Robot-assisted navigation for percutaneous localization of peripheral pulmonary nodule: an in vivo swine study
Introduction
With the development and promotion of computed tomography (CT) technology, smaller lung nodules are being screened out. These nodules comprise pure ground glass opacity (GGO), part-solid, or solid nodules on the tomogram and may develop into lung cancers (1-3). Early diagnosis of the nodules could reduce lung cancer-related mortality (4,5). Video-assisted thoracoscopic surgery (VATS) provides a standard approach for biopsy diagnosis and subsequent radical resection (6). As one of the essential procedures of VATS, however, preoperative localization is challenging, especially when the target nodule is small, non-solid, or deep below the visceral pleura (7,8). In clinical practice, CT-guided percutaneous puncture is widely adopted for lung nodule localization. Nevertheless, needle placement accuracy is often hindered by the presence of undetected nodules, patient’s respiratory movement, and manual needle manipulation (9), which leads to unnecessary radiation exposure, complications, or failures (10).
Robot-assisted surgery (RAS) has been proposed and applied to assist in lung nodule biopsies (11,12) and lobectomy or mediastinum tumor resections (13,14). Previous studies on robotic-assisted interventions have reported a high needle insertion accuracy as compared to manual insertion (15-17). The robot is responsible for applying the surgical plans on medical images to the corresponding real positions on patients, and helping physicians to precisely execute the plans (17-19). As a result, the number of needle adjustments is decreased, thereby reducing postoperative complications and radiation dosage caused by repeated needle insertion attempts.
Despite studies on RAS, it has rarely been applied to percutaneous lung nodule localization. In a previous animal study, we proposed a CT-guided robot-assisted navigation system that enables nodule localization through the process of preoperative planning and intraoperative assistance in needle placement (20). The current in vivo swine study aimed to evaluate the accuracy and safety of the system in CT-guided percutaneous lung nodule localization.
Methods
Ethical statement
Animal experiments were granted approval by the Institutional Review Board [IRB No. EC-2020-093(QX)] at the First Affiliated Hospital of Guangzhou Medical University in compliance with national guidelines for the care and use of experimental animals.
Robot-assisted navigation system
The robot-assisted navigation system (TrueHealth Medical Technology Co., Ltd., Hengqin, China) was developed for CT-guided percutaneous puncture, including minimally invasive sampling and treatment. The system consists of 3 subsystems (Figure 1): (I) the surgical planning system; (II) the photoelectric navigation system; and (III) the robotic arm positioning and puncture system.
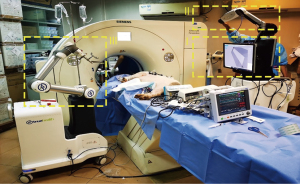
The photoelectric navigation system consists of an optical camera and positioning marker brackets. The marker’s position and orientation are tracked by the optical camera. The positioning marker bracket is pasted on the patient’s skin, fitting to the body curve, and used for registration of the patient space and the CT image space. In addition, the patient’s movement and breathing state can be tracked in real time. The surgical planning system contains surgical planning software. The patient’s preoperative CT images are reconstructed into a 3-dimensional (3D) model by the surgical planning system, which is automatically registered with the patient’s position information obtained from the photoelectric navigation system and provides a visible lung structure for surgeons to plan the needle path. The planned path is transformed into a real surgical space by registration. The robotic arm holds the needle guide stably and automatically moves to the preset position and orientation on the patient according to the planned path, which fixes the needle entry point and angle. The surgeon inserts the needle under the mechanical guidance of the needle guide.
Animal model
An in vivo swine study was conducted to assess the accuracy and feasibility of the robot-assisted navigation system in lung nodule localization. A total of 4 male Bama swine (age <1 year; mean weight, 51.35 kg) were studied. Considering that the results from manual nodule localization in swine lungs are not reflective of clinical performance, there was no control group. The experiments were conducted under the principles of replacement, reduction, and refinement (3R) and the study was in accord with the Animal Research: Reporting of In Vivo Experiments (ARRIVE) guidelines (21).
The swine was first injected subcutaneously with atropine sulfate (0.5 mg/kg) to inhibit the secretion of the respiratory glands. After 15 minutes, 250 mg of Telazol (4.4 mg/kg) was injected intravenously for anesthesia induction. Then, the swine underwent endotracheal intubation and was supported with a ventilator in the intermittent positive pressure ventilation (IPPV) mode. Anesthesia was maintained using propofol (1.0–4.0 µg/mL) and dexmedetomidine (0.3–1.0 µg/kg/h), with vecuronium bromide added every 45 minutes. Meloxicam (10 mg) was injected subcutaneously for intraoperative analgesia.
Different from previous studies with phantom-embedded metallic targets (22,23), lung nodules were simulated through implantation of the octyl-α-cyanoacrylate glue (Baiyun Medical Adhesive, Guangzhou, China). First, a CT scan was taken to confirm the swine lungs were intact and plan the approximate locations of the simulated nodules within 2 cm under the pleura. Then, an 18-G coaxial puncture needle (Bard Care, Murray Hill, NJ, USA) was inserted and 0.2 mL of octyl-α-cyanoacrylate glue was injected, which became a simulated lung nodule after 15 seconds. The number of simulated nodules implanted depended on the lung volume. At least 1 could be implanted in each lung lobe and no more than 5 or 6 in the ipsilateral lung. Finally, a verification scan was taken, and the exact locations of the simulated nodules were clear. Figure 2 shows a nodule implanted in the right superior lobe.
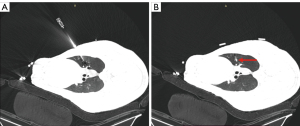
Robot-assisted nodule localization
The study procedures are illustrated in Figure 3 and Video 1. The nodule localization began with the swine’s lung CT scanning. A 64-slice spiral CT (Aquillion 64; Toshiba, Tokyo, Japan) was used and the scanning parameters were 120 kV, 60 mA, gantry rotation time of 0.6 s, and slice thickness of 1 mm. The swine was placed in the lateral position, attached with the flexible positioning marker brackets on the skin, and scanned at the end of inhalation controlled by the ventilator. The coordinates of the markers on CT images were automatically extracted and registered to those tracked by the optical camera. Thus, the spatial relationship between the CT image and the swine was obtained. The CT images were then imported into the surgical planning system to design the percutaneous needle path. 3D reconstruction and segmentation of the lung, skin, ribs, simulated nodules, and so on, were performed automatically (Figure 4A,4B). The surgeon clicked the mouse to select the target point and the entry point respectively via the software operation interface and the planned path was established accordingly (Figure 4C). In nodule localization surgery, the target point was selected to be near the nodule rather than the center of the nodule, and based on the reconstructed 3D model, the entry point was selected ensuring that the path avoids structures such as ribs and blood vessels and forming a shortest linear needle path. The insertion depth and thickness of the chest wall were automatically calculated according to the 3D reconstruction and segmentation result (Figure 4D).
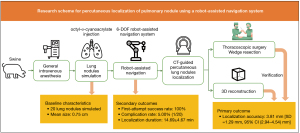
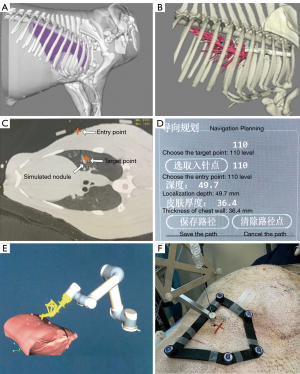
After confirmation of the planned needle path, the movement of the robot arm to the preset position was first simulated in a 3D virtual scene (Figure 4E). After a second confirmation of the correct moving path, the robot arm automatically moved to the final position on the swine. The needle entry point and puncture angle were determined with the needle guide held by the robot arm. Again, the swine’s breath was controlled at the end of inhalation by the ventilator, and the surgeon manually inserted the needle into the lung to the pre-calculated insertion depth (Figure 4F). Finally, a verification scan was taken and 0.3 mL indocyanine green (ICG) was injected for nodule localization. As all the simulated nodules were peripheral, the degree of ICG staining was expected to be acceptable. However, we advise against the use of ICG in nodules deeper than 2 cm from the visceral pleura. The verification CT images were imported into a standardized computer-assisted surgical (CAS) system (Hisense Medical Equipment Co., Ltd., Qingdao, China). The target point was selected again as the same point on the previous CT images, according to its relative position to the nodule and anatomical features. The 3D distance between the target point and the needle tip was measured. The measurement error of the standardized CAS system is within 2 mm.
Fluorescence-guided thoracoscopic surgery
A near-infrared thoracoscopy (Stryker, Kalamazoo, MI, USA) with a lens diameter of 10 mm was used for wedge resection after the nodule localization. The swine underwent the single-port or 2-port thoracoscopic surgery under general anesthesia. First, an ordinary video-assisted thoracoscopy was used to explore the entire thoracic cavity for adhesions and bleeding (Figure 5A). Next, the fluoroscope was adjusted to the indocyanine green fluorescence (ICGF) mode to detect the puncture site that was locally stained (Figure 5B). Then, wedge resection was performed (Figure 5C). A diameter of ≤20 mm and a consolidation-to-tumor ratio (CTR) of ≤0.25 were selected for wedge resection, consistent with the expert consensus on wedge resection of pulmonary nodules (24).
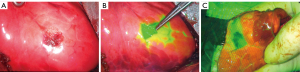
Study endpoints
- Localization accuracy: defined as the 3D distance between the target point and the needle tip on the verification image of nodule localization.
- Localization-related complication rate: including bleeding and pneumothorax during or after the localization.
- Localization duration: the duration between the verification scan of the simulated nodule implantation and the verification scan of nodule localization for a single nodule.
- First-attempt success rate: the first attempt is defined as success when the 3D distance between the target point and the needle tip is within 2 cm on the verification image of nodule localization.
Statistical analysis
The distribution of measurement data was tested with the Kolmogorov-Smirnov test. Continuous variables with normal distribution were given in mean ± standard deviation (SD) and 95% confidence interval (CI), and analyzed with an independent sample t-test. Categorical variables were analyzed with the χ2 test. All statistical analyses were 2-tailed with P<0.05 regarded as statistically significant, and conducted via SPSS software (version 19.0; IBM Corp., Armonk, NY, USA). Graphs were conducted via Graphpad Prism software (version 7.0; GraphPad Software, San Diego, CA, USA).
Results
Baseline characteristics
From 20 September 2020 to 30 September 2020, 4 male Bama swine were enrolled in this study in Guangzhou. In total, 20 lung nodules were simulated and accepted. All appeared as solid nodules under CT scanning. The mean size of the simulated nodules was 0.75 cm (SD: 0.15 cm). The most common location was the lower lobe (12/20, 60%). The mean distance from the nodule’s outer edge to the pleural surface was 1.26 cm (SD: 0.41 cm, 95% CI: 1.07–1.44 cm). The baseline characteristics of the lung nodules are summarized in Table 1.
Table 1
Swine No. | Lung nodule position | No. | Diameter (cm) | Distance from pleural surface (cm) |
---|---|---|---|---|
1 | Left side | 1 | 0.92 | 1.09 |
2 | 0.56 | 2.00 | ||
3 | 0.61 | 0.87 | ||
Right side | 1 | 0.83 | 1.12 | |
2 | 0.70 | 1.65 | ||
3 | 0.72 | 1.33 | ||
2 | Left side | 1 | 0.57 | 0.93 |
2 | 0.85 | 2.30 | ||
Right side | 1 | 0.59 | 1.00 | |
2 | 0.84 | 0.95 | ||
3 | Left side | 1 | 0.78 | 1.29 |
2 | 0.50 | 0.70 | ||
Right side | 1 | 0.54 | 1.81 | |
2 | 0.94 | 1.23 | ||
3 | 0.87 | 0.88 | ||
4 | Left side | 1 | 0.87 | 1.52 |
2 | 0.91 | 1.01 | ||
Right side | 1 | 0.92 | 0.92 | |
2 | 0.86 | 1.36 | ||
3 | 0.70 | 0.99 |
Mean diameter (cm): 0.75±0.15 (95% CI: 0.69–0.82). Mean distance from pleural surface (cm): 1.26±0.41 (95% CI: 1.07–1.44).
Study outcomes
The localization accuracy was 3.81 mm (SD: 1.29 mm, 95% CI: 2.94–4.54 mm) (Figure 6). A univariate analysis was conducted to investigate the factors influencing the deviation. For nodules in the upper/lower lung lobe, deviations were 3.47±0.99 and 4.03±1.46 mm respectively, without significant differences (P=0.31). For nodules at different distances from the pleural surface, deviations were close in the ≤10 mm (4.27±1.69 mm), 10–20 mm (3.57±1.13 mm), and >20 mm (4.10 mm, only one nodule in this group) groups, without significant differences (P=0.55) (Table 2).
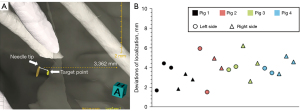
Table 2
Nodule characteristics | N | P value |
---|---|---|
Location | 0.31 | |
Upper lobe | 8 | |
Lower lobe | 12 | |
Distance from pleural surface | 0.55 | |
<10 mm | 6 | |
10–<20 mm | 13 | |
≥20 mm | 1 |
N, number of nodules in the group.
The first-attempt success rate was 100% (20/20). None of the nodules required adjustment of the needle entry point, puncture angle, or depth during the localization. One localization-related pneumothorax occurred, and the complication rate was 5% (1/20) (Table 3). The 3rd stimulated lung nodule of swine No. 4 was implanted in the right lower lung lobe. The verification scan of nodule localization revealed a moderate pneumothorax. The surgeon performed closed thoracic drainage with a pigtail until no pneumothorax was confirmed. The localization duration was 14.69±4.67 minutes in total. The mean duration was 21.00 minutes for swine No. 1, 14.74 minutes for swine No. 2, 11.40 minutes for swine No. 3, and 11.6 minutes for swine No. 4.
Table 3
Outcomes | Value |
---|---|
Complications | |
Pneumothorax | 1 |
Hemorrhage | 0 |
Mortality | 0 |
Localizing time (min) | 14.69±4.67 |
Margin distance (mm) | 27.96±4.54 |
Data are presented as number or mean ± standard deviation.
Wedge resection of lung nodules
The nodule’s specific location became visible immediately after the ICG was injected. The degree of ICG staining was acceptable and appeared as localized dispersion under the fluoroscope. All simulated lung nodules underwent successful wedge resection guided by the visible ICG stain and the preoperative nodule localization was considered entirely appropriate. The margin distance was measured as 27.96±4.54 mm, and the 95% CI was 25.83 to 30.09 mm. All resected specimens achieved parenchymal resection margins larger than 2 cm or larger than the nodule’s size.
Discussion
This in vivo animal study introduced a new method of lung nodule localization with an accuracy of 3.81 mm, a first-attempt success rate of 100%, and a complication rate of 5% (1/20), demonstrating promising outcomes of using the robot-assisted navigation system in CT-guided percutaneous peripheral lung nodule localization.
Percutaneous nodule localization is widely adopted in clinics, but needle placement accuracy is not guaranteed. Various technologies have been proposed to assist in nodule localization. Laser targeting (19) and augmented reality (25) enable visual guidance for the needle path and the surgical site, whereas the RAS system provides mechanical guidance or meticulous needle manipulation. To our knowledge, the RAS systems have been applied in percutaneous access to the kidney (PAKY) (26), tumor ablation (27-29), spine biopsy (30), and pain treatment (31), which adequately met the clinical need and achieved satisfactory surgical outcomes.
However, only a few RAS systems are used in the clinical studies of lung nodule localization. Maxio (Perfint Healthcare, Redmond, WA, USA) is one of the earliest Food and Drug Administration (FDA)-approved surgical robots and has been used in clinics for percutaneous biopsy and ablation. The robot arm provides mechanical guidance according to the surgical plan. However, during the entire procedure, patients need to keep still because their movements are not tracked or compensated. Zerobot (Okayama, Japan), a puncture robot guided by real-time CT fluoroscopy, has also been assessed in clinical trials. However, its clinical application is limited, since a large CT gantry diameter is needed because the robot arm operates inside the gantry. Moreover, the fluoroscopy module is not widely available in routine practice. A robot-assisted navigation system, Epione (Quantum Surgical, Montpellier, France), recently gained FDA approval. It has similar components and operation procedures to the robot-assisted navigation system utilized in this study, except that the marker bracket attached to the patient is rigid. A flexible bracket is considered to better fit the body contour and enable the optical camera to stably track the patient’s movement. More systems are still in the preliminary research stage through phantom studies (9,23). Compared to the existing surgical robots, the robot-assisted navigation system we proposed can be a better choice for lung nodule localization.
According to the results, the high accuracy of percutaneous lung nodule localization with the robot-assisted navigation system was not correlated with the nodule’s position or the distance from the pleural surface. We identified that 2 main factors potentially contributed to the localization accuracy. First, the robotic arm located the lung nodule according to the planned path. Second, the needle guide provided mechanical guidance so no needle manipulation deviation was introduced. In the previous studies, Hiraki et al. (17) reported an accuracy of 3.2 mm with the Zerobot and Ben-David et al. (9) reported 1.2–1.4 mm with the XACT robot (Xact Robotics, Ceasarea, Israel) both in animal experiments. The percutaneous puncture was performed under the guidance of CT fluoroscopy or repeated CT scans despite higher accuracy. Moreover, other interference factors that might affect localization accuracy in clinics, such as the patient’s movement and breathing, can be excluded with the system we used. The photoelectric navigation system can track the patient’s movement and breathing in real time. However, in this study, the swine was under general anesthesia to keep still and supported with ventilator so the breathing phase was reproducible. The promising accuracy demonstrates the reliability of the robot-assisted navigation system. Moreover, the system has the potential to reach the area where it is difficult for a human to perform a puncture. Since the complex and invisible structures are reconstructed into visible 3D models for planning the needle path and we can accurately execute the planned path, the system can still be accurate and safe enough even in some areas where manual puncture cannot reach or may be inaccurate and dangerous.
There was only 1 localization-related complication (1/20). A possible explanation might be that the needle insertion procedure caused a tearing action, leading to the pneumothorax. In related studies, Hiraki et al. (17) reported 1 minor pneumothorax and 1 minor parenchymal hemorrhage in 5 swine and Ben-David et al. (9) reported an 8% complication rate with 1 pneumothorax. In terms of complication rate, the robot-assisted navigation system is within acceptable safety limits. The first-attempt success rate was 100% and the localization duration was 14.69 minutes, which demonstrated efficient surgical procedures including 3D reconstruction, surgical planning, robot arm movement, and needle insertion. The relative clinical efficacy was demonstrated using VATS, which is also highlighted in our study.
The limitations of this study are as follows. First, all simulated lung nodules were implanted near the pleural surface restricted by the technical difficulty of nodule implantation. For nodules deep under the pleural surface, the feasibility and safety of the robot-assisted navigation system were not evaluated. Thus, further validation for deeper lesions is still warranted. Second, the photoelectric navigation system enables the operator to track the patient’s movement and breathing. However, in the animal experiment, the breathing was tracked and controlled using the ventilator. The capability of tracking and compensating patient’s respiratory motion requires further validation.
Conclusions
This pilot animal study demonstrated the promising potential of the robot-assisted navigation technique in peripheral lung nodule localization, with high accuracy and feasibility. Further clinical trials are needed to validate its safety compared to traditional manual localization.
Acknowledgments
Funding: This work was supported by
Footnote
Conflicts of Interest: All authors have completed the ICMJE uniform disclosure form (available at https://qims.amegroups.com/article/view/10.21037/qims-23-716/coif). XC, JS, HC, and MD are employees of TrueHealth Medical Technology Co. Ltd., Hengqin, Guangdong, China. The other authors have no conflicts of interest to declare.
Ethical Statement: The authors are accountable for all aspects of the work in ensuring that questions related to the accuracy or integrity of any part of the work are appropriately investigated and resolved. The study was approved by the institutional Ethics Board [No. EC-2020-093(QX)] of the First Affiliated Hospital of Guangzhou Medical University, in compliance with national guidelines for the care and use of animals.
Open Access Statement: This is an Open Access article distributed in accordance with the Creative Commons Attribution-NonCommercial-NoDerivs 4.0 International License (CC BY-NC-ND 4.0), which permits the non-commercial replication and distribution of the article with the strict proviso that no changes or edits are made and the original work is properly cited (including links to both the formal publication through the relevant DOI and the license). See: https://creativecommons.org/licenses/by-nc-nd/4.0/.
References
- Heuvelmans MA, Walter JE, Peters RB, Bock GH, Yousaf-Khan U, Aalst CMV, Groen HJM, Nackaerts K, Ooijen PMV, Koning HJ, Oudkerk M, Vliegenthart R. Relationship between nodule count and lung cancer probability in baseline CT lung cancer screening: The NELSON study. Lung Cancer 2017;113:45-50. [Crossref] [PubMed]
- Pedersen JH, Ashraf H, Dirksen A, Bach K, Hansen H, Toennesen P, Thorsen H, Brodersen J, Skov BG, Døssing M, Mortensen J, Richter K, Clementsen P, Seersholm N. The Danish randomized lung cancer CT screening trial--overall design and results of the prevalence round. J Thorac Oncol 2009;4:608-14. [Crossref] [PubMed]
- Naidich DP, Bankier AA, MacMahon H, Schaefer-Prokop CM, Pistolesi M, Goo JM, Macchiarini P, Crapo JD, Herold CJ, Austin JH, Travis WD. Recommendations for the management of subsolid pulmonary nodules detected at CT: a statement from the Fleischner Society. Radiology 2013;266:304-17. [Crossref] [PubMed]
- Torre LA, Bray F, Siegel RL, Ferlay J, Lortet-Tieulent J, Jemal A. Global cancer statistics, 2012. CA Cancer J Clin 2015;65:87-108. [Crossref] [PubMed]
- Aberle DR, Adams AM, Berg CD, Black WC, Clapp JD, Fagerstrom RM, Gareen IF, Gatsonis C, Marcus PM, Sicks JD. Reduced lung-cancer mortality with low-dose computed tomographic screening. N Engl J Med 2011;365:395-409. [Crossref] [PubMed]
- Hiebinger A, Weik T, Mertins H, Bodner J. Video-assisted thoracoscopic surgery (VATS) lower lobe bisegmentectomy (S7/8) for a central pulmonary metastasis. J Thorac Dis 2017;9:3296-8. [Crossref] [PubMed]
- Suzuki K, Nagai K, Yoshida J, Ohmatsu H, Takahashi K, Nishimura M, Nishiwaki Y. Video-assisted thoracoscopic surgery for small indeterminate pulmonary nodules: indications for preoperative marking. Chest 1999;115:563-8. [Crossref] [PubMed]
- Tamura M, Oda M, Fujimori H, Shimizu Y, Matsumoto I, Watanabe G. New indication for preoperative marking of small peripheral pulmonary nodules in thoracoscopic surgery. Interact Cardiovasc Thorac Surg 2010;11:590-3. [Crossref] [PubMed]
- Ben-David E, Shochat M, Roth I, Nissenbaum I, Sosna J, Goldberg SN. Evaluation of a CT-Guided Robotic System for Precise Percutaneous Needle Insertion. J Vasc Interv Radiol 2018;29:1440-6. [Crossref] [PubMed]
- Mokry A, Willmitzer F, Hostettler R, Richter H, Kircher P, Kneissl S, Wetzel S. Evaluation of a novel, patient-mounted system for CT-guided needle navigation-an ex vivo study. Neuroradiology 2019;61:55-61. [Crossref] [PubMed]
- Cleary K, Melzer A, Watson V, Kronreif G, Stoianovici D. Interventional robotic systems: applications and technology state-of-the-art. Minim Invasive Ther Allied Technol 2006;15:101-13. [Crossref] [PubMed]
- Kettenbach J, Kronreif G. Robotic systems for percutaneous needle-guided interventions. Minim Invasive Ther Allied Technol 2015;24:45-53. [Crossref] [PubMed]
- Soliman BG, Nguyen DT, Chan EY, Chihara RK, Meisenbach LM, Graviss EA, Kim MP. Impact of da Vinci Xi robot in pulmonary resection. J Thorac Dis 2020;12:3561-72. [Crossref] [PubMed]
- Chen K, Zhang X, Jin R, Xiang J, Han D, Zhang Y, Li H. Robot-assisted thoracoscopic surgery for mediastinal masses: a single-institution experience. J Thorac Dis 2020;12:105-13. [Crossref] [PubMed]
- Cornelis F, Takaki H, Laskhmanan M, Durack JC, Erinjeri JP, Getrajdman GI, Maybody M, Sofocleous CT, Solomon SB, Srimathveeravalli G. Comparison of CT Fluoroscopy-Guided Manual and CT-Guided Robotic Positioning System for In Vivo Needle Placements in Swine Liver. Cardiovasc Intervent Radiol 2015;38:1252-60. [Crossref] [PubMed]
- Heerink WJ, Ruiter SJS, Pennings JP, Lansdorp B, Vliegenthart R, Oudkerk M, de Jong KP. Robotic versus Freehand Needle Positioning in CT-guided Ablation of Liver Tumors: A Randomized Controlled Trial. Radiology 2019;290:826-32. [Crossref] [PubMed]
- Hiraki T, Kamegawa T, Matsuno T, Sakurai J, Kirita Y, Matsuura R, Yamaguchi T, Sasaki T, Mitsuhashi T, Komaki T, Masaoka Y, Matsui Y, Fujiwara H, Iguchi T, Gobara H, Kanazawa S. Robotically Driven CT-guided Needle Insertion: Preliminary Results in Phantom and Animal Experiments. Radiology 2017;285:454-61. [Crossref] [PubMed]
- Solomon SB, Patriciu A, Bohlman ME, Kavoussi LR, Stoianovici D. Robotically driven interventions: a method of using CT fluoroscopy without radiation exposure to the physician. Radiology 2002;225:277-82. [Crossref] [PubMed]
- Hsieh CP, Hsieh MJ, Fang HY, Chao YK. Imaging-guided thoracoscopic resection of a ground-glass opacity lesion in a hybrid operating room equipped with a robotic C-arm CT system. J Thorac Dis 2017;9:E416-9. [Crossref] [PubMed]
- Zhou G, Chen X, Niu B, Yan Y, Shao F, Fan Y, Wang Y. Intraoperative localization of small pulmonary nodules to assist surgical resection: A novel approach using a surgical navigation puncture robot system. Thorac Cancer 2020;11:72-81. [Crossref] [PubMed]
- Kilkenny C, Browne WJ, Cuthi I, Emerson M, Altman DG. Improving bioscience research reporting: the ARRIVE guidelines for reporting animal research. Vet Clin Pathol 2012;41:27-31. [Crossref] [PubMed]
- Pfeil A, Cazzato RL, Barbé L, De Marini P, Chiang JB, Garnon J, Renaud P, Gangi A. Robotically Assisted CBCT-Guided Needle Insertions: Preliminary Results in a Phantom Model. Cardiovasc Intervent Radiol 2019;42:283-8. [Crossref] [PubMed]
- Zhang W, Xia P, Liu S, Huang X, Zhao X, Liu Z, Dang H, Li X, Niu G. A coordinate positioning puncture method under robot-assisted CT-guidance: phantom and animal experiments. Minim Invasive Ther Allied Technol 2022;31:206-15. [Crossref] [PubMed]
- Hu J, Chen J, Chen C, Zhong W, Geng QEditorial Committee of Consensus on Wedge Resection of Lung Nodules. (≤2 cm) (2023 Edition). Wedge Resection of Pulmonary Nodules (≤2 cm): A Consensus Statement by Specialists of Thoracic Surgery (2023 Edition). Zhongguo Fei Ai Za Zhi 2023;26:338-47. [Crossref] [PubMed]
- Hecht R, Li M, de Ruiter QMB, Pritchard WF, Li X, Krishnasamy V, Saad W, Karanian JW, Wood BJ. Smartphone Augmented Reality CT-Based Platform for Needle Insertion Guidance: A Phantom Study. Cardiovasc Intervent Radiol 2020;43:756-64. [Crossref] [PubMed]
- Lima E, Rodrigues PL, Mota P, Carvalho N, Dias E, Correia-Pinto J, Autorino R, Vilaça JL. Ureteroscopy-assisted Percutaneous Kidney Access Made Easy: First Clinical Experience with a Novel Navigation System Using Electromagnetic Guidance (IDEAL Stage 1). Eur Urol 2017;72:610-6. [Crossref] [PubMed]
- Liu P, Qin J, Duan B, Wang Q, Tan X, Zhao B, Jonnathan PL, Chui CK, Heng PA. Overlapping radiofrequency ablation planning and robot-assisted needle insertion for large liver tumors. Int J Med Robot 2019;15:e1952. [Crossref] [PubMed]
- Capitanio U, Montorsi F. Renal cancer. Lancet 2016;387:894-906. [Crossref] [PubMed]
- Franco E, Ristic M, Rea M, Gedroyc WM. Robot-assistant for MRI-guided liver ablation: A pilot study. Med Phys 2016;43:5347. [Crossref] [PubMed]
- Nourbakhsh A, Grady JJ, Garges KJ. Percutaneous spine biopsy: a meta-analysis. J Bone Joint Surg Am 2008;90:1722-5. [Crossref] [PubMed]
- Jarebi M, Awaf A, Lefranc M, Peltier J. A matched comparison of outcomes between percutaneous endoscopic lumbar discectomy and open lumbar microdiscectomy for the treatment of lumbar disc herniation: a 2-year retrospective cohort study. Spine J 2021;21:114-21. [Crossref] [PubMed]