Diffusion along the perivascular space as a potential biomarker for glioma grading and isocitrate dehydrogenase 1 mutation status prediction
Introduction
Gliomas are the most common primary tumors of the central nervous system (1) and have been traditionally classified into grades I–IV according to the World Health Organization (WHO) based on histopathologic features (2). Since 2016, the central nervous system tumor classification added the isocitrate dehydrogenase 1 (IDH1) genotype for classification (2). Numerous previous studies have shown that gliomas with the IDH1 mutation have a better prognosis than do gliomas with the wild-type IDH1 gene (3,4). Thus, accurate glioma grading and genotype prediction before surgery are necessary for the treatment and prognosis of gliomas.
The glymphatic system is a waste drainage system in the brain which functions via aquaporin-4 (AQP4) water channels in astrocytic end-feet (5). A few recent studies have examined the relationship between the function of the glymphatic system and gliomas (6-8). In rodent models of glioma, the outflow of cerebrospinal fluid was found to be reduced, with the dorsal meningeal lymphatic vessels being extensively remodeled (6,7). In addition, impaired glymphatic function potentially allows for the accumulation of toxic proteins, cytokines, and chemokines, which may further promote tumor growth (9,10). As gliomas exhibit varying degrees of malignancy and aggressiveness, they may in turn cause varying degrees of damage and repair to the glymphatic system. Accordingly, we hypothesized that there may be differences in glymphatic function across grades and across IDH1 mutation statuses.
Diffusion tensor image analysis along the perivascular space (DTI-ALPS) method has been recently proposed for noninvasively detecting glymphatic system function alteration (11). The perivascular space is an important part of the glymphatic pathway, and its diffusivity in the direction of the in the periventricular white matter can indirectly indicate the state of glymphatic function. Thus, the ALPS-index has been applied as a potential biomarker for glymphatic system function in recent studies (12-14). The ALPS-index is a metric calculated at the level of the lateral ventricle body. It is based on the fact that the perivascular space lies largely orthogonal to the projection and association fibers in this area. In addition, DTI and diffusion kurtosis imaging (DKI) have been widely used for noninvasive glioma grading and identification of IDH1 mutation status (15-18). Most conventional diffusion metrics are measured in the tumor parenchyma, but the ALPS-index is measured in the peritumor areas, which may provide additional pathophysiological information complementary to these metrics for glioma diagnosis and prognosis.
Therefore, the purpose of this study was to comprehensively evaluate the glymphatic dysfunction in patients with glioma using the DTI-ALPS method, evaluate the role of ALPS-index in glioma grading and IDH1 mutation status prediction, and compare the diagnostic efficacy of ALPS-index with conventional DTI- and DKI-derived metrics.
Methods
Participants
This retrospective study was conducted in accordance with the Declaration of Helsinki (as revised in 2013) and approved by the Institutional Review Board of Tongji Hospital of Tongji Medical College of Huazhong University of Science and Technology (No. TJ-IRB202303157). Written informed consent was obtained from all patients.
The image data of consecutive patients admitted to our hospital between March 2017 to July 2021 were retrospectively analyzed. The inclusion criteria were as follows: (I) histopathologically confirmed glioma; (II) patients who underwent preoperative DTI examination; and (III) no chemotherapy or radiotherapy before examination. The exclusion criteria were as follows: (I) magnetic resonance imaging (MRI) images with artifacts or poor quality; (II) unknown IDH1 mutation status; (III) lesions extending across the midline and bilaterally occupying the cerebral hemispheres; and (IV) purely cystic glioma or glioma with severe destruction of the lateral ventricular structures. Ultimately, 81 patients and 31 healthy controls were recruited for analysis and matched in terms of age and gender. The screening process for participants is shown in Figure 1.
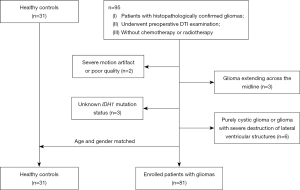
Data acquisition
All images were acquired from a 3.0 T MR scanner (Discovery MR750, GE HealthCare, Chicago, IL, USA) with a 32-channel head coil. The conventional MRI sequence included T1 fluid-attenuated inversion recovery (T1-FLAIR), T2 fast spin echo (T2-FSE), and T2-FLAIR and contrast-enhanced T1 (CE-T1). Diffusion MRI was performed under the following parameters: b=0, 1,250, and 2,500 s/mm2; 25 gradient directions; repetition time =6,500 ms; echo time =85 ms; number of excitations =1, field of view =24×24 cm2; matrix size =128×128; and slice thickness =3 mm without slice spacing.
Data processing and analysis
Diffusion image preprocessing was performed using the TractoFlow pipeline. The diffusion imaging data were first denoised using Marchenko-Pastur principal component analysis (MP-PCA) (19) and corrected for Gibbs ringing artifacts using Mrtrix3 (https://www.mrtrix.org). Subsequently, correction for motion and eddy current artifacts, along with skull stripping, was performed using the FMRIB Software Library (FSL) (version 6.0.1, University of Oxford, Oxford, UK). N4 correction was performed with b=0 mm2/s using the Advanced Normalization Tools (ANTs) command (N4BiasFieldCorrection algorithm). The bias field was then applied to the whole set of diffusion imaging data. The DTI model was applied to fit fractional anisotropy (FA), mean diffusivity (MD), and diffusion tensors along the x-, y-, and z -axis by using FSL DTIFIT command with a single shell (b=1,250 s/mm2). The DKI model was applied to fit mean kurtosis (MK) with the DIPY Toolbox (https://www.dipy.org) with two shells (b=1,250 and 2,500 s/mm2).
Two neuroradiologists (each with 5 years of experience) who were blinded to clinical data placed the regions of interest (ROIs) independently. According to anatomic images, neuroradiologists manually drew 3 to 6 circular ROIs with a 6-mm diameter on the enhanced tumor parenchyma (assumed to be tumor the core). For non-enhancing tumors, ROIs were placed based on T2-FLAIR hyperintensity. The peritumoral edema area corresponded to the regions within 1 cm of the peritumoral parts (hyperintense on FLAIR imaging but no enhancement on CE-T1-weighted imaging) (20). Necrotic, hemorrhagic, and cystic components were avoided. The measurements of FA, MD, and MK were calculated as the average values of the ROIs.
ROIs for DTI-ALPS analysis were placed at the layer of the lateral ventricle body by the two neuroradiologists mentioned above. In this region, the perivascular spaces (x-axis) nearly run perpendicular to the association (y-axis) and projection fibers (z-axis). The major differences in water molecule behavior between x-axis diffusivity in projection fibers (Dxproj) and association fibers (Dxassoc) and the diffusivity along the y-axis in the region of projection fibers (Dyproj) and z-axis diffusivity in the region of association fibers (Dzassoc) were derived from the perivascular space. Thus, the ALPS-index was calculated using the following formula:
Circular ROIs with a 5-mm diameter were drawn on the association fibers and projection fibers on both hemispheres at the level of the lateral ventricular body according to a color-coded V1 map. The direction of medullary veins is homologous at the level of the lateral ventricle body (21). Therefore, it is not difficult to place ROIs in the proper position. An example of ROI placement is shown in Figure 2.
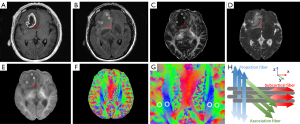
Evaluation of tumor grade and IDH1 mutation status
The tumor grade was diagnosed according to the WHO 2016 classification of tumors (2). Although the fifth edition of the classification was recently released with some changes (22), our study was retrospective in nature and patients were included before the release of the new version, and thus WHO 2016 was still used in this study. The IDH1 mutation status was determined with next-generation sequencing or immunohistochemistry.
Statistical analysis
All statistical analyses were performed with SPSS version 21 (IBM Corp., Armonk, NY, USA), MedCalc version 18 (MedCalc Software, Ostend, Belgium), and R version 4.1.3 (The R Foundation for Statistical Computing) software. Interobserver reliability was measured using the intraclass correlation coefficient (ICC), and the distribution normality of continuous variables was assessed with the Kolmogorov-Smirnov test. The chi-squared test and 1-way analysis of variance (ANOVA) were used to test the demographic characteristics.
The differences in ALPS-index between bilateral hemispheres were compared with the paired t-test. Since the diagnostic efficacy of FA, MD, and MK may be age-related (23,24) and since the ALPS-index was also found to be negatively correlated with age (25), the analysis of covariance (ANCOVA) with age adjustment was used to compare the differences in metrics between patients and healthy controls, between different grades of glioma, and between different IDH1 genotypes. Bonferroni correction was used for multiple comparisons. To investigate whether the combination of metrics could provide better diagnostic performance, a binary logistic regression analysis was conducted. Receiver operating characteristic (ROC) curves were used to calculate the area under the curve (AUC), sensitivity, specificity, and the cutoff value. The AUCs of metrics and combinations were compared using the DeLong test. Finally, the integrated discrimination improvement (IDI) indices were calculated to evaluate the added value of the combined models, with a positive IDI value indicating improved discrimination (26). P<0.05 was considered to be statistically significant.
Results
Demographic characteristics of participants
The demographic and clinical characteristics of 81 patients with glioma and 31 healthy controls are summarized in Table 1. There were 45 lower-grade gliomas (LrGGs) (20 grade II astrocytomas, 8 grade II oligodendrogliomas, 13 grade III anaplastic astrocytomas, 4 grade III anaplastic oligodendrogliomas) and 36 glioblastomas (GBMs; grade IV). Age and gender were not significantly different between the patients and controls (P=0.318 and P=0.139, respectively). There were significant differences in IDH1 mutation status (P<0.001) but not in lesion distribution (P=0.168) among patients with grade II–IV gliomas.
Table 1
Characteristic | LrGG | GBM | Healthy control (N=31) | P value | ||
---|---|---|---|---|---|---|
Grade II (N=28) | Grade III (N=17) | Grade IV (N=36) | ||||
Age (years) | 43.93±10.50 | 46.12±14.23 | 49.17±11.06 | 45.48±11.34 | 0.318 | |
Gender (female) | 12 (42.9) | 11 (64.7) | 23 (63.9) | 18 (58.1) | 0.139 | |
Hemisphere (left) | 17 (60.7) | 12 (70.6) | 16 (44.4) | – | 0.168 | |
IDH1 | <0.001 | |||||
IDH1(–) | 6 (21.4) | 6 (35.3) | 27 (75.0) | – | ||
IDH1(+) | 22 (78.6) | 11 (64.7) | 9 (25.0) | – |
Data are presented as mean ± standard deviation for continuous variables. Data are presented as numbers and frequencies for categorical variables. IDH1, isocitrate dehydrogenase 1; LrGG, lower-grade glioma; GBM, glioblastoma.
Repeatability of measurement
The interobserver variability of measurements is reported in Table S1. The ICCs of ALPS-index, FA, MD, and MK ranged from 0.809 to 0.972, showing excellent repeatability between the two observers (27). Thus, the final values of all measurements were calculated by averaging two independent measurements.
Differences in the ALPS-index between cerebral hemispheres and between patients and healthy controls
The results of comparisons of the ALPS-index between the cerebral hemispheres and between patients and healthy controls are presented in Figure 3. There was no significant difference in the ALPS-index between the left and right hemispheres (1.574±0.173 vs. 1.581±0.167; P=0.604) in healthy controls. In all patients with gliomas, the ALPS-index of the hemisphere ipsilateral to glioma was significantly lower than that of the contralateral hemisphere (1.417±0.177 vs. 1.478±0.165; P=0.002). Furthermore, the ALPS-index of the hemisphere ipsilateral to the tumor (left-sided glioma: 1.437±0.181; right-sided glioma: 1.392±0.171) and even the ALPS-index values of the contralateral hemisphere (left-sided glioma: 1.498±0.165; right-sided glioma: 1.454±0.165) were significantly decreased in patients with either left-sided or right-sided gliomas compared to those of the corresponding hemisphere in healthy controls (all P values <0.05). The ALPS-index of the hemisphere ipsilateral to the tumor showed no significant difference between patients with left-sided and right-sided glioma (1.437±0.181 vs. 1.392±0.171; P=0.258). Therefore, in the subsequent analysis, we did not distinguish if the tumor was located in the left or the right hemisphere.
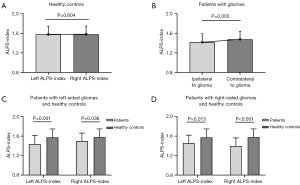
Correlation of metrics with glioma grade
The results of the differences in metrics between LrGGs and GBMs are shown in Figure 4A. For the tumor parenchyma, LrGGs, as compared to GBMs, had a significantly higher ALPS-index (ALPS-index: 1.495±0.151 vs. 1.320±0.159; P<0.001) and MD [(1.340±0.373) vs. (0.975±0.257)×10−3 mm2/s; P<0.001) but a significantly lower FA (0.123±0.052 vs. 0.166±0.103; P=0.021) and MK (0.506±0.155 vs. 0.686±0.172; P<0.001). The diffusion metrics in the peritumoral edema regions showed no significant difference between the LrGGs and GBMs [FA: 0.175±0.068 vs. 0.191±0.077, P=0.309; MD: (1.447±0.245) vs. (1.377±0.303)×10−3 mm2/s, P=0.234; MK: 0.504±0.086 vs. 0.547±0.110, P=0.094]. In addition, the differences in metrics for differentiating LrGGs from GBMs were further compared among grade II, III, and IV gliomas, as shown in Figure 4B and Table S2. The ALPS-index, MD, and MK were significantly different across the three groups.
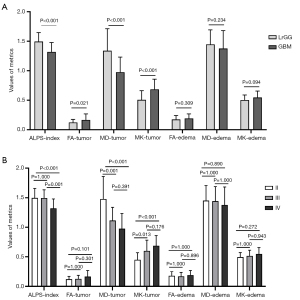
Table 2 and Figure 5A,5B show the results of ROC analyses for differentiating LrGGs from GBMs. In univariate analysis, MD showed the highest AUC of 0.807, followed by MK (0.794) and ALPS-index (0.794), and FA had a relatively low AUC of 0.614. The ALPS-index combined with FA, MD, or MK (Table S3) showed increased AUCs of 0.823, 0.854, and 0.854, respectively. The combination of FA and ALPS-index significantly improved the diagnostic efficacy compared with FA alone (P=0.002). However, the combination of MD and ALPS-index and the combination of MK and ALPS-index yielded no statistically significant improvement in diagnostic efficacy (P=0.259 and P=0.102, respectively). Although the Delong test showed no significant AUC difference for some combined models, significant improvement in discrimination (IDI: P<0.05) could be obtained by adding the APLS-index to other diffusion metrics. The ROC curves for the comparations among grade II, III, and IV gliomas are shown in Figure S1, and their corresponding diagnostic characteristics are shown in Table S4.
Table 2
Metric | AUC (95% CI) | Cutoff value | Sensitivity (%) | Specificity (%) | IDI |
---|---|---|---|---|---|
ALPS-index | 0.794 (0.694–0.895) | 1.381 | 72.22 | 80.00 | – |
FA | 0.614 (0.486–0.741) | 0.138 | 52.78 | 71.11 | – |
FA + ALPS-index | 0.823 (0.731–0.915) | – | 80.56 | 73.33 | 0.237 (P<0.001) |
MD | 0.807 (0.709–0.905) | 1.054 | 72.22 | 86.67 | – |
MD + ALPS-index | 0.854 (0.774–0.935) | – | 83.33 | 80.00 | 0.123 (P=0.004) |
MK | 0.794 (0.696–0.893) | 0.514 | 86.11 | 64.44 | – |
MK + ALPS-index | 0.854 (0.772–0.935) | – | 91.67 | 71.11 | 0.115 (P=0.005) |
MD is in units of 10−3 mm2/s. LrGG, lower-grade glioma; GBM, glioblastoma; AUC, area under the curve; CI, confidence interval; IDI, integrated discrimination index; ALPS, analysis along the perivascular space; FA, fractional anisotropy; MD, mean diffusivity; MK, mean kurtosis.
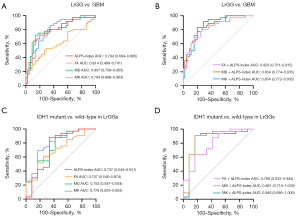
Correlation of metrics with IDH1 mutation status
Table 3 displays the differences in metrics between the IDH1-mutant group and the wild-type group in both LrGGs and GBMs. In LrGGs, the ALPS-index and MD of tumor parenchyma were significantly lower in the IDH1 wild-type group than in the IDH1-mutant group (ALPS-index: P=0.036; MD: P=0.019), while the FA and MK of the tumor parenchyma in the IDH1-wild-type group were significantly higher than those in the IDH1-mutant group (FA: P=0.015; MK: P=0.003). However, there was no significant difference in these metrics between IDH1-wild-type GBMs and IDH1-mutant GBMs (all P values >0.05). In addition, none of the peritumoral diffusion metrics showed a significant difference between IDH1 mutation statuses in either LrGGs and GBMs.
Table 3
Metric | IDH1 wild-type | IDH1 mutant | P value |
---|---|---|---|
LrGG | |||
ALPS-index | 1.400±0.185 | 1.530±0.123 | 0.036 |
FA-tumor | 0.153±0.060 | 0.112±0.046 | 0.015 |
MD-tumor | 1.103±0.313 | 1.426±0.359 | 0.019 |
MK-tumor | 0.627±0.193 | 0.462±0.114 | 0.003 |
FA-edema | 0.193±0.076 | 0.169±0.065 | 0.364 |
MD-edema | 1.332±0.340 | 1.485±0.199 | 0.144 |
MK-edema | 0.549±0.118 | 0.489±0.068 | 0.129 |
GBM | |||
ALPS-index | 1.300±0.169 | 1.380±0.110 | 0.175 |
FA-tumor | 0.174±0.111 | 0.142±0.075 | 0.448 |
MD-tumor | 0.985±0.247 | 0.947±0.300 | 0.781 |
MK-tumor | 0.685±0.170 | 0.690±0.187 | 0.999 |
FA-edema | 0.194±0.081 | 0.183±0.071 | 0.736 |
MD-edema | 1.421±0.279 | 1.249±0.350 | 0.186 |
MK-edema | 0.535±0.096 | 0.579±0.143 | 0.274 |
Data are presented as mean ± standard deviation. MD is in units of 10−3 mm2/s. IDH1, isocitrate dehydrogenase 1; LrGG, lower-grade glioma; GBM, glioblastoma; ALPS, analysis along the perivascular space; FA, fractional anisotropy; MD, mean diffusivity; MK, mean kurtosis.
The ROC curves for metrics in predicting IDH1 mutation are shown in Figure 5C,5D, and the corresponding AUCs, sensitivities, and specificities are summarized in Table 4. In univariate analysis, MK showed the highest AUC of 0.778, followed by MD (0.753), ALPS-index (0.737), and FA (0.707). The ALPS-index combined with FA, MD, and MK (Table S5) showed improved AUCs of 0.788, 0.861, and 0.846, respectively. Although the differences in AUCs were not significant (P=0.397, P=0.133, and P=0.444, respectively), all improvements in discrimination were significant (IDI: all P values <0.05).
Table 4
Metric | AUC (95% CI) | Cutoff value | Sensitivity (%) | Specificity (%) | IDI |
---|---|---|---|---|---|
ALPS-index | 0.737 (0.544, 0.931) | 1.406 | 87.88 | 66.67 | – |
FA | 0.707 (0.540, 0.874) | 0.117 | 60.61 | 75.00 | – |
FA + ALPS-index | 0.788 (0.632, 0.943) | – | 63.64 | 83.33 | 0.160 (P=0.045) |
MD | 0.753 (0.581, 0.924) | 1.093 | 87.88 | 58.33 | – |
MD + ALPS-index | 0.861 (0.710, 1.000) | – | 90.91 | 83.33 | 0.236 (P=0.002) |
MK | 0.778 (0.603, 0.953) | 0.496 | 69.70 | 83.33 | – |
MK + ALPS-index | 0.846 (0.685, 1.000) | – | 90.91 | 83.33 | 0.146 (P=0.017) |
MD is in units of 10−3 mm2/s. IDH1, isocitrate dehydrogenase 1; LrGG, lower-grade glioma; AUC, area under the curve; CI, confidence interval; IDI, integrated discrimination index; ALPS, analysis along the perivascular space; FA, fractional anisotropy; MD, mean diffusivity; MK, mean kurtosis.
Correlation among the metrics
The ALPS-index was significantly correlated with MD (rho =0.324; P=0.003) and MK (rho =–0.382; P<0.001). However, the correlation between the ALPS-index and FA was not significant (rho =–0.103; P=0.361). Moreover, none of the diffusion metrics in peritumoral region were correlated with the ALPS-index (FA: rho =–0.181, P=0.131; MD: rho =0.049, P=0.685; MK: rho =–0.200, P=0.095). These results are shown in Figure 6.

Discussion
Our results demonstrated that in patients with gliomas, the ALPS-index of the hemisphere ipsilateral to glioma was significantly lower than that of the contralateral hemisphere, and bilateral ALPS-index values in patients were significantly decreased compared with those in healthy controls. These findings may reflect the impairment of the glymphatic system in patients with glioma. The ALPS-index showed good efficacy in glioma grading and predicting IDH1 mutation status. In addition, our results showed that the ALPS-index improved diagnostic ability when added to other conventional diffusion metrics.
The ALPS-index reflects the diffusivity along the perivascular spaces of the medullary veins, which is an important part of the glymphatic system. Thus, a decreased ALPS-index value might partly indicate an impaired glymphatic system in patients with glioma, as observed in rodent experiments in which glioma could induce the reduction of cerebrospinal fluid outflow (6,8). It is also worth noting that the ALPS-index in the contralateral hemisphere of patients in our study was also significantly decreased compared with that in healthy controls. These findings indicate that the glioma might also induce glymphatic dysfunction in the contralateral hemisphere, with the glioma-induced global vasogenic edema potentially being the primary cause (28). In addition, some studies have proposed that gliomas can infiltrate into the contralateral normal-appearing white matter (29,30). However, due to the lack of histopathological confirmation, we cannot directly attribute the observed change to global vasogenic edema or tumor infiltration although this change of ALPS-index was observed in our study.
As it relates to lesion type, the ALPS-index of LrGGs was significantly higher than that of GBMs, which might suggest that LrGGs have better glymphatic function than do GBMs. In one experiment, glioma was found to induce the remodeling of glymphatic pathways in glioma-bearing mice (7). Given that LrGGs exhibit lower aggressiveness and typically result in longer survival time (31), it is reasonable to assume that the glymphatic system of LrGGs may endure less-severe destruction and be capable of maintain function through more extensive remolding. We also found that the IDH1-wild-type LrGGs had a lower ALPS-index as compared with the IDH1-mutant LrGGs, which suggests that the wild-type IDH1 gene might induce more severe dysfunction of the glymphatic system. Toh et al. (32) observed similar results and speculated that reduced glymphatic function of IDH1-wild-type LrGGs may be associated with greater aggressiveness and faster tumor growth, thus making remodeling of the glymphatic pathway and compensation of function more difficult. However, the mechanism for this is unclear, and further animal or human studies are needed.
The changes in diffusion metrics in peritumoral regions may be induced by both increased water content and tumor infiltration (33). In our study, we also analyzed diffusion metrics in peritumoral edema, but no significant difference was found between groups, which is consistent with previous studies (20,23) and might be related to the small sample size and tumor heterogeneity of the study. Additionally, nonsignificant correlations between ALPS and MD and MK were observed in our study. Since previous studies have found that AQP4 expression is negatively correlated with MD and positively correlated with MK (34,35), there might also be a potential correlation between ALPS-index and AQP4 expression in patients with glioma. The expression of AQP4 is upregulated as a reaction to glioma-associated edema (36,37), and the formation of peritumoral edema indirectly reflects the insufficient clearance of interstitial fluid and the disruption of the glymphatic system (32).
DTI- and DKI-derived metrics can be used for glioma grading and IDH1 mutation status prediction. Although imaging features such as enhancement and edema are widely used in traditional neurosurgical estimation, the extent of enhancement is not always reliable for distinguishing glioma grades (38). Advanced diffusion MRI techniques offer clinically relevant physiological data that are not obtainable via conventional MRI, resulting in more accurate glioma diagnosis. GBMs often have pseudopalisading structures with obvious vascular endothelial hyperplasia, which may result in increased FA values (39). However, this increase may be confused with changes induced by peritumoral edema, which shows tends to have higher FA compared to tumor parenchyma (20,40). With increasing tumor grade, GBMs exhibit increased tumor cellularity and caryoplasm ratios, which restrict the diffusion of water molecules and decrease MD values (24,41). MK may better reflect the complexity of microstructures under non-Gaussian distribution in an organism (15), and a higher MK reflects higher tissue complexity, including increased cell density, hemorrhage, and necrosis. In contrast, a lower MK reflects a higher number of homogeneous nests and well-differentiated cells (42,43). In agreement with previous studies, we also found significantly higher FA, MK, and lower MD values in IDH1-mutant LrGGs compared to IDH1-wild-type LrGGs (43,44). Similar to GBMs, LrGGs in the in IDH1-mutant group were less aggressive than in the wild-type-IDH1 group, the latter of which had a higher degree of microstructural complexity. In contrast, IDH1 mutation can inhibit cellular proliferation, leading to fewer diffusion restrictions and less complexity (45,46). However, no metrics were significantly different between IDH1 mutation statuses in GBMs possibly because GBMs have complex microstructures, perfusions, and other molecular changes besides IDH1 mutation (47).
In ROC analyses, the ALPS-index demonstrated equivalent efficacy compared to conventional DTI- and DKI-derived metrics in diagnosing glioma grade and IDH1 mutation status. It is worth noting that although significant differences in ALPS-index between groups might enhance the understanding of the disease progression pattern, there was some degree of overlap in the ALPS-index values between groups. This indicates that additional caution may be necessary when the ALPS-index is applied alone in individual diagnosis. Furthermore, a valuable finding was that the combination models further improved the discrimination abilities in glioma grading and IDH1 genotyping, which may suggest that conventional DTI and DKI metrics mostly measured in the areas of tumor parenchyma may only reflect the change of intertumoral microstructure (43). Meanwhile, ALPS-index measured outside the tumor may reflect additional pathophysiological information (11,32) and may act complementarily to conventional diffusion metrics, with their combination more comprehensively reflecting the microenvironment change. Furthermore, as the image acquisition time of DTI is acceptable in clinical practice and automated calculation techniques are being developed (48,49), the application of ALPS-index may provide more convenience in clinic.
There are some limitations in this study. First, a single-center design was employed, and the sample size was small. It is reasonable to assume that the different tumor locations might have different impacts on the glymphatic system. However, our sample size was insufficiently large for analyzing tumor location in more refined subgroupings. Considering the imbalanced distribution of IDH1 mutation status in different grades, we believe a larger sample size is needed in our future study. Second, although radiologists were experienced and ROIs were carefully drawn, subjectivity was inevitable, especially for GBMs with high heterogeneity. Generally, applying multiple ROIs can reduce the biases of subjective evaluation. Finally, although DTI-ALPS has been used in many studies such as Alzheimer disease (13), Parkinson disease (14,50), and normal pressure hydrocephalus (51) to evaluate the function of the glymphatic system, the relationship between the ALPS-index and gold standard contrast-based glymphatic imaging has not yet been substantially validated. Thus, the mechanism underlying the function of this deductive, model-based method should be further investigated.
Conclusions
A lower ALPS-index in patients with glioma may reflect the impairment of the glymphatic system, with these changes potentially being bilateral. In addition, the ALPS-index could provide peritumoral pathophysiological information and complement conventional diffusion metrics for glioma grading and IDH1 genotyping. Thus, the ALPS-index has considerable potential as a novel imaging biomarker for gliomas. The key findings of this study are summarized in Table 5.
Table 5
Metrics | Model | Potential pathophysiological implications | Correlation with tumor grade | Correlation with IDH1 mutation status | Value in diagnosis |
---|---|---|---|---|---|
ALPS-index | DTI | Potentially correlated with the function of the glymphatic system | Gliomas can lead to decrease in the ALPS-index, and patients with GBMs have a lower ALPS-index than do patients with LrGGs | Patients with IDH1-wild-type gliomas have a lower ALPS-index than do patients with IDH1 mutant gliomas | The ALPS-index could improve the diagnostic ability when added to other conventional diffusion metrics |
FA | The measurement of the directionality of molecular motion, which can reflect the white matter integrity in brain | GBMs have a higher FA than do LrGGs | IDH1-wild-type gliomas have a higher FA | These conventional DTI- and DKI-derived metrics have been used in glioma grading and IDH1 genotyping | |
MD | The rate of water molecules’ diffusional motion, which correlates with tumor cellularity | GBMs have a lower MD than do LrGGs | IDH1-wild-type gliomas have a lower MD | ||
MK | DKI | Can provide the kurtosis of water diffusion under non-Gaussian distribution in an organism | GBMs have a higher MK than do LrGGs | IDH1-wild-type gliomas have a higher MK |
IDH1, isocitrate dehydrogenase 1; ALPS, analysis along the perivascular space; FA, fractional anisotropy; MD, mean diffusivity; MK, mean kurtosis; DTI, diffusion tensor imaging; DKI, diffusion kurtosis imaging; LrGG, lower-grade glioma; GBM, glioblastoma.
Acknowledgments
Funding: This study received funding from
Footnote
Conflicts of Interest: All authors have completed the ICMJE uniform disclosure form (available at https://qims.amegroups.com/article/view/10.21037/qims-23-541/coif). The authors report that this study received funding from the National Natural Science Foundation of China (Nos. U22A20354 and 81730049). The authors have no other conflicts of interest to declare.
Ethical Statement: The authors are accountable for all aspects of the work in ensuring that questions related to the accuracy or integrity of any part of the work are appropriately investigated and resolved. The study was conducted in accordance with the Declaration of Helsinki (as revised in 2013) and approved by the Institutional Review Board of Tongji Hospital of Tongji Medical College of Huazhong University of Science and Technology (No. TJ-IRB202303157). Informed consent was obtained from all individual participants.
Open Access Statement: This is an Open Access article distributed in accordance with the Creative Commons Attribution-NonCommercial-NoDerivs 4.0 International License (CC BY-NC-ND 4.0), which permits the non-commercial replication and distribution of the article with the strict proviso that no changes or edits are made and the original work is properly cited (including links to both the formal publication through the relevant DOI and the license). See: https://creativecommons.org/licenses/by-nc-nd/4.0/.
References
- Ricard D, Idbaih A, Ducray F, Lahutte M, Hoang-Xuan K, Delattre JY. Primary brain tumours in adults. Lancet 2012;379:1984-96. [Crossref] [PubMed]
- Louis DN, Perry A, Reifenberger G, von Deimling A, Figarella-Branger D, Cavenee WK, Ohgaki H, Wiestler OD, Kleihues P, Ellison DW. The 2016 World Health Organization Classification of Tumors of the Central Nervous System: a summary. Acta Neuropathol 2016;131:803-20. [Crossref] [PubMed]
- Hartmann C, Hentschel B, Wick W, Capper D, Felsberg J, Simon M, Westphal M, Schackert G, Meyermann R, Pietsch T, Reifenberger G, Weller M, Loeffler M, von Deimling A. Patients with IDH1 wild type anaplastic astrocytomas exhibit worse prognosis than IDH1-mutated glioblastomas, and IDH1 mutation status accounts for the unfavorable prognostic effect of higher age: implications for classification of gliomas. Acta Neuropathol 2010;120:707-18. [Crossref] [PubMed]
- Turkalp Z, Karamchandani J, Das S. IDH mutation in glioma: new insights and promises for the future. JAMA Neurol 2014;71:1319-25. [Crossref] [PubMed]
- Iliff JJ, Wang M, Liao Y, Plogg BA, Peng W, Gundersen GA, Benveniste H, Vates GE, Deane R, Goldman SA, Nagelhus EA, Nedergaard M. A paravascular pathway facilitates CSF flow through the brain parenchyma and the clearance of interstitial solutes, including amyloid β. Sci Transl Med 2012;4:147ra111. [Crossref] [PubMed]
- Ma Q, Schlegel F, Bachmann SB, Schneider H, Decker Y, Rudin M, Weller M, Proulx ST, Detmar M. Lymphatic outflow of cerebrospinal fluid is reduced in glioma. Sci Rep 2019;9:14815. [Crossref] [PubMed]
- Hu X, Deng Q, Ma L, Li Q, Chen Y, Liao Y, et al. Meningeal lymphatic vessels regulate brain tumor drainage and immunity. Cell Res 2020;30:229-43. [Crossref] [PubMed]
- Xu D, Zhou J, Mei H, Li H, Sun W, Xu H. Impediment of Cerebrospinal Fluid Drainage Through Glymphatic System in Glioma. Front Oncol 2021;11:790821. [Crossref] [PubMed]
- He W, You J, Wan Q, Xiao K, Chen K, Lu Y, Li L, Tang Y, Deng Y, Yao Z, Yue J, Cao G. The anatomy and metabolome of the lymphatic system in the brain in health and disease. Brain Pathol 2020;30:392-404. [Crossref] [PubMed]
- das Neves SP. Delivanoglou N, Da Mesquita S. CNS-Draining Meningeal Lymphatic Vasculature: Roles, Conundrums and Future Challenges. Front Pharmacol 2021;12:655052. [Crossref] [PubMed]
- Taoka T, Masutani Y, Kawai H, Nakane T, Matsuoka K, Yasuno F, Kishimoto T, Naganawa S. Evaluation of glymphatic system activity with the diffusion MR technique: diffusion tensor image analysis along the perivascular space (DTI-ALPS) in Alzheimer's disease cases. Jpn J Radiol 2017;35:172-8. [Crossref] [PubMed]
- Zhang W, Zhou Y, Wang J, Gong X, Chen Z, Zhang X, Cai J, Chen S, Fang L, Sun J, Lou M. Glymphatic clearance function in patients with cerebral small vessel disease. Neuroimage 2021;238:118257. [Crossref] [PubMed]
- Hsu JL, Wei YC, Toh CH, Hsiao IT, Lin KJ, Yen TC, Liao MF, Ro LS. Magnetic Resonance Images Implicate That Glymphatic Alterations Mediate Cognitive Dysfunction in Alzheimer Disease. Ann Neurol 2023;93:164-74. [Crossref] [PubMed]
- Cai X, Chen Z, He C, Zhang P, Nie K, Qiu Y, Wang L, Wang L, Jing P, Zhang Y. Diffusion along perivascular spaces provides evidence interlinking compromised glymphatic function with aging in Parkinson's disease. CNS Neurosci Ther 2023;29:111-21. [Crossref] [PubMed]
- Jiang R, Jiang J, Zhao L, Zhang J, Zhang S, Yao Y, Yang S, Shi J, Shen N, Su C, Zhang J, Zhu W. Diffusion kurtosis imaging can efficiently assess the glioma grade and cellular proliferation. Oncotarget 2015;6:42380-93. [Crossref] [PubMed]
- Bai Y, Lin Y, Tian J, Shi D, Cheng J, Haacke EM, Hong X, Ma B, Zhou J, Wang M. Grading of Gliomas by Using Monoexponential, Biexponential, and Stretched Exponential Diffusion-weighted MR Imaging and Diffusion Kurtosis MR Imaging. Radiology 2016;278:496-504. [Crossref] [PubMed]
- Xiong J, Tan WL, Pan JW, Wang Y, Yin B, Zhang J, Geng DY. Detecting isocitrate dehydrogenase gene mutations in oligodendroglial tumors using diffusion tensor imaging metrics and their correlations with proliferation and microvascular density. J Magn Reson Imaging 2016;43:45-54. [Crossref] [PubMed]
- Gates EDH, Lin JS, Weinberg JS, Hamilton J, Prabhu SS, Hazle JD, Fuller GN, Baladandayuthapani V, Fuentes D, Schellingerhout D. Guiding the first biopsy in glioma patients using estimated Ki-67 maps derived from MRI: conventional versus advanced imaging. Neuro Oncol 2019;21:527-36. [Crossref] [PubMed]
- Veraart J, Novikov DS, Christiaens D, Ades-Aron B, Sijbers J, Fieremans E. Denoising of diffusion MRI using random matrix theory. Neuroimage 2016;142:394-406. [Crossref] [PubMed]
- Qiu J, Deng K, Wang P, Chen C, Luo Y, Yuan S, Wen J. Application of diffusion kurtosis imaging to the study of edema in solid and peritumoral areas of glioma. Magn Reson Imaging 2022;86:10-6. [Crossref] [PubMed]
- Okudera T, Huang YP, Fukusumi A, Nakamura Y, Hatazawa J, Uemura K. Micro-angiographical studies of the medullary venous system of the cerebral hemisphere. Neuropathology 1999;19:93-111. [Crossref] [PubMed]
- Louis DN, Perry A, Wesseling P, Brat DJ, Cree IA, Figarella-Branger D, Hawkins C, Ng HK, Pfister SM, Reifenberger G, Soffietti R, von Deimling A, Ellison DW. The 2021 WHO Classification of Tumors of the Central Nervous System: a summary. Neuro Oncol 2021;23:1231-51. [Crossref] [PubMed]
- Van Cauter S, Veraart J, Sijbers J, Peeters RR, Himmelreich U, De Keyzer F, Van Gool SW, Van Calenbergh F, De Vleeschouwer S, Van Hecke W, Sunaert S. Gliomas: diffusion kurtosis MR imaging in grading. Radiology 2012;263:492-501. [Crossref] [PubMed]
- Qi XX, Shi DF, Ren SX, Zhang SY, Li L, Li QC, Guan LM. Histogram analysis of diffusion kurtosis imaging derived maps may distinguish between low and high grade gliomas before surgery. Eur Radiol 2018;28:1748-55. [Crossref] [PubMed]
- Zhang Y, Zhang R, Ye Y, Wang S, Jiaerken Y, Hong H, Li K, Zeng Q, Luo X, Xu X, Yu X, Wu X, Yu W, Zhang M, Huang P. The Influence of Demographics and Vascular Risk Factors on Glymphatic Function Measured by Diffusion Along Perivascular Space. Front Aging Neurosci 2021;13:693787. [Crossref] [PubMed]
- Pencina MJ, D'Agostino RB Sr, D'Agostino RB Jr, Vasan RS. Evaluating the added predictive ability of a new marker: from area under the ROC curve to reclassification and beyond. Stat Med 2008;27:157-72; discussion 207-12. [Crossref] [PubMed]
- Cao T, Jiang R, Zheng L, Zhang R, Chen X, Wang Z, Jiang P, Chen Y, Zhong T, Chen H, Wu P, Xue Y, Lin L. T1 and ADC histogram parameters may be an in vivo biomarker for predicting the grade, subtype, and proliferative activity of meningioma. Eur Radiol 2023;33:258-69. [Crossref] [PubMed]
- Horváth A, Perlaki G, Tóth A, Orsi G, Nagy S, Dóczi T, Horváth Z, Bogner P. Biexponential diffusion alterations in the normal-appearing white matter of glioma patients might indicate the presence of global vasogenic edema. J Magn Reson Imaging 2016;44:633-41. [Crossref] [PubMed]
- Mehrabian H, Lam WW, Myrehaug S, Sahgal A, Stanisz GJ. Glioblastoma (GBM) effects on quantitative MRI of contralateral normal appearing white matter. J Neurooncol 2018;139:97-106. [Crossref] [PubMed]
- Horváth A, Perlaki G, Tóth A, Orsi G, Nagy S, Dóczi T, Horváth Z, Bogner P. Increased diffusion in the normal appearing white matter of brain tumor patients: is this just tumor infiltration? J Neurooncol 2016;127:83-90. [Crossref] [PubMed]
- Ostrom QT, Bauchet L, Davis FG, Deltour I, Fisher JL, Langer CE, Pekmezci M, Schwartzbaum JA, Turner MC, Walsh KM, Wrensch MR, Barnholtz-Sloan JS. The epidemiology of glioma in adults: a "state of the science" review. Neuro Oncol 2014;16:896-913. [Crossref] [PubMed]
- Toh CH, Siow TY. Factors Associated With Dysfunction of Glymphatic System in Patients With Glioma. Front Oncol 2021;11:744318. [Crossref] [PubMed]
- Okita Y, Takano K, Tateishi S, Hayashi M, Sakai M, Kinoshita M, Kishima H, Nakanishi K. Neurite orientation dispersion and density imaging and diffusion tensor imaging to facilitate distinction between infiltrating tumors and edemas in glioblastoma. Magn Reson Imaging 2023;100:18-25. [Crossref] [PubMed]
- Tan Y, Zhang H, Zhao RF, Wang XC, Qin JB, Wu XF. Comparison of the values of MRI diffusion kurtosis imaging and diffusion tensor imaging in cerebral astrocytoma grading and their association with aquaporin-4. Neurol India 2016;64:265-72. [Crossref] [PubMed]
- Behnam M, Motamedzadeh A, Aalinezhad M, Dadgostar E, Rashidi Noshabad FZ, Pourfridoni M, Raei M, Mirzaei H, Aschner M, Tamtaji OR. The role of aquaporin 4 in brain tumors: implications for pathophysiology, diagnosis and therapy. Mol Biol Rep 2022;49:10609-15. [Crossref] [PubMed]
- Mou K, Chen M, Mao Q, Wang P, Ni R, Xia X, Liu Y. AQP-4 in peritumoral edematous tissue is correlated with the degree of glioma and with expression of VEGF and HIF-alpha. J Neurooncol 2010;100:375-83. [Crossref] [PubMed]
- Yang L, Wang X, Zhen S, Zhang S, Kang D, Lin Z. Aquaporin-4 upregulated expression in glioma tissue is a reaction to glioma-associated edema induced by vascular endothelial growth factor. Oncol Rep 2012;28:1633-8. [Crossref] [PubMed]
- Togao O, Hiwatashi A, Yamashita K, Kikuchi K, Keupp J, Yoshimoto K, Kuga D, Yoneyama M, Suzuki SO, Iwaki T, Takahashi M, Iihara K, Honda H. Grading diffuse gliomas without intense contrast enhancement by amide proton transfer MR imaging: comparisons with diffusion- and perfusion-weighted imaging. Eur Radiol 2017;27:578-88. [Crossref] [PubMed]
- Liu X, Tian W, Kolar B, Yeaney GA, Qiu X, Johnson MD, Ekholm S. MR diffusion tensor and perfusion-weighted imaging in preoperative grading of supratentorial nonenhancing gliomas. Neuro Oncol 2011;13:447-55. [Crossref] [PubMed]
- Flores-Alvarez E, Durand-Muñoz C, Cortes-Hernandez F, Muñoz-Hernandez O, Moreno-Jimenez S, Roldan-Valadez E. Clinical Significance of Fractional Anisotropy Measured in Peritumoral Edema as a Biomarker of Overall Survival in Glioblastoma: Evidence Using Correspondence Analysis. Neurol India 2019;67:1074-1081. [Crossref] [PubMed]
- Cha S. Update on brain tumor imaging: from anatomy to physiology. AJNR Am J Neuroradiol 2006;27:475-87.
- Hempel JM, Bisdas S, Schittenhelm J, Brendle C, Bender B, Wassmann H, Skardelly M, Tabatabai G, Vega SC, Ernemann U, Klose U. In vivo molecular profiling of human glioma using diffusion kurtosis imaging. J Neurooncol 2017;131:93-101. [Crossref] [PubMed]
- Zhao J, Wang YL, Li XB, Hu MS, Li ZH, Song YK, Wang JY, Tian YS, Liu DW, Yan X, Jiang L, Yang ZY, Chu JP. Comparative analysis of the diffusion kurtosis imaging and diffusion tensor imaging in grading gliomas, predicting tumour cell proliferation and IDH-1 gene mutation status. J Neurooncol 2019;141:195-203. [Crossref] [PubMed]
- Xu Z, Ke C, Liu J, Xu S, Han L, Yang Y, Qian L, Liu X, Zheng H, Lv X, Wu Y. Diagnostic performance between MR amide proton transfer (APT) and diffusion kurtosis imaging (DKI) in glioma grading and IDH mutation status prediction at 3 T. Eur J Radiol 2021;134:109466. [Crossref] [PubMed]
- Bralten LB, Kloosterhof NK, Balvers R, Sacchetti A, Lapre L, Lamfers M, Leenstra S, de Jonge H, Kros JM, Jansen EE, Struys EA, Jakobs C, Salomons GS, Diks SH, Peppelenbosch M, Kremer A, Hoogenraad CC, Smitt PA, French PJ. IDH1 R132H decreases proliferation of glioma cell lines in vitro and in vivo. Ann Neurol 2011;69:455-63. [Crossref] [PubMed]
- Sun C, Zhao Y, Shi J, Zhang J, Yuan Y, Gu Y, et al. Isocitrate dehydrogenase1 mutation reduces the pericyte coverage of microvessels in astrocytic tumours. J Neurooncol 2019;143:187-96. [Crossref] [PubMed]
- Xie Y, Li S, Shen N, Gan T, Zhang S, Liu WV, Zhu W. Assessment of Isocitrate Dehydrogenase 1 Genotype and Cell Proliferation in Gliomas Using Multiple Diffusion Magnetic Resonance Imaging. Front Neurosci 2021;15:783361. [Crossref] [PubMed]
- Saito Y, Kamagata K, Uchida W, Takabayashi K, Aoki S. Improved reproducibility of diffusion tensor image analysis along the perivascular space (DTI-ALPS) index calculated by manual and automated methods. Jpn J Radiol 2023;41:1033-4. [Crossref] [PubMed]
- Saito Y, Kamagata K, Andica C, Uchida W, Takabayashi K, Yoshida S, Nakaya M, Tanaka Y, Kamiyo S, Sato K, Nishizawa M, Akashi T, Shimoji K, Wada A, Aoki S. Reproducibility of automated calculation technique for diffusion tensor image analysis along the perivascular space. Jpn J Radiol 2023;41:947-54. [Crossref] [PubMed]
- Chen HL, Chen PC, Lu CH, Tsai NW, Yu CC, Chou KH, Lai YR, Taoka T, Lin WC. Associations among Cognitive Functions, Plasma DNA, and Diffusion Tensor Image along the Perivascular Space (DTI-ALPS) in Patients with Parkinson's Disease. Oxid Med Cell Longev 2021;2021:4034509. [Crossref] [PubMed]
- Yokota H, Vijayasarathi A, Cekic M, Hirata Y, Linetsky M, Ho M, Kim W, Salamon N. Diagnostic Performance of Glymphatic System Evaluation Using Diffusion Tensor Imaging in Idiopathic Normal Pressure Hydrocephalus and Mimickers. Curr Gerontol Geriatr Res 2019;2019:5675014. [Crossref] [PubMed]