Noncontact remote sensing of abnormal blood pressure using a deep neural network: a novel approach for hypertension screening
Introduction
Hypertensive disease remains the leading cause of death and chronic disability from cardiovascular diseases worldwide (1,2). However, the disease is currently characterized by low control rates and high morbidity (3) and has become the most significant risk factor for fatal events globally (4). In 2019, hypertensive disease was a major risk factor in 19.2% (16.9–21.3%) of deaths worldwide (1). It has been shown that elevated blood pressure (BP) at a young age (18–45 years) may increase the risk of cardiovascular diseases such as coronary heart disease, stroke, and all-cause mortality later in life. Compared with individuals with ideal BP [systolic blood pressure (SBP) <120 mmHg/diastolic blood pressure (DBP) <80 mmHg], those with class 1 hypertension (140–159/90–99 mmHg) and class 2 hypertension (160–180/100–110 mmHg) had 42% and 101% elevated risks of all-cause mortality, respectively (5). However, many young people tend to ignore this potential risk because hypertension is perceived to be an age-related disease (6,7). In fact, not only is it ignored by young people, hypertension is ignored by people of all ages. A study showed that the detection, monitoring, and control of hypertension were inadequate in all age groups, with only 46% of hypertensive patients having their condition under control (8). Therefore, hypertension should be taken seriously by people of all ages for early detection, diagnosis, and BP as early as possible, we urgently require an abnormal BP detection tool that is efficient and suitable for mass screening.
Deep learning (DL) is widely used in research domains such as computer vision, speech analysis, and natural language processing (NLP). DL is a subset of machine learning (ML) differentiated by the ability of DL algorithms to automatically learn representations from data such as images, videos, or text without bringing in knowledge from the human domain. The term “deep” in DL denotes a multi-layer algorithm or neural network that recognizes patterns in data. The highly flexible architecture of DL allows it to learn directly from raw data, similar to how the human brain operates, and its predictive accuracy improves as more data becomes available. Recently, DL algorithms such as convolutional neural network (CNN) have been introduced to disease diagnosis and other medical activities. In addition to traditional cuff BP measurement, novel approaches to the monitoring of BP are being developed by DL. Several studies have reported that photoplethysmography (PPG) can be used to measure BP. A CNN has been utilized to estimate BP from PPG signals without the need for waveform analysis and signal feature extraction (9). Zhang et al. (10) proposed a refined BP prediction strategy that uses single-channel PPG signals to stratify populations by cardiovascular status before BP estimation and a DL model (BiLSTM-At) to estimate the long-term BP trend for different cardiovascular diseases groups. Furthermore, based on a conformal and flexible strain sensor array and DL neural networks, an intelligent BP and cardiac function monitoring system has been developed. A user can wear the conformal flexible sensor array on the wrist and acquire high-quality pulse signals without precise positioning or wired connections (11).
Since the global outbreak of coronavirus disease 2019 (COVID-19), body temperature measurement by remote infrared thermography has become a routine screening procedure in many public places, especially in crowded places such as subways, train stations, and airports (12,13). Therefore, it is of great clinical and socioeconomic value to develop an abnormal BP detection system using existing remote infrared imaging equipment and data, thereby enabling large-scale population screening. In particular, with the global spread of COVID-19, it is important for studies on disease screening to take the special group of novel coronavirus carriers into consideration. Therefore, this study included people who tested positive for COVID-19 nucleic acids. Moreover, the establishment of noncontact abnormal BP detection systems in airports and stations could facilitate the detection of high-risk populations and help reduce cardiovascular emergencies on airplanes, trains, and other means of transportation (14).
Therefore, we developed a new DL model that can be used with existing equipment and data to achieve accurate and efficient noncontact remote detection of abnormal BP. The model is suitable for all people with abnormal BP, including patients who were coronavirus-positive during the COVID-19 pandemic. We present this article in accordance with the STARD reporting checklist (available at https://qims.amegroups.com/article/view/10.21037/qims-23-970/rc).
Methods
Data
We used the dataset from a pilot cohort study on COVID-19 (15,16) for this cross-sectional study. BP was measured by a Welch Allyn 71WT monitor (Welch Allyn, Skaneateles Falls, NY, USA) prior to infrared imaging, and an abnormal increase in BP was defined as a SBP ≥140 mmHg and/or DBP ≥90 mmHg. In addition, the dataset included participant information about physical activity, symptoms such as fever or cough, and alcohol and tobacco consumption over the last 2 hours. Thermal images were recorded in video mode using a digital thermal imaging camera TI-128 from Omega Engineering, Inc. (Norwalk, CT, USA) with a speed of 5 frames per second. The body image was segmented by three registered physicians into the head, chest, abdomen, and hands (including forearms), and each final segmentation required the consensus of all three physicians. Individuals aged 18 and above who requested a polymerase chain reaction (PCR) test and signed the informed consent were included in the criteria. Individuals who were unable to maintain a deep breath for a minimum of 10 seconds or were unwilling to reveal their bare skin on their backs were excluded from the criteria. The volunteers included in Figures 1-3 are from pilot cohort study on COVID-19 (15,16). The study was conducted in accordance with the Declaration of Helsinki (as revised in 2013).
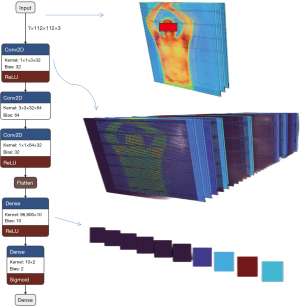
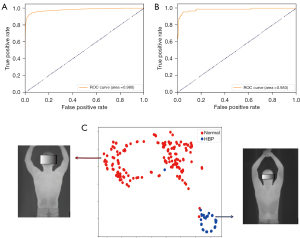
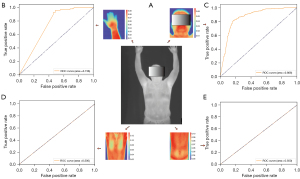
PanycNet neural network model
We designed a deep neural network model specifically for this type of data. First, the infrared thermogram videos were randomly divided into training and validation sets with a ratio of 9:1, and then the videos were sliced using an interval of 5 frames to obtain a total of 3,800 upper body infrared thermograms. The data were normalized before they were entered into the network to make the data more suitable for the network weights. The main deep network structure consisted of 3 convolutional layers, 1 flattened layer, 1 fully connected layer, and the output layer of the category to which the input belonged. The output of the last fully connected layer of the network was set according to the number of categories, and the input image size was 112×112×3 (image matrix size). The model was visualized (Figure 1) using the Netron package, and a three-dimensional visualization of the model data analysis and transmission process was performed using Zetane Engine (https://github.com/Zetane/viewer). Regarding the optimization hyper-parameters, we made combinations of learning rate, number of network layers, and number of training times, respectively, in the training phase, and finally determined the following settings for the hyper-parameters after continuous combinations of the hyper-parameters. The learning rate was 1e−4 and epochs were 50. The optimizer used was Adam. Convolutional network layers were 3. The activation, classification layer, and loss functions were rectified linear unit (ReLu), Sigmoid, and mean square error (MSE), respectively. Optimizing hyperparameters was not performed automatically. To investigate the role of the information from each body part in BP detection, we calculated the diagnostic area under the receiver operating characteristic curve (AUC) values of the upper body, head, chest, abdomen, and hand (including forearms) segments individually. The principal codes of the PanycNet neural network model are provided in Appendix 1.
Evaluation and data analysis
Precision, recall, and the AUC were calculated for the hypertension detection algorithm using normalized BP values measured by the monitors. We evaluated the abnormal BP prediction ability overall (upper body) and of the head, hands (including forearms), chest, and abdomen. Thermograms were used to show the importance of each region of the image in the classification task, and t-distribution stochastic neighbor embedding (t-SNE) (17) was used to reduce dimensionality and visualize the data. The deep neural network model, plotting, and body segmentation were performed using Python 3.8, the Matplotlib package, and LabelImg software, respectively (18). We conducted subgroup analyses to demonstrate model stability. We performed subgroup analyses according to age (greater than or equal to 40 years group and less than 40 years group) and gender (male and female groups).
Statistics
Deep spatio-temporal convolutional models were built using Python software (3.9). Consecutive values were compared using the Student’s t-test or Mann-Whitney U test. All comparisons were two-sided, and a P value <0.05 was considered significant. Randomized segmentation was implemented using the NumPy package random function. The main hardware is the graphics card RTX 3080 Ti (Nvidia, Santa Clara, CA, USA).
Results
We used the dataset from a pilot cohort study on COVID-19 (15,16) for this cross-sectional study, which included a total of 252 volunteers recruited from July 22 to September 4, 2020 (Table 1). Among the participants, 158 (62.70%) were males, and 94 (37.30%) were females, with a mean age of 37.08 (18.00–75.00) years and abnormally high blood pressure (HBP) in 73 people (29.20%). All participants underwent novel coronavirus PCR testing using nasopharyngeal swabs prior to videotaping, with 59 (23.51%) found to be positive. Only 32.00% of the febrile population had hypertension, and the effect of fever on the diagnosis of hypertension was excluded.
Table 1
Characteristics | Values |
---|---|
Age (years) | 37.08±13.50/34.00 (18.00–75.00) |
Weight (kg) | 78.50±17.65/77.00 (45.00–125.00) |
Height (cm) | 169.41±8.60/170.00 (147.00–196.00) |
Body temperature (℃) | 36.66±0.62/36.70 (34.50–38.60) |
Sex | |
Female | 94 (37.30) |
Male | 158 (62.70) |
Resting (<2 hours) | |
No | 98 (38.89) |
Yes | 154 (61.11) |
Walking (<2 hours) | |
No | 241 (95.63) |
Yes | 11 (4.37) |
Running (<2 hours) | |
No | 251 (99.60) |
Yes | 1 (0.40) |
Gym (<2 hours) | |
No | 251 (99.60) |
Yes | 1 (0.40) |
Fever (<24 hours) | |
No | 196 (77.78) |
Yes | 56 (22.22) |
Chills | |
No | 228 (90.48) |
Yes | 24 (9.52) |
PCR results | |
Negative | 192 (76.49) |
Positive | 59 (23.51) |
Abnormally HBP | |
No | 177 (70.80) |
Yes | 73 (29.20) |
Abnormally HBP of the febrile population | |
No | 38 (68.00) |
Yes | 18 (32.00) |
Data are shown as mean ± standard deviation/median (min–max) or n (%). There was no significant difference in baseline data (including age, height, weight, and body temperature) between individuals with abnormal BP and those with normal BP, with P values >0.05. PCR, polymerase chain reaction; HBP, high blood pressure; BP, blood pressure.
The model obtained an AUC of 0.980 (0.973–0.989) in the training set, with an accuracy of 0.930 (0.898–0.967) in identifying abnormally HBP and an accuracy of 0.972 (0.956–0.983) in identifying normal BP (Table 2, Figure 2A). The model obtained an AUC of 0.983 (0.904–1.000) in the validation set, with an accuracy of 0.931 (0.778–1.000) in identifying abnormally HBP and 0.959 (0.818–1.000) in identifying normal BP (Table 2, Figure 2B). J indexes of the training set and testing set were both 0.94. The t-SNE visualization showed that the two types of data were clearly separable (Figure 2C). AUCs of 0.868 (0.603–0.994) for head information classification, 0.500 (0.091–0.663) for chest information classification, 0.500 (0.364–0.818) for abdominal information classification, and 0.736 (0.500–1.000) for hand information classification were calculated (Figure 3). A conceptual diagram of the application of this technology in public places is shown in Figure 4. To completely protect the privacy of volunteers, we masked their faces in all displayed images (Figures 1-3).
Table 2
Evaluation indicators | Training set | Validation set |
---|---|---|
AUC (95% CI) | 0.980 (0.973–0.989) | 0.983 (0.904–1.000) |
HBP | ||
Precision (95% CI) | 0.930 (0.898–0.967) | 0.931 (0.778–1.000) |
Recall (95% CI) | 0.932 (0.909–0.960) | 0.930 (0.750–1.000) |
F1-score (95% CI) | 0.931 (0.910–0.953) | 0.927 (0.800–1.000) |
Non-HBP | ||
Precision (95% CI) | 0.972 (0.956–0.983) | 0.959 (0.818–1.000) |
Recall (95% CI) | 0.971 (0.957–0.985) | 0.959 (0.800–1.000) |
F1-score (95% CI) | 0.971 (0.962–0.979) | 0.958 (0.842–1.000) |
AUC, area under the receiver operating characteristic curve; CI, confidence interval; HBP, high blood pressure.
The subgroup results showed an AUC of 0.999 (0.994–1.000) in the lower age group and 0.974 (0.883–1.000) in the higher age group. In the male group, AUC was 0.983 (0.953–0.997) and in female group AUC was 0.995 (0.982–1.000). The above results indicated that the model was very stable in all subgroups and there was no overfitting.
Discussion
The application of artificial intelligence (AI) in the medical field is becoming increasingly widespread. Cardiovascular disease, as an important factor in the current threat to human health, urgently needs early detection, diagnosis, and treatment, and at present, the screening and prediction of cardiovascular disease through AI is rapidly developing; the results have been or will soon be clinically implemented. A study has shown that AI plays an important role in the management of hypertension, including measuring BP, predicting arterial hypertension (AH) development, diagnosing AH, predicting AH treatment success, and predicting AH prognosis (19). Besides, a brain signature, capable of accurately predicting BP level from individually unique connectivity profiles, can be identified by ML, which corroborates the model robustness in capturing reliable brain-BP relationships, and furthermore emphasizes the demand of early and optimum control of BP (20). In addition to AH, ML has also reported the potential applicability to provide useful clinical prognostic information to stratify complex heterogeneity in patients with heart failure (21). Therefore, AI is promising in the management of cardiovascular diseases.
As the understanding of hypertension and its complications increases, early detection and timely intervention have become important tools for preventing and treating these diseases as well as reducing mortality (22). However, early detection of hypertension remains difficult because of limited medical resources and generally insufficient attention in society (23). With the global spread of COVID-19, remote temperature detection has become an important means of epidemic prevention and control in public places, which has further promoted the widespread use of an infrared imaging technique that was originally mainly used in transportation facilities such as railroads and airports (24). Infrared thermography captures the infrared light emitted by an object and uses the infrared spectrum to characterize its surface temperature (25). Thermal imagers, which are relatively inexpensive and easy to operate, have become widely used. It has been reported that this technique has been used to detect breast tumors and pleural effusions with acceptable results (26). In contrast, to our knowledge, no studies have yet used this technique to detect abnormal BP levels. According to our findings, there is a correlation between BP and infrared thermograms and, theoretically, a causal relationship between them. First, from a physical point of view, all objects with a temperature above absolute zero emit infrared radiation, the intensity of which is related to the temperature of the object. According to Planck’s law, dry skin has an emissivity of 0.98, which is almost an ideal blackbody and can be considered a long-wave infrared radiator. Therefore, human skin emits infrared radiation depending on its temperature. Physiologically, blood carries body heat and distributes it throughout the body via the vascular system. When blood flows through the blood vessels under the skin, it transfers heat to the surrounding tissues including the skin. When the BP changes, the blood flow rate, and blood flow volume will change accordingly, which will result in a change in the temperature of the skin. Thus, there is a causal relationship between BP and infrared thermography, and BP can be indirectly monitored by infrared imaging through skin surface temperature. However, because there has been a decline in the willingness of people to attend hospitals for examinations and consultations because of the spread of COVID-19 (27), this convenient large-scale screening technique, which can utilize available equipment and information, is even more valuable. In particular, given the global spread of COVID-19, the development of new medical techniques should take into account possible novel coronavirus-infected people and carriers. The viremia stage of infection is characterized by changes in basic vital signs such as body temperature and respiratory patterns (28,29), which may have a considerable impact on prediction and analysis based on these characteristics. In particular, it has been demonstrated that AI models may reflect and amplify human bias (30) and degrade their performance on data from populations that make up a relatively small portion of the training data (e.g., women, ethnic minorities, or patients with low socioeconomic status). Similarly, if the population of novel coronavirus carriers is not involved in the study, the AI algorithm may make incorrect diagnoses from the data of this population, which may delay their access to treatment. This is particularly important in large-scale population screening.
To address this, we developed a DL model for remote noncontact abnormal BP monitoring that uses infrared thermograms and was trained using datasets containing images of novel coronavirus carriers. This model is expected to provide a reliable method for abnormal BP screening in an epidemic situation.
In this study, we found that when detecting abnormal BP by infrared thermography, data from as many body parts as possible were needed for optimal results (AUC =0.983). In the upper body structures, the head (AUC =0.868) and the hands (AUC =0.736) were more important, whereas the chest and abdomen were less predictive (AUC =0.500), which is probably related to the fact that the head has the densest network of blood vessels and heat dissipation system on the body surface, and the hands have more superficial major blood vessels, allowing both sites to better respond to BP changes. Meanwhile, the chest and abdominal AUC was 0.500, indicating that infrared thermography through the chest and abdomen is as useful as randomized testing for the detection of hypertension and is not valuable for screening for hypertension. This suggested that we should obtain as much information as possible from all parts of the body, focusing on the head and hands, when developing applications. This may mean that comprehensive imaging data for security screening using infrared light at airports and other places will provide ideal conditions for the developed model. We chose the DL model PanycNET, because the data in this study involved dynamic images, and this amount of data can best be supported by an end-to-end DL model. DL is more suitable for our application scenario of rapid screening than the traditional method of extracting features before ML modeling.
The strengths of our study can be summarized as follows. First, our technique requires less-specialized equipment and can conduct BP screening at larger distances than some of the reported techniques using photoelectric volumetric pulse waves for noncontact BP measurement (31). Additionally, our method can screen a larger number of subjects because it does not require the acquisition of equipment or installation of relevant software to examine people. Moreover, infrared imaging is less affected by body skin obscured by clothing or accessories (25), allowing more detection information to be utilized and improving reliability.
Second, in contrast to some previously reported remote BP monitoring systems (32,33), our protocol eliminates the need for contact with the subject and can minimize the impact on both the screening organizer and the subject. For patients with infectious diseases, remote vital sign detection is also possible and accessible, which will reduce the cost of care and the risk of cross-infection.
Third, we trained the model using a dataset containing thermograms of novel coronavirus carriers, which ensures that this technique can be applied to this population and avoid the further loss of healthcare opportunities for this vulnerable group.
Fourth, given that infrared imaging is currently commonly used for security screening at airports and railroads or epidemic prevention in public places (24,34,35), our technique allows hypertension screening to be performed simultaneously with those tests necessary for travel, minimizing screening costs. It also ensures a sufficiently large screening population to help maximize the detection of potential hypertension patients, especially BP abnormalities that remain undiscovered. In particular, for people with BP abnormalities who are about to travel by airplane or train, early warning could improve responses and reduce cardiovascular emergencies (14).
Fifth, the data used to build the model came from different individuals in both healthy and diseased states (e.g., fever, cough, sore throat, diarrhea, vomiting, loss of smell, loss of taste, shivering/chills, headache, myalgia, and generalized joint pain) and from different individuals who had engaged in specific behaviors that may affect BP, such as exercising, smoking, and drinking. Therefore, we believe that the data used to train the model can cover a considerable number of real-life scenarios.
Limitations
The present study also has several limitations. First, as a single-center cross-sectional study, it was performed using a dataset from the Mexican population. As a result, its applicability to populations in other regions still needs to be evaluated using larger multicenter datasets. The reliability validation of this study would benefit from an independent external validation dataset; performing cross-validation will also support the stability of the model.
Second, only 1 type of infrared imaging equipment was used in this study, and the effect of the data acquired by other types of infrared imaging devices on performance remains to be evaluated on larger multicenter datasets.
Third, because special groups such as minors (<18 years old) and pregnant women were not included, further validation of the performance of the model on such groups is warranted.
Fourth, because the dataset contained information on individuals with symptoms such as fever and chills, as well as those who had performed activities that could affect BP, such as exercise or alcohol consumption, before data collection, further examination is needed to diagnose hypertensive disease, although it is possible to accurately distinguish people with abnormally HBP.
Fifth, abnormal BP was not discussed separately in the results section according to SBP, DBP, and mean BP, and therefore the relationship between the infrared thermograms of each type of BP is not clear and should be analyzed in more detail in subsequent studies. However, according to the definition of hypertension, an SBP ≥140 mmHg and/or a DBP ≥90 mmHg should be screened for either type of abnormally elevated BP, and therefore distinguishing between what type of hypertension is not the focus of the discussion in this study.
Sixth, due to the “black box”, shorthand for models that are sufficiently complex that they are not straightforwardly interpretable to humans, humans can not completely trust the performance of DL, which is a common limitation of DL (36).
Finally, deploying this technology on a population without COVID-19 carriers will require further validation; given the nature of the dataset used for building the DL model.
Conclusions
We developed a remote noncontact abnormal BP detection model based on infrared thermography using a DL approach. This model has excellent detection performance and is suitable for all population with abnormal BP including the population with COVID-19, which may help to promote early detection and early intervention among people with abnormal BP, ultimately reducing the incidence of severe complications and mortality.
Acknowledgments
This study benefited from the high-quality data of previous studies and authors whose true generosity has advanced cardiovascular medicine.
Funding: This evaluation study was supported by
Footnote
Reporting Checklist: The authors have completed the STARD reporting checklist. Available at https://qims.amegroups.com/article/view/10.21037/qims-23-970/rc.
Conflicts of Interest: All authors have completed the ICMJE uniform disclosure form (available at https://qims.amegroups.com/article/view/10.21037/qims-23-970/coif). The authors have no conflicts of interest to declare.
Ethical Statement: The authors are accountable for all aspects of the work in ensuring that questions related to the accuracy or integrity of any part of the work are appropriately investigated and resolved. The study was conducted in accordance with the Declaration of Helsinki (as revised in 2013).
Open Access Statement: This is an Open Access article distributed in accordance with the Creative Commons Attribution-NonCommercial-NoDerivs 4.0 International License (CC BY-NC-ND 4.0), which permits the non-commercial replication and distribution of the article with the strict proviso that no changes or edits are made and the original work is properly cited (including links to both the formal publication through the relevant DOI and the license). See: https://creativecommons.org/licenses/by-nc-nd/4.0/.
References
- Global burden of 87 risk factors in 204 countries and territories, 1990-2019: a systematic analysis for the Global Burden of Disease Study 2019. Lancet 2020;396:1223-49. [Crossref] [PubMed]
- Del Rio AI, Moreno Velásquez I, Roa R, Montenegro Mendoza R, Motta J, Quintana HK. Prevalence of hypertension and possible risk factors of hypertension unawareness among individuals aged 30-75 years from two Panamanian provinces: Results from population-based cross-sectional studies, 2010 and 2019. PLoS One 2022;17:e0276222. [Crossref] [PubMed]
- Hajjar I, Kotchen JM, Kotchen TA. Hypertension: trends in prevalence, incidence, and control. Annu Rev Public Health 2006;27:465-90. [Crossref] [PubMed]
- Kwan GF, Mayosi BM, Mocumbi AO, Miranda JJ, Ezzati M, Jain Y, Robles G, Benjamin EJ, Subramanian SV, Bukhman G. Endemic Cardiovascular Diseases of the Poorest Billion. Circulation 2016;133:2561-75. [Crossref] [PubMed]
- Luo D, Cheng Y, Zhang H, Ba M, Chen P, Li H, Chen K, Sha W, Zhang C, Chen H. Association between high blood pressure and long term cardiovascular events in young adults: systematic review and meta-analysis. BMJ 2020;370:m3222. [Crossref] [PubMed]
- Liu J, Bu X, Wei L, Wang X, Lai L, Dong C, Ma A, Wang T. Global burden of cardiovascular diseases attributable to hypertension in young adults from 1990 to 2019. J Hypertens 2021;39:2488-96. [Crossref] [PubMed]
- Dhungana RR, Pedisic Z, Dhimal M, Bista B, de Courten M. Hypertension screening, awareness, treatment, and control: a study of their prevalence and associated factors in a nationally representative sample from Nepal. Glob Health Action 2022;15:2000092. [Crossref] [PubMed]
- Merai R, Siegel C, Rakotz M, Basch P, Wright J, Wong B, Thorpe P. CDC Grand Rounds: A Public Health Approach to Detect and Control Hypertension. MMWR Morb Mortal Wkly Rep 2016;65:1261-4. [Crossref] [PubMed]
- Liang H, He W, Xu Z. A deep learning method for continuous noninvasive blood pressure monitoring using photoplethysmography. Physiol Meas 2023; [Crossref] [PubMed]
- Zhang Y, Ren X, Liang X, Ye X, Zhou C. A Refined Blood Pressure Estimation Model Based on Single Channel Photoplethysmography. IEEE J Biomed Health Inform 2022;26:5907-17. [Crossref] [PubMed]
- Li S, Wang H, Ma W, Qiu L, Xia K, Zhang Y, Lu H, Zhu M, Liang X, Wu XE, Liang H, Zhang Y. Monitoring blood pressure and cardiac function without positioning via a deep learning-assisted strain sensor array. Sci Adv 2023;9:eadh0615. [Crossref] [PubMed]
- Chiang MF, Lin PW, Lin LF, Chiou HY, Chien CW, Chu SF, Chiu WT. Mass screening of suspected febrile patients with remote-sensing infrared thermography: alarm temperature and optimal distance. J Formos Med Assoc 2008;107:937-44. [Crossref] [PubMed]
- Khaksari K, Nguyen T, Hill B, Quang T, Perreault J, Gorti V, Malpani R, Blick E, González Cano T, Shadgan B, Gandjbakhche AH. Review of the efficacy of infrared thermography for screening infectious diseases with applications to COVID-19. J Med Imaging (Bellingham) 2021;8:010901. [Crossref] [PubMed]
- Cummins RO, Chapman PJ, Chamberlain DA, Schubach JA, Litwin PE. In-flight deaths during commercial air travel. How big is the problem? JAMA 1988;259:1983-8. [Crossref] [PubMed]
- Tamez-Peña J, Yala A, Cardona S, Ortiz-Lopez R, Trevino V. Upper body thermal images and associated clinical data from a pilot cohort study of COVID-19. Version 1.1. PhysioNet 2021. Available online:
10.13026/wfr2-5973 10.13026/wfr2-5973 - Goldberger AL, Amaral LA, Glass L, Hausdorff JM, Ivanov PC, Mark RG, Mietus JE, Moody GB, Peng CK, Stanley HE. PhysioBank, PhysioToolkit, and PhysioNet: components of a new research resource for complex physiologic signals. Circulation 2000;101:E215-20. [Crossref] [PubMed]
- Linderman GC, Steinerberger S. Clustering with t-SNE, provably. SIAM J Math Data Sci 2019;1:313-32. [Crossref] [PubMed]
- LabelImg TD. GitHub repository. Available online: https://github.com/tzutalin/labelImg
- Visco V, Izzo C, Mancusi C, Rispoli A, Tedeschi M, Virtuoso N, Giano A, Gioia R, Melfi A, Serio B, Rusciano MR, Di Pietro P, Bramanti A, Galasso G, D'Angelo G, Carrizzo A, Vecchione C, Ciccarelli M. Artificial Intelligence in Hypertension Management: An Ace up Your Sleeve. J Cardiovasc Dev Dis 2023;10:74. [Crossref] [PubMed]
- Avvisato R, Forzano I, Varzideh F, Mone P, Santulli G. A machine learning model identifies a functional connectome signature that predicts blood pressure levels: imaging insights from a large population of 35 882 patients. Cardiovasc Res 2023;119:1458-60. [Crossref] [PubMed]
- Nakano K, Nochioka K, Yasuda S, Tamori D, Shiroto T, Sato Y, Takaya E, Miyata S, Kawakami E, Ishikawa T, Ueda T, Shimokawa H. Machine learning approach to stratify complex heterogeneity of chronic heart failure: A report from the CHART-2 study. ESC Heart Fail 2023;10:1597-604. [Crossref] [PubMed]
- Schmidt BM, Durao S, Toews I, Bavuma CM, Hohlfeld A, Nury E, Meerpohl JJ, Kredo T. Screening strategies for hypertension. Cochrane Database Syst Rev 2020;5:CD013212. [PubMed]
- Madela S, James S, Sewpaul R, Madela S, Reddy P. Early detection, care and control of hypertension and diabetes in South Africa: A community-based approach. Afr J Prim Health Care Fam Med 2020;12:e1-9. [Crossref] [PubMed]
- Dell'Isola GB, Cosentini E, Canale L, Ficco G, Dell'Isola M. Noncontact Body Temperature Measurement: Uncertainty Evaluation and Screening Decision Rule to Prevent the Spread of COVID-19. Sensors (Basel) 2021;21:346. [Crossref] [PubMed]
- Ring EF, Ammer K. Infrared thermal imaging in medicine. Physiol Meas 2012;33:R33-46. [Crossref] [PubMed]
- Arora N, Martins D, Ruggerio D, Tousimis E, Swistel AJ, Osborne MP, Simmons RM. Effectiveness of a noninvasive digital infrared thermal imaging system in the detection of breast cancer. Am J Surg 2008;196:523-6. [Crossref] [PubMed]
- Liu Z, Huang S, Lu W, Su Z, Yin X, Liang H, Zhang H. Modeling the trend of coronavirus disease 2019 and restoration of operational capability of metropolitan medical service in China: a machine learning and mathematical model-based analysis. Glob Health Res Policy 2020;5:20. [Crossref] [PubMed]
- Guan WJ, Zhong NS. Clinical Characteristics of Covid-19 in China. N Engl J Med 2020;382:1861-2. Reply. [PubMed]
- Gong J, Sun Y, Xie L. ACEI/ARB Drug Therapy in COVID-19 Patients: Yes Or No? J Transl Int Med 2021;9:8-11. [Crossref] [PubMed]
- Seyyed-Kalantari L, Zhang H, McDermott MBA, Chen IY, Ghassemi M. Underdiagnosis bias of artificial intelligence algorithms applied to chest radiographs in under-served patient populations. Nat Med 2021;27:2176-82. [Crossref] [PubMed]
- Mukherjee R, Ghosh S, Gupta B, Chakravarty T. A Universal Noninvasive Continuous Blood Pressure Measurement System for Remote Healthcare Monitoring. Telemed J E Health 2018;24:803-10. [Crossref] [PubMed]
- Kario K. Management of Hypertension in the Digital Era: Small Wearable Monitoring Devices for Remote Blood Pressure Monitoring. Hypertension 2020;76:640-50. [Crossref] [PubMed]
- Sana F, Isselbacher EM, Singh JP, Heist EK, Pathik B, Armoundas AA. Wearable Devices for Ambulatory Cardiac Monitoring: JACC State-of-the-Art Review. J Am Coll Cardiol 2020;75:1582-92. [Crossref] [PubMed]
- Chen Y, Wang B, Zang Y, Zhang C, Zhang H, Yuan Y, Zhou D, Hou L, Pan M, Wang X. The high-performance imaging verification of Si: P blocked impurity band detector for very-long-wave-infrared spectral range. IEEE J Quantum Electron 2020;56:1-6.
- Li R, Liu Z, Yuan S, Zhu Z, Ye J, Zhang X. Infrared Sensor ZTP-135SR and Its Application in Infrared Body Temperature Measurement. Zhongguo Yi Liao Qi Xie Za Zhi 2022;46:160-3. [PubMed]
- Petch J, Di S, Nelson W. Opening the Black Box: The Promise and Limitations of Explainable Machine Learning in Cardiology. Can J Cardiol 2022;38:204-13. [Crossref] [PubMed]