The role of genetic and epigenetic factors in determining the risk of spinal fragility fractures: new insights in the management of spinal osteoporosis
Introduction
Spinal fragility fractures stemming from osteoporosis have been shown to be more prevalent in Caucasians relative to Eastern Asian populations. For example, the prevalence of osteoporotic vertebral fractures (OVF) is much higher in Italian Caucasian in comparison to Hong Kong Chinese women (1), and osteoporotic fracture prevalence is more than 50% lower in the elderly Chinese in comparison with a European cohort (2). The differences in prevalence are important and should be taken into account in order to optimise diagnostic protocols, such as those based on T-score values, for different groups with varying risks for developing fragility fractures (3). One of the difficulties with the current knowledge base is that there is a lack of a defined standard for OVF risk prognostication through imaging (4). As such, there is an ongoing search for factors that can protect the vertebrae from osteoporosis or promote their healing process; as well as for quantitative and qualitative measures of disease monitoring useful in the context of a comprehensive clinical-radiological workup. As the global burden of low bone mineral density (BMD) and the disability-adjusted life years attributed to this phenomenon are growing each year, even in countries with good protective measures, this poses a significant challenge to improving outcomes for patients (5). We carried out a narrative literature review to synthesize novel discoveries across relevant and recent articles in the PubMed database with no language restriction. With a focus on spinal fragility fractures, we clarify the potential genetic and epigenetic risk factors that could be contributing to the different incidence and prevalence across different populations (Figure 1), and make use of this opportunity to outline the advancements in diagnostic approaches, including novel biomarkers and state-of-the-art imaging modalities.
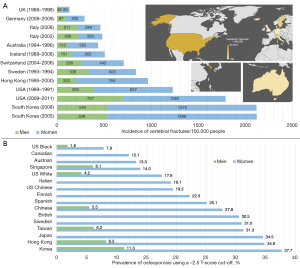
Genetic factors
Genetic causes of osteoporosis have been extensively investigated; however, less is known about those specifically causing OVF (8). One of the key contributors to a higher rate of spinal osteoporotic fractures in western countries could be the occurrence of mutations in specific genetic loci conveying higher susceptibility to the Caucasian population (or vice versa, a lower risk in East Asian cohorts). For instance, one of the hallmarks of osteoporosis is low BMD, and several genetic loci have been implicated in genome-wide association studies (GWAS) showing a strong association with low BMD, and in some cases a direct correlation with the occurrence of vertebral fractures (9). Unfortunately, many of these studies do not make a clear distinction in different fracture phenotypes and examine them altogether; however spinal fractures are clinically different and could be affected by different genetic loci.
So far only two GWAS focused specifically on vertebral osteoporotic fractures (Table 1) (13). A GWAS of a Dutch cohort in the Rotterdam Study found that a single nucleotide polymorphism (SNP) at the 16q24 locus mapping to FOXC2 was associated with a higher vertebral fracture risk, but when this SNP was evaluated through a meta-analysis covering 14 more studies this association, although confirmed, was not significant (10). These data point to one of the first challenges in investigating these genetic loci: the wide heterogeneity in the definition of the fractures included across multiple studies (8). The second study identified a locus on chromosome 2q13, which was significantly associated with vertebral fractures. Of note, such locus was not known from previous studies to affect BMD: hence we could argue that the correlation demonstrated by the authors could highlight an independent mechanism of susceptibility to osteoporosis (12). This is an interesting point because a significantly larger quantity of loci has been found with associations with BMD at various sites: a recent effort found at least 15 fracture loci (not specific to the spine), all of which were already associated with BMD, nonetheless the effect of the SNPs was smaller on the fracture risk than on BMD itself (14). All those aspects ultimately indicate that many factors can influence the different incidence and prevalence of spinal osteoporotic fractures around the world. In terms of GWAS specific to the Eastern Asian population, the JAG1 gene has been found to be associated with increased BMD in lumbar vertebrae in a female Hong Kong cohort of patients; its levels of expression (based on the increased BMD conferred to those with a specific SNPs) could be a potential factor for fragility fracture pathogenesis (15).
Table 1
Gene | Locus | Relevance | Bibliography |
---|---|---|---|
FOXC2 | Ch 16; q24 | • Highly expressed in bone tissue and codes for a transcription factor | Oei et al. 2014, (10) |
• Contributes to osteoblast differentiation via Wnt/β-catenin signals | |||
• Associated with other non-random vertebral birth defects (VACTERL association) (11) | |||
SLC20A1 or TLL | Ch 2; q13 | • Uncertainty if the SNP here is associated with TLL or SLC20A1—SLC20A1 is involved in mineralisation whilst TLL is involved in neuronal development and injury signalling | Alonso et al. 2018, (12) |
• SLC20A1 codes for a sodium-phosphate symporter which regulates phosphate transport in osteoblasts | |||
• SLC20A1 not previously associated with BMD or fractures | |||
• No association when compared for association with volumetric vertebral BMD—suggesting a novel, independent pathway for vertebral fracture independent of BMD |
Many other factors have been associated with low BMD, here we focus only on those that are spinal fracture specific. VACTERL, vertebral, ano-rectal, cardiac, tracheo-esophageal, renal, and limb; SNP, single nucleotide polymorphism; BMD, bone mineral density.
Another aspect to consider is peak bone mass, increases in which have been shown to reduce the fracture risk at the population level (16). The bone mass gain in children could act as a protective factor from future osteoporosis; in children, the genes IZUMO3 and RBFOX1 have been associated in a GWAS with BMD of the lumbar spine in a site-specific manner (17). In addition, a non-coding variant near the EN1 locus was found with whole genome sequencing and showed age-specific effects; it was associated with a decreased risk of fracture and, when conditionally lost in a mouse model, led to low bone mass (18). EN1 and RANKL both displayed age-specific effects and suggest that either peak bone mass or peak BMD are the consequence of some of the signalling pathways in early life, and that the consequences of these expression patterns remains throughout life (19). It is possible, therefore, that the focus should be more on acquiring peak bone mass in childhood as well as preventing bone resorption and osteopenia as a protective measure against osteoporotic fractures. However, there are no studies comparing the peak bone mass of Caucasian versus Asian children, and the difference in peak bone mass seen between women of European and South Asian background was no longer significant when adjusting for weight and height (20).
An additional approach to unveil the genetic basis of OVF could be to consider genes conveying protective traits to bone health. In this way, studying disorders and rare mutations that are associated with a high bone mass (which will reduce bone fragility and fracture risk), could also help clarify the differences between populations. In sclerosteosis, the LRP family of genes have been suggested to increase signalling in the WNT/β-catenin pathway and confer this increase in bone mass (21). Indeed, this pathway is indicated in epigenetic regulation of osteoblast differentiation (Table 2). Conversely, an insight into the risk factors for osteoporotic fractures could be gained by studying the hallmarks of inherited osteolysis disorders in which bone undergoes spontaneous and rapid lysis and resorption (30). Some disorders in this family of conditions are hereditary and the causative mutation have been identified, such as the MAFB gene in multicentric carpotarsal osteolysis syndrome (31). Some, like the non-hereditary Gorham-Stout disease (GSD), also known as phantom bone disease, display the involvement of pathways such as the mTOR, PTEN and RANKL. mTOR contributes to changes in angiogenic and osteoclast signalling and leads to the replacement of bone with vascular or connective tissue (32). Indeed, the mTOR inhibitor sirolimus has successfully been used to treat a number of patients with GSD (33). Alternatively, the RANK-ligand inhibitor denosumab could be a useful anti-resorptive agent. In a very small study, it was shown to increase BMD and reduce fractures in Japanese children with osteogenesis imperfecta with osteoporosis (34), whereas in a very large study of 7,868 post-menopausal women with osteoporosis, it decreased the risk of radiographic vertebral fracture by 68% (35).
Table 2
Epigenetic factor families | Genes and pathways involved | Bibliography |
---|---|---|
DNA methylation | • BMP2, RUNX2, OSX | Zhang et al. 2011, (22); Cao et al. 2018, (23) |
• Wnt/β-Catenin, OPG/RANKL/RANK pathways | ||
Histone acetylation | • OPN, OCN, ALP, OSX, RUNX2 | Piao et al. 2013, (24); Xu et al. 2021, (25) |
• SIRT1 and its downstream post-transcriptional pathways (p53, FoxOs, PPAR) | ||
Histone methylation | • Wnt/β-Catenin pathway, BNMP2, RUNX2, OSX—inhibit OB | Flowers et al. 2010, (26) |
• DLX, HOX promotes OB | ||
lncRNAs | • RUNX2, SBP2 and Wnt/β-Catenin, RANK/RANKL/OPG pathways to promote OB | Li et al. 2020, (27) |
• P38 MAPK and BMP/TGF-β to inhibit OB | ||
miRNAs | • NF-κB, PI3K/AKT pathways promote OB | Letarouilly et al. 2019, (28) |
• OSX, TCF, Wnt, MARK pathways inhibit OB | ||
circRNAs | • Act on a variety of miRNA targets which subsequently act on the above-mentioned pathways | Dou et al. 2016, (29) |
Many other factors have been associated with low BMD, here we focus only on those that are spinal fracture specific. OB, osteoblast differentiation; (lnc)RNAs, long non-coding RNAs; (mi)RNAs, micro RNAs; (circ)RNAs, circular RNAs; BMD, bone mineral density.
Whilst there is evidence for aberrant activity of certain genetically-encoded pathways, genetic risk factors that were identified in Europeans did not improve the prediction of fracture rate in Chinese populations (36). By contrast, 20% of the BMD variation in the genetic studies can be explained by the more common genetic variants [as opposed to risk factors like body mass index (BMI) or age which contribute only 8%] (37), suggesting that genetic factors do play some significant role in the disease process (12). Therefore, while we know by fact that different genetic loci can be implicated in specific populations, the lack of direct comparison between Chinese and Caucasian populations partly limits our inferences on prevention strategies.
Epigenetic factors
A healthier lifestyle (as calculated by the healthy lifestyle scores which includes smoking, BMI, physical activity, diet, alcohol, sleep and anxiety) has been shown to be associated with greater BMD (38). Many other factors, such as vitamin D and calcium levels have been generally accepted and included in guidelines on prevention of osteoporosis and general bone health. However, apart from homocysteine levels, no other clinically relevant risk factors (such as rheumatoid arthritis, vitamin D, calcium intake, cardiovascular disease or diabetes) had a statistically significant effect on fracture risk (14).
The impact of lifestyle factors can be queried because of the finding that, over a 5-year follow-up period, Chinese Canadians retained more BMD than Caucasian Canadians (39). This suggests that, even upon immigration from one country or region to another, the lower risk of osteoporotic fractures seen in the Chinese population remains. Various epigenetic factors, which are involved in the regulation of almost all cells in the bone marrow, could for instance indicate that the decrease in bone mass seen in osteoporosis depends from the differentiation of precursor cells towards an adipocyte lineage as opposed to the bone-forming osteoblasts (28). The three key epigenetic regulators involved in this process are DNA methylation, histone modifications and regulatory action of non-coding (nc)RNA (Table 2, Figure 3) (25).
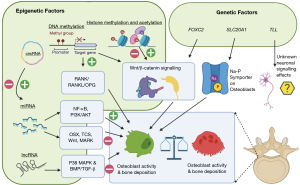
There are number of genes which, when methylated by DNA methyltransferases (DNMTs), up- or downregulate osteogenic differentiation, and by extension affect the risk of developing osteoporosis. In this way, methylation inhibitors like 5-Aza-C have been used to demethylate DLX5 and OSX promoters, increasing the expression of markers of osteogenesis (40). These, among others like RUNX2 (22) and ESR1 (41), are genes that promote osteogenic differentiation when hypomethylated. Conversely, some pathways have the reverse effect and promote bone resorptive or osteoclastic differentiation when in a hypermethylated state, such as RANKL (part of the key OPG/RANKL/RANK signalling pathway), SOST (strongly correlated with fracture risk) (23,42) and Alu elements (43). The last of these, Alu elements, which contribute to variable splicing and transcription regulation were increased during periods of rapid growth in children (44). This, much like peak bone mass, could be an early marker of future bone health and osteoporotic fracture risk, paving a potential new approach to risk stratification.
Other epigenetic factors that could influence osteoporotic fracture risk are related to post-translational histone modifications, through changes in acetylation or methylation. Acetylation levels are controlled by a balance of the action of a pro-acetylation and a de-acetylation enzyme, histone acetyltransferase (HAT) and a histone deacetylase (HDAC), respectively (24,25). Through inhibition studies, HDACs have been shown to regulate osteoblast differentiation, with some cross-over with genes affected by DNA methylation like RUNX2 (45). Alternatively, histones can be methylated to different extents too. For example, the histone demethylase KDM5A exerts an effect on RUNX2 and inhibits osteoblast differentiation, whereas another demethylase (26), JMJD3 targets HOX and promotes their differentiation (46). This variety shows how complex, and difficult, it may be to arrive at one pathway to stratify risk between different groups.
The last of the three families involved in epigenetic regulation are non-coding RNAs. These span in variety from long non-coding (lnc)RNAs and micro (mi)RNAs to circular (circ)RNAs (25). In osteoporotic postmenopausal women, several lncRNAs were found to regulate osteoclast differentiation, potentially contributing to osteoporosis by affecting mRNA expression (27,47). Their effects are varied: depending on the target genes, some promote, and some inhibit osteoblast differentiation. Furthermore, miRNAs regulate genes that affect osteogenic differentiation and they do so with even more variety [almost 40 have been identified (25)]. Lastly, the relatively novel circRNAs have proven their involvement in many aspects of genetic regulation, practically at each step by targeting miRNAs themselves (29); however, the contribution of circRNAs to bone metabolism and their direct relevance in osteoporotic fractures is not yet clear.
Biomarkers
Whilst environmental factors are difficult to characterise and evaluate reliably, the elucidation of the pathways potentially contributing to osteoporotic fracture via genetic and epigenetic mechanisms suggests a potential ground for identification of useful biomarkers. This could enable more precise ways of comparing and estimating the burden of spinal fragility fractures between different populations.
Previously, conventional markers of bone metabolism and turnover would have been used; more recently, novel markers are being evaluated, such as the aforementioned miRNAs. Some of the biomarkers have mapped to specific populations: miR-133a was upregulated in those with low BMD in postmenopausal Caucasian women (48), whilst miR-194-5p elevation was correlated with T-scores in Chinese osteoporotic women (49).
What remains to be seen is if a comparison of one marker between different groups to evaluate whether they could partially explain the difference in risk of spinal fragility fractures. These miRNAs are also part of a wider drive of RNA therapies for various disorders, although bone-specific miRNAs are yet to enter clinical trials. Alternatively, novel genome-wide DNA methylation analysis could enable the characterisation of biomarkers of osteoporosis peripherally, in the blood.
Whilst some studies did not find significant methylation patterns in the blood (50), a study of methylation in whole blood samples of Canadian postmenopausal osteoporotic women showed that it is possibly to detect markers associated with osteoporosis in its early stages in white blood cells (51). However, as we are considering spinal fragility fractures, it is important to note that DNA methylation patterns vary between, and are specific to, different tissues; the blood may not reveal these markers effectively (25). Going forward, adjustments in the T-score cut-offs based on biomarkers could help improve characterisation of an individual’s risk (52).
Imaging
In addition to biomarkers, innovative imaging approaches are being developed to better detect osteoporosis and subsequent vertebral fractures (Table 3). The aim is to achieve both earlier detection and to improve post-fracture screening. At present, the commonest modalities with the most data and experience are classical radiography for fracture detection and dual-energy X-ray absorptiometry (DXA) for BMD measurement. Despite its limitations in the overestimation of spinal BMD in older patients with sclerotic lesions which obfuscate the BMD value (54), DXA is still the current gold standard with several established guidelines (55). More recently, new data can be extracted from DXA images, including a trabecular bone score (TBS) which evaluates not just the BMD, but also the bone quality (56).
Table 3
Technique | Technique details and hallmarks | Advantages | Disadvantages |
---|---|---|---|
TBS | • Quantifies changes from adjacent pixels in grey-level texture | • Correlated with fracture risk in older males | • Cannot be used in isolation yet |
• Low TBS suggests increased fracture risk | • Complementary to BMD | • Age-dependent, and insensitive between 30–45 years | |
• Derived from existing DXA scans | • Not a direct measure of bone microarchitecture | ||
QCT | • Osteoporosis—vBMD <80 mg/cm3 | • Differentiates osteoporosis from other pathologies (e.g., tumour) | • Higher radiation dose (cf. DXA) |
• Severe osteoporosis—vBMD <80 mg/cm3 + insufficiency fracture | • Not affected by scoliosis, weight, degeneration (cf. DXA) | ||
• Ability to measure BMD in a specific volume (vBMD) | |||
HR-pQCT | • Assesses vBMD as well as trabecular vs cortical BMD | • Shows correlation with QCT values in spine, could be used to infer central BMD despite being a peripheral scan | • Restricted to the peripheral skeleton so not as useful for spinal fracture evaluation |
• Individual trabecula segmentation to quantify the ratio of rod-like to plate-like | • Can give very low effective dose and avoid radiosensitive organs | ||
Spectral CT and dual-energy CT | • Two oscillator elements with a photodiode providing spectral information | • Can differentiate tissue (e.g., water and fat) with material-specific values | • Higher radiation dose than QCT |
MRI | • Changes in trabeculae from plate-like to rod-like associated vertebral disease risk (53) | • Avoids ionising radiation exposure | • Signal lacks standardisation |
• Can show early changes in bone marrow (cf. CT or X-ray) | • Direct signal not diagnostic | ||
• Can discern subtle fracture from other pathologies | |||
Novel MRI sequences | • BMFF by chemical shift for example with modified Dixon sequence | • Quantifies osteoporosis through bone architecture | • Cannot directly measure BMD |
• DWI for water diffusion | • Potential of effective integration with AI approaches in the future | • Practicalities—available technology and cost | |
• Ultrashort TE for cortical bone | |||
Bone scintigraphy | • Radiotracer uptake increased in a linear pattern | • Cheaper than PET | • Is only able to provide 2D information |
• Allows bone metabolism visualisation (cf. X-ray/CT) | |||
SPECT, SPECT/CT and PET/MRI | • Hot spots with radioactive tracer uptake then computationally quantified | • Detect and differentiate different causes of secondary osteoporosis | • Cannot be used for primary osteoporosis diagnosis |
• Provides 3D information (cf. traditional scintigraphy) | • Should be combined with an existing QCT integrated scanner |
TBS, trabecular bone score; BMD, bone mineral density; DXA, dual-energy X-ray absorptiometry; QCT, quantitative computed tomography; vBMD, volumetric measurement of BMD (rather than area-based); HR-pQCT, high-resolution peripheral quantitative computed tomography; CT, computed tomography; MRI, magnetic resonance imaging; BMFF, bone marrow fat fraction; DWI, diffusion-weighted imaging; TE, time to echo; AI, artificial intelligence; PET, positron emission tomography; SPECT, single-photon emission computed tomography.
Quantitative computed tomography (QCT) scans can also be used in the assessment of the lumbar spine for spinal fragility fractures; they have several advantages over DXA. Overall, they are equal if not better at assessing BMD than DXA methods, however only in postmenopausal women (57). QCT has the advantage of generating a volumetric (rather than area-based) measurement of BMD (vBMD) and it can measure purely trabecular bone (which is more metabolically active than cortical bone and hence can be a better predictor of bone health and fracture risk than DXA). At present, DXA is still preferred for the spine over QCT because of the smaller radiation dose it delivers. Alternatively, QCT scanning could be carried out at the time of the injury (to prevent loss to follow-up) or as part of a ‘opportunistic’ screening programme in those being scanned for other reasons (58). More recently, high resolution peripheral QCT (HR-pQCT) scanners have been evaluated and showed greater spatial resolution. Whilst HR-pQCT is restricted to the peripheral skeleton, it shows a correlation with QCT values in the lumbar spine, suggesting it could be used to infer central BMD (59). In contrast to computed tomography (CT), the lack of ionising radiation is key advantage of magnetic resonance imaging (MRI) scanning. MRI has shown to perform just as well in imaging trabeculated bone as QCT and HR-pQCT (60). More recently, ultra-high-field MRI was used to show that vertebral bone microarchitecture changes are strongly correlated with parameters such as failure load and stress (61). Whilst high-resolution CT and MRI imaging can evaluate the microarchitecture of bone on a finer scale, their use is not yet established in practice—due to cost and practicality and the novelty of these approaches. By way of example, diagnosing patients with the WHO classification can only be done at present with BMD and T-scores obtained from DXA (62).
Looking to the future, several emerging technologies as well as novel sequences show promise for evaluating not just the bone structure and BMD, but also the bone quality (4). For example, measuring the bone marrow fat fraction (BMFF) with the modified Dixon MRI sequence predicted abnormal bone density (63), which has now improved further with deep learning integration (64). In another approach, MRI T2* mapping of vertebral bone marrow was used to differentiate between those with low-energy and high-energy traumatic vertebral fractures, potentially paving a way for use in fracture risk evaluation (65). In terms of improvements on CT, novel spectral CT and dual-energy CT are also being evaluated as they are additionally able to better distinguish different tissues, however, their clinical value is still uncertain (4).
With regard to nuclear medicine bone scans, it is well known that they could answer different clinical questions, for instance they can be employed to monitor the response of compression fractures to therapy. These scans include bone scintigraphy, single-photon emission computed tomography (SPECT), SPECT/CT as well as positron emission tomography (PET)/MRI. Whilst nuclear bone scans can be used to differentiate between different causes of secondary osteoporosis, they are not used for the diagnosis of primary osteoporosis (4). Nevertheless, scintigraphy has been shown to detect a greater percentage of vertebral fractures than conventional radiography and, additionally, could also differentiate between old and new fractures (66).
Finally, in the pre-clinical sphere, vibro-acoustography (a technique based on ultrasound radiation) was able to differentiate between osteoporosis and control rodent groups; yet another potential future screening tool (67).
In summary, whilst radiographic and DXA scans are the norm for detecting vertebral fractures and evaluating BMD, they face competition from emerging adaptations to existing technologies like MRI and CT, with new sequences or new combined scanning protocols. The addition of artificial intelligence (AI) to these data sets will enable, in future clinical practice, the extraction of even richer data and the ability to predict fracture risk (68). Whilst the hunt for a biosignature of spinal fragility fractures or osteoporosis continues, the finding that HR-pQCT measurements of bone architecture and vBMD are heritable across a number of generations gives further incentive to look for specific signatures of reduced vBMD and try to elucidate the genetic and epigenetic factors contributing to them (69).
Lastly, it is important to consider that improving imaging techniques, potentially with the help of recent advances in nanomedicine with regards to techniques meant to optimize the of role of contrast media and radiotracers (70), which are directly translated from strategies to enhance the bioavailability of chemotherapics (71), is just one of the future hopes of early diagnosis in patients with osteoporosis. Identifying this condition early is just the beginning of a long and complex management course: equally important will be an efficient follow-up imaging to detect subsequent fragility fractures with a close comparison of previous imaging, even in those that have been treated medically or surgically with vertebroplasty (72).
Conclusions and future directions
The genetic and epigenetic landscape for spinal fragility fracture risk is being investigated, with several spine-specific loci linked to BMD and metabolism having been identified in GWAS studies. Only two loci have been found which directly associate with vertebral fracture risk. Studies comparing the prevalence of these loci in different populations are needed to understand if they underpin the risk differences, such as that existing between East Asian and Caucasian cohorts.
When analysing this risk, it is important to consider the particularities of how osteoporosis and fragility fracture prevalence and incidence are estimated across different countries or regions. For example, there is an argument to be made that using the traditional cut-off T-score of −2.5 is not appropriate in all populations; it can be argued that the population in certain Asian countries or regions has a different risk profile to Caucasian populations and hence should have their diagnostic cut-offs and modelling of fracture prevalence tailored more appropriately (73-75). Using the −2.5 cut-off score can therefore greatly overestimate the prevalence of osteoporosis and make projections about fragility fractures seem inflated (73). It is therefore important to analyse the methodology used to project these figures (6,76,77). Whilst in Figure 1A we highlight the incidence of fragility fractures (a directly measurable quantity), the projections used in prevalence estimates for osteoporosis can be flawed if they use the −2.5 T-score cut-off in populations where this may not be appropriate (Figure 1B). Recently, more appropriate T-score cut-offs for several Asian populations have been suggested to more appropriately represent the burden of osteoporosis and vertebral fractures in those countries (Figure 2) (7,73,78). In addition, the incidence rates reported in different regions are clearly highly affected by the healthcare systems in place there, particularly relating to access to healthcare and appropriate diagnostic investigations.
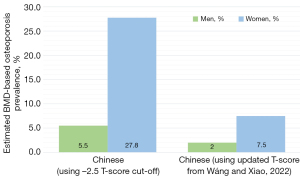
Lifestyle factors, whilst widely discussed as being important for general bone health, do not reach the same statistical significance to explain these variations. However, epigenetic factors are being elucidated extensively, and are showing promise both in understanding risk profiles, at detecting risk peripherally in the blood as biomarkers, but also as potential future therapies. This information will help clinicians in obtaining a better diagnosis and enable tighter treatment monitoring; advancements in imaging techniques and data extraction from existing scans will further accelerate the exiting translational path for patients harbouring OVF.
In terms of potential future directions of research, there is a current pertinent needed for an integrated multi-omics approach that can bring together the various advances from imaging and (epi)genomics that we have highlighted in order to generate a practical tool for clinicians to improve management and outcomes for patients (11,53,79). The benefit of this would be two-fold; first it can decrease the necessity for duplicating research efforts, and secondly it would bring together what are at present a complex interplay of various factors. Rather than interpreting these various genetic and epigenetic factors, biomarkers and imaging findings in isolation, an integrated approach requiring an international effort could be particularly useful.
Acknowledgments
Funding: None.
Footnote
Conflicts of Interest: All authors have completed the ICMJE uniform disclosure form (available at https://qims.amegroups.com/article/view/10.21037/qims-23-513/coif). MG serves as an unpaid editorial board member of Quantitative Imaging in Medicine and Surgery. The other authors have no conflicts of interest to declare.
Ethical Statement: The authors are accountable for all aspects of the work in ensuring that questions related to the accuracy or integrity of any part of the work are appropriately investigated and resolved.
Open Access Statement: This is an Open Access article distributed in accordance with the Creative Commons Attribution-NonCommercial-NoDerivs 4.0 International License (CC BY-NC-ND 4.0), which permits the non-commercial replication and distribution of the article with the strict proviso that no changes or edits are made and the original work is properly cited (including links to both the formal publication through the relevant DOI and the license). See: https://creativecommons.org/licenses/by-nc-nd/4.0/.
References
- Wáng YXJ, Diacinti D, Leung JCS, Iannacone A, Kripa E, Kwok TCY, Diacinti D. Further evidence supports a much lower prevalence of radiographic osteoporotic vertebral fracture in Hong Kong Chinese women than in Italian Caucasian women. Arch Osteoporos 2022;17:13. [Crossref] [PubMed]
- Wáng YXJ. Fragility fracture prevalence among elderly Chinese is no more than half of that of elderly Caucasian. Quant Imaging Med Surg 2022;12:874-81. [Crossref] [PubMed]
- Wáng YXJ, Deng M, Griffith JF, Kwok AWL, Leung JCS, Lam PMS, et al. ‘Healthier Chinese spine’: an update of osteoporotic fractures in men (MrOS) and in women (MsOS) Hong Kong spine radiograph studies. Quant Imaging Med Surg 2022;12:2090-105. [Crossref] [PubMed]
- Cheng X, Yuan H, Cheng J, Weng X, Xu H, Gao J, Huang M, Wáng YXJ, Wu Y, Xu W, Liu L, Liu H, Huang C, Jin Z, Tian W. Chinese expert consensus on the diagnosis of osteoporosis by imaging and bone mineral density. Chinese expert consensus on the diagnosis of osteoporosis by imaging and bone mineral density. Quant Imaging Med Surg 2020;10:2066-77. [Crossref] [PubMed]
- Shen Y, Huang X, Wu J, Lin X, Zhou X, Zhu Z, et al. The Global Burden of Osteoporosis, Low Bone Mass, and Its Related Fracture in 204 Countries and Territories, 1990-2019. Front Endocrinol 2022;13: [Internet].
- Ballane G, Cauley JA, Luckey MM, El-Hajj Fuleihan G. Worldwide prevalence and incidence of osteoporotic vertebral fractures. Osteoporos Int 2017;28:1531-42. [Crossref] [PubMed]
- Wáng YXJ, Xiao BH. Estimations of bone mineral density defined osteoporosis prevalence and cutpoint T-score for defining osteoporosis among older Chinese population: a framework based on relative fragility fracture risks. Quant Imaging Med Surg 2022;12:4346-60. [Crossref] [PubMed]
- Koromani F, Trajanoska K, Rivadeneira F, Oei L. Recent Advances in the Genetics of Fractures in Osteoporosis. Front Endocrinol (Lausanne) 2019;10:337. [Crossref] [PubMed]
- Zhu X, Bai W, Zheng H. Twelve years of GWAS discoveries for osteoporosis and related traits: advances, challenges and applications. Bone Res 2021;9:23. [Crossref] [PubMed]
- Oei L, Estrada K, Duncan EL, Christiansen C, Liu CT, Langdahl BL, et al. Genome-wide association study for radiographic vertebral fractures: a potential role for the 16q24 BMD locus. Bone 2014;59:20-7.
- Stankiewicz P, Sen P, Bhatt SS, Storer M, Xia Z, Bejjani BA, et al. Genomic and genic deletions of the FOX gene cluster on 16q24.1 and inactivating mutations of FOXF1 cause alveolar capillary dysplasia and other malformations. Am J Hum Genet 2009;84:780-91. [Crossref] [PubMed]
- Alonso N, Estrada K, Albagha OME, Herrera L, Reppe S, Olstad OK, et al. Identification of a novel locus on chromosome 2q13, which predisposes to clinical vertebral fractures independently of bone density. Ann Rheum Dis 2018;77:378-85. [Crossref] [PubMed]
- Trajanoska K, Rivadeneira F. The genetic architecture of osteoporosis and fracture risk. Bone 2019;126:2-10. [Crossref] [PubMed]
- Trajanoska K, Morris JA, Oei L, Zheng HF, Evans DM, Kiel DP, Ohlsson C, Richards JB, Rivadeneira F. GEFOS/GENOMOS consortium and the 23andMe research team. Assessment of the genetic and clinical determinants of fracture risk: genome wide association and mendelian randomisation study. BMJ 2018;362:k3225. [Crossref] [PubMed]
- Kung AW, Xiao SM, Cherny S, Li GH, Gao Y, Tso G, et al. Association of JAG1 with bone mineral density and osteoporotic fractures: a genome-wide association study and follow-up replication studies. Am J Hum Genet 2010;86:229-39. [Crossref] [PubMed]
- Zhu X, Zheng H. Factors influencing peak bone mass gain. Front Med 2021;15:53-69. [Crossref] [PubMed]
- Chesi A, Mitchell JA, Kalkwarf HJ, Bradfield JP, Lappe JM, Cousminer DL, Roy SM, McCormack SE, Gilsanz V, Oberfield SE, Hakonarson H, Shepherd JA, Kelly A, Zemel BS, Grant SF. A Genomewide Association Study Identifies Two Sex-Specific Loci, at SPTB and IZUMO3, Influencing Pediatric Bone Mineral Density at Multiple Skeletal Sites. J Bone Miner Res 2017;32:1274-81. [Crossref] [PubMed]
- Zheng HF, Forgetta V, Hsu YH, Estrada K, Rosello-Diez A, Leo PJ, et al. Whole-genome sequencing identifies EN1 as a determinant of bone density and fracture. Nature 2015;526:112-7. [Crossref] [PubMed]
- Medina-Gomez C, Kemp JP, Trajanoska K, Luan J, Chesi A, Ahluwalia TS, et al. Life-Course Genome-wide Association Study Meta-analysis of Total Body BMD and Assessment of Age-Specific Effects. Am J Hum Genet 2018;102:88-102. [Crossref] [PubMed]
- Roy D, Swarbrick C, King Y, Pye S, Adams J, Berry J, Silman A, O'Neill T. Differences in peak bone mass in women of European and South Asian origin can be explained by differences in body size. Osteoporos Int 2005;16:1254-62. [Crossref] [PubMed]
- Gregson CL, Duncan EL. The Genetic Architecture of High Bone Mass. Front Endocrinol [Internet]. 2020 [cited 2022 Feb 15];11. Available online: https://www.frontiersin.org/article/10.3389/fendo.2020.595653
- Zhang RP, Shao JZ, Xiang LX. GADD45A protein plays an essential role in active DNA demethylation during terminal osteogenic differentiation of adipose-derived mesenchymal stem cells. J Biol Chem 2011;286:41083-94. [Crossref] [PubMed]
- Cao Y, Yang H, Jin L, Du J, Fan Z. Genome-Wide DNA Methylation Analysis during Osteogenic Differentiation of Human Bone Marrow Mesenchymal Stem Cells. Stem Cells Int 2018;2018:8238496. [Crossref] [PubMed]
- Piao J, Tsuji K, Ochi H, Iwata M, Koga D, Okawa A, Morita S, Takeda S, Asou Y. Sirt6 regulates postnatal growth plate differentiation and proliferation via Ihh signaling. Sci Rep 2013;3:3022. [Crossref] [PubMed]
- Xu F, Li W, Yang X, Na L, Chen L, Liu G. The Roles of Epigenetics Regulation in Bone Metabolism and Osteoporosis. Front Cell Dev Biol 2021;8: [Internet].
- Flowers S, Beck GR Jr, Moran E. Transcriptional activation by pRB and its coordination with SWI/SNF recruitment. Cancer Res 2010;70:8282-7. [Crossref] [PubMed]
- Li Y, Li J, Chen L, Xu L. The Roles of Long Non-coding RNA in Osteoporosis. Curr Stem Cell Res Ther 2020;15:639-45. [Crossref] [PubMed]
- Letarouilly JG, Broux O, Clabaut A. New insights into the epigenetics of osteoporosis. Genomics 2019;111:793-8. [Crossref] [PubMed]
- Dou C, Cao Z, Yang B, Ding N, Hou T, Luo F, Kang F, Li J, Yang X, Jiang H, Xiang J, Quan H, Xu J, Dong S. Changing expression profiles of lncRNAs, mRNAs, circRNAs and miRNAs during osteoclastogenesis. Sci Rep 2016;6:21499. [Crossref] [PubMed]
- Al Kaissi A, Scholl-Buergi S, Biedermann R, Maurer K, Hofstaetter JG, Klaushofer K, Grill F. The diagnosis and management of patients with idiopathic osteolysis. Pediatr Rheumatol Online J 2011;9:31. [Crossref] [PubMed]
- Mumm S, Huskey M, Duan S, Wenkert D, Madson KL, Gottesman GS, Nenninger AR, Laxer RM, McAlister WH, Whyte MP. Multicentric carpotarsal osteolysis syndrome is caused by only a few domain-specific mutations in MAFB, a negative regulator of RANKL-induced osteoclastogenesis. Am J Med Genet A 2014;164A:2287-93. [Crossref] [PubMed]
- Ganau M, Prasad V, Ligarotti GKI, Syrmos NC, Ellamushi H. An overview of the neurosurgical implications, pathophysiology, diagnosis and recent treatment strategies for Grade IV idiopathic osteolysis, also known as Gorham-Stout or phantom bone disease. Hell J Nucl Med 2018;21:198-201. [Crossref] [PubMed]
- Liang Y, Tian R, Wang J, Shan Y, Gao H, Xie C, Li J, Xu M, Gu S. Gorham-Stout disease successfully treated with sirolimus (rapamycin): a case report and review of the literature. BMC Musculoskelet Disord 2020;21:577. [Crossref] [PubMed]
- Kobayashi T, Nakamura Y, Suzuki T, Yamaguchi T, Takeda R, Takagi M, Hasegawa T, Kosho T, Kato H. Efficacy and Safety of Denosumab Therapy for Osteogenesis Imperfecta Patients with Osteoporosis-Case Series. J Clin Med 2018;7:479. [Crossref] [PubMed]
- Cummings SR, San Martin J, McClung MR, Siris ES, Eastell R, Reid IR, Delmas P, Zoog HB, Austin M, Wang A, Kutilek S, Adami S, Zanchetta J, Libanati C, Siddhanti S, Christiansen C. FREEDOM Trial. Denosumab for prevention of fractures in postmenopausal women with osteoporosis. N Engl J Med 2009;361:756-65. [Crossref] [PubMed]
- Li YM, Peng C, Zhang JG, Zhu W, Xu C, Lin Y, Fu XY, Tian Q, Zhang L, Xiang Y, Sheng V, Deng HW. Genetic risk factors identified in populations of European descent do not improve the prediction of osteoporotic fracture and bone mineral density in Chinese populations. Sci Rep 2019;9:6086. [Crossref] [PubMed]
- Valero C, Zarrabeitia MT, Hernández JL, Zarrabeitia A, González-Macías J, Riancho JA. Bone mass in young adults: relationship with gender, weight and genetic factors. J Intern Med 2005;258:554-62. [Crossref] [PubMed]
- Deng YY, Liu YP, Ling CW, Li YH, Wu YY, Ke YB, Chen YM. Higher healthy lifestyle scores are associated with greater bone mineral density in middle-aged and elderly Chinese adults. Arch Osteoporos 2020;15:129. [Crossref] [PubMed]
- Morin SN, Berger C, Liu W, Prior JC, Cheung AM, Hanley DA, Boyd SK, Wong AKO, Papaioannou A, Rahme E, Goltzman D. CaMos Research Group. Differences in fracture prevalence and in bone mineral density between Chinese and White Canadians: the Canadian Multicentre Osteoporosis Study (CaMos). Arch Osteoporos 2020;15:147. [Crossref] [PubMed]
- Farshdousti Hagh M, Noruzinia M, Mortazavi Y, Soleimani M, Kaviani S, Abroun S, Dehghani Fard A, Mahmoodinia M. Different Methylation Patterns of RUNX2, OSX, DLX5 and BSP in Osteoblastic Differentiation of Mesenchymal Stem Cells. Cell J 2015;17:71-82. [Crossref] [PubMed]
- Penolazzi L, Lambertini E, Giordano S, Sollazzo V, Traina G, del Senno L, Piva R. Methylation analysis of the promoter F of estrogen receptor alpha gene: effects on the level of transcription on human osteoblastic cells. J Steroid Biochem Mol Biol 2004;91:1-9. [Crossref] [PubMed]
- Reppe S, Noer A, Grimholt RM, Halldórsson BV, Medina-Gomez C, Gautvik VT, Olstad OK, Berg JP, Datta H, Estrada K, Hofman A, Uitterlinden AG, Rivadeneira F, Lyle R, Collas P, Gautvik KM. Methylation of bone SOST, its mRNA, and serum sclerostin levels correlate strongly with fracture risk in postmenopausal women. J Bone Miner Res 2015;30:249-56. [Crossref] [PubMed]
- Jintaridth P, Tungtrongchitr R, Preutthipan S, Mutirangura A. Hypomethylation of Alu elements in post-menopausal women with osteoporosis. PLoS One 2013;8:e70386. [Crossref] [PubMed]
- Rerkasem K, Rattanatanyong P, Rerkasem A, Wongthanee A, Rungruengthanakit K, Mangklabruks A, Mutirangura A. Higher Alu methylation levels in catch-up growth in twenty-year-old offsprings. PLoS One 2015;10:e0120032. [Crossref] [PubMed]
- Kim JH, Kim K, Youn BU, Jin HM, Kim JY, Moon JB, Ko A, Seo SB, Lee KY, Kim N. RANKL induces NFATc1 acetylation and stability via histone acetyltransferases during osteoclast differentiation. Biochem J 2011;436:253-62. [Crossref] [PubMed]
- Ye L, Fan Z, Yu B, Chang J, Al Hezaimi K, Zhou X, Park NH, Wang CY. Histone demethylases KDM4B and KDM6B promotes osteogenic differentiation of human MSCs. Cell Stem Cell 2012;11:50-61. [Crossref] [PubMed]
- Fei Q, Bai X, Lin J, Meng H, Yang Y, Guo A. Identification of aberrantly expressed long non-coding RNAs in postmenopausal osteoporosis. Int J Mol Med 2018;41:3537-50. [Crossref] [PubMed]
- Wang Y, Li L, Moore BT, Peng XH, Fang X, Lappe JM, Recker RR, Xiao P. MiR-133a in human circulating monocytes: a potential biomarker associated with postmenopausal osteoporosis. PLoS One 2012;7:e34641. [Crossref] [PubMed]
- Meng J, Zhang D, Pan N, Sun N, Wang Q, Fan J, Zhou P, Zhu W, Jiang L. Identification of miR-194-5p as a potential biomarker for postmenopausal osteoporosis. PeerJ 2015;3:e971. [Crossref] [PubMed]
- Fernandez-Rebollo E, Eipel M, Seefried L, Hoffmann P, Strathmann K, Jakob F, Wagner W. Primary Osteoporosis Is Not Reflected by Disease-Specific DNA Methylation or Accelerated Epigenetic Age in Blood. J Bone Miner Res 2018;33:356-61. [Crossref] [PubMed]
- Cheishvili D, Parashar S, Mahmood N, Arakelian A, Kremer R, Goltzman D, Szyf M, Rabbani SA. Identification of an Epigenetic Signature of Osteoporosis in Blood DNA of Postmenopausal Women. J Bone Miner Res 2018;33:1980-9. [Crossref] [PubMed]
- Wáng YXJ, Xiao BH, Su Y, Leung JCS, Lam PMS, Kwok TCY. Fine-tuning the cutpoint T-score as an epidemiological index with high specificity for osteoporosis: methodological considerations for the Chinese population. Quant Imaging Med Surg 2022;12:882-5. [Crossref] [PubMed]
- Wehrli FW, Gomberg BR, Saha PK, Song HK, Hwang SN, Snyder PJ. Digital topological analysis of in vivo magnetic resonance microimages of trabecular bone reveals structural implications of osteoporosis. J Bone Miner Res 2001;16:1520-31. [Crossref] [PubMed]
- Bolotin HH. DXA in vivo BMD methodology: an erroneous and misleading research and clinical gauge of bone mineral status, bone fragility, and bone remodelling. Bone 2007;41:138-54. [Crossref] [PubMed]
- Guerri S, Mercatelli D, Aparisi Gómez MP, Napoli A, Battista G, Guglielmi G, Bazzocchi A. Quantitative imaging techniques for the assessment of osteoporosis and sarcopenia. Quant Imaging Med Surg 2018;8:60-85. [Crossref] [PubMed]
- Oei L, Koromani F, Rivadeneira F, Zillikens MC, Oei EH. Quantitative imaging methods in osteoporosis. Quant Imaging Med Surg 2016;6:680-98. [Crossref] [PubMed]
- Engelke K, Lang T, Khosla S, Qin L, Zysset P, Leslie WD, Shepherd JA, Schousboe JT. Clinical Use of Quantitative Computed Tomography (QCT) of the Hip in the Management of Osteoporosis in Adults: the 2015 ISCD Official Positions-Part I. J Clin Densitom 2015;18:338-58. [Crossref] [PubMed]
- Ziemlewicz TJ, Binkley N, Lubner MG, Pickhardt PJ. Opportunistic Osteoporosis Screening. J Am Coll Radiol JACR 2015;12:1036-41. [Crossref] [PubMed]
- Liu XS, Cohen A, Shane E, Yin PT, Stein EM, Rogers H, Kokolus SL, McMahon DJ, Lappe JM, Recker RR, Lang T, Guo XE. Bone density, geometry, microstructure, and stiffness: Relationships between peripheral and central skeletal sites assessed by DXA, HR-pQCT, and cQCT in premenopausal women. J Bone Miner Res 2010;25:2229-38. [Crossref] [PubMed]
- Krug R, Carballido-Gamio J, Burghardt AJ, Kazakia G, Hyun BH, Jobke B, Banerjee S, Huber M, Link TM, Majumdar S. Assessment of trabecular bone structure comparing magnetic resonance imaging at 3 Tesla with high-resolution peripheral quantitative computed tomography ex vivo and in vivo. Osteoporos Int 2008;19:653-61. [Crossref] [PubMed]
- Guenoun D, Fouré A, Pithioux M, Guis S, Le Corroller T, Mattei JP, Pauly V, Guye M, Bernard M, Chabrand P, Champsaur P, Bendahan D. Correlative Analysis of Vertebral Trabecular Bone Microarchitecture and Mechanical Properties: A Combined Ultra-high Field (7 Tesla) MRI and Biomechanical Investigation. Spine (Phila Pa 1976) 2017;42:E1165-72. [Crossref] [PubMed]
- Messina C, Maffi G, Vitale JA, Ulivieri FM, Guglielmi G, Sconfienza LM. Diagnostic imaging of osteoporosis and sarcopenia: a narrative review. Quant Imaging Med Surg 2018;8:86-99. [Crossref] [PubMed]
- Zhao Y, Huang M, Ding J, Zhang X, Spuhler K, Hu S, Li M, Fan W, Chen L, Zhang X, Li S, Zhou Q, Huang C. Prediction of Abnormal Bone Density and Osteoporosis From Lumbar Spine MR Using Modified Dixon Quant in 257 Subjects With Quantitative Computed Tomography as Reference. J Magn Reson Imaging 2019;49:390-9. [Crossref] [PubMed]
- Zhao Y, Zhao T, Chen S, Zhang X, Serrano Sosa M, Liu J, Mo X, Chen X, Huang M, Li S, Zhang X, Huang C. Fully automated radiomic screening pipeline for osteoporosis and abnormal bone density with a deep learning-based segmentation using a short lumbar mDixon sequence. Quant Imaging Med Surg 2022;12:1198-213. [Crossref] [PubMed]
- Leonhardt Y, Gassert FT, Feuerriegel G, Gassert FG, Kronthaler S, Boehm C, Kufner A, Ruschke S, Baum T, Schwaiger BJ, Makowski MR, Karampinos DC, Gersing AS. Vertebral bone marrow T2* mapping using chemical shift encoding-based water-fat separation in the quantitative analysis of lumbar osteoporosis and osteoporotic fractures. Quant Imaging Med Surg 2021;11:3715-25. [Crossref] [PubMed]
- Dugonjić S, Ajdinović B, Ćirković M, Ristić G. Bone scintigraphy can diagnose osteoporotic vertebral compression fractures better than conventional radiography. Hell J Nucl Med 2017;20:155.
- Agnollitto PM, de Araújo Braz G, Spirlandeli AL, de Paula FJA, Carneiro AAO, Nogueira-Barbosa MH. Ex vivo vibro-acoustography characterization of osteoporosis in an experimental mice model. Quant Imaging Med Surg 2021;11:586-96. [Crossref] [PubMed]
- Ferizi U, Besser H, Hysi P, Jacobs J, Rajapakse CS, Chen C, Saha PK, Honig S, Chang G. Artificial Intelligence Applied to Osteoporosis: A Performance Comparison of Machine Learning Algorithms in Predicting Fragility Fractures From MRI Data. J Magn Reson Imaging 2019;49:1029-38. [Crossref] [PubMed]
- Karasik D, Demissie S, Zhou Y, Lu D, Broe KE, Bouxsein ML, Cupples LA, Kiel DP. Heritability and Genetic Correlations for Bone Microarchitecture: The Framingham Study Families. J Bone Miner Res 2017;32:106-14. [Crossref] [PubMed]
- Ganau M, Syrmos NC, D'Arco F, Ganau L, Chibbaro S, Prisco L, Ligarotti GKI, Ambu R, Soddu A. Enhancing contrast agents and radiotracers performance through hyaluronic acid-coating in neuroradiology and nuclear medicine. Hell J Nucl Med 2017;20:166-8. [Crossref] [PubMed]
- Ganau M. Tackling gliomas with nanoformulated antineoplastic drugs: suitability of hyaluronic acid nanoparticles. Clin Transl Oncol 2014;16:220-3. [Crossref] [PubMed]
- Ganau M, Kato S, Oshima Y. Letter to the Editor concerning "Osteoporotic thoracolumbar compression fractures: long-term retrospective comparison between vertebroplasty and conservative treatment" by K. Martikos et al. Eur Spine J 2018;27:1662-3. [Eur Spine J (2018) doi: 101007/s00586-018-5605-1].
- Wáng YXJ. The definition of spine bone mineral density (BMD)-classified osteoporosis and the much inflated prevalence of spine osteoporosis in older Chinese women when using the conventional cutpoint T-score of -2.5. Ann Transl Med 2022;10:1421. [Crossref] [PubMed]
- So TY, Diacinti D, Leung JCS, Iannacone A, Kripa E, Kwok TCY, Diacinti D, Wang YXJ. Lower Prevalence and Severity of Degenerative Changes in the Lumbar Spine in Elderly Hong Kong Chinese Compared With Age-Matched Italian Caucasian Women. Spine (Phila Pa 1976) 2022;47:1710-8. [Crossref] [PubMed]
- Cong E, Walker MD. The Chinese skeleton: insights into microstructure that help to explain the epidemiology of fracture. Bone Res 2014;2:14009. [Crossref] [PubMed]
- Wang L, Yu W, Yin X, Cui L, Tang S, Jiang N, et al. Prevalence of Osteoporosis and Fracture in China: The China Osteoporosis Prevalence Study. JAMA Netw Open 2021;4:e2121106. [Crossref] [PubMed]
- Wang J, Shu B, Tang D, Li C, Xie X, Jiang L, et al. The prevalence of osteoporosis in China, a community based cohort study of osteoporosis. Front Public Health 2023;11: [Internet].
- Wáng YXJ, Diacinti D, Leung JCS, Iannacone A, Kripa E, Kwok TCY, Diacinti D. Conversion of osteoporotic vertebral fracture severity score to osteoporosis T-score equivalent status: a framework and a comparative study of Hong Kong Chinese and Rome Caucasian older women. Arch Osteoporos 2022;18:1. [Crossref] [PubMed]
- Tobias JH, Duncan EL, Kague E, Hammond CL, Gregson CL, Bassett D, et al. Opportunities and Challenges in Functional Genomics Research in Osteoporosis: Report From a Workshop Held by the Causes Working Group of the Osteoporosis and Bone Research Academy of the Royal Osteoporosis Society on October 5th 2020. Front Endocrinol 2021;11: [Internet].