Multisequence MRI-based radiomics analysis for early prediction of the risk of T790M resistance in new brain metastases
Introduction
The incidence of lung cancer is increasing year by year and is associated with the highest cancer-related mortality globally (1). Epidermal growth factor receptor (EGFR) is the major targeted driver gene in non-small cell lung cancer (NSCLC) and accounts for almost half of all lung cancer cases among Asians (2). Tyrosine kinase inhibitors (TKIs) have remarkably extended the progression-free survival (PFS) for NSCLC patients and become the first-line treatment regimen for patients harboring EGFR mutations (3). However, there are still several problems with targeted therapy. NSCLC patients who initially respond well to the first- or second-generation TKIs inevitably develop acquired resistance (4). The EGFR gatekeeper mutation T790M is the primary molecular mechanism and the third-generation TKI osimertinib can effectively overcome it (5). The brain is the most common site of metastasis and relapse for NSCLC (6). Rational selection of targeted drugs is crucial for patients with brain metastases (BMs). Evidence has revealed that T790M resistance may emerge almost 9–14 months after receiving EGFR TKIs for NSCLC which is likely to develop BM (4). However, there is still a large proportion of NSCLC patients who do not develop T790M resistance at the time of BM. Besides, the time for developing resistance differs between individuals. Hence, for patients who have BM after progression on TKIs but are not harboring T790M, timely prediction of the risk of developing resistance is essential to regulate the treatment regime, thus taking advantages of TKIs and minimizing the economic burden.
The main approach to determine secondary resistance mutation is re-biopsy of the primary lung cancer or metastatic lesions (7). However, due to its invasive nature, re-biopsy can cause damage or tumor cell spread (7,8). Furthermore, the T790M-mutated cells might exist in a part of the tumor that remains undetected by puncture biopsy for sampling a small tissue (2). In clinical practice, plasma circulating tumor DNA (ctDNA) is gradually being used to assess the T790M mutation, although its application is limited by low abundance in blood and high cost (9). Consequently, there has remained a lack of a non-invasive, repeatable, and comprehensive-assessed method that can effectively evaluate the risk of developing T790M resistance for NSCLC patients with disease progression.
Magnetic resonance imaging (MRI) with excellent soft tissue resolution has already been regarded as a noninvasive diagnostic modality of BM (10). Radiomics is an emerging field, extracting high-throughput features from medical images to reflect tumor information (11). Studies that have applied radiomics based on metastatic lesions to identify mutation status have reported the excellent performance of this method (12-15), demonstrating the strong relationship between radiomics features and genotype of tumors. Recently, several articles have shown that MRI-based radiomics could identify T790M resistance mutation (16,17). Meanwhile, to our knowledge, few studies have explored the risk of emerging T790M mutation in a relatively short time based on multisequence MRI radiomics when patients experience disease progression of BM.
The purpose of this study was to evaluate the risk of T790M resistance mutation in NSCLC patients who developed BM without T790M after EGFR TKIs treatment through conventional sequences of new BM, and to give warning to the high-risk population prone to drug resistance. We present this article in accordance with the TRIPOD reporting checklist (available at https://qims.amegroups.com/article/view/10.21037/qims-23-822/rc).
Methods
Patient selection
The study was conducted in accordance with the Declaration of Helsinki (as revised in 2013). The study was approved by the Ethics Committee of Beijing Chest Hospital, Capital Medical University (No. YJS-2022-27) and the requirement for individual consent for this retrospective analysis was waived.
We searched 1,356 NSCLC patients with BM from January 2014 to January 2023 in 2 hospitals. The inclusion criteria were as follows: (I) patients were confirmed with EGFR mutation by pathology or blood genetic test and regular detection of T790M. (II) First- or second-generation EGFR-TKIs had been used as the first-line treatment. (III) BM was diagnosed within 14 months of using TKIs. (IV) Patients underwent high-quality multisequence MRI scans before any treatment for BM. The exclusion criteria were as follows: (I) other treatment currently underway. (II) Poor or incomplete image quality. (III) Other tumors including multiple primary lung cancer.
Previous studies (4,18) have revealed that NSCLC patients may develop T790M resistance within up to 14 months after receiving TKIs. As a result, we divided these patients into T790M-positive and T790M-negative groups according to T790M occurrence within 14 months after starting EGFR-TKIs. Finally, 294 patients from June 2017 to January 2023 were enrolled in the training cohort of hospital 1, 111 patients from January 2014 to May 2017 were selected in the testing cohort of hospital 2. The detailed enrollment of the whole patients is shown in Figure 1.
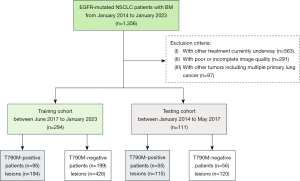
MRI acquisition and preprocessing
All included patients in this study underwent an enhanced brain MRI, using a 3.0 T (SIGAL Architect; GE Healthcare, Chicago, IL, USA) or 1.5 T (Signa HDXT; GE Healthcare) MR scanner equipped with a 48 or 32 head coil. The parameters of 4 MR sequences were used based on a 3.0 or 1.5 T scanner as follows: (I) T2-weighted imaging (T2WI), repetition time (TR) =4,000 or 4,240 ms, echo time (TE) =113 or 102 ms, and slice thickness =5 mm. (II) T2 fluid-attenuated inversion recovery (T2-FLAIR), TR =7,000 or 8,600 ms, TE =79 or 120 ms, inversion time (TI) =2,500 ms and slice thickness =5 mm. (III) Diffusion-weighted imaging (DWI) sequence with b values =1,000/0 s/mm2, TR =4,028 or 6,000 ms, TE =80 ms, and slice thickness =5 mm. (IV) Contrast-enhanced T1-weighted imaging (T1-CE), TR =250 or 380 ms, TE =2.46 ms, field of view (FOV) =240×240 mm and slice thickness =5 mm. The gadolinium-diethylenetriamine penta-acetic acid was used as the contrast media for the T1-CE. The dose was 0.1 mmol/kg. Then, to perform the registration of T2WI, T2-FLAIR, DWI, and T1-CE sequence, we used the elastix toolbox to proceed the four sequences into the equal geometric space. Besides, all images of 4 MR sequences were resampled to the same voxels of 1×1×1 mm3 to reduce the effect of slice thickness variation. These two steps of MRI preprocessing were based on the open-source 3D Slicer software (https://www.slicer.org).
BM segmentation and radiomics feature extraction
The regions of interest (ROIs) including the whole boundary of all BMs were manually drawn by a radiologist with 5 years’ working experience. In the process of segmentation, small tumors with a diameter smaller than 5 mm were excluded because they cannot provide two consecutive slices, which was the minimal requirement for the 3D approach. Then, all ROIs were reviewed by a senior radiologist with 10 years of experience in oncologic imaging. The two radiologists resolved any disagreements by consensus, and if a consensus could not be reached, a radiologist with more than 15 years of experience in oncological imaging would make the final decision. All radiologists were blinded to the gene mutation results of all patients. The process of segmentation was also performed by 3D slicer. To compare the four MR sequences fairly, we implemented a registration process. We registered T2-FLAIR, T2WI, and DWI to the corresponding T1-CE sequence. Therefore, the segmentation boundaries should have been rendered suitable for other sequences. At last, we separately segmented 194 and 426 lesions in T790M-positive and T790M-negative groups in the training cohort, respectively. Furthermore, 115 and 120 lesions were segmented respectively in the testing cohort between T790M-positive and T790M-negative groups, respectively.
The process of radiomics feature extraction was performed by the PyRadiomics package in python (version 3.7; https://pypi.org/project/pyradiomics/). The package default image normalization (z score normalization) was firstly performed to brain-extracted images to mitigate differences across different machines and image quality. The aim of normalization was to reduce variability of radiomics features. Then, the radiomics features of four MR sequences based on the original images, LoG-sigma-transformed (n=1.0, 2.0, 3.0, 4.0, and 5.0) images, and wavelet-transformed images were separately extracted. The categories of radiomics features included first-order, shape, grey level co-occurrence matrix (GLCM), grey level run length matrix (GLRLM), grey level size zone matrix (GLSZM), grey level dependence matrix (GLDM), and neighboring grey tone difference matrix (NGTDM). The detailed information of the radiomics features can be found in official documentation (https://pyradiomics.readthedocs.io/en/latest/features.html).
Radiomics feature selection and model construction
Firstly, we used the MinMaxScaler method to normalize the radiomics features in two groups. Then, the synthetic minority oversampling technique (SMOTE) was applied to balance the amounts of two groups in order to avoid bias toward due to the unbalance dataset of T790M-positive and T790M-negative. Secondly, we randomly selected 30 patients from the training cohort to evaluate the reliability of radiomics features based on the inter-class correlation coefficient (ICC). Radiomics features with ICCs more than 0.8 were selected. After that we used the maximal information coefficient (MIC) to select the top 300 radiomics features separately based on four MR sequences according to the MIC values. Thirdly, the Mann-Whitney U test was applied on the selected features. The features with P<0.05 were retained. Finally, to avoid multicollinearity and overfitting phenomena, least absolute shrinkage and selection operator (LASSO) algorithm was used to select the optimized subset of features and evaluate the corresponding coefficients with tenfold cross-validation. Then, the radiomics scores (rad-score) of each MR sequence were calculated for each lesion via a linear combination of selected radiomics features which were weighted by their corresponding coefficients and we also applied this formula in the independent testing cohort to compute the rad-score. Besides, according to the rad-score of each lesion, we calculated the mean rad-score of each patient based on four MR sequences in the training and testing cohort respectively. The detailed formula is listed in the Appendix 1.
We used random forest classifier (RFC) method to construct the prediction model due to its high variance-bias trade-off capability. The radiomics models via rad-scores of four MR sequences based on lesion- and patient-level were separately built to predict T790M positivity. All lesions or patients in the training cohort were randomly divided into training and validation datasets at a 7:3 ratio. At lesion-level, patients with multiple lesions were assigned either to the training or validation set to prevent bias. These two models (lesion- or patient-level) were trained and validated in the training cohort by tenfold cross-validation. At last, we used grid search cross validation (CV) method to optimize the model performance and tested in the independent testing cohort. All processes of radiomics feature selection and model construction were performed by the Python scikit-learn package (version 3.8, Scikit-learn Version 0. 21; http://scikit-learn.org/). The radiomics-based machine-learning workflow pipeline is shown in Figure 2.
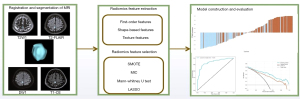
Statistical analysis
The statistical analysis was performed with SPSS software (version 26; IBM Corp., Armonk, NY, USA) and the Python scikit-learn package. Differences between qualitative variables which are presented as frequencies were compared with the Chi-square test. We used the Kolmogorov-Smirnov test to test normality for continuous variables. The independent t-test was used to analyze the normally distributed variables and they were shown as the mean ± standard deviation (SD). Differences between nonnormally distributed variables were analyzed with the Mann-Whitney U test and these variables were presented as the median and interquartile range (IQR). The performance of the prediction models (lesion- and patient-level) were evaluated with receiver operator characteristic (ROC) curve and calculated area under the curve (AUC) in both the training and testing cohort. The paired t-test was used to calculate 95% confidence intervals (CIs). The prediction models’ performances were also assessed by the accuracy, precision, recall, and F1 score in two cohorts, respectively. Decision curve analysis (DCA) was performed to determine the clinical usefulness of the radiomics models by calculating the net benefits at different threshold probabilities in the training and testing cohort. Comparisons were considered statistically significant at P<0.05 (2-tailed test).
Results
Clinical characteristics
There were no significant differences for sex, smoking history, alcohol consumption, and mutation subtype between two groups in two cohorts (all P>0.05). Details of the demographic data and clinical characteristics of the training and testing cohorts are summarized in Table 1.
Table 1
Characteristic | Training cohort (n=294) | Testing cohort (n=111) | |||||
---|---|---|---|---|---|---|---|
T790M-positive (n=95) | T790M-negative (n=199) | P value | T790M-positive (n=55) | T790M-negative (n=56) | P value | ||
Gender, n (%) | |||||||
Male | 31 (32.63) | 75 (37.69) | 0.398 | 23 (41.82) | 20 (35.71) | 0.509 | |
Female | 64 (67.37) | 124 (62.31) | 32 (58.18) | 36 (64.29) | |||
Age (years), mean ± SD | 57.54±9.24 | 56.03±10.30 | 0.226 | 57.14±8.91 | 56.31±10.21 | 0.649 | |
Alcohol consumption, n (%) | |||||||
Yes | 10 (10.53) | 38 (19.10) | 0.063 | 6 (10.91) | 9 (16.07) | 0.426 | |
No | 85 (89.47) | 161 (80.90) | 49 (89.09) | 47 (83.93) | |||
Smoking, n (%) | |||||||
Yes | 19 (20.00) | 55 (27.64) | 0.158 | 6 (10.91) | 12 (21.43) | 0.133 | |
No | 76 (80.00) | 144 (72.36) | 49 (89.09) | 44 (78.57) | |||
Subtype, n (%) | |||||||
19Del | 45 (47.37) | 95 (47.74) | 0.953 | 22 (40.00) | 24 (42.86) | 0.760 | |
21L858R | 50 (52.63) | 104 (52.26) | 33 (60.00) | 32 (57.14) |
Differences were assessed by independent t-test or Chi-square test. SD, standard deviation.
Radiomics feature selection and rad-score evaluation
For predicting T790M-positivity, we separately selected 25 radiomics features based on T2WI sequence, 14 radiomics features based on T2-FLAIR sequence, 20 radiomics features based on DWI sequence, and 20 radiomics features based on T1-CE sequence.
The rad-scores of DWI, T2-FLAIR, and T1-CE in T790M-positive groups were significantly higher than those in T790M-negative groups both in the training and testing cohort (P<0.05), regardless of whether in the lesion- or patient-level. As for the rad-score of T2WI, T790M-positive groups were significantly lower than T790M-negative groups in two cohorts and levels (P<0.05). The detailed values of rad-scores based on four MR sequences are shown in Table 2. The waterfall plots of total radiomics scores in testing cohorts (lesion- or patient-level) are displayed in Figures 3,4. The normalized importance of T2WI, DWI, T2-FLAIR, and T1-CE in the combined model was displayed in Figure S1.
Table 2
Sequence | Training cohort | Testing cohort | |||||||||||||
---|---|---|---|---|---|---|---|---|---|---|---|---|---|---|---|
Lesion level | Patient level | Lesion level | Patient level | ||||||||||||
Positive | Negative | P | Positive | Negative | P | Positive | Negative | P | Positive | Negative | P | ||||
DWI | 0.93 (0.49, 1.16) |
0.66 (0.37,1.00) | <0.001 | 0.84 (0.46, 1.15) |
0.75 (0.09, 0.91) |
0.004 | 1.11±0.21 | 0.94 (0.78, 1.10) |
<0.001 | 0.93 (0.44, 1.14) |
0.43±0.30 | <0.001 | |||
T2-FLAIR | 0.54±0.12 | 0.44±0.16 | <0.001 | 0.54 (0.48, 0.58) |
0.45±0.11 | <0.001 | 0.55±0.14 | 0.46 (0.37, 0.57) | <0.001 | 0.54±0.10 | 0.47±0.13 | 0.001 | |||
T1-CE | 0.53±0.13 | 0.44±0.17 | <0.001 | 0.50±0.13 | 0.45±0.16 | 0.035 | 0.58 (0.50, 0.64) |
0.50 (0.35, 0.61) |
<0.001 | 0.51±0.13 | 0.41±0.14 | <0.001 | |||
T2WI | −0.03 (−0.16, 0.20) |
0.13 (0.02, 0.22) |
<0.001 | 0.00 (−0.11, 0.21) |
0.11 (0.07, 0.18) |
0.002 | −0.07±0.14 | 0.12±0.15 | <0.001 | −0.06 (−0.12, 0.15) |
0.13±0.20 | 0.001 |
Differences were assessed by Mann-Whitney U test or independent t-test. Data follow normal distribution are presented as mean ± standard deviation and data follow skewed normal distribution are presented as median (interquartile range). DWI, diffusion-weighted imaging; T2-FLAIR, T2 fluid-attenuated inversion recovery; T1-CE, contrast-enhanced T1-weighted imaging; T2WI, T2-weighted imaging.
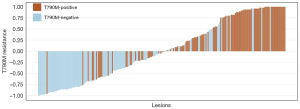
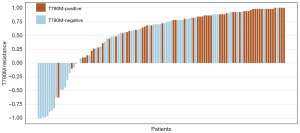
Model performance
The model based on lesion-level via rad-scores of the four MR sequences showed good performance for predicting T790M-positive with AUCs of 0.879 (95% CI: 0.834–0.917) and 0.834 (95% CI: 0.797–0.873) in the training and testing cohorts, respectively (Figure 5A,5B). The F1 score of the lesion-level model was 0.798 in the training cohort and 0.742 in the testing cohort. The model of patient-level also showed a favorable discriminatory ability in the training cohort with an AUC and F1 score of 0.851 (95% CI: 0.732–0.944) and 0.837, which was also confirmed in the testing cohort with an AUC and F1 score of 0.734 (95% CI: 0.657–0.807) and 0.716 (Figure 5C,5D), respectively. DCA (Figure 6) showed that these two models (lesion- or patient-level) would offer net benefits over the “treat-all” or “treat-none” scheme within a certain range of threshold in the combined training cohort. Similar results could be found in the testing cohort. The accuracy, precision, recall, and F1 score of these two models are summarized in Table 3.
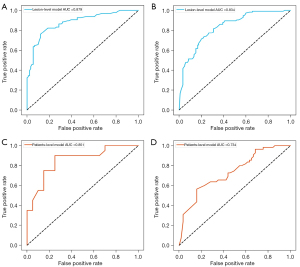
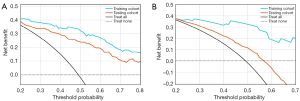
Table 3
Index | Lesion-level | Patient-level | |||
---|---|---|---|---|---|
Training cohort | Testing cohort | Training cohort | Testing cohort | ||
AUC | 0.879 | 0.834 | 0.851 | 0.734 | |
Accuracy | 0.807 | 0.746 | 0.825 | 0.625 | |
Precision | 0.866 | 0.746 | 0.783 | 0.570 | |
Recall | 0.740 | 0.739 | 0.900 | 0.964 | |
F1 score | 0.798 | 0.742 | 0.837 | 0.716 |
AUC, area under the ROC curve; ROC, receiver operating characteristic.
Discussion
In this study, we followed up 405 NSCLC patients from the progression of BM to the time point of receiving 14 months of the first- or second-generation EGFR TKIs. Then we developed and validated a fused model that included the routine MRI sequence (T1-CE, T2WI, DWI, T2-FLAIR) to assess the risk of emerging T790M resistance for NSCLC patients with disease progression of new BM. In the end, we revealed that multisequence fusion radiomics as a noninvasive quantitative method has the potential to identify patients at high risk of developing resistance.
Adding clinical factors to radiomics features may improve predictive performance. Unfortunately, in our research, the basic clinical features in terms of age, sex, smoking history, and alcohol consumption were not indicated to be statistically significantly associated with the time when T790M emerges. A previous report (19) showed that patients with EGFR L858R tended to have a lower incidence of developing T790M mutation compared with EGFR exon19 deletion. However, the EGFR mutation subtype in the current work has no significant difference to assess whether the resistance would occur in the early stage.
Studies have demonstrated that most NSCLC patients have a PFS of 9–14 months and will inevitably undergo disease progression owing to acquired resistance (4,18). T790M, as the main resistance mutation, is responsible for approximately a half of all resistance mechanism against the first- or second-generation EGFR-TKI (5,20). Currently, the standard treatment strategy for T790M-positive patients is osimertinib, which is relatively costly. BM is the most common site of metastasis in lung cancer and is indicative of progression (6), but in practice, not all patients who develop BM will develop T790M resistance. In the TREM 2 clinical trial (21), researchers found that the median overall survival for BM patients with T790M-negative status was substantially worse than that for those with T790M-positive status (7.5 vs. 21.8 months). In a prospective noninterventional study, Rybarczyk-Kasiuchnicz et al. (22) demonstrated that 90% of BM patients obtained control under the first-line treatment of the second-generation TKI afatinib. It may be reasonable and economic for T790M-negative BM patients to use the second-generation TKIs continuously and monitor the emergence of resistance regularly. Therefore, aiming to reduce the economic burden of patients, promptly adjust the medication and maximize the effect of EGFR-TKIs, we established a radiomics model using baseline progressive BM images after TKIs treatment to evaluate the risk of T790M resistance individually.
BM occurs under three different conditions. In the majority of patients [about 80% (23)], BM is discovered after the primary cancer, which means metachronous diagnosis. Far less commonly, BM is identified at the same time as or before the primary lung cancer, which is called synchronous diagnosis or precocious presentation, respectively. The genetic mutation is completely random and owing to the tumor heterogeneity; EGFR mutation status may not be detected in the early discovery of lung cancer. As a result, for the NSCLC patients in the first condition, they may take radiation, neoadjuvant chemotherapy, or even surgical excision rather than prioritizing targeted therapies. Instead of using primary lesions that have been subjected to various treatments, a radiomics signature based on treatment naïve new BM may be much more accurate.
Many articles have demonstrated the relationship between radiomics features extracted from BM and genetic status in primary tumor (12-14,24-26), whereas few studies have discussed the issue of T790M resistance. Several previous studies have applied brain radiomics to identify T790M mutation directly and achieved satisfactory performance (16,17). Fan et al. (16) built a multi-region combined radiomics signature to differentiate T790M-positive patients from T790M-negative patients using features selected from the tumor active area and peritumoral edema area. However, they only considered the metastatic lesions with edema and the lesions without peritumoral edema were ignored. Li et al. (17) revealed that multisequence MRI-based radiomics has potential to be a biomarker for identifying EGFR T790M and the DWI model yielded a high AUC of 0.886 in the training cohort. To evaluate whether T790M mutation exists or not presently, the study did not assess the risk of emerging resistance longitudinally and there was a lack of patient-level evaluation. The two studies mentioned above just discussed whether progressive BM patients were harboring T790M rather than assessing the risk of resistance for those T790M-negative patients in the early period. Our research is novel, it is the first study to discuss whether NSCLC patients who experience BM after progression on TKIs without T790M mutation will develop resistance in a short term. More importantly, to provide varieties of information for a more comprehensive assessment, radiomics features extracted from multiple sequences of baseline BM were fused. In addition, we performed an analysis at the lesion- and patient-levels respectively, which was not mentioned in similar studies. The results showed that although both levels performed well in the training cohort, the evaluation from the lesion-level performed stabler than that from the patient-level. This may be attributed to the reason that we simply took the average of multiple lesions while ignoring the influence of factors such as lesion size and solid composition.
The main finding of this study was that we proposed radiomics models (lesion- and patient-level) using RFC involving rad scores of T2WI, T2-FLAIR, DWI, and T1-CE sequences, respectively, and AUCs of 0.834 and 0.734, F1 scores of 0.742 and 0.716 based on lesion- and patient-level separately in the testing cohort were achieved, respectively. These two models both showed robust predictive power for predicting T790M mutation status and F1 score provided a more realistic assessment for radiomics models which revealed that the fused model still performs well when the datasets are imbalanced. The performance of predictive models might be substantially impacted by the selection of radiomics features; this is crucial to model performance and too many features could easily lead to model overfitting and reduce the performance. As a result, the process of feature selection was composed of SMOTE to balance the training dataset, MIC, and Mann-Whitney U test to characterize features and LASSO to select the most crucial radiomics features allowing for a credible result. Then, we calculated the rad-score of four MR sequences separately based on selected radiomics features which included 25 features of T2WI, 14 features of T2-FLAIR, 20 features of DWI, and 20 features of T1-CE. Besides, we used RFC to establish predictive models (lesion- or patient-level) according to the rad-score because RFC was a model of integrated learning that consists of a number of decision tree classification machines. Thus, it can improve the prediction accuracy without significantly increasing the computation. Above all, the two predictive models we established could offer net benefits over all the “treat-all” or “treat-none” strategies within certain threshold probability and show quality generalizability in routine clinical practice.
There were several limitations to this study. Firstly, it was a retrospective study, and the sample size was modest, a prospective trial with a larger sample size will be needed to verify our conclusion. Secondly, the performance of the patient-level model in our work was unsatisfactory in the testing cohort, other statistical variables, such as variance, SD, and quartile will be used to evaluate in the further research.
Conclusions
This study revealed that a radiomics signature integrating multisequence MRI rad-score had predictive value for T790M resistance, which enabled earlier selection of follow-up and different EGFR TKIs according to individual risk profile for those patients without T790M who developed BM after the progression of the first- or second-generation TKIs.
Acknowledgments
Funding: This research was supported by
Footnote
Reporting Checklist: The authors have completed the TRIPOD reporting checklist. Available at https://qims.amegroups.com/article/view/10.21037/qims-23-822/rc
Conflicts of Interest: All authors have completed the ICMJE uniform disclosure form (available at https://qims.amegroups.com/article/view/10.21037/qims-23-822/coif). The authors have no conflicts of interest to declare.
Ethical Statement: The authors are accountable for all aspects of the work in ensuring that questions related to the accuracy or integrity of any part of the work are appropriately investigated and resolved. The study was conducted in accordance with the Declaration of Helsinki (as revised in 2013). The study was approved by the Ethics Committee of Beijing Chest Hospital, Capital Medical University (No.
Open Access Statement: This is an Open Access article distributed in accordance with the Creative Commons Attribution-NonCommercial-NoDerivs 4.0 International License (CC BY-NC-ND 4.0), which permits the non-commercial replication and distribution of the article with the strict proviso that no changes or edits are made and the original work is properly cited (including links to both the formal publication through the relevant DOI and the license). See: https://creativecommons.org/licenses/by-nc-nd/4.0/.
References
- Wang Q, Zeng A, Zhu M, Song L. Dual inhibition of EGFR VEGF: An effective approach to the treatment of advanced non small cell lung cancer with EGFR mutation Int J Oncol 2023;62:26. (Review). [Crossref] [PubMed]
- Imyanitov EN, Iyevleva AG, Levchenko EV. Molecular testing and targeted therapy for non-small cell lung cancer: Current status and perspectives. Crit Rev Oncol Hematol 2021;157:103194. [Crossref] [PubMed]
- Fan Y, Zhao Z, Wang X, Ai H, Yang C, Luo Y, Jiang X. Radiomics for prediction of response to EGFR-TKI based on metastasis/brain parenchyma (M/BP)-interface. Radiol Med 2022;127:1342-54. [Crossref] [PubMed]
- Ho HL, Wang FY, Chiang CL, Tsai CM, Chiu CH, Chou TY. Dynamic Assessment of Tissue and Plasma EGFR-Activating and T790M Mutations with Droplet Digital PCR Assays for Monitoring Response and Resistance in Non-Small Cell Lung Cancers Treated with EGFR-TKIs. Int J Mol Sci 2022;23:11353. [Crossref] [PubMed]
- Yang JC, Ohe Y, Chiu CH, Ou X, Cantarini M, Jänne PA, Hartmaier RJ, Ahn MJ. Osimertinib plus Selumetinib in EGFR-Mutated Non-Small Cell Lung Cancer After Progression on EGFR-TKIs: A Phase Ib, Open-Label, Multicenter Trial (TATTON Part B). Clin Cancer Res 2022; Epub ahead of print. [Crossref] [PubMed]
- Matzenauer M, Vrana D, Melichar B. Treatment of brain metastases. Biomed Pap Med Fac Univ Palacky Olomouc Czech Repub 2016;160:484-90. [Crossref] [PubMed]
- Zhang X, Lu B, Yang X, Lan D, Lin S, Zhou Z, Li K, Deng D, Peng P, Zeng Z, Long L. Prognostic analysis and risk stratification of lung adenocarcinoma undergoing EGFR-TKI therapy with time-serial CT-based radiomics signature. Eur Radiol 2023;33:825-35. [Crossref] [PubMed]
- Fan Y, Dong Y, Wang H, Wang H, Sun X, Wang X, Zhao P, Luo Y, Jiang X. Development and externally validate MRI-based nomogram to assess EGFR and T790M mutations in patients with metastatic lung adenocarcinoma. Eur Radiol 2022;32:6739-51. [Crossref] [PubMed]
- Merker JD, Oxnard GR, Compton C, Diehn M, Hurley P, Lazar AJ, Lindeman N, Lockwood CM, Rai AJ, Schilsky RL, Tsimberidou AM, Vasalos P, Billman BL, Oliver TK, Bruinooge SS, Hayes DF, Turner NC. Circulating Tumor DNA Analysis in Patients With Cancer: American Society of Clinical Oncology and College of American Pathologists Joint Review. J Clin Oncol 2018;36:1631-41. [Crossref] [PubMed]
- Heynold E, Zimmermann M, Hore N, Buchfelder M, Doerfler A, Stadlbauer A, Kremenevski N. Physiological MRI Biomarkers in the Differentiation Between Glioblastomas and Solitary Brain Metastases. Mol Imaging Biol 2021;23:787-95. [Crossref] [PubMed]
- Binczyk F, Prazuch W, Bozek P, Polanska J. Radiomics and artificial intelligence in lung cancer screening. Transl Lung Cancer Res 2021;10:1186-99. [Crossref] [PubMed]
- Li Y, Lv X, Wang B, Xu Z, Wang Y, Gao S, Hou D. Differentiating EGFR from ALK mutation status using radiomics signature based on MR sequences of brain metastasis. Eur J Radiol 2022;155:110499. [Crossref] [PubMed]
- Wang G, Wang B, Wang Z, Li W, Xiu J, Liu Z, Han M. Radiomics signature of brain metastasis: prediction of EGFR mutation status. Eur Radiol 2021;31:4538-47. [Crossref] [PubMed]
- Jung WS, Park CH, Hong CK, Suh SH, Ahn SJ. Diffusion-Weighted Imaging of Brain Metastasis from Lung Cancer: Correlation of MRI Parameters with the Histologic Type and Gene Mutation Status. AJNR Am J Neuroradiol 2018;39:273-9. [Crossref] [PubMed]
- Jiang X, Ren M, Shuang X, Yang H, Shi D, Lai Q, Dong Y. Multiparametric MRI-Based Radiomics Approaches for Preoperative Prediction of EGFR Mutation Status in Spinal Bone Metastases in Patients with Lung Adenocarcinoma. J Magn Reson Imaging 2021;54:497-507. [Crossref] [PubMed]
- Fan Y, He L, Yang H, Wang Y, Su J, Hou S, Luo Y, Jiang X. Preoperative MRI-Based Radiomics of Brain Metastasis to Assess T790M Resistance Mutation After EGFR-TKI Treatment in NSCLC. J Magn Reson Imaging 2023;57:1778-87. [Crossref] [PubMed]
- Li Y, Lv X, Wang B, Xu Z, Wang Y, Sun M, Hou D. Predicting EGFR T790M Mutation in Brain Metastases Using Multisequence MRI-Based Radiomics Signature. Acad Radiol 2023;30:1887-95. [Crossref] [PubMed]
- Maemondo M, Inoue A, Kobayashi K, Sugawara S, Oizumi S, Isobe H, et al. Gefitinib or chemotherapy for non-small-cell lung cancer with mutated EGFR. N Engl J Med 2010;362:2380-8. [Crossref] [PubMed]
- Yang S, Mao S, Li X, Zhao C, Liu Q, Yu X, et al. Uncommon EGFR mutations associate with lower incidence of T790M mutation after EGFR-TKI treatment in patients with advanced NSCLC. Lung Cancer 2020;139:133-9. [Crossref] [PubMed]
- Yun CH, Mengwasser KE, Toms AV, Woo MS, Greulich H, Wong KK, Meyerson M, Eck MJ. The T790M mutation in EGFR kinase causes drug resistance by increasing the affinity for ATP. Proc Natl Acad Sci U S A 2008;105:2070-5. [Crossref] [PubMed]
- Eide IJZ, Helland Å, Ekman S, Mellemgaard A, Hansen KH, Cicenas S, Koivunen J, Grønberg BH, Brustugun OT. Osimertinib in T790M-positive and -negative patients with EGFR-mutated advanced non-small cell lung cancer (the TREM-study). Lung Cancer 2020;143:27-35. [Crossref] [PubMed]
- Rybarczyk-Kasiuchnicz A, Ramlau R, Stencel K. Treatment of Brain Metastases of Non-Small Cell Lung Carcinoma. Int J Mol Sci 2021;22:593. [Crossref] [PubMed]
- Sacks P, Rahman M. Epidemiology of Brain Metastases. Neurosurg Clin N Am 2020;31:481-8. [Crossref] [PubMed]
- Shofty B, Artzi M, Shtrozberg S, Fanizzi C, DiMeco F, Haim O, Peleg Hason S, Ram Z, Bashat DB, Grossman R. Virtual biopsy using MRI radiomics for prediction of BRAF status in melanoma brain metastasis. Sci Rep 2020;10:6623. [Crossref] [PubMed]
- Cao R, Pang Z, Wang X, Du Z, Chen H, Liu J, Yue Z, Wang H, Luo Y, Jiang X. Radiomics evaluates the EGFR mutation status from the brain metastasis: a multi-center study. Phys Med Biol 2022;67: [Crossref] [PubMed]
- Dong Y, Jiang Z, Li C, Dong S, Zhang S, Lv Y, Sun F, Liu S. Development and validation of novel radiomics-based nomograms for the prediction of EGFR mutations and Ki-67 proliferation index in non-small cell lung cancer. Quant Imaging Med Surg 2022;12:2658-71. [Crossref] [PubMed]