] [PubMed]
- Ha EJ, Lee JH, Lee DH, Moon J, Lee H, Kim YN, Kim M, Na DG, Kim JH. Artificial Intelligence Model Assisting Thyroid Nodule Diagnosis and Management: A Multicenter Diagnostic Study. J Clin Endocrinol Metab 2023; Epub ahead of print. [Crossref] [PubMed]
- Wang F, Li X, Wen R, Luo H, Liu D, Qi S, Jing Y, Wang P, Deng G, Huang C, Du T, Wang L, Liang H, Wang J, Liu C. Pneumonia-Plus: a deep learning model for the classification of bacterial, fungal, and viral pneumonia based on CT tomography. Eur Radiol 2023; Epub ahead of print. [Crossref] [PubMed]
- Zhang YH, Hu XF, Ma JC, Wang XQ, Luo HR, Wu ZF, Zhang S, Shi DJ, Yu YZ, Qiu XM, Zeng WB, Chen W, Wang J. Clinical Applicable AI System Based on Deep Learning Algorithm for Differentiation of Pulmonary Infectious Disease. Front Med (Lausanne) 2021;8:753055. [Crossref] [PubMed]