Extracellular volume fraction as a potential predictor to differentiate lung cancer from benign lung lesions with dual-layer detector spectral CT
Introduction
Worldwide, lung cancer (LC) remains the leading cause of cancer death (1). The mortality of LC can be greatly reduced by early detection and diagnosis (2). At present, the National Lung Screening Trial (NLST) has shown that low-dose computed tomography (LDCT) screening can detect 13% more LC and result in a 20% reduction in LC-specific 5-year mortality compared to radiography (3), whereas another LC screening trial [the Nederlands-Leuvens Screening Onderzoek trial (NELSON)] has shown that LDCT screening can reduce mortality by more than 25% (4). Therefore, early detection and diagnosis of LC have greatly benefited from LDCT screening. Numerous imaging studies on the differential diagnosis of LC have been performed, including the conventional morphological features, imaging quantitative parameters, and the application of radiomics and artificial intelligence. However, there are still some overlaps between the diagnostic criteria of pulmonary benign lesions and LC. Therefore, the search for more accurate differential diagnosis methods remains critical, especially with the development of the latest imaging techniques.
The development of dual-layer detector computed tomography (DLCT) has been a significant advancement for computed tomography (CT) (5,6). In addition to reflecting the morphological characteristics of lesions, it can provide multiparametric information, such as effective atomic number (Zeff), iodine concentration (IC), and extracellular volume (ECV) fraction, among others (7). IC has been demonstrated to have potential clinical value in lung disease. Hou et al. (8) reported that normalized iodine concentration (NIC) could be a novel method for distinguishing lung malignancies from inflammatory masses. Also, as reported by Zhang et al. (9), the iodine content acquired in dual energy spectral CT may be a useful and valuable parameter for differentiating solitary pulmonary nodules.
ECV provides a reflection on the changes in the cellular microenvironment, which is determined by equilibrium contrast-enhanced CT images and calculated with hematocrit (10). At present, the ECV score is mainly used in cardiovascular diseases, pancreatic (11) and hepatic fibrosis (12), and some abdominal cancers, focusing on fibrosis evaluation. Fibrotic processes exist in both LC and benign lung lesions (BLLs). The inflammatory reaction will induce the fibrosis process during its development (13). It is not known whether ECV, as a new quantitative parameter, adds value in the differential diagnosis of LC. To the best of our knowledge, scanty research is performed to evaluate the performance of ECV in lung disease. As aforementioned, the ECV evaluation should use the equilibrium or delayed contrast-enhanced CT images of abdominal and cardiovascular lesions. After the injection of contrast agent, chest contrast-enhanced CT scans routinely include the arterial and delayed phases. To explore the ECV in pulmonary lesions, the delayed phase has been favored. Therefore, the purpose of this study was to explore the feasibility and performance of ECV derived from chest contrast-enhanced delayed phase CT on the aspect of differential diagnosis of LC. We present this article in accordance with the STARD reporting checklist (available at https://qims.amegroups.com/article/view/10.21037/qims-23-736/rc).
Methods
This study was conducted in accordance with the Declaration of Helsinki (as revised in 2013). Retrospectively, spectral chest CT images and clinical data were collected in the Second Affiliated Hospital, Navy Medical University (Shanghai, China) and Nanjing Drum Tower Hospital (Nanjing, China) from June 2022 to November 2022. This retrospective study was approved by the Ethics Committees of Navy Medical University and Nanjing University Medical School, and individual consent for this retrospective analysis was waived. The following criteria were used for inclusion: (I) chest CT showing solitary nodule (≥1 cm) or mass; (II) diagnosis was confirmed by histopathology or improved after taking anti-inflammatory medications; (III) accurate hematocrit within 1 week before CT examination; and (IV) contrast-enhanced spectral CT scans. The following were the criteria for exclusion: (I) interval between the operation and spectral CT scan ≥2 weeks; (II) lesion with a large amount of necrosis, cystic changes, or calcification; (III) history of other malignancies; (IV) insufficient image quality. The flowchart of the inclusion and exclusion criteria is shown in Figure 1.
DLCT scanning protocol
A dual-layer spectral detector CT (Spectral 7500 or IQon spectral CT, Philips Healthcare, Best, The Netherlands) was used. The scanning parameters were as follows: tube voltage, 120 kV; tube current, modulated by automated radiation dose control; collimation, 128×0.625 mm; rotation time, 0.5 s; pitch factor, 1.0; reconstruction slice thickness, 1 mm. During enhancement scanning, all patients received intravenous injection of contrast agent (Iopromide; Bayer Healthcare, Berlin, Germany; 1.2–1.3 mL/kg, with an injection rate of 3.0–3.5 mL/s). Then, 30 mL normal saline was injected with the same injection rate. The delay times for the arterial and delayed phases were fixed at 25 and 90 seconds (14) after the contrast agent injection, respectively. For subsequent analysis, all the original data were reconstructed with a slice thickness of 1 mm.
Image analysis
Images were analyzed on the post-processing workstation (IntelliSpace Portal, Version 12.1; Philips Healthcare, Best, The Netherlands).
Conventional CT characteristics were assessed and analyzed on images with the lung [window width, 1,500 Hounsfield units (HU); window level −600 HU] and mediastinal (window width, 400 HU; window level, 40 HU) window settings. The transverse CT with the biggest nodule slice was used to measure quantitatively with the lung window. Lesion size was determined by averaging 3 long-axis diameter measurements. The morphologic characteristics were as follows: shape (round/oval, irregular), lesion border (lobulated, non-lobulated), lesion margin (spiculated, non-spiculated), boundary (clear, blurred), pleural indentation (present, absent), vascular convergence sign (present, absent), enlarged lymph nodes in the mediastinum (present, absent).
In spectral quantitative analysis, the homogeneous enhancement area of the lesion was manually marked with a circular region of interest (ROI), avoiding vascular, calcified, and necrotic areas that might have an impact on the measurement outcome. The ROI’s surface area was directed to be larger than 50% of the lesion’s biggest axial section if the lesion was homogeneous. In cases where there was an uneven density of lesions, the ROI was located in the region that contained the most solid components. On the nonenhanced images, Zeff and electron density (ED) were evaluated, then IC, arterial enhancement fraction (AEF), and ECV were analyzed on the contrast-enhanced images. According to the ECV formula: ECV (%) = (1 − hematocrit) × (IClesion/ICaorta) ×100%, where IClesion and ICaorta are delayed phase lesion and aortic iodine densities, respectively. NIC was calculated by dividing the IC of the lung lesion by the IC of the thoracic aorta in the same slice, with the goal of reducing adverse impact due to the patient’s circulation condition and scanning times.
All analyses were independently evaluated by two thoracic radiologists with 3 years of thoracic CT clinical experience who were unaware of the pathological results. The average results were used for statistical analysis. When there was a wide discrepancy, the perspective of a senior radiologist with 20 years of thoracic CT experience was adopted.
Statistical analysis
The measurements were analyzed using the software SPSS 25.0 (IBM Corp., Armonk, NY, USA). Mean ± standard deviation (SD) and proportions were used to express continuous and categorical variables, respectively. Shapiro-Wilk tests were used to determine the normality of quantitative variables. The independent-sample t-test was used to assess normally distributed data. The Mann-Whitney U test was used to assess nonnormally distributed data. For categorical variables, the chi-square and Fisher’s exact tests were performed. A P value <0.05 was considered statistically significant. After conducting a univariate analysis, the variables that had a P value of less than 0.05 were subsequently incorporated in a multivariate logistic regression analysis in order to create appropriate regression models. The area under the curve (AUC), accuracy, sensitivity, specificity, and 95% confidence interval (CI) of the receiver operating characteristic (ROC) curve were also calculated. To determine whether the efficiency disparity between the models was statistically significant, the DeLong test was applied.
Results
In total, 128 patients (42 females and 86 males; age 59.9±12.0 years; median age, 61.0 years) were enrolled, of which 86 (67.2%) were LC (age 61.5±11.0 years; median age, 62.0 years), including 48 (37.5%) adenocarcinoma, 15 (11.7%) squamous cell carcinoma, 9 (7.0%) small cell carcinoma, 2 (1.6%) pulmonary sarcomatoid carcinoma, and the remaining 12 (9.4%) were reported only as non-small cell lung cancer. A total of 42 (32.8%) cases (age 59.2±12.1 years; median age, 59.5 years) were benign lesions with 40 (31.3%) inflammatory masses and 2 (1.6%) tuberculosis.
Comparison of conventional CT risk factors between LC and BLLs
A comparison of the demographic and conventional CT parameters between LC and BLLs is presented in Table 1. There was no significant difference between the LC and BLLs groups in terms of sex, age, shape, boundary, or vascular convergence sign (P>0.05). Lesion size, lobulation, spiculation, pleural indentation, and enlarged lymph node revealed significant within-group differences (P<0.05).
Table 1
Variable | BLLs (n=42) | LC (n=86) | P value |
---|---|---|---|
Sex | 0.376 | ||
Male | 26 (61.9) | 60 (69.8) | |
Female | 16 (38.1) | 26 (30.2) | |
Age (years) | 0.301 | ||
Mean ± SD | 59.2±12.1 | 61.5±11.0 | |
Median [range] | 59.5 [29–79] | 62.0 [36–86] | |
Conventional CT parameters | |||
Lesion size (mm) | 24.29±13.47 | 32.58±17.49 | 0.008 |
Round/oval | 13 (31.0) | 41 (47.7) | 0.855 |
Lobulation | 17 (40.5) | 65 (75.6) | <0.001 |
Spiculation | 6 (14.3) | 38 (44.2) | 0.001 |
Clear boundary | 25 (59.5) | 51 (59.3) | 0.981 |
Pleural indentation | 6 (14.3) | 33 (38.4) | 0.005 |
Vascular convergence sign | 21 (50.0) | 57 (66.3) | 0.076 |
Enlarged lymph nodes | 12 (28.6) | 44 (51.2) | 0.016 |
Data are presented as n (%), mean ± SD, or median [range]. CT, computed tomography; LC, lung cancer; BLLs, benign lung lesions; SD, standard deviation.
Comparison of quantitative parameters on DLCT between LC and BLLs
The two sets of typical spectral CT images of LC and BLLs are displayed in Figure 2 and Figure 3, respectively. Table 2 presents the estimations of spectral parameters and their pairwise comparisons between LC and BLLs. Statistical differences were found in the following indicators, Zeff (P=0.031), ED (P<0.001), ECV (P<0.001), arterial phase iodine concentration (IC-AP) (P=0.006), delayed phase iodine concentration (IC-DP) (P=0.043), arterial phase normalized iodine concentration (NIC-AP) (P=0.004), and delayed phase normalized iodine concentration (NIC-DP) (P<0.001). The difference in AEF between the two groups was not statistically significant (P=0.145).
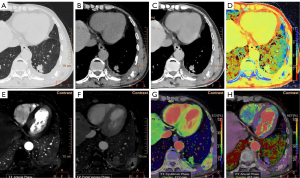
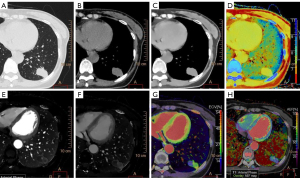
Table 2
Variable | BLLs (n=42) | LC (n=86) | P value |
---|---|---|---|
Zeff | 7.56 (7.52, 7.64) | 7.53 (7.46, 7.57) | 0.031 |
ED (%) | 100.60 (98.73, 102.33) | 103.00 (100.65, 103.63) | <0.001 |
ECV (%) | 29.82±6.34 | 23.21±7.73 | <0.001 |
IC (mg/mL) | |||
IC-AP | 1.96 (1.76, 2.23) | 1.74 (1.41, 2.01) | 0.006 |
IC-DP | 2.11±0.46 | 1.84±0.78 | 0.043 |
AEF (%) | 42.78 (36.15, 52.53) | 39.25 (28.75, 49.23) | 0.145 |
NIC | |||
NIC-AP | 0.20±0.08 | 0.16±0.07 | 0.004 |
NIC-DP | 0.49±0.11 | 0.38±0.12 | <0.001 |
Mean ± standard deviation for normally distributed variables, median (interquartile range) for non-normally distributed variables. CT, computed tomography; LC, lung cancer; BLLs, benign lung lesions; Zeff, effective atomic number; ED, electron density; ECV, extracellular volume; IC, iodine concentration; AP, arterial phase; DP, delayed phase; AEF, arterial enhancement fraction; NIC, normalized iodine concentration.
Model construction and comparison
Lesion size, lobulation, spiculation, pleural indentation, and enlarged lymph nodes were used as input variables for multiple logistic regression analysis to establish the conventional CT-based model (Model A). To establish a DLCT based model (Model B), we incorporated Zeff, ED, ECV, IC, and NIC into multiple logistic regression analysis.
After that, the statistically significant independent variables in Table 1 and Table 2 were included in the multivariate regression analysis and a combined diagnostic model was obtained (Model C). Lesion size, lobulation, and spiculation were independent predictors in Model C (P<0.05). The corresponding adjusted odds ratios (ORs) were 1.068 [95% confidence interval (CI): 1.016–1.124; P=0.010], 0.139 (95% CI: 0.040–0.482; P=0.002), and 0.206 (95% CI: 0.052–0.808; P=0.024), respectively.
The ECV based model was established by univariate logistic regression with the ECV quantitative parameter as the only independent variable. The corresponding adjusted OR was 0.885 (95% CI: 0.835–0.938; P<0.001).
The ROC curves are presented in Table 3 and Figure 4. Among single parameters in the spectral CT, ECV performed the best in distinguishing between LC and BLLs, with an AUC (95% CI), sensitivity, and specificity of 0.754 (0.671–0.826), 0.733, and 0.762, respectively. And a cut-off value of ECV ≤26.250 was the optimal for predicting LC. Model A included all parameters for which Table 1 displayed a significant difference, with AUC, sensitivity, and specificity values of 0.801 (0.721–0.866), 0.663, and 0.857, respectively. The diagnostic performance improved further when all the DLCT parameters were combined into Model B, with AUC, sensitivity, and specificity of 0.805 (0.726–0.870), 0.698, and 0.810, respectively. When conventional CT parameters were combined with spectral CT parameters, Model C exhibited the best diagnostic performance, with an AUC, sensitivity, and specificity of 0.925 (0.865–0.964), 0.698, and 1.00, respectively. Model D was composed of ECV only, so its results of ROC curve analysis were consistent with ECV (Table 3, Figure 4). In the DeLong test (Table 4), AUC comparisons between Model D and A, Model D and B, or Model A and B did not show any statistically significant difference (DeLong test, P>0.05).
Table 3
Model types | AUC (95% CI) | YI | Cutoff | Accuracy | Sensitivity | Specificity |
---|---|---|---|---|---|---|
Model A | 0.801 (0.721–0.866) | 0.520 | – | 0.727 | 0.663 | 0.857 |
Model B | 0.805 (0.726–0.870) | 0.507 | – | 0.734 | 0.698 | 0.810 |
Zeff | 0.617 (0.527–0.702) | 0.238 | 7.535 | 0.586 | 0.523 | 0.714 |
ED | 0.708 (0.621–0.785) | 0.367 | 102.450 | 0.648 | 0.581 | 0.786 |
IC-AP | 0.650 (0.561–0.732) | 0.310 | 1.700 | 0.594 | 0.477 | 0.833 |
IC-DP | 0.680 (0.592–0.760) | 0.359 | 1.505 | 0.586 | 0.407 | 0.952 |
ECV | 0.754 (0.671–0.826) | 0.494 | 26.250 | 0.742 | 0.733 | 0.762 |
NIC-AP | 0.689 (0.601–0.768) | 0.365 | 0.165 | 0.680 | 0.674 | 0.690 |
NIC-DP | 0.747 (0.662–0.819) | 0.497 | 0.389 | 0.703 | 0.616 | 0.881 |
Model C | 0.925 (0.865–0.964) | 0.698 | – | 0.797 | 0.698 | 1.00 |
Model D | 0.754 (0.671–0.826) | 0.494 | 26.250 | 0.742 | 0.733 | 0.762 |
Model A, conventional CT-based model; Model B, DLCT-based model; Model C, combined diagnostic model; Model D, ECV-based model. ROC, receiver operating characteristic; CT, computed tomography; AUC, area under the curve; CI, confidence interval; YI, Youden index; Zeff, effective atomic number; ED, electron density; IC, iodine concentration; AP, arterial phase; DP, delayed phase; ECV, extracellular volume; NIC, normalized iodine concentration; CT, computed tomography; DLCT, dual-layer detector CT.
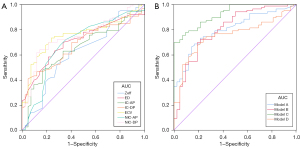
Table 4
Model comparison | P value |
---|---|
Model D and A | 0.464 |
Model D and B | 0.194 |
Model D and C | <0.001 |
Model A and B | 0.946 |
Model A and C | <0.001 |
Model B and C | 0.001 |
Model A, conventional CT-based model; Model B, DLCT-based model; Model C, combined diagnostic model; Model D, ECV-based model. AUC, area under the curve; CT, computed tomography; DLCT, dual-layer detector CT.
Discussion
In our study, we discovered significant differences between LC and BLLs in ECV in 120 kV images (P<0.001). Among the single spectral CT parameters, ECV fraction had the overall best performance. Moreover, ECV had a similar diagnostic performance compared to conventional CT based model (Model A) and DLCT based model (Model B). The ECV in dual-layer detector spectral chest CT has its potential value to differentiate the focal lung lesions. When conventional CT signs and DLCT parameters were combined, diagnostic performance was obviously improved.
BLLs, especially inflammatory masses, often present as focal, solitary, and peripheral lung nodules, or masses. Moreover, CT findings of LC are variable but not specific (15). Significant overlap exists between the two pulmonary diseases on conventional CT scans, making it difficult to distinguish LC from BLLs (16,17). Spectral CT imaging is a growing field that offers radiologists the opportunity to benefit from tissue energy dependence through virtual monochromatic images and the breakdown of matter into water, iodine, and other components (18). Recent studies have demonstrated that quantitative parameters from spectral CT may be useful in the clinical diagnosis of lung diseases (7-9,19,20). According to Zhang et al. (9), the iodine content derived from dual-energy spectral CT (DESCT) could be a useful parameter for distinguishing solitary pulmonary nodules. Also, as reported by Hou et al. (8), LCs and inflammatory masses may be distinguished using DESCT imaging with quantitative parameters such as NIC. These are consistent with our findings. Our study showed that IC and NIC of BLLs were much higher than those of LC. ECV has been considered a state-of-the-art and steady quantitative parameter, which is independent of a number of technical confounders and physiological variations (21). Nevertheless, to our best knowledge, the use of ECV to distinguish malignant from BLLs has not been reported previously.
A macromolecule known as the extracellular matrix (ECM) both regulates the physiological processes of the cell and also maintains the structure of the cell (22), which is an important component of the tumor microenvironment (23). Quantification of ECM is expressed in ECV. ECV can reflect the degree of fibrosis, tumor angiogenesis, and tumor microenvironment (24). The connection between elevated ECV during the equilibrium phase and widespread fibrosis in the liver, pancreas, and heart has been well supported by recent investigations (11,25,26). However, in these studies, the time for delayed phase imaging is inconsistent. Yoon et al. (27) reported that when evaluating liver fibrosis, the delayed phase imaging with a 3-minute delay time could provide slightly better visualization of lesions and a more accurate measurement of ECV. Due to no reference about the ECV in pulmonary lesions, and considering the delay time of abdominal and cardiac scanning is relatively long, we selected the delayed phase with 90 seconds delay after injection of contrast media. This delay time is the routine in clinical practice without prolonging the scanning time (14). A 90-second delayed time could allow contrast agent to circulate freely in the extracellular space. In our study, the ECV was significantly higher in BLLs than in LC. This discrepancy between two groups may be caused by the different degrees of fibrosis. Lung inflammation has been reported to cause chronic fibrosis, which is a common complication of inflammation (28). At least in the lung, the release of inflammatory cytokines by fibroblasts, such as CCL8, contributes to the pathophysiology of fibrosis. Inflammation is one of the primary factors that might lead to fibrosis (29). The ECV increases with progression of fibrosis (11,30). BLLs, especially inflammatory masses, are usually accompanied by a considerable degree of fibrosis, which may be responsible for the elevated ECVs in BLLs patients.
Similar to previous studies (8,9), IC and NIC showed significant differences between two groups in our study, although our research was based on 120 keV images. The IC of the lesion, conversely, is affected not only by the lesion’s microvascular environment (including blood flow, blood volume, permeability, and extracellular extravascular components), but also by technical (contrast material and CT scanning protocols) and physiological (patient body weight and hemodynamic status) variations (31). In other studies, evidence suggests that the ECV has been a reliable and steady quantitative parameter (32,33). In our study, among single parameters in the spectral CT, the ECV achieved the highest AUC score at 0.754. Under the threshold of 26.25 for the ECV, the accuracy, sensitivity, and specificity for differentiating LC from BLLs were 0.742, 0.733, and 0.762, respectively. Moreover, the ECV-based model (Model D) had a similar diagnostic performance compared to the conventional CT-based model (Model A) and DLCT-based model (Model B). All these results suggest that ECV in dual-layer detector spectral CT may be a useful and valuable parameter for differentiating focal lung lesions.
This study had several limitations. First, as a proportion of patients with BLLs can be judged as benign by imaging, this group of patients will not undergo surgery and therefore fewer patients with BLLs were included in this retrospective study than patients with LC. To validate and generalize our findings, we need additional prospective studies with large sample size of patient populations. Second, we did not classify subtypes and categories of LC or BLLs, without considering the correlation between histopathological type and DECT features. Third, we only used a single-CT vendor. Other DECT scanners should be used for testing. Fourth, the lesions we included were large in diameter (≥1 cm), and further research is needed for lung lesions with a diameter of less than 1 cm. Finally, since we selected 120 kV image datasets, further research might expand the application of ECV in the focal lung lesions, for example, to evaluate the diagnostic performance of ECV in 100 kV images.
Conclusions
ECV calculated from DLCT may be a potential new method to diagnose focal lung lesions with the clinical routine delayed scanning without prolonging scanning time. Spectral CT parameters combined with conventional CT signs can improve the differential diagnosis efficiency between LC and BLLs.
Acknowledgments
Funding: This work was supported by
Footnote
Reporting Checklist: The authors have completed the STARD reporting checklist. Available at https://qims.amegroups.com/article/view/10.21037/qims-23-736/rc
Conflicts of Interest: All authors have completed the ICMJE uniform disclosure form (available at https://qims.amegroups.com/article/view/10.21037/qims-23-736/coif). X Zhang is an employee of Philips Healthcare, the manufacturer of the CT system used in this study. The other authors have no conflicts of interest to declare.
Ethical Statement: The authors are accountable for all aspects of the work by ensuring that questions related to the accuracy or integrity of any part of the work are appropriately investigated and resolved. The study was conducted in accordance with the Declaration of Helsinki (as revised in 2013). The study was approved by the Ethics Committees of Navy Medical University and Nanjing University Medical School, and individual consent for this retrospective analysis was waived.
Open Access Statement: This is an Open Access article distributed in accordance with the Creative Commons Attribution-NonCommercial-NoDerivs 4.0 International License (CC BY-NC-ND 4.0), which permits the non-commercial replication and distribution of the article with the strict proviso that no changes or edits are made and the original work is properly cited (including links to both the formal publication through the relevant DOI and the license). See: https://creativecommons.org/licenses/by-nc-nd/4.0/.
References
- Sung H, Ferlay J, Siegel RL, Laversanne M, Soerjomataram I, Jemal A, Bray F. Global Cancer Statistics 2020: GLOBOCAN Estimates of Incidence and Mortality Worldwide for 36 Cancers in 185 Countries. CA Cancer J Clin 2021;71:209-49. [Crossref] [PubMed]
- Adams SJ, Stone E, Baldwin DR, Vliegenthart R, Lee P, Fintelmann FJ. Lung cancer screening. Lancet 2023;401:390-408. [Crossref] [PubMed]
- Aberle DR, Adams AM, Berg CD, Black WC, Clapp JD, Fagerstrom RM, Gareen IF, Gatsonis C, Marcus PM, Sicks JD. Reduced lung-cancer mortality with low-dose computed tomographic screening. N Engl J Med 2011;365:395-409. [Crossref] [PubMed]
- de Koning HJ, van der Aalst CM, de Jong PA, Scholten ET, Nackaerts K, Heuvelmans MA, et al. Reduced Lung-Cancer Mortality with Volume CT Screening in a Randomized Trial. N Engl J Med 2020;382:503-13. [Crossref] [PubMed]
- Andersen MB, Ebbesen D, Thygesen J, Kruis M, Rasmussen F. Impact of spectral body imaging in patients suspected for occult cancer: a prospective study of 503 patients. Eur Radiol 2020;30:5539-50. [Crossref] [PubMed]
- Wen Q, Yue Y, Shang J, Lu X, Gao L, Hou Y. The application of dual-layer spectral detector computed tomography in solitary pulmonary nodule identification. Quant Imaging Med Surg 2021;11:521-32. [Crossref] [PubMed]
- Zhang G, Li S, Yang K, Shang L, Zhang F, Huang Z, Ren J, Zhang Z, Zhou J, Pu H, Man Q, Kong W. The value of dual-energy spectral CT in differentiating solitary pulmonary tuberculosis and solitary lung adenocarcinoma. Front Oncol 2022;12:1000028. [Crossref] [PubMed]
- Hou WS, Wu HW, Yin Y, Cheng JJ, Zhang Q, Xu JR. Differentiation of lung cancers from inflammatory masses with dual-energy spectral CT imaging. Acad Radiol 2015;22:337-44. [Crossref] [PubMed]
- Zhang Y, Cheng J, Hua X, Yu M, Xu C, Zhang F, Xu J, Wu H. Can Spectral CT Imaging Improve the Differentiation between Malignant and Benign Solitary Pulmonary Nodules? PLoS One 2016;11:e0147537. [Crossref] [PubMed]
- Oda S, Emoto T, Nakaura T, Kidoh M, Utsunomiya D, Funama Y, Nagayama Y, Takashio S, Ueda M, Yamashita T, Tsujita K, Ando Y, Yamashita Y. Myocardial Late Iodine Enhancement and Extracellular Volume Quantification with Dual-Layer Spectral Detector Dual-Energy Cardiac CT. Radiol Cardiothorac Imaging 2019;1:e180003. [Crossref] [PubMed]
- Sofue K, Ueshima E, Masuda A, Shirakawa S, Zen Y, Ueno Y, Tsujita Y, Yamaguchi T, Yabe S, Tanaka T, Inomata N, Toyama H, Fukumoto T, Kodama Y, Murakami T. Estimation of pancreatic fibrosis and prediction of postoperative pancreatic fistula using extracellular volume fraction in multiphasic contrast-enhanced CT. Eur Radiol 2022;32:1770-80. [Crossref] [PubMed]
- Zissen MH, Wang ZJ, Yee J, Aslam R, Monto A, Yeh BM. Contrast-enhanced CT quantification of the hepatic fractional extracellular space: correlation with diffuse liver disease severity. AJR Am J Roentgenol 2013;201:1204-10. [Crossref] [PubMed]
- Heukels P, Moor CC, von der Thüsen JH, Wijsenbeek MS, Kool M. Inflammation and immunity in IPF pathogenesis and treatment. Respir Med 2019;147:79-91. [Crossref] [PubMed]
- Fan L, Liu SY, Li QC, Yu H, Xiao XS. Multidetector CT features of pulmonary focal ground-glass opacity: differences between benign and malignant. Br J Radiol 2012;85:897-904. [Crossref] [PubMed]
- Kim TS, Han J, Kim GY, Lee KS, Kim H, Kim J. Pulmonary inflammatory pseudotumor (inflammatory myofibroblastic tumor): CT features with pathologic correlation. J Comput Assist Tomogr 2005;29:633-9. [Crossref] [PubMed]
- Swensen SJ, Viggiano RW, Midthun DE, Müller NL, Sherrick A, Yamashita K, Naidich DP, Patz EF, Hartman TE, Muhm JR, Weaver AL. Lung nodule enhancement at CT: multicenter study. Radiology 2000;214:73-80. [Crossref] [PubMed]
- Zhang M, Kono M. Solitary pulmonary nodules: evaluation of blood flow patterns with dynamic CT. Radiology 1997;205:471-8. [Crossref] [PubMed]
- Greffier J, Villani N, Defez D, Dabli D, Si-Mohamed S. Spectral CT imaging: Technical principles of dual-energy CT and multi-energy photon-counting CT. Diagn Interv Imaging 2023;104:167-77. [Crossref] [PubMed]
- Deng L, Zhang G, Lin X, Han T, Zhang B, Jing M, Zhou J. Comparison of Spectral and Perfusion Computed Tomography Imaging in the Differential Diagnosis of Peripheral Lung Cancer and Focal Organizing Pneumonia. Front Oncol 2021;11:690254. [Crossref] [PubMed]
- Wang T, Fan Z, Zou L, Hou Y. Can quantitative parameters of spectral computed tomography predict lymphatic metastasis in lung cancer? a systematic review and meta-analysis. Radiother Oncol 2023;183:109643. [Crossref] [PubMed]
- Kim PK, Hong YJ, Sakuma H, Chawla A, Park JK, Park CH, Hong D, Han K, Lee JY, Hur J, Lee HJ, Kim YJ, Suh YJ, Choi BW. Myocardial Extracellular Volume Fraction and Change in Hematocrit Level: MR Evaluation by Using T1 Mapping in an Experimental Model of Anemia. Radiology 2018;288:93-8. [Crossref] [PubMed]
- Kehlet SN, Willumsen N, Armbrecht G, Dietzel R, Brix S, Henriksen K, Karsdal MA. Age-related collagen turnover of the interstitial matrix and basement membrane: Implications of age- and sex-dependent remodeling of the extracellular matrix. PLoS One 2018;13:e0194458. [Crossref] [PubMed]
- Dilshat R, Fock V, Kenny C, Gerritsen I, Lasseur RMJ, Travnickova J, et al. MITF reprograms the extracellular matrix and focal adhesion in melanoma. Elife 2021;10:e63093. [Crossref] [PubMed]
- Zhou Z, Wang R, Wang H, Liu Y, Lu D, Sun Z, Yang G, Xu L. Myocardial extracellular volume fraction quantification in an animal model of the doxorubicin-induced myocardial fibrosis: a synthetic hematocrit method using 3T cardiac magnetic resonance. Quant Imaging Med Surg 2021;11:510-20. [Crossref] [PubMed]
- Oda S, Nakaura T, Utsunomiya D, Hirakawa K, Takashio S, Izumiya Y, Tsujita K, Hata H, Ando Y, Yamashita Y. Late iodine enhancement and myocardial extracellular volume quantification in cardiac amyloidosis by using dual-energy cardiac computed tomography performed on a dual-layer spectral detector scanner. Amyloid 2018;25:137-8. [Crossref] [PubMed]
- Tago K, Tsukada J, Sudo N, Shibutani K, Okada M, Abe H, Ibukuro K, Higaki T, Takayama T. Comparison between CT volumetry and extracellular volume fraction using liver dynamic CT for the predictive ability of liver fibrosis in patients with hepatocellular carcinoma. Eur Radiol 2022;32:7555-65. [Crossref] [PubMed]
- Yoon JH, Lee JM, Klotz E, Jeon JH, Lee KB, Han JK, Choi BI. Estimation of hepatic extracellular volume fraction using multiphasic liver computed tomography for hepatic fibrosis grading. Invest Radiol 2015;50:290-6. [Crossref] [PubMed]
- Chen SS, Yin ZF, Chen T, Qiu H, Wei YR, Du SS, Jin YP, Zhao MM, Wu Q, Weng D, Li HP. Development of a non-infectious rat model of acute exacerbation of idiopathic pulmonary fibrosis. J Thorac Dis 2017;9:96-105. [Crossref] [PubMed]
- Lee JU, Cheong HS, Shim EY, Bae DJ, Chang HS, Uh ST, Kim YH, Park JS, Lee B, Shin HD, Park CS. Gene profile of fibroblasts identify relation of CCL8 with idiopathic pulmonary fibrosis. Respir Res 2017;18:3. [Crossref] [PubMed]
- Bak S, Kim JE, Bae K, Cho JM, Choi HC, Park MJ, Choi HY, Shin HS, Lee SM, Kim HO. Quantification of liver extracellular volume using dual-energy CT: utility for prediction of liver-related events in cirrhosis. Eur Radiol 2020;30:5317-26. [Crossref] [PubMed]
- Fukukura Y, Kumagae Y, Higashi R, Hakamada H, Nakajo M, Maemura K, Arima S, Yoshiura T. Extracellular volume fraction determined by equilibrium contrast-enhanced dual-energy CT as a prognostic factor in patients with stage IV pancreatic ductal adenocarcinoma. Eur Radiol 2020;30:1679-89. [Crossref] [PubMed]
- Qi RX, Jiang JS, Shao J, Zhang Q, Zheng KL, Xiao J, Huang S, Gong SC. Measurement of myocardial extracellular volume fraction in patients with heart failure with preserved ejection fraction using dual-energy computed tomography. Eur Radiol 2022;32:4253-63. [Crossref] [PubMed]
- Robinson AA, Chow K, Salerno M. Myocardial T1 and ECV Measurement: Underlying Concepts and Technical Considerations. JACC Cardiovasc Imaging 2019;12:2332-44. [Crossref] [PubMed]