A background parenchymal enhancement quantification framework of breast magnetic resonance imaging
Introduction
The addition of semi-quantitative background parenchymal enhancement (BPE) to the fifth edition of the Breast Imaging Reporting and Data System (BI-RADS) marks a significant milestone in the interpretation of breast magnetic resonance imaging (MRI) (1). BPE refers to the proportion of normal fibroglandular tissue (FGT) that enhances at varying grades on breast MRI after the administration of gadolinium-based contrast material (2). BPE can be a confounding factor in the interpretation of breast MRI, as it can mimic or mask the presence of malignant lesions (3,4). However, visual semi-quantitative assessment of BPE has faced challenges due to poor inter- and intrareader agreements (2,5,6). Quantitative assessment has the potential to help distinguish BPE from lesions, address potential MRI diagnostic challenges, and reduce the risk of overdiagnosis or missed diagnosis (2,4). Notably, BPE holds promise as a reliable risk factor in clinical breast cancer risk assessment methods and as an independent prognostic and predictive biomarker of neoadjuvant chemotherapy (NAC) (5,7-9).
In theory, BPE quantification could involve calculating its ratio of range, area, or volume ratio to the that of the FGT or the breast as well as the intensity of enhancement on dynamic contrast enhancement (DCE) (1). The proposed framework aims to quantify BPE by integrating its volume and intensity of enhancement on breast MRI. The resulting BPE quantitative imaging biomarker (QIB) could provide a better insight into the impact of BPE on breast MRI interpretation. This paper outlines this framework and demonstrates its efficacy.
Methods
Committee approval
The study was conducted in accordance with the Declaration of Helsinki (as revised in 2013) and was reviewed and approved by the Ethics Committee of Chinese People’s Liberation Army General Hospital (No. S2019-093-01). Written informed consent from the patients for this retrospective analysis was waived.
Study population
This study included a total of 2,786 cases that were quantified using the proposed framework from August 2012 to September 2019. Initially, there were 2,907 cases, but 121 cases were excluded based on the exclusion criteria to ensure the quality of the data. The exclusion criteria included a history of surgical intervention before MRI examination, implant or injection mammoplasty, unilateral breast data deficiency (a lack of bilateral breast symmetry for evaluation), and poor image quality.
Imaging protocols
A 3.0-Tesla MRI scanner with an 8-channel phased array breast coil was used to conduct the MRI examinations (Discovery 750, GE HealthCare, Chicago, IL, USA). The imaging protocol, lasting for 18 minutes, included 4 pulse sequences: diffusion-weighted imaging (DWI), T2-weighted imaging (T2WI), T1-weighted imaging (T1WI), and DCE. All sequences were spatially matched in axial view, with a field of view of 320 mm × 320 mm and 190 mm of z-axis coverage. The b value of DWI was 0 and 1,000 s/mm2 in 3 orthogonal diffusion gradients, the inversion recovery (IR) was 250 ms for fat suppression, the repetition time (TR) was 5,400 ms, minimum echo time (TE), and the matrix size was 128×128. The T2WI used iterative decomposition of water and fat with echo asymmetric and least squares estimation (IDEAL) for fat suppression, with a TR of 5,000 ms, a TE of 68 ms, and a matrix size of 320×256 (10). Both T1WI and DCE were performed with the same volume imaging for breast assessment (VIBRANT), spectral inversion at lipids (SPECIAL), and 3-dimensional spoiled gradient recall sequences. The SPECIAL option was disabled for non-fat-suppression T1WI. The T1WI and DCE had the same geometric location and were performed with the following parameters: an isotropic spatial resolution of 1.0 mm × 1.0 mm × 1.0 mm, 192 partitions in the axial view, a minimum TR/TE, and a flip angle of 120°. The DCE scan repeated 6 continuous phases without interruption, each of which lasted 120 seconds (s). Following the pre-contrast phase, 0.5 M of gadopentetic acid (Gd-DTPA) was administered into the antecubital vein at a rate of 2 mL/s and a dose of 0.1 mmol/kg body weight and was followed by a 20-mL flush of saline. Table 1 summarizes the parameters of MRI.
Table 1
Group | Mean (95% CI) | Comparison | |||
---|---|---|---|---|---|
Among the 3 groups (Asymp. Sig.*) | Pairwise comparisons (Adj. Sig.*) | ||||
Mild vs. moderate | Mild vs. marked | Moderate vs. marked | |||
Mild | 0.0756 (0.0739, 0.0772) | <0.001 | <0.001 | <0.001 | <0.001 |
Moderate | 0.1263 (0.1236, 0.1290) | ||||
Marked | 0.1585 (0.1519, 0.1650) |
*, significance values adjusted with the Bonferroni correction for multiple tests. Comparisons between multiple groups of nonnormally distributed BPE integral were performed using the Kruskal-Wallis test. The significance level is 0.05. BPE, background parenchymal enhancement; CI, confidence interval; Asymp., asympotic; Sig., significance; Adj., adjusted.
The imaging processing included early enhancement ratio and time-intensity curve (TIC). The intensity of the enhancement ratio (R) was the proportion of the enhanced phase to the pre-contrast phase. Before administration of the injection medication, a pre-scan baseline image was taken and labeled as phase 0. The subsequent images taken after the injection of medication were labeled as time phases 1, 2, 3, and 4, respectively. The R for each phase (n = phase, I = intensity of enhancement) is shown in Eq. [1] below:
The TIC was classified using the enhancement ratio difference between the first-enhanced phase and the last-enhanced phase (8–10 minutes after contrast delivery) (1). The persistent, plateau, and washout TICs were defined as enhancement ratios of <−10%, −10% to 10%, and >10%, respectively (1).
Semi-quantitative BPE assessment
In order to acquire semi-quantitative BPE results, 3 experienced radiologists (with 2, 3, and 5 years of experience, respectively) independently assessed the BPE grade as mild, moderate, or marked based on the fifth edition of the BI-RADS.
Model architecture of the BPE quantification framework
Figure 1 illustrates the process of the BPE quantification framework.
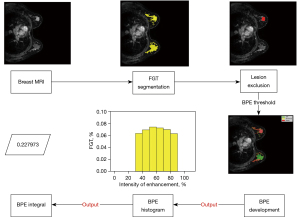
FGT segmentation and lesion exclusion
FGT was automatically segmented using a 2D convolutional neural network, Deep High-Resolution Net (HR-Net), the details of which are shown in the Appendix 1. Before BPE grading, the breast lesions were excluded using a lesion segmentation model, which developed in house using V-Net (11).
BPE identification and quantification
To quantify BPE, we calculated the voxel-wise relative intensity of enhancement from the pre-contrast sequence to the post-contrast sequence using Eq. [1] with n=1 of Eq. [1] as follows: . This yielded the intensity of enhancement ratio for the first phase (early intensity enhancement rate). A voxel was identified as BPE if it satisfied 2 criteria: (I) its first rate of intensity enhancement (R1) fell within the range of 30–90%, and (II) it belonged to the FGT. Only voxels exhibiting persistent TIC were identified as BPE, as plateau and washout TICs were indicative of lesions. Additionally, we excluded voxels with high signals on DWI, as this is also a sign of a lesion.
Voxels that satisfied the BPE definition in terms of R (%) were selected to construct the BPE histogram, as the intensity of enhancement and volume of intensity of enhancement were the primary parameters used to define BPE. In the histogram, the Y-axis (FGT volume ratio) represented the ratio of the BPE volume to that of FGT, while the X-axis (intensity enhancement) represented the intensity of enhancement with a 10% interval.
The BPE integral was identified as the QIB of BPE and was calculated from the BPE histogram (Figure 1) using the following equation:
The i=3 means intensity of enhancement ranging from 30–40%. Continuing in this manner, until i=8 signifies the range of 80–90% intensity of enhancement.
The BPE quantification of 2,786 cases was performed on MatLab 2018b (MathWorks, Natick, MA, USA).
Statistical analysis
The FGT segmentation and lesion labeling models were assessed using the Dice similarity coefficient (DSC). Comparisons between multiple groups of the nonnormally distributed BPE integral were performed using the Kruskal-Wallis test. Table 1 was carried out using SPSS version 26.0 (IBM Corp., Armonk, NY, USA). Other statistical analyses of Figure 2 were performed with R software version 4.2.1 (The R Foundation for Statistical Computing) with the “ggplot2” package being used for data visualization. P<0.05 indicated a statistically significant difference.
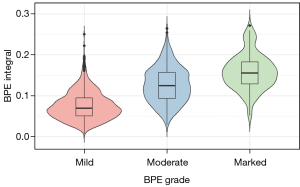
Results
A clear association between BPE integral and BPE grades was demonstrated. The findings indicated statistically significant differences in the BPE integral between the 3 BPE grades labeled by the radiologists (P<0.001): mild-moderate (P<0.001), mild-marked (P<0.001), and moderate-marked (P<0.001) (Table 1). As shown in Figure 2 and Table 2, further post-hoc analyses revealed that marked BPE exhibited the highest BPE integral, followed by moderate BPE, with mild BPE demonstrating the lowest BPE integral. The results of FGT segmentation and the lesion-labeling models are available in Appendix 2.
Table 2
Sequence | Plane | FOV (cm) | Slice thickness (mm) | Gap | TR (ms) | TE (ms) | Matrix | FA (°) | NEX | Fat-sat technique | RBW (kHZ) | Parallel acceleration | TA (min:s) |
---|---|---|---|---|---|---|---|---|---|---|---|---|---|
VIBRANT T1WI | Ax | 32 | 1 | 3D | 4.9 | 2.3 | 320×320 | 10 | 1 | – | 62.5 | 2 | 1:49 |
IDEAL T2WI | Ax | 32 | 4 | 1 | 4,000 | 68 | 320×256 | 111 | 1 | Dixon | 83.3 | 2 | 4:00 |
STIR DWI | Ax | 32 | 4 | 1 | 4,000 | Minimum | 128×128 | – | 6 | STIR | 250 | 2 | 2:40 |
VIBRANT dynamic T1WI | Ax | 32 | 1 | 3D | 7.7 | 4.3 | 320×320 | 10 | 1 | SPECIAL/water excitation | 125 | 3 | 9:51 |
MRI, magnetic resonance imaging; FOV, field of view; TR, repetition time; TE, echo time; FA, flip angle; NEX, number of excitation; Fat-sat, fat saturation; RBW, resolution bandwidth; TA, acquisition time; VIBRANT, volume imaging for breast assessment; T1WI, T1-weighted imaging; IDEAL, iterative decomposition of water and fat with echo asymmetric and least squares estimation; T2WI, T2-weighted imaging; STIR, short time inversion recovery; DWI, diffusion-weighted imaging; Ax, Axial; 3D, three-dimensional; SPECIAL, spectral inversion at lipids.
Discussion
The purpose of this study was to develop a BPE quantification framework that can output a BPE integral value by combining both volume and intensity of enhancement to establish a standardized and automated approach for breast MRI interpretation and contribute to a BPE QIB. A total of 2,786 cases were included in this study.
Our study found a considerable correspondence between our BPE quantitative integral framework and the semi-quantitative assessments performed by radiologists, underscoring the reliability and reproducibility of our approach. Our BPE integral was shown to be an effective and valuable QIB that demonstrated meaningful variations among different BPE grades labeled by radiologists. Importantly, the BPE histograms effectively presented comprehensive BPE information in a clear and concise manner, as illustrated in Figure 1. These findings highlight the viability of using the BPE integral as a QIB for accurate and standardized interpretation of breast MRI.
The BPE quantification framework was developed according to the expertise of radiology professionals. BPE is defined by both the volume and intensity of enhancement (1). However, a QIB that combines volume and intensity of enhancement has not been developed (12-19). To construct this BPE quantification framework, the BPE voxels were defined as those with an intensity of enhancement falling within the range of 30–90%, according to clinical experience that suggests voxels with a <30% intensity of enhancement are typically imperceptible to radiologists. Additionally, voxels with an intensity of enhancement greater than 90% may not accurately represent normal tissue. Given the challenge of distinguishing between lesions and severe BPE, accurate quantification of BPE requires the removal of lesions to obtain a more reliable measurement of BPE. A lesion segmentation model is used for this purpose. Voxels with an intensity of enhancement between 90% and 120% are considered to be in an early stage of lesion development, while those with an intensity of enhancement greater than 120% are identified as lesions (1,20). Furthermore, the persistent TIC limit was added to the exclusion criteria, as plateau TIC and washout TIC are typically indicative of malignancy or non-mass enhancement (1,20).
The problem of lacking distinct BPE integral ranges between different BPE grades labeled by radiologists may be partly attributable to the inherent subjectivity of the radiologist’s interpretation, which is a pervasive phenomenon in clinical practice. This phenomenon can be explained by the following variables: (I) BPE is a dynamic procedure that varies from woman to woman and across time in the same woman (1); (II) the clinical absence of restricting MRI scheduling based on the menstrual cycle phase could partly impair the interpretation of radiologists (2); (III) patient movement during the MRI procedure will affect the interpretation. The lack of consensus among radiologists in the semi-quantitative grading of BPE on breast MRI highlights the need for a standardized and automated quantification approach. Therefore, the BPE quantification pipelines developed in this study offer a promising approach to standardizing the interpretation of BPE, potentially reducing the impact of interreader and intrareader variation.
It is important to note that the use of BPE quantification pipelines is not intended to replace expert radiological interpretation but to rather act as a complementary tool that can improve accuracy and consistency, potentially reducing subjectivity and variability (21,22). Therefore, combining expert radiological interpretation with quantitative analysis may provide a comprehensive and reliable assessment of BPE. Furthermore, the development of BPE research may produce an updated framework.
Further research should include reader studies to evaluate the effectiveness of the BPE quantification framework and to assess the confidence of radiologists in adopting this method. It may also be useful to compare the performance of specialists and those without expertise in interpreting BPE using this framework.
Additionally, BPE has the potential to function as an independent risk factor for breast cancer and as a predictive biomarker in NAC (5,7-9,23). However, various BPE measurement techniques have yielded inconsistent results, which could be attributed to the fact that there is a wide diversity of BPE measurement methods and no reliable or reproducible quantification framework for BPE (5,7-9,23,24). The development of an automated and standardized BPE quantitative evaluation framework, such as the one presented in this study, has the potential to facilitate the incorporation of BPE as a QIB into clinical practice, particularly in the era of personalized medicine.
The study has several main limitations. First, the BPE histograms did not include other texture elements such as distortion, kurtosis, and skewness (17,22). Second, there was no restriction on the menstrual cycle phase. Third, as there was an exclusive focus on Chinese females, who typically exhibit higher breast density, resulting in only mild, moderate, and marked BPE grades (25). Consequently, this study was unable to investigate the quantification of minimal BPE despite the proposed framework’s capability to quantify it.
Conclusions
We developed a novel and reliable BPE quantification framework that output a BPE integral value according to clinical experience, incorporating both the volume and intensity of enhancement. Moreover, the use of the BPE histogram as a visual representation of the BPE quantification parameters further enhances the interpretability and ease of application of the framework. Our approach may improve the accuracy and reproducibility of BPE assessment and facilitate its incorporation into clinical practice.
Acknowledgments
Funding: None.
Footnote
Conflicts of Interest: All authors have completed the ICMJE uniform disclosure form (available at https://qims.amegroups.com/article/view/10.21037/qims-23-514/coif). The authors have no conflicts of interest to declare.
Ethical Statement: The authors are accountable for all aspects of the work in ensuring that questions related to the accuracy or integrity of any part of the work are appropriately investigated and resolved. The study was conducted in accordance with the Declaration of Helsinki (as revised in 2013) and was reviewed and approved by the Ethics Committee of Chinese People’s Liberation Army General Hospital (No. S2019-093-01). Written informed consent from the patients for this retrospective analysis was waived.
Open Access Statement: This is an Open Access article distributed in accordance with the Creative Commons Attribution-NonCommercial-NoDerivs 4.0 International License (CC BY-NC-ND 4.0), which permits the non-commercial replication and distribution of the article with the strict proviso that no changes or edits are made and the original work is properly cited (including links to both the formal publication through the relevant DOI and the license). See: https://creativecommons.org/licenses/by-nc-nd/4.0/.
References
- Fowler EE, Sellers TA, Lu B, Heine JJ. Breast Imaging Reporting and Data System (BI-RADS) breast composition descriptors: automated measurement development for full field digital mammography. Med Phys 2013;40:113502. [Crossref] [PubMed]
- Rella R, Bufi E, Belli P, Contegiacomo A, Giuliani M, Rosignuolo M, Rinaldi P, Manfredi R. Background parenchymal enhancement in breast magnetic resonance imaging: A review of current evidences and future trends. Diagn Interv Imaging 2018;99:815-26. [Crossref] [PubMed]
- Giess CS, Yeh ED, Raza S, Birdwell RL. Background parenchymal enhancement at breast MR imaging: normal patterns, diagnostic challenges, and potential for false-positive and false-negative interpretation. Radiographics 2014;34:234-47. [Crossref] [PubMed]
- Ray KM, Kerlikowske K, Lobach IV, Hofmann MB, Greenwood HI, Arasu VA, Hylton NM, Joe BN. Effect of Background Parenchymal Enhancement on Breast MR Imaging Interpretive Performance in Community-based Practices. Radiology 2018;286:822-9. [Crossref] [PubMed]
- Rella R, Bufi E, Belli P, Scrofani AR, Petta F, Borghetti A, Marazzi F, Valentini V, Manfredi R. Association between contralateral background parenchymal enhancement on MRI and outcome in patients with unilateral invasive breast cancer receiving neoadjuvant chemotherapy. Diagn Interv Imaging 2022;103:486-94. [Crossref] [PubMed]
- Liao GJ, Henze Bancroft LC, Strigel RM, Chitalia RD, Kontos D, Moy L, Partridge SC, Rahbar H. Background parenchymal enhancement on breast MRI: A comprehensive review. J Magn Reson Imaging 2020;51:43-61. [Crossref] [PubMed]
- Lee SH, Jang MJ, Yoen H, Lee Y, Kim YS, Park AR, Ha SM, Kim SY, Chang JM, Cho N, Moon WK. Background Parenchymal Enhancement at Postoperative Surveillance Breast MRI: Association with Future Second Breast Cancer Risk. Radiology 2023;306:90-9. [Crossref] [PubMed]
- Brooks JD, Christensen RAG, Sung JS, Pike MC, Orlow I, Bernstein JL, Morris EA. MRI background parenchymal enhancement, breast density and breast cancer risk factors: A cross-sectional study in pre- and post-menopausal women. NPJ Breast Cancer 2022;8:97. [Crossref] [PubMed]
- Bauer E, Levy MS, Domachevsky L, Anaby D, Nissan N. Background parenchymal enhancement and uptake as breast cancer imaging biomarkers: A state-of-the-art review. Clin Imaging 2022;83:41-50. [Crossref] [PubMed]
- Reeder SB, McKenzie CA, Pineda AR, Yu H, Shimakawa A, Brau AC, Hargreaves BA, Gold GE, Brittain JH. Water-fat separation with IDEAL gradient-echo imaging. J Magn Reson Imaging 2007;25:644-52. [Crossref] [PubMed]
- Zhu J, Geng J, Shan W, Zhang B, Shen H, Dong X, Liu M, Li X, Cheng L. Development and validation of a deep learning model for breast lesion segmentation and characterization in multiparametric MRI. Front Oncol 2022;12:946580. [Crossref] [PubMed]
- Borkowski K, Rossi C, Ciritsis A, Marcon M, Hejduk P, Stieb S, Boss A, Berger N. Fully automatic classification of breast MRI background parenchymal enhancement using a transfer learning approach. Medicine (Baltimore) 2020;99:e21243. [Crossref] [PubMed]
- Ha R, Mema E, Guo X, Mango V, Desperito E, Ha J, Wynn R, Zhao B. Three-Dimensional Quantitative Validation of Breast Magnetic Resonance Imaging Background Parenchymal Enhancement Assessments. Curr Probl Diagn Radiol 2016;45:297-303. [Crossref] [PubMed]
- Wei D, Jahani N, Cohen E, Weinstein S, Hsieh MK, Pantalone L, Kontos D. Fully automatic quantification of fibroglandular tissue and background parenchymal enhancement with accurate implementation for axial and sagittal breast MRI protocols. Med Phys 2021;48:238-52. [Crossref] [PubMed]
- Goodburn R, Kousi E, Sanders C, Macdonald A, Scurr E, Bunce C, Khabra K, Reddy M, Wilkinson L, O'Flynn E, Allen S, Schmidt MA. Quantitative background parenchymal enhancement and fibro-glandular density at breast MRI: Association with BRCA status. Eur Radiol 2023;33:6204-12. Erratum in: Eur Radiol 2023;33:6621. [Crossref] [PubMed]
- Luczynska E, Pawlak M, Piegza T, Popiela TJ, Heinze S, Dyczek S, Rudnicki W. Analysis of background parenchymal enhancement (BPE) on contrast enhanced spectral mammography compared with magnetic resonance imaging. Ginekol Pol 2021;92:92-7. [Crossref] [PubMed]
- Lam DL, Hippe DS, Kitsch AE, Partridge SC, Rahbar H. Assessment of Quantitative Magnetic Resonance Imaging Background Parenchymal Enhancement Parameters to Improve Determination of Individual Breast Cancer Risk. J Comput Assist Tomogr 2019;43:85-92. [Crossref] [PubMed]
- Moliere S, Oddou I, Noblet V, Veillon F, Mathelin C. Quantitative background parenchymal enhancement to predict recurrence after neoadjuvant chemotherapy for breast cancer. Sci Rep 2019;9:19185. [Crossref] [PubMed]
- Niell BL, Abdalah M, Stringfield O, Raghunand N, Ataya D, Gillies R, Balagurunathan Y. Quantitative Measures of Background Parenchymal Enhancement Predict Breast Cancer Risk. AJR Am J Roentgenol 2021;217:64-75. [Crossref] [PubMed]
- Zhong Y, Li M, Zhu J, Zhang B, Liu M, Wang Z, Wang J, Zheng Y, Cheng L, Li X. A simplified scoring protocol to improve diagnostic accuracy with the breast imaging reporting and data system in breast magnetic resonance imaging. Quant Imaging Med Surg 2022;12:3860-72. [Crossref] [PubMed]
- Eskreis-Winkler S, Sutton EJ, D'Alessio D, Gallagher K, Saphier N, Stember J, Martinez DF, Morris EA, Pinker K. Breast MRI Background Parenchymal Enhancement Categorization Using Deep Learning: Outperforming the Radiologist. J Magn Reson Imaging 2022;56:1068-76. [Crossref] [PubMed]
- Nam Y, Park GE, Kang J, Kim SH. Fully Automatic Assessment of Background Parenchymal Enhancement on Breast MRI Using Machine-Learning Models. J Magn Reson Imaging 2021;53:818-26. [Crossref] [PubMed]
- Sorin V, Yagil Y, Shalmon A, Gotlieb M, Faermann R, Halshtok-Neiman O, Sklair-Levy M. Background Parenchymal Enhancement at Contrast-Enhanced Spectral Mammography (CESM) as a Breast Cancer Risk Factor. Acad Radiol 2020;27:1234-40. [Crossref] [PubMed]
- Xu C, Yu J, Wu F, Li X, Hu D, Chen G, Wu G. High-background parenchymal enhancement in the contralateral breast is an imaging biomarker for favorable prognosis in patients with triple-negative breast cancer treated with chemotherapy. Am J Transl Res 2021;13:4422-36.
- Mariapun S, Li J, Yip CH, Taib NA, Teo SH. Ethnic differences in mammographic densities: an Asian cross-sectional study. PLoS One 2015;10:e0117568. [Crossref] [PubMed]