Correlation between 18F-FDG PET-derived parameters and quantitative pathological characteristics of soft tissue sarcoma
Introduction
Soft tissue sarcoma (STS) is a malignant tumor of mesenchymal origin that exists in almost all parts of the body. Its biological characteristics include aggressiveness, high recurrence, and metastatic potential (1,2). In recent years, despite great progress in multidisciplinary treatment, the survival outcomes of patients with STS have not significantly improved, thus severely endangering human health (2-4). Hence, a dire need exists to explore new methods to improve STS management strategies.
Some researchers have found that malignant tumors are mixed complexes composed of tumor cells and their tumor microenvironment (5-8), which promote the occurrence and development of tumors under their combined action. STS, a group of highly heterogeneous malignant tumors, shows diverse morphologic manifestations in their tumor tissues. This diversity has been found to be an important cause of heterogeneity in STS biological behavior, thus complicating the selection of their clinical management strategies. In addition, in STS, as in most solid tumors, the tumor stroma is composed primarily of high-density tumor-associated macrophage (TAM) and tumor infiltrating lymphocyte (TIL). Macrophages, the main components of the innate immune system, account for approximately 30–50% of the tumor tissue (6). M2 TAM have been shown to have biological effects that promote STS cell growth and metastasis, stimulate angiogenesis, modulate immunosuppression, and mediate antitumor activity of chemoradiation (7,8). Cytotoxic T lymphocytes CD8 directly kill tumor cells with tumor-associated antigens, and CD4 is a donor receptor that enables helper T cells to recognize antigens and participates in signal transduction. Both these functions are essential for adaptive immune responses in tumors, and are tightly associated with STS prognosis and the effectiveness of anti-immunotherapy (7,8).
The classical molecular imaging technique of 18F-fluorodeoxyglucose (18F-FDG) positron emission tomography/computed tomography (PET/CT) has been widely used in the evaluation of STS, and can also serve as a supplement to traditional imaging methods (9). Previous studies have demonstrated the importance of metabolic parameters of FDG PET in assessing the therapeutic response, prognosis, and histological grading of patients with STS (10-12). However, in some studies, standardized uptake value (SUV) in patients with STS with the French Federation of Cancer Centres (FNCLCC) II and III was found to have approximate median (12). In addition, FDG uptake can greatly vary among individuals, even those with the same histologic grading level. The intrinsic reasons underlying this association must be further explored.
Consequently, the present study retrospectively collected a group of patients who underwent 18F-FDG PET/CT and had pathologically confirmed STS. Continuous sections of tumor tissues were stained with HE and IHC, digitally processed, and to perform quantitative analysis of both tumor nucleated cell characteristics and tumor immune cell infiltration. The correlations between FDG metabolic parameters and these quantitative pathological characteristics were further investigated. Our aim was to provide new ideas for exploring STS management strategies based on PET/CT. We present this article in accordance with the STROBE reporting checklist (available at https://qims.amegroups.com/article/view/10.21037/qims-23-412/rc).
Methods
Patient selection and data management
The present study was performed in accordance with the Declaration of Helsinki (as revised in 2013) and was approved by the Institutional Ethics Committee of The First Hospital of Dalian Medical University (No. PJ-KS-KY-2021-249). The requirement for individual consent for this retrospective analysis was waived. We retrospectively screened 39 patients with STS who underwent 18F-FDG PET/CT examination before treatment, from December 2012 to October 2021. The specific enrollment process is shown in Figure 1. The cohort consisted of 19 men and 20 women with a median age of 59.0 (range, 32.0–84.0) years.
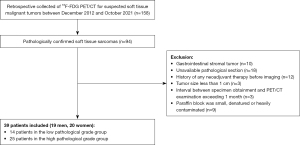
18F-FDG PET/CT imaging protocol and image analysis
All patients were required to fast for more than 6 hours. Their blood glucose was less than 10.0 mmol/L, and 18F-FDG was injected intravenously at 5.55 MBq/kg for approximately 60 min before machine collection. The same PET scanner (Biograph True-Point Row 64, Siemens, Erlangen, Germany) was used for all patients. CT and PET images were collected successively, with a matrix of 168×168, tube voltage of 120 kV, tube current for care-dose automatic adjustment, range of 60–80 mAs, and pitch of 0.8. The PET scans used 3-dimensional acquisition mode, 1.5 min/bed, with an axial field of view of 500 mm and a layer thickness of 5 mm. The OSEM and PSF reconstruction method was used with three iterations, 21 subsets, and a Gaussian filter half height width of 4 mm. CT was used for attenuation correction of the PET data set.
The image data were processed on a standard MMWP workstation in TureD software (Siemens Healthcare). First, the circular ROI was manually selected on the maximum cross-section of the tumor; a spherical volume of interest (VOI) was automatically generated, and finally, manual fine-tuning was performed to ensure that the VOI sufficiently covered the tumor target in three planes (coronal, sagittal, and transverse). The maximum standardized uptake value (SUVmax) and mean maximal standardized uptake value (SUVmean) were automatically obtained. Metabolic tumor volume (MTV) was defined as the total volume of voxels within the VOI, which was higher than SUV =2.5 (13). Total lesion glycolysis (TLG) was obtained by multiplication of MTV and SUVmean. Additionally, intratumoral FDG uptake heterogeneity (IFH) was calculated on the basis of the derivative of the metabolic volume/threshold (dV/dT); according to previous studies, the volume-based threshold was adjusted to 30–70% (14). Two experienced radiologists independently performed double-blinded readings and measurements. Consistency analysis was performed on the determined measurements, and the average value was finally used in statistical analysis.
Histopathologic staining and analysis
Two pathologists who were blinded to the 18F-FDG PET/CT results re-interpreted the histological subtypes of the enrolled patients according to the 2020 WHO guidelines for the classification of soft tissue tumors. The pathological subtypes included liposarcoma (n=4), fibrosarcoma (n=6), synovia sarcoma (n=6), undifferentiated sarcoma (n=8), leiomyosarcoma (n=7), angiosarcoma (n=5), rhabdosarcoma (n=1), and extrasolar-skeletal muscle sarcoma (n=2). STS were histopathologically classified as grade I, II, and III according to the FNCLCC grading system (15): FNCLCC I (n=6), FNCLCC II (n=8), FNCLCC III (n=25). According to previous study (16), the enrolled population with grade I and II STS was further defined as the low-grade group (n=14), and the population with grade III STS was defined as the high-grade group (n=25), as summarized in Table 1.
Table 1
Histopathological type | Total | FNCLCC | ||
---|---|---|---|---|
I | II | III | ||
Liposarcoma | 4 | 1 | 0 | 3 |
Fibrosarcoma | 6 | 1 | 2 | 3 |
Synovia sarcoma | 6 | 0 | 2 | 4 |
Undifferentiated sarcoma | 8 | 0 | 0 | 8 |
Leiomyosarcoma | 7 | 3 | 0 | 4 |
Angiosarcoma | 5 | 1 | 2 | 2 |
Rhabdosarcoma | 1 | 0 | 0 | 1 |
Extrasolar-skeletal muscle sarcoma | 2 | 0 | 2 | 0 |
Total | 39 | 6 | 8 | 25 |
FNCLCC, French Federation of Cancer Centers.
Tumor sample staining
Two pathologists selected the tissue paraffin blocks corresponding to the sections with the most tumor histopathologic characteristics and performed continuous sampling to prepare multiple 4-µm-thick tissue sections. Tumor cell characteristics were obtained from hematoxylin-eosin-stained tumor sections through routine histological assessment. Immunohistochemistry (IHC) was performed with the indirect streptavidin-biotin method. Briefly, the operation steps were as follows. Tissue white slices were baked, degreased, and rehydrated, then placed in citric acid repair solution for antigen repair. Different primary antibody dilutions were added. After incubation for 60 min in a 37 ℃ incubator, secondary antibodies were added and incubated in parallel at room temperature. This was followed by re-staining with DAB immunostaining agent, hematoxylin staining, dehydration with a graded alcohol series, and neutral resin sealing. With respect to a spleen tissue positive control during the same period, brownish-yellow staining of cytoplasm and/or membrane of tumor cells in tumor tissue sections was defined as positive staining.
Tumor sample analysis
Observations and digital image capture were performed through investigational light microscopy (Leica Laboratories, Wetzlar, Germany) with the assistance of two experienced pathologists. The most densely packed area of tumor cells, according to hematoxylin and eosin (H&E) staining, and the most representative areas of IHC cell staining were selected under low magnification (×40). Subsequently, digital images were acquired under high magnification (×400), in five randomly selected non-overlapping areas. Quantitative analysis was performed in Fiji ImageJ software 1.48v as previously described (17,18). Analytical values were obtained for tumor cell characteristics, including cell counts, total nuclear area, and mean nuclear area (Figure 2). The total protein expression of each immune cell marker stain was quantified as the integrated optical density (IOD), and the average values for five images were used in the analysis (Figure 3).
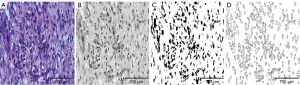
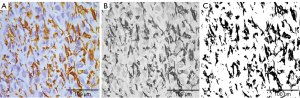
Statistical analysis
IBM SPSS 26.0 software was used. The Kolmogorov-Smirnov method was used to test the normality of continuous variables. Statistical descriptions of continuous variables with a non-normal distribution are described by median (Q1–Q3). The correlation test of two factors was performed with Spearman’s correlation analysis by using the correlation coefficient. Two-group comparisons of continuous variables were assessed with Mann-Whitney U tests. Multiple linear regression analysis was used to evaluate factors independently associated with metabolic parameters. The intra-group correlation coefficient test was used for consistency analysis. A probability (P) <0.05 was considered statistically significant.
Results
18F-FDG PET-derived parameters in patients with STS
The metabolic parameters of PET/CT in patients with STS were summarized in Table 2. Each metabolic parameter in the high-grade group was higher than that in the low-grade group. Moreover, heterogeneity in metabolic parameters was observed among patients with the same pathological type.
Table 2
Metabolic parameters | Total (n=39) | FNCLCC grouping | |
---|---|---|---|
Low-grade group (n=14) | High-grade group (n=25) | ||
SUVmax | 12.80 (7.69–18.60) | 8.60 (4.90–11.35) | 14.20 (9.83–20.15) |
MTV, cm3 | 57.87 (11.2–138.38) | 9.75 (3.12–76.29) | 97.79 (32.63–201.26) |
TLG | 219.43 (42.01–900.14) | 37.46 (10.05–335.92) | 433.72 (166.92–1,145.13) |
IFH | 0.92 (0.35–2.03) | 0.39 (0.19–0.92) | 1.24 (0.63–3.29) |
Statistical description by median (Q1–Q3). FDG, fluorodeoxyglucose; STS, soft tissue sarcoma; FNCLCC, French Federation of Cancer Centers; SUVmax, maximum standardized uptake value; MTV, metabolic tumor volume; TLG, total lesion glycolysis; IFH, intertumoral FDG uptake heterogeneity.
Quantitative pathological characteristics of patients with STS
The quantitative pathological characteristics of patients with STS were summarized. The quantitative values of tumor nucleated cell characteristics analyzed in patients, as determined by HE staining, included cell count, total nuclear area, and mean nuclear area, with median of 470 (Q1–Q3: 316–569), 13,731.61 (Q1–Q3: 10,463.36–17,710.37), and 30.51 (Q1–Q3: 25.21–36.64), respectively. Heterogeneity in cell counts, total nuclear area, and mean nuclear area was observed among individuals.
Immunohistochemical staining for CD163, CD68, CD8, and CD4 appeared as brown or brownish-yellow cytoplasm and/or membranes of tumor cells, respectively. The IODs of these immune markers were 43,493.03 (Q1–Q3: 11,570.00–18,069.51), 12,106.21 (Q1–Q3: 2,804.34–34,703.97), 6,384.48 (Q1–Q3: 3,180.59–16,727.14), and 8,850.24 (Q1–Q3: 4,689.25–24,689.3), respectively (Table 3). We also observed heterogeneous expression of these tumor-infiltrating immune cells (TIICs) (Figure 4). Among them, TAM (CD163 and CD68) had more intense staining than TIL (CD8 and CD4), and CD163 had the highest intensity.
Table 3
Pathological characteristics | Total (n=39) | FNCLCC Grouping | |
---|---|---|---|
Low-grade group (n=14) | High-grade group (n=25) | ||
Cell count | 470 (316–569) | 487.8 (304–717.9) | 457.6 (345.9–548.35) |
Total nuclear area | 13,731.61 (10,463.36–17,710.37) | 12,484.43 (8,573.66–14,552.81) | 14,454.61 (12,851.46–18,363.69) |
Mean nuclear area | 30.51 (25.21–36.64) | 25.56 (21.31–28.1) | 31.4 (27.69–37.45) |
CD163+ IOD | 43,493.03 (11,570.00–18,069.51) | 14,610.18 (7,855.08–47,464.03) | 28,769.51 (13,709.18–73,075.97) |
CD68+ IOD | 12,106.21 (2,804.34–34,703.97) | 11,226.06 (3,744.11–25,239.30) | 12,703.74 (3,692.15–35,727.09) |
CD8+ IOD | 6,384.48 (3,180.59–16,727.14) | 3,281.45 (2,528.79–9,891.13) | 8,703.94 (4,036.73–17,808.18) |
CD4+ IOD | 8,850.24 (4,689.25–24,689.3) | 7,291.78 (3,835.78–16,309.04) | 10,255.3 (5,495.27–22,603.12) |
Statistical description by median (Q1–Q3). STS, soft tissue sarcoma; FNCLCC, French Federation of Cancer Centers; IOD, integrated optical density.
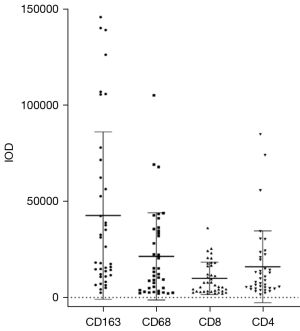
Correlation between the 18F-FDG PET-derived parameters and quantitative pathologic characteristics in patients with STS
The metabolic parameter SUVmax of PET in patients in the enrolled cohort was positively correlated with the total nuclear area of tumor cells (r=0.355, P=0.027). SUVmax was also positively correlated with the expression of CD163, CD68, CD8, and CD4 in tumor tissues (r=0.582, 0.485, 0.343, and 0.324, all P<0.05). The correlation between SUVmax and CD163 was more significant than the correlations with the other immune markers. However, SUVmax was not significantly correlated with cell count and mean nuclear area, and the MTV, TLG, and IFH values were not significantly correlated with the cell count, total nuclear area, and mean nuclear area, respectively (all P>0.05). Moreover, the MTV, TLG, and IFH values were not significantly correlated with the expression levels of the above TIICs (Table 4).
Table 4
Metabolic parameters | Quantitative pathologic characteristics | ||||||
---|---|---|---|---|---|---|---|
Cell counts | Total nuclear area | Mean nuclear area | CD163 | CD68 | CD8 | CD4 | |
SUVmax | |||||||
r | −0.013 | 0.355 | 0.153 | 0.582 | 0.485 | 0.343 | 0.324 |
P | 0.935 | 0.027* | 0.352 | 0.000* | 0.002* | 0.032* | 0.044* |
MTV, cm3 | |||||||
r | −0.210 | 0.015 | 0.109 | 0.196 | 0.213 | 0.291 | 0.241 |
P | 0.200 | 0.929 | 0.508 | 0.232 | 0.193 | 0.072 | 0.139 |
TLG | |||||||
r | −0.188 | 0.095 | 0.135 | −0.283 | 0.278 | 0.287 | 0.283 |
P | 0.251 | 0.565 | 0.413 | 0.081 | 0.087 | 0.052 | 0.081 |
IFH | |||||||
r | −0.068 | 0.143 | 0.110 | −0.091 | −0.003 | 0.162 | 0.022 |
P | 0.681 | 0.385 | 0.506 | 0.581 | 0.985 | 0.323 | 0.896 |
*, P value was statistically significant. FDG, fluorodeoxyglucose; STS, soft tissue sarcoma; SUVmax, maximum standardized uptake value; r, correlation coefficient; MTV, metabolic tumor volume; TLG, total lesion glycolysis; IFH, intertumoral FDG uptake heterogeneity.
Multiple linear regression analysis of the correlation between SUVmax and quantitative pathologic characteristics in patients with STS
With SUVmax as the dependent variable, according to the collinearity test results, the collinearity factor CD68 was first removed; finally, histological grade, the total nuclear area of tumor cells, and the expression of CD163, CD8, and CD4 were included as independent variables in multiple linear regression analysis. Histological grade and CD163 expression of STS were the independent factors associated with SUVmax (Table 5), and the standardized coefficient β was 0.285 and 0.616, respectively (P=0.048 and 0.005). A positive correlation between SUVmax and CD163 expression was observed in both the low-grade group (n=14, r=0.820, P=0.001) and high-grade group (n=25, r=0.430, P=0.028), as presented as a scatter plot in Figure 5. The representative cases are shown in Figures 6,7.
Table 5
Variables | Multiple analysis | ||
---|---|---|---|
β | t value | P value | |
Histological grading | 0.285 | 2.049 | 0.048* |
Total nuclear area | 0.177 | 1.349 | 0.187 |
CD163+ IOD | 0.616 | 3.007 | 0.005* |
CD8+ IOD | 0.212 | 1.503 | 0.142 |
CD4+ IOD | −0.333 | −1.592 | 0.121 |
*, P value was statistically significant. FDG, fluorodeoxyglucose; PET, positron emission tomography; SUVmax, maximum standardized uptake value; IOD, integrated optical density.
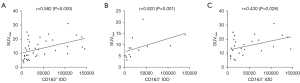
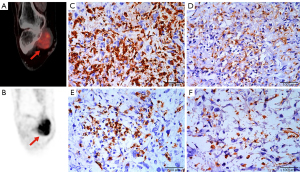

Discussion
Otto Warburg et al., as early as 1920s, indicated that in malignant cells, glycolytic pathways are preferred for metabolic energy supply, even when oxygen is sufficient, and high rates of glucose-metabolism are observed, i.e., the “Warburg Effect” (19). This effect also provides the basis for 18F-FDG PET for tumor imaging using radiotracers to “illuminate” malignant cells on the basis of glucose metabolism in cancer cells (20). According to current understanding, tumors are not considered masses formed by the accumulation of tumor cells present in only specific organs or sites, except in heterogeneous malignant tumor cells, TIIC in the tumor stroma are understood to account for a large proportion of the tumor mass (21). In the present study, we performed the first quantitative digitized analysis of the characteristics of tumor nucleated cells and tumor stromal immune cell infiltrates in STS patient tissues. Further analysis indicated that SUVmax in patients with STS was associated with these described histopathological characteristics, thus providing a new direction and a preliminary theoretical basis for determining the heterogeneity of the biological behavior of STS according to 18F-FDG PET.
Like most other solid tumors, in STS, the tumor stroma is dominated by high density of TAM and TIL (7). TAM are the most abundant tumor stromal cells involved in the host immune system of malignant tumors (22). Previous studies have shown that most macrophages in the STS tumor microenvironment have an M2 macrophage phenotype and high CD163 expression (23). M2 macrophages play crucial roles in promoting STS tumor cell growth and metastasis, stimulating angiogenesis, mediating immunosuppression, and limiting the antitumor activity of conventional chemoradiotherapy, thus revealing that tumors with greater M2 macrophage infiltration have poor prognosis (24,25). Typically, the expression of TIIC in the target type can be identified by pathological examination, but this method is limited by preoperative small sample sampling error and a postoperative pathological lag, and cannot dynamically reflect the global immune cell distribution of the tumor. Therefore, if noninvasive methods can be used to identify and assess levels of important immune cells infiltrating the tumor stroma before treatment, they might aid in predicting prognosis and providing preclinical evidence for screening dominant groups for individualized treatment. Nevertheless, to date, PET-based targeting of the tumor immune microenvironment has not been incorporated into routine clinical practice; therefore, exploring alternative conventional imaging methods is a meaningful research direction.
Hyperglycemia is one of the top ten features of malignant tumors, and FDG uptake detected by PET imaging is a good indicator of its aerobic glycolysis. We hypothesized that STS is a highly heterogeneous malignancy. In addition to tumor cells, the TIIC in tumor stroma may also be associated with altered uptake of 18F-FDG, thus resulting in different PET visual images. A recent cytological experimental study by Reinfeld et al. has shown that macrophages have higher glucose uptake capacity than rectal and breast cancer cells, and other immune cells (26). Another study has found that macrophages promote glycolysis by secreting TNFα in NCLCC (27). Therefore, we speculated that observation of differences in 18F-FDG PET imaging might enable the assessment of the heterogeneity of pathological features in vivo among patients with STS.
Given the diversity of STS histological types, tumors’ nucleated cell features and immune cell infiltration were simultaneously observed in the present study. We observed varying degrees of TAM and TIL in the tumor stroma in patients with STS. The number of TAM exceeded that of TIL, and CD163 was more abundant than CD68, in agreement with previous findings (28). These results might have been because, during the development of malignancy, as tumor tissue cell metabolism is reprogrammed, the TME transforms into a microenvironment conducive to tumor growth. The results of the present study suggest that the SUVmax of STS is positively correlated with the total area of nucleated cells in the tumor, similar to findings in head and neck squamous cell carcinoma and cervical cancer (29,30). We also observed that patients with higher SUVmax had more TAM and TIL in the tumor stroma. SUVmax was more strongly correlated with CD163+ TAM than CD68+ TAM, CD8+ TIL, and CD4+ TIL, and higher than the total area of tumor nucleated cells. In multivariate analysis corrected for total tumor nucleated cell area and other immune cell infiltration, SUVmax remained associated with CD163.
As compared with previous studies (18,31,32), most existing studies have observed correlations between the pathological features of one of the above tumors and imaging markers, ignoring the co-existence of tumor cells and the tumor immune cells as a whole. Few reports have described the correlation in STS. Only a study by Skubitz et al. (22) in 31 patients with STS has found that SUVmax is positively correlated with CD68 expression, but that CD68, a pan-TAM marker, is less clinically meaningful than M2 TAM. In this study, associated tissue sections staining and digital quantification methods were used to analyze the tumor nucleated cell characteristics of STS in H&E stained tissue and tumor immune cell infiltration levels in IHC stained tissue, thus overcoming subjective inter-observer errors, decreasing the shortcomings of including patients with diverse histological types in the cohort, and improving the reliability of the results. According to the results of the present study, higher SUVmax within the same histological grade group was associated with greater infiltration of M2 TAM within the tumor stroma, thus suggesting that higher SUVmax might predict poor prognosis and potential benefits of immunotherapy. However, the results of this study indicated only a moderate correlation between SUVmax and immune markers. To better apply to clinical practice, future studies with large sample sizes remain needed.
In addition, according to the data from this study, no correlations were found between SUVmax on FDG PET and the tumor nucleated cell count and mean nuclear area; moreover, no correlation was found between other metabolic parameters (MTV, TLG, and IFH) and tumor nucleated cell characteristics, as well as the amount of tumor immune cell infiltration. These findings might have been because FDG uptake in malignant cells is related primarily to the proportion of tumor cellularity, but the correlation between FDG uptake and cell density is not significant. However, the biological effects caused by the pathological features of tumor cells, particularly the heterogeneous changes at the TIIC level, span the entire process of tumor occurrence and development (24); consequently, no significant correlation with tumor diameter or volume/size was observed. Recently, IFH values as a parameter of heterogeneity in the distribution of FDG uptake have been proposed by scholars and reported to be predictive of tumor biomarkers in some tumor types (33,34). However, this study did not find an association between IFH and pathological characteristics of STS, possibly because of the small number of enrolled samples or population differences in this cohort. The sample size will be expanded to further perform continuous observation.
Our study has several limitations. First, 18F-FDG PET/CT was performed predominantly before surgery in patients with high grade STS, because clinicians believe that these patients are relatively likely to develop distant metastases. This aspect inevitably led to a smaller sample size in the low-grade group. Second, because of the low morbidity of STS, the sample size was limited, and a single pathological type could not be explored separately. Third, because this was a retrospective study, the choice of radiographically delineated ROIs with pathological tissue sections was inevitably influenced by tumor heterogeneity. Finally, this study describes only preliminary results; whether these immune cells act directly on 18F-FDG uptake and what mechanism might underlie this association remain unclear. A large sample prospective study design and cytological experimental validation will be pursued in our future research.
Conclusions
In conclusion, this study found that FDG uptake may meaningfully reflect the heterogeneity of histopathological features of STSs, and may be considered a surrogate marker for determining the heterogeneity in biological behavior in patients with STS.
Acknowledgments
The authors would like to express their gratitude to colleagues for helping in patient collection.
Funding: This work was supported by grants from
Footnote
Reporting Checklist: The authors have completed the STROBE reporting checklist. Available at https://qims.amegroups.com/article/view/10.21037/qims-23-412/rc
Conflicts of Interest: All authors have completed the ICMJE uniform disclosure form (available at https://qims.amegroups.com/article/view/10.21037/qims-23-412/coif). The authors have no conflicts of interest to declare.
Ethical Statement: The authors are accountable for all aspects of the work in ensuring that questions related to the accuracy or integrity of any part of the work are appropriately investigated and resolved.
Open Access Statement: This is an Open Access article distributed in accordance with the Creative Commons Attribution-NonCommercial-NoDerivs 4.0 International License (CC BY-NC-ND 4.0), which permits the non-commercial replication and distribution of the article with the strict proviso that no changes or edits are made and the original work is properly cited (including links to both the formal publication through the relevant DOI and the license). See: https://creativecommons.org/licenses/by-nc-nd/4.0/.
References
- Rothermundt C, Fischer GF, Bauer S, et al. Pre- and Postoperative Chemotherapy in Localized Extremity Soft Tissue Sarcoma: A European Organization for Research and Treatment of Cancer Expert Survey. Oncologist 2018;23:461-7. [Crossref] [PubMed]
- Hui JY. Epidemiology and Etiology of Sarcomas. Surg Clin North Am 2016;96:901-14. [Crossref] [PubMed]
- Ezuddin NS, Pretell-Mazzini J, Yechieli RL, et al. Local recurrence of soft-tissue sarcoma: issues in imaging surveillance strategy. Skeletal Radiol 2018;47:1595-606. [Crossref] [PubMed]
- In GK, Hu JS, Tseng WW. Treatment of advanced, metastatic soft tissue sarcoma: latest evidence and clinical considerations. Ther Adv Med Oncol 2017;9:533-50. [Crossref] [PubMed]
- Arner EN, Rathmell JC. Metabolic programming and immune suppression in the tumor microenvironment. Cancer Cell 2023;41:421-33. [Crossref] [PubMed]
- Castaneda CA, Castillo M, Aliaga K, et al. Level of tumor-infiltrating lymphocytes and density of infiltrating immune cells in different malignancies. Biomark Med 2019;13:1481-91. [Crossref] [PubMed]
- Fujiwara T, Healey J, Ogura K, et al. Role of Tumor-Associated Macrophages in Sarcomas. Cancers (Basel) 2021;13:1086. [Crossref] [PubMed]
- Ruiz-Mesa C, Goldberg JM, Coronado Munoz AJ, et al. Rhabdomyosarcoma in adults: new perspectives on therapy. Curr Treat Options Oncol 2015;16:27. [Crossref] [PubMed]
- Blay JY, Hindi N, Bollard J, et al. SELNET clinical practice guidelines for soft tissue sarcoma and GIST. Cancer Treat Rev 2022;102:102312. [Crossref] [PubMed]
- Annovazzi A, Ferraresi V, Anelli V, Covello R, Vari S, Zoccali C, Biagini R, Sciuto R. [18F]FDG PET/CT quantitative parameters for the prediction of histological response to induction chemotherapy and clinical outcome in patients with localised bone and soft-tissue Ewing sarcoma. Eur Radiol 2021;31:7012-21.
- Kitao T, Shiga T, Hirata K, et al. Volume-based parameters on FDG PET may predict the proliferative potential of soft-tissue sarcomas. Ann Nucl Med 2019;33:22-31. [Crossref] [PubMed]
- Zhang Q, Xi Y, Li D, et al. The utility of (18)F-FDG PET and PET/CT in the diagnosis and staging of chondrosarcoma: a meta-analysis. J Orthop Surg Res 2020;15:229. [Crossref] [PubMed]
- Iwasa H, Nagamachi S, Nakayama S, et al. The reproducibility of MTV and TLG of soft tissue tumors calculated by FDG-PET: Comparison between the lower limit by the fixed value SUV 2.5 and that value by 30% of SUVmax. Jpn J Radiol 2023;41:531-40. [Crossref] [PubMed]
- Kimura M, Kato I, Ishibashi K, et al. The prognostic significance of intratumoral heterogeneity of 18F-FDG uptake in patients with oral cavity squamous cell carcinoma. Eur J Radiol 2019;114:99-104. [Crossref] [PubMed]
- Coindre JM. Grading of soft tissue sarcomas: review and update. Arch Pathol Lab Med 2006;130:1448-53. [Crossref] [PubMed]
- Sagiyama K, Watanabe Y, Kamei R, et al. Multiparametric voxel-based analyses of standardized uptake values and apparent diffusion coefficients of soft-tissue tumours with a positron emission tomography/magnetic resonance system: Preliminary results. Eur Radiol 2017;27:5024-33. [Crossref] [PubMed]
- Zhang Y, Li H, Jia Y, et al. Noninvasive Assessment of Carotid Plaques Calcification by (18)F-Sodium Fluoride Accumulation: Correlation with Pathology. J Stroke Cerebrovasc Dis 2018;27:1796-801. [Crossref] [PubMed]
- Du P, Ma Q, Zhu ZD, et al. Mechanism of Corilagin interference with IL-13/STAT6 signaling pathways in hepatic alternative activation macrophages in schistosomiasis-induced liver fibrosis in mouse model. Eur J Pharmacol 2016;793:119-26. [Crossref] [PubMed]
- Hardie DG. 100 years of the Warburg effect: a historical perspective. Endocr Relat Cancer 2022;29:T1-13.
- Sigal IR, Sebro R. Preclinical PET tracers for the evaluation of sarcomas: understanding tumor biology. Am J Nucl Med Mol Imaging 2018;8:428-40.
- Fendler WP, Chalkidis RP, Ilhan H, et al. Evaluation of several FDG PET parameters for prediction of soft tissue tumour grade at primary diagnosis and recurrence. Eur Radiol 2015;25:2214-21. [Crossref] [PubMed]
- Skubitz KM, Wilson JD, Cheng EY, et al. Effect of chemotherapy on cancer stem cells and tumor-associated macrophages in a prospective study of preoperative chemotherapy in soft tissue sarcoma. J Transl Med 2019;17:130. [Crossref] [PubMed]
- Cheng N, Bai X, Shu Y, et al. Targeting tumor-associated macrophages as an antitumor strategy. Biochem Pharmacol 2021;183:114354. [Crossref] [PubMed]
- Dumars C, Ngyuen JM, Gaultier A, et al. Dysregulation of macrophage polarization is associated with the metastatic process in osteosarcoma. Oncotarget 2016;7:78343-54.
- Cassetta L, Pollard JW. Tumor-associated macrophages. Curr Biol 2020;30:R246-8. [Crossref] [PubMed]
- Reinfeld BI, Madden MZ, Wolf MM, et al. Cell-programmed nutrient partitioning in the tumour microenvironment. Nature 2021;593:282-8. [Crossref] [PubMed]
- Jeong H, Kim S, Hong BJ, et al. Tumor-Associated Macrophages Enhance Tumor Hypoxia and Aerobic Glycolysis. Cancer Res 2019;79:795-806. [Crossref] [PubMed]
- Dancsok AR, Gao D, Lee AF, et al. Tumor-associated macrophages and macrophage-related immune checkpoint expression in sarcomas. Oncoimmunology 2020;9:1747340. [Crossref] [PubMed]
- Surov A, Meyer HJ, Höhn AK, Winter K, Sabri O, Purz S. Associations Between [18F]FDG-PET and Complex Histopathological Parameters Including Tumor Cell Count and Expression of KI 67, EGFR, VEGF, HIF-1α, and p53 in Head and Neck Squamous Cell Carcinoma. Mol Imaging Biol 2019;21:368-74.
- Surov A, Meyer HJ, Schob S, et al. Parameters of simultaneous 18F-FDG-PET/MRI predict tumor stage and several histopathological features in uterine cervical cancer. Oncotarget 2017;8:28285-96. [Crossref] [PubMed]
- Lim HJ, Johnny Ong CA, Tan JW, et al. Utility of positron emission tomography/computed tomography (PET/CT) imaging in the evaluation of sarcomas: A systematic review. Crit Rev Oncol Hematol 2019;143:1-13. [Crossref] [PubMed]
- Murakami W, Tozaki M, Sasaki M, et al. Correlation between (18)F-FDG uptake on PET/MRI and the level of tumor-infiltrating lymphocytes (TILs) in triple-negative and HER2-positive breast cancer. Eur J Radiol 2020;123:108773. [Crossref] [PubMed]
- Hua T, Zhou W, Zhou Z, et al. Heterogeneous parameters based on (18)F-FET PET imaging can non-invasively predict tumor grade and isocitrate dehydrogenase gene 1 mutation in untreated gliomas. Quant Imaging Med Surg 2021;11:317-27. [Crossref] [PubMed]
- Li R, Lin J, Wang L, et al. The association between 18F-fluorodeoxyglucose PET intratumoral metabolic heterogeneity and pathological parameters in non-small cell lung cancer. Nucl Med Commun 2019;40:1022-8. [Crossref] [PubMed]