Deep learning radiomics analysis based on computed tomography for survival prediction in gastric neuroendocrine neoplasm: a multicenter study
Introduction
Gastric neuroendocrine neoplasms (gNENs) are rare and heterogeneous tumors whose incidence is increasing worldwide due to improved recognition and diagnosis technologies of neuroendocrine neoplasms as distinct tumor types (1). The latest World Health Organization (WHO) guidelines classify gNENs into well-differentiated neuroendocrine tumor (NET), poorly differentiated neuroendocrine carcinoma (NEC), and mixed neuroendocrine-nonneuroendocrine neoplasms (MiNENs) based on their molecular characteristics (2).
The prognosis of poorly differentiated gNENs, which includes gastric NEC and MiNENs, differs significantly from the well-differentiated gNENs. According to a previous study of 900 patients with gNENs, the 3- and 5-year overall survival (OS) rates of gastric NEC are much lower than those of gastric NET (3). Several reports suggest that there is a significant difference in biological behavior between NET and NEC, with NEC being more aggressive and being associated with a poorer prognosis (4,5), Nevertheless, purely anatomically based prognostic systems may not fully account for tumor heterogeneity for predicting long-term outcomes for NEN (6-9). The heterogeneity of gNENs introduces a challenge, and these require different treatment strategies. To address this urgent issue, it is necessary to identify a novel and dependable biomarker that can accurately differentiate gNENs for clinical decision-making (10).
Endoscopic biopsies, which are invasive in nature, are widely regarded as the most reliable method for diagnosing gNENs and have been endorsed by the European Neuroendocrine Tumor Society (ENETS) (11). However, with biopsies, sample errors in diagnosing histopathological features are unavoidable. Computed tomography (CT) is a useful tool for predicting the prognosis of both gastric cancer and gNENs, making it an indispensable diagnostic method (12). Its widespread recognition is due to its high diagnostic accuracy and consistent availability. The sensitivity and specificity of CT for diagnosing gNENs and gastric cancer range from 61% to 93% and from 71% to 100%, respectively (13). Nevertheless, the prognostic significance of conventional medical imaging is fundamentally restricted by mutual accuracy and reproducibility. As a newly emerging image quantization technique, radiomics has been widely applied across various diseases, especially in the diagnosis and prognosis of tumors, and can provide a basis for the accurate treatment of tumors (14-16). A radiomics nomogram, a noninvasive model visualization tool that integrates radiomics signatures and clinical factors, has potential application in differential diagnosis and the prediction of clinical outcomes (17-19). The emergence of deep learning (DL) techniques has significantly enhanced the capabilities of radiomics, facilitating the automated acquisition of correlative quantitative representations of tumor phenotypes (20). Prior studies have demonstrated that the DL radiomics analysis based on the on convolutional neural networks (CNNs) are effective in cancer prognosis (21,22). However, the application of DL radiomics analysis for individualized evaluation of OS in gNENs has not yet been reported.
Given the above considerations, we collected CT images and independent clinical factors before treatment to build and evaluate a DL-based radiomics model and investigated whether DL radiomics analysis could predict the prognosis of patients with gNENs. We present this article in accordance with the TRIPOD reporting checklist (available at https://qims.amegroups.com/article/view/10.21037/qims-23-577/rc).
Methods
Patients
In this retrospective, multicenter study, patients with gNENs who had been pathologically confirmed through endoscopic biopsy or surgical resection were recruited in two hospitals (The First Affiliated Hospital of Zhengzhou University and The Henan Cancer Hospital). The inclusion criteria were as follows: (I) patients aged older than 18 years; (II) patients with gNENs with confirmed biopsy pathology or postoperative pathological diagnosis; (III) multiphase enhanced abdominal CT imaging performed before treatment; (IV) relatively complete clinical and pathological data; and (V) complete and dependable follow-up information. The following exclusion criteria were applied: (I) pathological findings obtained after neoadjuvant chemotherapy, radiotherapy, or other treatments; (II) metastatic gNENs; (III) poor CT image quality with severe artifacts; (IV) a minimum diameter of the tumor <5 mm insufficient to contain the region of interest (ROI); and (V) severe heart, liver, or kidney dysfunction or other primary tumors. The recruitment process is shown in Figure 1. All clinical data were gathered from the medical and endoscopic reports. The eight edition of the American Joint Committee on Cancer (AJCC) TNM staging manual [2017] was used for tumor staging (23).
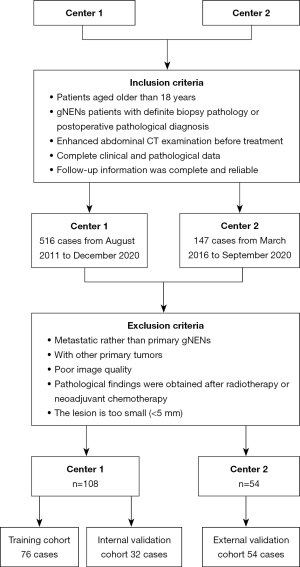
This retrospective study was approved by the Institutional Review Board of the First Affiliated Hospital of Zhengzhou University (No. 2021-KY-1070-002), and the requirement for informed consent was waived due to the retrospective nature of the study. Both participating hospitals were informed of and agreed with the study. The study was conducted in accordance with the Declaration of Helsinki (as revised in 2013).
Baseline staging evaluations included ultrasound endoscopy and CT. The treatment was jointly decided upon by multidisciplinary discussion with oncologists, radiologists, and surgeons for the patients with gNENs in accordance with the AJCC guidelines. Finally, 162 patients with gNENs were enrolled from two centers. Among them, 108 patients from the First Affiliated Hospital of Zhengzhou University were randomly divided into a training cohort (n=76) and an internal validation cohort (n=32) at a ratio of 7:3 between August 2011 and December 2020. Additionally, 54 patients from the Henan Cancer Hospital were placed in the external validation between March 2016 and September 2020. The primary endpoint of this study was OS, which was considered to be the duration from the date of diagnosis to death or the end date of follow-up. The follow-up information for OS is detailed in Supplementary file (Appendix 2) .
CT acquisition and tumor segmentation
All patients from the two hospitals underwent biphasic (arterial and venous phase) enhanced CT scanning before surgery. The detailed CT acquisition procedure and protocols are described in Table S1. Manual segmentation was performed on the tumor in arterial and venous phases CT images using ITK-SNAP software (version3.6.0; www.itk-snap.org). The tumor ROIs were manually segmented along the margin of the tumor on the cross-section of biphasic phases. The segmentation procedure was performed by two radiologists with more than 10 years of experience. For each phase, 1 slice with the maximum cross-sectional area of the lesion was chosen visually by the radiologist, and a 2-dimensional ROI of the tumor was delineated. If a consensus concerning tumor region segmentation could not be reached, this would be decided upon by a third senior abdominal radiologist. After 1 month, we randomly selected 30 patients in the training cohort for ROI segmentation again to evaluate the intraobserver reproducibility. The features with intraclass correlation coefficients (ICCs) greater than 0.8 were considered reliable and retained for further analysis (24).
DL feature extraction
CT images in venous and arterial phases need to first be standardized to avoid variability in reconstruction slice thickness and voxel spacing. By applying the bicubic spline interpolation algorithm, we resampled the raw images into a 1×1×1 mm3 voxel size (24). We constructed a DL model based on residual CNN (ResNet-50 architecture), with the input of segment CT images (size: 224 mm × 224 mm) (25). ResNet-50 significantly outperforms conventional CNNs on challenging object recognition benchmark tasks while requiring less computational resources and having fewer parameters and is thus more practical and accessible. The pretrained ResNet-50 model is available online, but for this study, the last fully connected layer at the top of the model was removed. Global max pooling strategies were used to extract the maximum values from each layer of the feature maps, which were then transformed into their original feature values. The details of the DL model are described in the Supplementary file (Appendix 1 and Appendix 2).
Building of the DL-based radiomics signature
Feature selection and radiomics signatures building were performed in the training cohort. The two radiomics signatures were respectively built as follows. First, ICCs were calculated from the resegmentation data to evaluate the reproducibility of features. Only those features with ICCs >0.8 were selected further analysis. We then applied the least absolute shrinkage and selection operator (LASSO) Cox regression model to identify the most useful prognostic features among all the DL features. Subsequently, a multiple feature-based radiomics signature, known as the radiomics score, was built to predict survival in the training cohort. Finally, we built two DL-based radiomics signatures to reflect the phenotypic characteristics of the primary tumor from two phases of CT images: the arterial and venous phases. The “glmnet” package in R (The R Foundation of Statistical Computing) was used to perform the LASSO Cox regression model analysis.
Construction of an individualized radiomics nomogram
To evaluate the predictive performance of the DL-based radiomics signature (Modeldl) for OS, we conducted initial assessments in the training cohort and then verified its performance in the internal validation cohort and external validation cohort. Additionally, to compare the prognostic performance between the DL-based radiomics signatures and conventional clinical features, we built a clinical model (Modelclinic) using the multivariable Cox proportional hazards (CPH) method based on independent clinical prognostic factors.
The univariate and multivariate CPH models were employed to investigate the association between the clinical risk factors and OS of gNENs in the training cohort. Subsequently, a combined model (Modelclinic + dl) incorporating the DL radiomics signatures and clinical factors was built through the multivariable CPH method. Figure 2 shows the architectures of the DL radiomics analysis in this study. Finally, we visualized Modelclinic + dl as a radiomics nomogram to assist clinicians in conveniently obtaining individualized OS estimates. The performance of Modelclinic + dl was quantitatively evaluated using hazard ratio (HR) and the Harrell concordance index (C-index). Furthermore, risk stratification for the radiomics nomogram was performed, and a specific threshold was determined in the training cohort and locked during the validation phase.
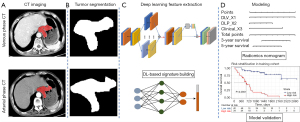
Verification and evaluation of the radiomics nomogram
Kaplan-Meier (KM) curves were adopted to investigate the potential association between the models and OS. To evaluate the agreement between the observed actual OS rate and the nomogram-predicted OS rate, calibration curves were employed. In order to compare the predictive power of Modelclinic + dl with Modeldl and Modelclinic, time-dependent receiver operating characteristic (TDROC) analysis (26), which could handle censored data, was adopted. To ascertain the network benefit, we adopted decision curve analysis (DCA) to validate the prognostic value of Modelclinic + dl.
Statistical analysis
A 2-tailed P value <0.05 was set as the threshold for statistical significance. Univariate analysis was performed with the independent t-test or Mann-Whitney test for numeric variables. The differences of categorical data were analyzed with the chi-squared test or Fisher exact test. The KM curves for OS were plotted, with 1 month being defined as 30 days. The HRs and C-index were analyzed with confidence intervals (CIs) of 95%, along with the corresponding P values (27,28). In the multivariable CPH method, Akaike information was used as the optimization criterion, and backward stepwise selection was adopted. The Schoenfeld residuals test was performed for each clinical factors, factors with P value larger than 0.05 were considered eligible for Cox regression. We used R version 4.0.3 (https://www.r-project.org) and Python version 3.6 (The Python Software Foundation; https://www.python.org) to conduct statistical analysis and graphic representation.
Results
Baseline clinical characteristics for the training, internal validation, and external validation cohorts are summarized in Table 1. Of the 162 patients included in the study, 119 (73.5%) were men, and the median age of all patients was 62.57 years. The median OS in the training cohort, internal validation cohort, and external validation cohort was 32, 34 and 25 months, respectively.
Table 1
Characteristics | Training cohort (n=76) | Internal validation cohort (n=32) | External validation cohort (n=54) | P value |
---|---|---|---|---|
Age (years) | 61.70±11.31 | 63.06±11.39 | 64.50±9.15 | 0.274 |
Sex | 0.617 | |||
Female | 23 (30.3) | 8 (25.0) | 12 (22.2) | |
Male | 53 (69.7) | 24 (75.0) | 42 (77.8) | |
Location | 0.364 | |||
Cardia/fundus | 41 (54.0) | 17 (53.1) | 27 (50.0) | |
Body | 21 (27.6) | 11 (34.4) | 15 (27.8) | |
Antrum | 9 (11.8) | 1 (3.1) | 2 (3.7) | |
≥2/3 stomach | 5 (6.6) | 3 (9.4) | 10 (18.5) | |
Long diameter (mm) | 44.80±27.49 | 44.57±29.71 | 53.97±27.55 | 0.163 |
CT-reported LN | 0.912 | |||
No | 39 (51.3) | 15 (46.9) | 26 (48.1) | |
Yes | 37 (48.7) | 17 (53.1) | 28 (51.9) | |
M stage | 0.927 | |||
M0 | 59 (77.6) | 25 (78.1) | 43 (79.6) | |
M1 | 17 (22.4) | 7 (21.9) | 11 (20.4) | |
Ki-67 | 53.08±34.21 | 49.25±33.19 | 66.83±24.25 | 0.365 |
Grade | 0.218 | |||
NETG1 | 15 (19.7) | 7 (21.9) | 0 (0.0) | |
NETG2 | 6 (7.9) | 3 (9.4) | 5 (9.3) | |
NETG3 | 3 (4.0) | 0 (0.0) | 2 (3.7) | |
MiNEN | 38 (50.0) | 15 (46.8) | 30 (55.5) | |
NEC | 14 (18.4) | 7 (21.9) | 17 (31.5) |
Values are shown as n (%) or mean ± SD. The analysis of variance test was used to compare the characteristics. CT, computed tomography; LN, lymph node status; NETG, neuroendocrine tumor grade; MiNEN, mixed neuroendocrine-nonneuroendocrine neoplasm; NEC, neuroendocrine carcinoma; SD, standard deviation.
For each clinical factor, the Schoenfeld residuals test with chi-squared test was calculated (Figure S1). Factors with a P value larger than 0.05 were considered eligible for Cox regression. The test results indicated that all clinical factors were eligible for employ Cox regression in univariable and multivariable analyses (Figure S1). M stage, Ki-67 index, and longest diameter were independent predictors for risk prediction (P value <0.05; Table 2) in the construction of Modelclinic. Modelclinic yielded worse results than did the DL-based radiomics signature Modeldl in the training (C-index: 0.80; 95% CI: 0.73–0.86), internal validation (C-index: 0.71; 95% CI: 0.60–0.84), and external validation (C-index: 0.66; 95% CI: 0.52–0.72) cohorts (Table 3).
Table 2
Characteristic | Univariable analysis | Multivariable analysis | |||
---|---|---|---|---|---|
HR (95% CI) | P value | HR (95% CI) | P value | ||
Age (years) | 1.05 (1.05–1.13) | 0.001* | 1.05 (0.97–1.07) | 0.065 | |
Sex | |||||
Female | 1 (reference) | 1 (reference) | |||
Male | 0.33 (0.16–0.68) | 0.002* | 1.24 (0.52–2.93) | 0.629 | |
Location | |||||
Cardia/fundus | 1 (reference) | ||||
Body | 0.29 (0.11–0.75) | 0.002* | 1.14 (0.45–2.81) | 0.787 | |
Antrum | 0.42 (0.13–1.37) | 0.152 | 1.29 (0.39–4.23) | 0.860 | |
≥2/3 stomach | 1.29 (0.39–4.22) | 0.678 | 0.40 (0.09–1.88) | 0.256 | |
Long diameter | 1.03 (1.02–1.04) | <0.001* | 1.02 (0.99–1.04) | 0.030* | |
CT-reported LN | |||||
No | 1 (reference) | 1 (reference) | |||
Yes | 5.65 (2.66–11.97) | <0.001* | 1.66 (0.77–3.59) | 0.198 | |
M stage | |||||
M0 | 1 (reference) | 1 (reference) | |||
M1 | 4.59 (2.21–7.33) | <0.001* | 2.26 (1.00–4.36) | 0.037* | |
Ki-67 | 1.03 (1.01–1.05) | 0.001* | 1.04 (0.98–1.08) | 0.049* | |
Grade | |||||
NETG1 | 1 (reference) | 1 (reference) | |||
NETG2 | 0.001 (0.0–Inf) | 0.99 | 0.001 (0–Inf) | 0.997 | |
NETG3 | 13.05 (1.52–147.51) | 0.012* | 5.16 (0.32–71.15) | 0.2892 | |
MiNEN | 11.50 (2.77–47.63) | 0.003* | 0.33 (0.01–12.95) | 0.5207 | |
NEC | 7.59 (1.56–36.97) | 0.012* | 0.15 (0.01–4.08) | 0.1638 |
On a 95% CI, the result is considered significant when the P value is less than 0.05; *, P<0.05. For the P value, the “Coxph” package of R software was used with the Wald test and likelihood ratio test, where appropriate. HR, hazard ratio; CI, confidence interval; CT, computed tomography; LN, lymph node status; NETG, neuroendocrine tumors grade; MiNEN, mixed neuroendocrine-nonneuroendocrine neoplasm; NEC, neuroendocrine carcinoma.
Table 3
Model | C-index (95% CI) | Hazard ratio (95% CI) | |||||
---|---|---|---|---|---|---|---|
Training | Internal validation | External validation | Training | Internal validation | External validation | ||
Modelclinic | 0.80 (0.73–0.86) | 0.71 (0.60–0.84) | 0.66 (0.52–0.72) | 2.68 (1.93–3.83) | 2.27 (1.32–3.90) | 1.52 (1.19–1.95) | |
Modeldl | 0.84(0.79–0.92) | 0.73 (0.61–0.86) | 0.65 (0.56–0.75) | 2.71 (2.02–3.66) | 2.16 (1.44–3.22) | 1.25 (0.89–1.77) | |
Modelclinic + dl | 0.86 (0.81–0.92) | 0.77 (0.66–0.89) | 0.71 (0.62–0.83) | 3.12 (2.34–3.93) | 2.51 (1.57–3.99) | 1.77 (1.21–2.59) |
C-index, concordance index; CI, confidence interval; Modelclinic, a clinical model; Modeldl, the deep learning-based radiomics signature; Modelclinic + dl, the combined model.
In the building of the combined model (Modelclinic + dl), Modelclinic + dl adopted DL-based radiomics signatures, Ki-67 index, M stage, and longest diameter. Subsequently, the combined model was visualized as a radiomics nomogram for OS estimation using regression coefficients of Modelclinic + dl, as shown in Figure 3. Compared with Modelclinic and Modeldl, Modelclinic + dl demonstrated the strongest prognostic ability of OS in the training cohort [C-index: 0.86, 95% confidence interval (CI): 0.81–0.92; HR 3.12, 95% CI 2.34–3.93; P<0.01], internal validation cohort (C-index: 0.77, 95% CI: 0.66–0. vv89; HR 2.51, 95% CI: 1.57–3.99; P<0.01), and external validation cohort (C-index: 0.71, 95% CI: 0.62–0.83; HR 1.77, 95% CI: 1.21–2.59; P<0.01) (Table 3). Moreover, Modelclinic + dl was capable of dividing all patients into 2 distinct risk subgroups (the low-risk and high-risk group). Figure 4 presents the KM curves for Modelclinic + dl, while Table 4 displays the clinical factor analysis of the different risk groups in the three cohorts. In the training cohort, the median survival time was 42.5 months for the low-risk group and 16.3 months for the high-risk group; in the internal validation cohort, the median survival time was 36.7 months for the low-risk group and 9.9 months for the high-risk group; and in external validation cohort, the median survival time was 20.6 months for the low-risk group and 15.5 months for the high-risk group.
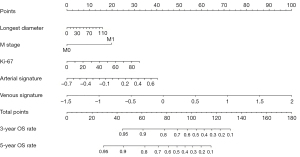
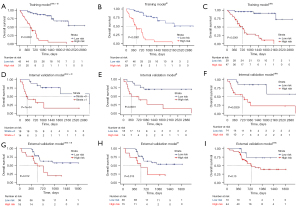
Table 4
Characteristics | Training cohort (n=76) | Internal validation cohort (n=32) | External validation cohort (n=54) | ||||||||
---|---|---|---|---|---|---|---|---|---|---|---|
Low risk | High risk | P value | Low risk | High risk | P value | Low risk | High risk | P value | |||
Age (years) | 59.43±12.11 | 64.50±9.69 | 0.051 | 59.17±12.46 | 68.07±7.64 | 0.031 | 62.44±9.91 | 68.00±6.51 | 0.060 | ||
Sex | 0.004* | 0.264 | 0.970 | ||||||||
Female | 19 (45.2) | 4 (11.8) | 6 (46.2) | 2 (10.5) | 7 (20.6) | 5 (25.0) | |||||
Male | 23 (54.8) | 30 (88.2) | 7 (53.8) | 17 (89.5) | 27 (79.4) | 15 (75.0) | |||||
Location | 0.074 | 0.223 | 0.007 | ||||||||
Cardia/fundus | 20 (47.6) | 21 (61.7) | 7 (38.9) | 10 (71.4) | 22 (64.7) | 5 (25.0) | |||||
Body | 16 (38.1) | 5 (14.7) | 10 (55.6) | 1 (7.2) | 9 (26.5) | 6 (30.0) | |||||
Antrum | 5 (11.9) | 4 (11.8) | 1 (5.5) | 0 (0.0) | 1 (2.9) | 1 (5.0) | |||||
≥2/3 stomach | 1 (2.4) | 4 (11.8) | 0 (0.0) | 3 (21.4) | 2 (5.9) | 8 (40.0) | |||||
Long diameter (mm) | 32.08±23.74 | 60.52±23.58 | <0.001* | 24.34±17.48 | 70.58±20.26 | <0.001* | 43.31±13.40 | 72.10±35.43 | <0.001* | ||
CT-reported LN | 0.001* | 0.448 | 0.078 | ||||||||
No | 29 (69.0) | 10 (29.4) | 10 (55.6) | 5 (35.7) | 20 (58.8) | 6 (30.0) | |||||
Yes | 13 (31.0) | 24 (70.6) | 8 (44.4) | 9 (64.3) | 14 (41.2) | 14 (70.0) | |||||
M stage | <0.001* | 0.003* | 0.050* | ||||||||
M0 | 40 (95.2) | 19 (55.9) | 18 (100.0) | 7 (50.0) | 30 (88.2) | 13 (65.0) | |||||
M1 | 2 (4.8) | 15 (44.1) | 0 (0.0) | 7 (50.0) | 4 (11.8) | 7 (35.0) | |||||
Ki-67 | 41.48±37.13 | 67.41±23.74 | <0.001* | 33.67±35.70 | 69.29±13.85 | 0.001* | 64.61±28.21 | 70.50±15.72 | 0.396 | ||
Grade | <0.001* | 0.221 | 0.195 | ||||||||
NETG1 | 13 (31.0) | 2 (5.9) | 7 (38.9) | 0 (0.0) | 0 (0.0) | 0 (0.0) | |||||
NETG2 | 6 (14.2) | 0 (0.0) | 3 (16.7) | 0 (0.0) | 5 (14.7) | 0 (0.0) | |||||
NETG3 | 1 (2.4) | 2 (5.9) | 0 (0.0) | 0 (0.0) | 1 (2.9) | 1 (5.0) | |||||
MiNEN | 13 (31.0) | 25 (73.5) | 6 (33.3) | 9 (64.3) | 16 (47.1) | 14 (70.0) | |||||
NEC | 9 (21.4) | 5 (14.7) | 2 (11.1) | 5 (35.7) | 12 (35.3) | 5 (25.0) | |||||
Follow-up (months) | 42.5 | 16.3 | 36.7 | 9.9 | 20.6 | 15.5 |
Values are shown as n (%) or mean ± SD. *, P<0.05. CT, computed tomography; LN, lymph node; NETG, neuroendocrine tumor grade; MiNEN, mixed neuroendocrine-nonneuroendocrine neoplasm; NEC, neuroendocrine carcinoma; SD, standard deviation.
To evaluate the performance of the three models, their KM curves were compared (Figure 4). The cutoff values of Modelclinic + dl (0.567), Modeldl (0.512), and Modelclinic (0.367) were obtained in the training cohort. The distribution of risk scores for prediction with Modelclinic + dl in the three cohorts is shown in Figure 5. In the distribution shape of the risk scores in the training, internal validation, and external validation cohorts, all patients were divided into three subgroups. Furthermore, as seen in Table 3, the Modelclinic + dl had the best predictive performance with a high C-index in the training, internal validation, and external validation cohorts (0.86 vs. 0.77 vs. 0.71).
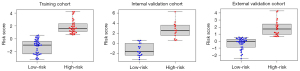
As shown in Table 3, Modelclinic + dl had the highest HR in the training cohort: (Modelclinic + dlvs. Modeldlvs. Modelclinic: 3.12 vs. 2.71 vs. 2.68), indicating that the high-risk groups predicted by Modelclinic + dl were at a higher risk of death than those of the other models. In the internal and external cohort, the findings were similar. Moreover, in comparing the TDROC of the three models, we observed that the performances of the Modelclinic + dl were equally effective across all three cohorts (Figure S2). Calibrations were performed, and DCA curves of Modelclinic + dl for individualized OS prediction were drawn (Figures 5,6). The DCA revealed that Modelclinic + dl provided a more significant net benefit to patients compared to the other models.

Discussion
In this study, we established and verified the prognostic value of CT-based radiomics for individualized assessment of OS in patients with gNENs using a DL method in three cohorts. The DL-based radiomics signatures from dual-phase CT images showed notable prognostic value for estimating OS. This research demonstrated that incorporating DL-based radiomics signatures with independent clinical factors can provide significantly enhanced predictive power as compared to a clinical model and effectively classify patients into high- and low-risk groups, thus indicating its feasibility as a noninvasive tool for predicting the prognosis of patients with gNENs. To our knowledge, the current study is the first to explore the prognostic value of DL radiomics analysis for individualized evaluation of OS in patients with gNENs.
The clinical factors and survival outcomes of patients with gNENs have been investigated in a few previous studies. One research team assessed the prognosis of 64,971 patients with NENs from the Surveillance, Epidemiology, and End Results (SEER) database, and the findings revealed that G1 grade undifferentiated tumors were associated with the highest median OS (16.2 years), while G2 grade differentiated tumors were associated with poor OS (8.3 years), and G3 grade poorly differentiated tumors were associated with the worst OS (10 months) (1). In one study, the prognosis of 51 cases with gastric NEC was investigated, and it was found that for patients who received palliative resection, the median OS was 21 months and the 5-year survival rate was 10.0% (29). In another study, the median OS of patients with stage I–III and those with stage IV gNENs was 32 and 11 months, respectively (30). Taken together, these studies indicate that early detection of patients with poorly differentiated gNENs can significantly improve their survival.
Some researchers have recently adopted radiomics analysis to predict the prognosis of patients with gastrointestinal and pancreatic NENs or gastric NECs, obtaining satisfactory predictive results (31,32). Radiomics as a new image quantification method can extract high-throughput medical imaging features and has been broadly adopted in the diagnosis and prognosis of patients with cancer (33-35). Prior investigations have indicated that the proteomic and phenotypic information extracted from radiological images of tumors can provide significant predictive value, particularly in terms of tumor recurrence and metastasis, which may thus be considered crucial prognostic biomarkers (36,37). In our previous research, we found that radiomics nomograms had significant clinical implications for the preoperative detection of gastric malignancies, and radiomics analysis demonstrated satisfactory performance in distinguishing GNEC from gastric adenocarcinoma (38). In our study, a DL radiomics signature from dual-phase CT images was constructed. The DL-based radiomics signatures were highly associated with OS in the training, internal validation, and external validation cohorts (P<0.01). The combined model incorporating the independent clinical risk factors and radiomics signatures demonstrated notable enhancements in predicting OS as compared to the clinical model.
The Ki-67 index serves as a crucial metric for assessing tumor proliferation activity (39-43). According to the recommendations of previous literature, there is a close relationship between Ki-67 and tumor grade, and patients can be categorized into four groups: grade 1 NET, grade 2 NET, grade 3 NET, and NEC, with Ki-67 index criteria of <3%, 3–20%, 20–55%, and >55%, respectively (2). Our study examined the association of the Ki-67 index and distant metastasis with patient prognosis. After conducting univariate and multivariate Cox regression analysis, we found that the Ki-67 index and distant metastasis were independent risk factors affecting prognosis, which is consistent with previous studies (31). Tumor grade and Ki-67 index may have overlapping statistical efficacy in predicting survival and thus interfere with one another. However, the Ki-67 index showed greater predictive ability in the multivariate Cox regression analysis. Therefore, we included the Ki-67 index in the model construction, while the tumor grade was discarded.
Advanced DL models have become the most important method for radiomics analysis based on large-scale medical imaging data (44,45). Based on the fixed size bounding box of the tumor region, DL features can be easily extracted, and the subjectivity of manual segmentation in the radiomics procedure can be reduced (46). Therefore, we developed a CT-based DL radiomics nomogram for individualized evaluation of OS in patients with gNENs. The experimental results showed that the DL radiomics analysis had good performance in preoperatively predicting the OS of patients with gNENs.
Our study still has several limitations. First, manually segmenting tumor ROIs on CT images is a laborious and expensive task. A semiautomatic or automatic segmentation approach may be preferable. Second, we employed a retrospective design, and the CT images were obtained from different CT scanners, which might have introduced some deviation and interference. Third, the DL model we adopted consisted of a limited dataset, and a larger dataset should be used to ensure the effectiveness of model. Furthermore, we used 2-dimensional features from a single slice instead of 3-dimensional features, and 2-dimensional segmentation may not represent the whole tumor, as well as 3-dimensional segmentation. Although 3-dimensional lesion segmentation is a time-consuming process, further exploration of this technique is warranted.
Conclusions
The value of DL-based radiomics analysis on CT images for prognostic prediction in patients with gNENs was confirmed. Our DL-based radiomics model could successfully stratify individual patients into two groups with different prognoses and could thus be used as a noninvasive tool for the prognostic prediction and risk stratification of patients with gNENs.
Acknowledgments
We would like to express our gratitude to the investigators at all participating study sites.
Funding: This work was supported by
Footnote
Reporting Checklist: The authors have completed the TRIPOD reporting checklist. Available at https://qims.amegroups.com/article/view/10.21037/qims-23-577/rc
Conflicts of Interest: All authors have completed the ICMJE uniform disclosure form (available at https://qims.amegroups.com/article/view/10.21037/qims-23-577/coif). The authors have no conflicts of interest to declare.
Ethical Statement: The authors are accountable for all aspects of the work in ensuring that questions related to the accuracy or integrity of any part of the work are appropriately investigated and resolved. This study was approved by the Institutional Review Board of the First Affiliated Hospital of Zhengzhou University (No. 2021-KY-1070-002). Both participating hospitals were informed of and agreed with the study. The study was conducted in accordance with the Declaration of Helsinki (as revised in 2013). The requirement for informed consent was waived due to the retrospective nature of the study.
Open Access Statement: This is an Open Access article distributed in accordance with the Creative Commons Attribution-NonCommercial-NoDerivs 4.0 International License (CC BY-NC-ND 4.0), which permits the non-commercial replication and distribution of the article with the strict proviso that no changes or edits are made and the original work is properly cited (including links to both the formal publication through the relevant DOI and the license). See: https://creativecommons.org/licenses/by-nc-nd/4.0/.
References
- Dasari A, Shen C, Halperin D, Zhao B, Zhou S, Xu Y, Shih T, Yao JC. Trends in the Incidence, Prevalence, and Survival Outcomes in Patients With Neuroendocrine Tumors in the United States. JAMA Oncol 2017;3:1335-42. [Crossref] [PubMed]
- Nagtegaal ID, Odze RD, Klimstra D, Paradis V, Rugge M, Schirmacher P, Washington KM, Carneiro F, Cree IAWHO Classification of Tumours Editorial Board. The 2019 WHO classification of tumours of the digestive system. Histopathology 2020;76:182-8. [Crossref] [PubMed]
- Hu P, Bai J, Liu M, Xue J, Chen T, Li R, Kuai X, Zhao H, Li X, Tian Y, Sun W, Xiong Y, Tang Q. Trends of incidence and prognosis of gastric neuroendocrine neoplasms: a study based on SEER and our multicenter research. Gastric Cancer 2020;23:591-9. [Crossref] [PubMed]
- Roseland ME, Francis IR, Shampain KL, Stein EB, Wasnik AP, Millet JD. Gastric neuroendocrine neoplasms: a primer for radiologists. Abdom Radiol (NY) 2022;47:3993-4004. [Crossref] [PubMed]
- Choi NY, Kim BS, Oh ST, Yook JH, Kim BS. Comparative Outcomes in Patients With Small- and Large-Cell Neuroendocrine Carcinoma (NEC) and Mixed Neuroendocrine-Non-Neuroendocrine Neoplasm (MiNEN) of the Stomach. Am Surg 2021;87:631-7. [Crossref] [PubMed]
- Bukhari MH, Coppola D, Nasir A. Clinicopathologic analysis of primary gastroenteropancreatic poorly differentiated neuroendocrine carcinoma; A ten year retrospective study of 68 cases at Moffit Cancer Center. Pak J Med Sci 2020;36:265-70. [Crossref] [PubMed]
- Kasajima A, Konukiewitz B, Schlitter AM, Weichert W, Klöppel G. An analysis of 130 neuroendocrine tumors G3 regarding prevalence, origin, metastasis, and diagnostic features. Virchows Arch 2022;480:359-68. [Crossref] [PubMed]
- Pelosi G, Bianchi F, Dama E, Metovic J, Barella M, Sonzogni A, Albini A, Papotti M, Gong Y, Vijayvergia N. A Subset of Large Cell Neuroendocrine Carcinomas in the Gastroenteropancreatic Tract May Evolve from Pre-existing Well-Differentiated Neuroendocrine Tumors. Endocr Pathol 2021;32:396-407. [Crossref] [PubMed]
- Heetfeld M, Chougnet CN, Olsen IH, Rinke A, Borbath I, Crespo G, Barriuso J, Pavel M, O'Toole D, Walter T. other Knowledge Network members. Characteristics and treatment of patients with G3 gastroenteropancreatic neuroendocrine neoplasms. Endocr Relat Cancer 2015;22:657-64. [Crossref] [PubMed]
- Lin J, Zhao Y, Zhou Y, Tian Y, He Q, Lin J, et al. Comparison of Survival and Patterns of Recurrence in Gastric Neuroendocrine Carcinoma, Mixed Adenoneuroendocrine Carcinoma, and Adenocarcinoma. JAMA Netw Open 2021;4:e2114180. [Crossref] [PubMed]
- Koffas A, Giakoustidis A, Papaefthymiou A, Bangeas P, Giakoustidis D, Papadopoulos VN, Toumpanakis C. Diagnostic work-up and advancement in the diagnosis of gastroenteropancreatic neuroendocrine neoplasms. Front Surg 2023;10:1064145. [Crossref] [PubMed]
- Yan S, Liu T, Li Y, Zhu Y, Jiang J, Jiang L, Zhao H. Value of computed tomography evaluation in pathologic classification and prognosis prediction of gastric neuroendocrine tumors. Ann Transl Med 2019;7:545. [Crossref] [PubMed]
- Sundin A, Arnold R, Baudin E, Cwikla JB, Eriksson B, Fanti S, Fazio N, Giammarile F, Hicks RJ, Kjaer A, Krenning E, Kwekkeboom D, Lombard-Bohas C, O'Connor JM, O'Toole D, Rockall A, Wiedenmann B, Valle JW, Vullierme MP. ENETS Consensus Guidelines for the Standards of Care in Neuroendocrine Tumors: Radiological, Nuclear Medicine & Hybrid Imaging. Neuroendocrinology 2017;105:212-44. [Crossref] [PubMed]
- Chen T, Wu J, Cui C, He Q, Li X, Liang W, Liu X, Liu T, Zhou X, Zhang X, Lei X, Xiong W, Yu J, Li G. CT-based radiomics nomograms for preoperative prediction of diffuse-type and signet ring cell gastric cancer: a multicenter development and validation cohort. J Transl Med 2022;20:38. [Crossref] [PubMed]
- Lambin P, Leijenaar RTH, Deist TM, Peerlings J, de Jong EEC, van Timmeren J, Sanduleanu S, Larue RTHM, Even AJG, Jochems A, van Wijk Y, Woodruff H, van Soest J, Lustberg T, Roelofs E, van Elmpt W, Dekker A, Mottaghy FM, Wildberger JE, Walsh S. Radiomics: the bridge between medical imaging and personalized medicine. Nat Rev Clin Oncol 2017;14:749-62. [Crossref] [PubMed]
- Berenguer R, Pastor-Juan MDR, Canales-Vázquez J, Castro-García M, Villas MV, Mansilla Legorburo F, Sabater S. Radiomics of CT Features May Be Nonreproducible and Redundant: Influence of CT Acquisition Parameters. Radiology 2018;288:407-15. [Crossref] [PubMed]
- Wang Y, Liu W, Yu Y, Liu JJ, Xue HD, Qi YF, Lei J, Yu JC, Jin ZY. CT radiomics nomogram for the preoperative prediction of lymph node metastasis in gastric cancer. Eur Radiol 2020;30:976-86. [Crossref] [PubMed]
- Wang Y, Liu W, Yu Y, Han W, Liu JJ, Xue HD, Lei J, Jin ZY, Yu JC. Potential value of CT radiomics in the distinction of intestinal-type gastric adenocarcinomas. Eur Radiol 2020;30:2934-44. [Crossref] [PubMed]
- Chen Q, Zhang L, Liu S, You J, Chen L, Jin Z, Zhang S, Zhang B. Radiomics in precision medicine for gastric cancer: opportunities and challenges. Eur Radiol 2022;32:5852-68. [Crossref] [PubMed]
- Krizhevsky A, Sutskever I, Hinton GE. Imagenet classification with deep convolutional neural networks. Adv Neural Inf Process Syst 2012;1097-105.
- Ardila D, Kiraly AP, Bharadwaj S, Choi B, Reicher JJ, Peng L, Tse D, Etemadi M, Ye W, Corrado G, Naidich DP, Shetty S. End-to-end lung cancer screening with three-dimensional deep learning on low-dose chest computed tomography. Nat Med 2019;25:954-61. [Crossref] [PubMed]
- Peng H, Dong D, Fang MJ, Li L, Tang LL, Chen L, Li WF, Mao YP, Fan W, Liu LZ, Tian L, Lin AH, Sun Y, Tian J, Ma J. Prognostic Value of Deep Learning PET/CT-Based Radiomics: Potential Role for Future Individual Induction Chemotherapy in Advanced Nasopharyngeal Carcinoma. Clin Cancer Res 2019;25:4271-9. [Crossref] [PubMed]
- Amin MB, Edge S, Greene F, David RB, Robert KB, Mary KW, Jeffrey EG, Carolyn CC, Kenneth RH, Daniel CS, J. MJ, James DB, Lauri EG, Richard LS, Charles MB, David PW, Elliot AA, Martin M, Donna MG, Laura RM. AJCC Cancer Staging Manual, 8th ed. New York: Springer-Verlag; 2017. doi:
10.1007/978-3-319-40618-3 . - Zhang AQ, Zhao HP, Li F, Liang P, Gao JB, Cheng M. Computed tomography-based deep-learning prediction of lymph node metastasis risk in locally advanced gastric cancer. Front Oncol 2022;12:969707. [Crossref] [PubMed]
- He K, Zhang X, Ren S, Sun J. Deep residual learning for image recognition. In: IEEE Conference on Computer Vision and Pattern Recognition 2016. CVPR 2016. Las Vegas, NV, USA: IEEE; 2016.
- Heagerty PJ, Lumley T, Pepe MS. Time-dependent ROC curves for censored survival data and a diagnostic marker. Biometrics 2000;56:337-44. [Crossref] [PubMed]
- Hernán MA. The hazards of hazard ratios. Epidemiology 2010;21:13-5. [Crossref] [PubMed]
- Zhang L, Dong D, Zhang W, Hao X, Fang M, Wang S, Li W, Liu Z, Wang R, Zhou J, Tian J. A deep learning risk prediction model for overall survival in patients with gastric cancer: A multicenter study. Radiother Oncol 2020;150:73-80. [Crossref] [PubMed]
- Ishida M, Sekine S, Fukagawa T, Ohashi M, Morita S, Taniguchi H, Katai H, Tsuda H, Kushima R. Neuroendocrine carcinoma of the stomach: morphologic and immunohistochemical characteristics and prognosis. Am J Surg Pathol 2013;37:949-59. [Crossref] [PubMed]
- Pommergaard HC, Nielsen K, Sorbye H, Federspiel B, Tabaksblat EM, Vestermark LW, Janson ET, Hansen CP, Ladekarl M, Garresori H, Hjortland GO, Sundlöv A, Galleberg R, Knigge P, Kjaer A, Langer SW, Knigge U. Surgery of the primary tumour in 201 patients with high-grade gastroenteropancreatic neuroendocrine and mixed neuroendocrine-non-neuroendocrine neoplasms. J Neuroendocrinol 2021;33:e12967. [Crossref] [PubMed]
- Fang C, Wang W, Feng X, Sun J, Zhang Y, Zeng Y, Wang J, Chen H, Cai M, Lin J, Chen M, Chen Y, Li Y, Li S, Chen J, Zhou Z. Nomogram individually predicts the overall survival of patients with gastroenteropancreatic neuroendocrine neoplasms. Br J Cancer 2017;117:1544-50. [Crossref] [PubMed]
- Zheng H, Zhao Y, He Q, Hao H, Tian Y, Zou B, et al. Multi-institutional development and validation of a nomogram to predict recurrence after curative resection of gastric neuroendocrine/mixed adenoneuroendocrine carcinoma. Gastric Cancer 2021;24:503-14. [Crossref] [PubMed]
- Dong D, Fang MJ, Tang L, Shan XH, Gao JB, Giganti F, Wang RP, Chen X, Wang XX, Palumbo D, Fu J, Li WC, Li J, Zhong LZ, De Cobelli F, Ji JF, Liu ZY, Tian J. Deep learning radiomic nomogram can predict the number of lymph node metastasis in locally advanced gastric cancer: an international multicenter study. Ann Oncol 2020;31:912-20. [Crossref] [PubMed]
- Wang S, Dong D, Zhang W, Hu H, Li H, Zhu Y, Zhou J, Shan X, Tian J. Specific Borrmann classification in advanced gastric cancer by an ensemble multilayer perceptron network: a multicenter research. Med Phys 2021;48:5017-28. [Crossref] [PubMed]
- Liu S, He J, Liu S, Ji C, Guan W, Chen L, Guan Y, Yang X, Zhou Z. Radiomics analysis using contrast-enhanced CT for preoperative prediction of occult peritoneal metastasis in advanced gastric cancer. Eur Radiol 2020;30:239-46. [Crossref] [PubMed]
- Zhang J, Fujimoto J, Zhang J, Wedge DC, Song X, Zhang J, et al. Intratumor heterogeneity in localized lung adenocarcinomas delineated by multiregion sequencing. Science 2014;346:256-9. [Crossref] [PubMed]
- Rutman AM, Kuo MD. Radiogenomics: creating a link between molecular diagnostics and diagnostic imaging. Eur J Radiol 2009;70:232-41. [Crossref] [PubMed]
- Wang R, Liu H, Liang P, Zhao H, Li L, Gao J. Radiomics analysis of CT imaging for differentiating gastric neuroendocrine carcinomas from gastric adenocarcinomas. Eur J Radiol 2021;138:109662. [Crossref] [PubMed]
- Klöppel G, La Rosa S. Ki67 labeling index: assessment and prognostic role in gastroenteropancreatic neuroendocrine neoplasms. Virchows Arch 2018;472:341-9. [Crossref] [PubMed]
- Sorbye H, Welin S, Langer SW, Vestermark LW, Holt N, Osterlund P, Dueland S, Hofsli E, Guren MG, Ohrling K, Birkemeyer E, Thiis-Evensen E, Biagini M, Gronbaek H, Soveri LM, Olsen IH, Federspiel B, Assmus J, Janson ET, Knigge U. Predictive and prognostic factors for treatment and survival in 305 patients with advanced gastrointestinal neuroendocrine carcinoma (WHO G3): the NORDIC NEC study. Ann Oncol 2013;24:152-60. [Crossref] [PubMed]
- Boo YJ, Park SS, Kim JH, Mok YJ, Kim SJ, Kim CS. Gastric neuroendocrine carcinoma: clinicopathologic review and immunohistochemical study of E-cadherin and Ki-67 as prognostic markers. J Surg Oncol 2007;95:110-7. [Crossref] [PubMed]
- Xie JW, Sun YQ, Feng CY, Zheng CH, Li P, Wang JB, Lin JX, Lu J, Chen QY, Cao LL, Lin M, Tu RH, Yang YH, Huang CM. Evaluation of clinicopathological factors related to the prognosis of gastric neuroendocrine carcinoma. Eur J Surg Oncol 2016;42:1464-70. [Crossref] [PubMed]
- Han D, Li YL, Zhou ZW, Yin F, Chen J, Liu F, Shi YF, Wang W, Zhang Y, Yu XJ, Xu JM, Yang RX, Tian C, Luo J, Tan HY. Clinicopathological characteristics and prognosis of 232 patients with poorly differentiated gastric neuroendocrine neoplasms. World J Gastroenterol 2021;27:2895-909. [Crossref] [PubMed]
- Hu Y, Xie C, Yang H, Ho JWK, Wen J, Han L, Lam KO, Wong IYH, Law SYK, Chiu KWH, Vardhanabhuti V, Fu J. Computed tomography-based deep-learning prediction of neoadjuvant chemoradiotherapy treatment response in esophageal squamous cell carcinoma. Radiother Oncol 2021;154:6-13. [Crossref] [PubMed]
- Li J, Dong D, Fang M, Wang R, Tian J, Li H, Gao J. Dual-energy CT-based deep learning radiomics can improve lymph node metastasis risk prediction for gastric cancer. Eur Radiol 2020;30:2324-33. [Crossref] [PubMed]
- Zhu Y, Man C, Gong L, Dong D, Yu X, Wang S, Fang M, Wang S, Fang X, Chen X, Tian J. A deep learning radiomics model for preoperative grading in meningioma. Eur J Radiol 2019;116:128-34. [Crossref] [PubMed]