Exploring a simplified way to diagnose pelvic lipomatosis: prediction of pelvic fat volume using a single cross-sectional image
Introduction
Pelvic lipomatosis (PL) is a rare benign disease characterized by an overgrowth of non-encapsulated adipose tissue (AT) in the perivesical and perirectal spaces of unknown etiology (1), firstly described by Engels (2) and introduced by Fogg and Smyth (3). The incidence of PL in the United States was reported as 0.6–1.7 per 100,000 hospital admissions, with a male-to-female ratio of 18:1 and a tendency toward the Black race (1). PL may cause obstruction of the urinary tract, lower intestinal tract, and vascular system. Patients may present with a range of corresponding symptoms, such as pollakiuria, dysuria, nocturia, hematuria, constipation, tenesmus, and edema of the lower extremities. Some 40% of PL patients may develop ureteral obstruction with hydroureteronephrosis within 5 years. Some patients may develop obstructive renal failure in the late stage (4).
The diagnosis of PL mainly depends on clinical symptoms and imaging manifestations. Typical imaging findings include a pear-shaped bladder, elevation of the bladder and sigmoid colon, tubular narrowing of the recto-sigmoid colon, and reduced attenuation of the pelvic soft tissues (5). In our previous study, we measured morphological parameters in 50 PL patients and developed a multiple regression model to predict PL (6). However, with this method, it is rather difficult to make an accurate diagnosis for PL patients without typical morphological changes.
Pelvic fat volume (PFV) is an objective and intuitive index to reflect the content of pelvic AT, with higher sensitivity (SEN), especially in early-stage patients (7), but the practical measurement is complicated, and more precise 3-dimensional (3D) models are still to be established (6). Therefore, we considered a simple alternative with high SEN and specificity (SPE) to help predict the diagnosis of PL. A single abdominal cross-sectional image can relate to total body AT volumes (8) and visceral adipose tissue (VAT) volumes (9). We aimed to investigate relationships between AT areas of a single image and PFV in the study. Based on reproducibility and quantification, AT areas might be useful in the diagnosis of PL. Accordingly, the purpose of this study was to evaluate the feasibility to estimate the value of PFV and the accuracy to predict PL with AT area from a single image. We present this article in accordance with the STARD reporting checklist (available at https://qims.amegroups.com/article/view/10.21037/qims-23-128/rc).
Methods
Clinical data
Patients who underwent computed tomography urography (CTU) examination in Peking Union Medical College Hospital from September 2014 to December 2019 were retrospectively studied. The study was conducted in accordance with the Declaration of Helsinki (as revised in 2013) and approved by the Institutional Review Board of Peking Union Medical College Hospital. The study is a retrospective study and the requirement for written informed consent was waived by the Institutional Review Board of Peking Union Medical College Hospital. The inclusion criteria of cases in the PL group were as follows: (I) CT imaging diagnosis included “pelvic lipomatosis”; (II) clinical diagnosis included “pelvic lipomatosis”; and (III) available images and complete clinical data. A total of 50 consecutive cases were obtained. For 14 patients who underwent more than one CTU examinations, the CT images at the time of the first diagnosis were selected. Among the 50 PL patients, 5 patients were diagnosed with PL by laparoscope and 23 patients were pathologically diagnosed with glandular cystitis after transurethral resection of bladder tumor (TURBT). Controls were matched in a 1:1 ratio to cases, according to the inclusion criteria for the controls: (I) age and sex were the same as the matched cases, (II) normal or diseased without effects on the AT, and (III) the control with the closest examination date to the case’s examination date was selected. A total of 50 controls matched with the PL group were obtained (Figure 1). The demographic characteristics of the study population are summarized in Table 1.
Table 1
Characteristics | Patients (n=50) | Controls (n=50) | P value |
---|---|---|---|
Gender | Male | Male | – |
Age (years) | 42.6±10.7 | 42.6±10.7 | – |
Height (cm) | 171.3±5.4 | 172.9±5.9 | 0.197 |
Weight (kg) | 76.6±11.0 | 76.2±10.9 | 0.879 |
BMI (kg/m2)† | 26.0±3.3 | 25.4±3.0 | 0.416 |
Underweight, <18.5 (n) | 0 | 0 | – |
Normal weight, 18.5–24.9 (n) | 20 | 20 | – |
Overweight, 25.0–29.9 (n) | 20 | 22 | – |
Obese, ≥30.0 (n) | 3 | 4 | – |
Body width (cm) | 57.1±4.0 | 55.9±5.8 | 0.233 |
Characteristics are described using numbers or mean ± standard deviations. †, data for BMI were available in 43 patients of the PL group and 46 patients of the control group. BMI, body mass index; PL, pelvic lipomatosis.
CT examination
All patients underwent CTU examinations using Somatom Definition Flash (Siemens Healthcare, Forchheim, Germany). The patients were placed in the supine position with a scan range from above the hemidiaphragm to the pubic symphysis. Abdominal un-enhanced, nephrographic, and excretory phases were performed. The CT parameters were as follows: slice thickness 1 mm, pitch 0.9, collimation 128×0.6 mm, rotation time 0.28 seconds, kernel filter B30f (medium smooth), tube voltage 120 kV, and the real-time dynamic exposure dose adjustment control system CARE Dose 4D (Siemens). For contrast-enhanced scanning, 90 mL of non-ionic contrast agent (Ultravist 370, Bayer Schering Pharma, Berlin, Germany) was injected with a double-barrel high-pressure syringe at a rate of 4 mL/s, followed by 100 mL of normal saline. The region of interest (ROI) was placed at the junction of descending aorta and abdominal aorta, and the automatic trigger threshold was set at 100 Hounsfield units (HU). After reaching the threshold, the nephrographic phase scan was delayed by 7 seconds and the excretory phase scan was delayed by 55 seconds.
Areas of AT
All patient information was removed from the CT images before proceeding to the analysis. Thin-slice nephrographic phase images were collected from patients and controls. In the abdomen, the L3 vertebra was set as the zero point (L0), and a total of 201 slices were selected with 1 mm intervals (L−100 − L+100), covering the upper margin of the L1 vertebra and L5/S1 disc. In the pelvic cavity, we selected 5 slices, including 4 slices from the anterior superior margin of the S1 vertebra to the anterior superior margin of the S4 vertebra and the section above the bilateral femoral head (FH) (Figure 2A,2B). We utilized the nnU-Net architecture to segment subcutaneous adipose tissue (SAT) and VAT of each section automatically (10). After segmentation, we used the ITK-snap software (http://www.itksnap.org/pmwiki/pmwiki.php) to display the SAT region and the VAT region. We set the SAT region to be displayed in red and the VAT region in green (Figure 3A). Areas of SAT and VAT were measured at each slice in patients and controls.
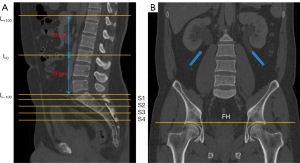
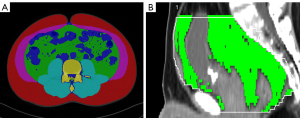
PFV
PFVs of 50 patients and 50 controls were measured by MRIcron (Version 1.0.20190902 for macOS; https://www.nitrc.org/projects/mricron) and Syngo Volume (Siemens) separately. The measurement ranges were from the anterior upper edge of the first sacral vertebra to the pelvic diaphragm (CT values between −150 and −30 HU) (Figure 3B).
Statistical analysis
All data were analyzed using the software SPSS 26.0 (IBM Corp., Armonk, NY, USA). Student’s t-test or rank-sum test were performed to compare the significant differences in areas and volumes between patients and controls. A 2-sided P value <0.05 was considered to be statistically significant. Intraclass correlation coefficient (ICC) of PFV was used to show between-group agreement. The Pearson correlation between the area of each slice and PFV was calculated. The linear regression models were established to estimate PFV and the binary logistic regression models were developed to predict PL with AT areas at different anatomical locations. Receiver operating characteristic (ROC) curves of logistic regression models were calculated to reflect SEN and SPE. The area under the ROC curve (AUC) values indicated the diagnostic efficiency of models.
Results
Participant characteristics
All the patients and controls were male in our study. The heights and weights between patients and controls were not statistically different (P=0.197 and P=0.879 respectively). Among the patients, 3 were obese [body mass index (BMI) ≥30 kg/m2], 20 were overweight (BMI: 25.0–29.9 kg/m2), and 20 were of normal weight (BMI: 18.5–24.9 kg/m2). There was no significant difference in BMI between patients and controls (obese: 4; overweight: 22; normal weight: 20 among controls). Body widths of patients ranged from 49.42 to 65.19 cm with a median value of 57.29 cm, compared to the body widths of controls (44.30–67.45 cm, median: 55.50 cm) (P=0.233) (Table 1, Figure 4).
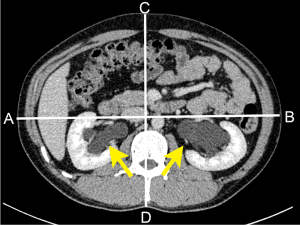
SAT areas and VAT areas in the abdomen
SAT areas and VAT areas of patients and controls at each abdominal slice were obtained. VAT areas at 29 out of 201 slices of the abdomen showed statistically significant differences between patients and controls: L−94 – −100 (from 9.4 to 10 cm below L3 vertebra) and L+79 – +100 (from 7.9 to 10 cm above L3 vertebra) (P<0.05) (Table 2, Figure S1). There was no statistically significant difference in SAT area at each slice of the abdomen.
Table 2
Slices | VAT areas | SAT areas | |||||||
---|---|---|---|---|---|---|---|---|---|
Patients | Controls | P value | 95% CI | Patients | Controls | P value | 95% CI | ||
L+79 (cm2) | 149.56±73.54 | 118.91±75.28 | 0.042 | 1.11 to 60.19 | 81.90±25.50 | 76.06±36.32 | 0.354 | −6.62 to 18.31 | |
L+80 (cm2) | 148.86±73.40 | 118.15±74.96 | 0.041 | 1.27 to 60.15 | 81.52±25.44 | 75.65±36.16 | 0.351 | −6.56 to 18.29 | |
L+81 (cm2) | 148.18±73.10 | 117.42±74.68 | 0.040 | 1.44 to 60.10 | 81.03±25.28 | 75.26±35.99 | 0.355 | −6.58 to 18.14 | |
L+82 (cm2) | 147.26±72.92 | 116.61±74.25 | 0.040 | 1.44 to 59.86 | 80.58±25.18 | 74.95±35.88 | 0.367 | −6.70 to 17.94 | |
L+83 (cm2) | 146.31±73.32 | 115.48±73.72 | 0.039 | 1.66 to 60.02 | 80.25±25.11 | 74.62±35.66 | 0.363 | −6.62 to 17.89 | |
L+84 (cm2) | 145.19±73.32 | 114.32±73.12 | 0.038 | 1.81 to 59.93 | 79.93±25.09 | 74.34±35.46 | 0.365 | −6.61 to 17.80 | |
L+85 (cm2) | 144.27±73.15 | 113.18±72.68 | 0.036 | 2.14 to 60.02 | 79.54±25.01 | 74.05±35.27 | 0.371 | −6.66 to 17.64 | |
L+86 (cm2) | 143.07±72.72 | 118.90±72.28 | 0.034 | 2.40 to 59.94 | 79.29±24.87 | 73.67±35.13 | 0.359 | −6.48 to 17.71 | |
L+87 (cm2) | 141.64±72.30 | 110.55±71.92 | 0.034 | 2.47 to 59.71 | 78.83±24.82 | 73.34±34.92 | 0.367 | −6.55 to 17.53 | |
L+88 (cm2) | 140.24±72.24 | 109.18±71.43 | 0.033 | 2.54 to 59.57 | 78.45±24.75 | 73.09±34.76 | 0.376 | −6.63 to 17.35 | |
L+89 (cm2) | 138.97±71.94 | 107.88±70.99 | 0.032 | 2.72 to 59.45 | 78.25±24.65 | 72.85±34.66 | 0.371 | −6.54 to 17.36 | |
L+90 (cm2) | 137.92±71.83 | 106.57±70.79 | 0.030 | 3.05 to 59.65 | 77.99±24.47 | 72.59±34.57 | 0.370 | −6.50 to 17.30 | |
L+91 (cm2) | 136.97±71.50 | 105.63±70.91 | 0.030 | 3.08 to 59.60 | 77.57±24.40 | 72.35±34.38 | 0.383 | −6.62 to 17.07 | |
L+92 (cm2) | 136.33±71.25 | 104.60±70.86 | 0.028 | 3.54 to 59.94 | 77.27±24.35 | 72.16±34.22 | 0.391 | −6.69 to 16.92 | |
L+93 (cm2) | 135.69±70.90 | 103.38±70.45 | 0.024 | 4.26 to 60.37 | 76.97±24.30 | 71.88±34.14 | 0.393 | −6.69 to 16.86 | |
L+94 (cm2) | 135.14±70.86 | 102.34±69.97 | 0.022 | 4.85 to 60.75 | 76.79±24.29 | 71.69±33.96 | 0.391 | −6.64 to 16.82 | |
L+95 (cm2) | 134.41±71.14 | 101.00±68.93 | 0.019 | 5.60 to 61.20 | 76.57±24.37 | 71.49±33.75 | 0.391 | −6.62 to 16.77 | |
L+96 (cm2) | 133.35±71.18 | 99.84±68.08 | 0.018 | 5.86 to 61.15 | 76.35±24.40 | 71.27±33.57 | 0.389 | −6.58 to 16.74 | |
L+97 (cm2) | 132.40±71.24 | 98.83±67.36 | 0.017 | 6.05 to 61.08 | 76.26±24.33 | 71.03±33.41 | 0.373 | −6.38 to 16.84 | |
L+98 (cm2) | 131.32±71.53 | 97.84±66.64 | 0.017 | 6.04 to 60.91 | 76.10±24.23 | 70.78±33.23 | 0.362 | −6.23 to 16.88 | |
L+99 (cm2) | 130.05±71.61 | 97.01±65.77 | 0.018 | 5.75 to 60.33 | 75.88±24.18 | 70.52±33.11 | 0.358 | −6.16 to 16.88 | |
L+100 (cm2) | 129.05±71.67 | 96.00±64.89 | 0.017 | 5.92 to 60.18 | 75.74±24.11 | 70.38±33.04 | 0.357 | −6.13 to 16.85 | |
L−94 (cm2) | 111.32±36.42 | 93.32±43.53 | 0.027 | 1.67 to 33.84 | 176.68±62.59 | 154.79±64.32 | 0.088 | −3.29 to 47.08 | |
L−95 (cm2) | 107.61±32.86 | 92.16±43.09 | 0.047 | 0.24 to 30.66 | 175.68±62.55 | 153.84±63.94 | 0.087 | −3.26 to 46.94 | |
L−96 (cm2) | 106.76±32.73 | 91.02±42.98 | 0.042 | 0.58 to 30.90 | 174.56±62.35 | 152.87±63.58 | 0.088 | −3.29 to 46.68 | |
L−97 (cm2) | 106.07±32.50 | 89.91±42.68 | 0.036 | 1.10 to 31.22 | 173.25±62.16 | 151.80±63.22 | 0.090 | −3.42 to 46.34 | |
L−98 (cm2) | 105.41±32.22 | 88.85±42.34 | 0.030 | 1.62 to 31.49 | 172.04±62.04 | 150.68±62.94 | 0.090 | −3.43 to 46.17 | |
L−99 (cm2) | 104.79±32.04 | 87.84±42.16 | 0.026 | 2.08 to 31.81 | 171.12±61.96 | 149.65±62.65 | 0.088 | −3.26 to 46.19 | |
L−100 (cm2) | 104.29±32.00 | 87.26±42.09 | 0.025 | 2.20 to 31.88 | 170.12±61.88 | 148.56±62.34 | 0.086 | −3.09 to 46.21 | |
S1 (cm2) | 99.65±31.35 | 83.46±41.45 | 0.030 | 1.61 to 30.78 | – | – | – | – | |
S2 (cm2) | 101.80±28.95 | 81.54±42.31 | 0.006 | 5.86 to 34.68 | – | – | – | – | |
S3 (cm2) | 102.80±27.29 | 76.31±35.07 | <0.001 | 14.02 to 38.96 | – | – | – | – | |
S4 (cm2) | 105.84±27.68 | 72.05±31.63 | <0.001 | 21.99 to 45.58 | – | – | – | – | |
FH (cm2) | 103.04±27.08 | 57.58±24.30 | <0.001 | 35.25 to 55.67 | – | – | – | – | |
PFV (cm3) | 924.66±235.95 | 621.24±248.21 | <0.001 | 207.31 to 399.53 | – | – | – | – |
Data are shown as mean ± SDs. VAT, visceral adipose tissue; SAT, subcutaneous adipose tissue; PFV, pelvic fat volume; 95% CI, 95% confidence interval; FH, femoral head.
VAT areas in the pelvic cavity
All 5 sections we measured from S1 to S4 and FH in the pelvic cavity showed statistical differences (P<0.05). The means and standard deviations of the 5 sections for the 2 groups are shown in Table 2 (Figure S1). Taking the VAT area of S1 level as the baseline, area ratios of S2/S1, S3/S1, S4/S1, and FH/S1 were obtained. The area ratios of S3/S1, S4/S1, and FH/S1 demonstrated statistical differences (P<0.05) (Figure S2).
PFV
The mean PFVs in patients calculated by MRIcron and Syngo Volume were 924.60 and 924.72 cm3, respectively. The mean PFVs of controls calculated by the 2 software packages were 621.15 and 621.33 cm3, respectively. The ICC of PFV was 1.000 (P<0.001), indicating excellent between-group agreement of PFV. Averagely, the mean PFV of patients was 924.66 cm3 and the standard deviation was 235.95 cm3, whereas the mean and standard deviation of PFV of controls were 621.24 and 248.21 cm3, respectively. The 2 independent-sample t-tests showed that the difference in PFV between the PL group and the control group was statistically significant (P<0.001) (Table 2).
Intergroup differences in SAT areas, VAT areas, and PFV by matching patients to controls based on BMI
PL patients were matched to controls according to BMI. Intergroup differences in SAT areas, VAT areas, and PFV were compared by paired t-test or Wilcoxon rank-sum test. There were statistical differences in the PFV and VAT areas of all 5 pelvic slices (P<0.05) (Table 3) and there was no statistical difference in the SAT area between patients and controls, which is consistent with the result without matching patients and controls. In the abdomen, VAT areas in 22 out of 201 slices of the abdomen showed statistic differences between patients and controls: L−87 − L−100 (from 8.7 to 10 cm below the L3 vertebra) and L+93 − L+100 (from 9.3 to 10 cm above the L3 vertebra), which was slightly but not significantly different from the previous results for unmatched BMI.
Table 3
Slices | P value |
---|---|
L+93 (cm2) | 0.048* |
L+94 (cm2) | 0.0.45* |
L+95 (cm2) | 0.041* |
L+96 (cm2) | 0.040* |
L+97 (cm2) | 0.040* |
L+98 (cm2) | 0.041* |
L+99 (cm2) | 0.044* |
L+100 (cm2) | 0.044* |
L−87 (cm2) | 0.023 |
L−88 (cm2) | 0.016 |
L−89(cm2) | 0.014 |
L−90 (cm2) | 0.013 |
L−91 (cm2) | 0.014 |
L−92 (cm2) | 0.012 |
L−93 (cm2) | 0.010 |
L−94 (cm2) | 0.008 |
L−95 (cm2) | 0.006 |
L−96 (cm2) | 0.006 |
L−97 (cm2) | 0.006 |
L−98 (cm2) | 0.004 |
L−99 (cm2) | 0.004 |
L−100 (cm2) | 0.004 |
S1 (cm2) | 0.021 |
S2 (cm2) | 0.008 |
S3 (cm2) | <0.001 |
S4 (cm2) | <0.001* |
FH (cm2) | <0.001* |
PFV (cm3) | 0.001* |
*, statistical analysis performed using paired t-test, otherwise Wilcoxon rank-sum test. VAT, visceral adipose tissue; PFV, pelvic fat volume; BMI, body mass index; FH, femoral head.
Correlations and linear regression models between VAT areas and PFV
As there were apparent outliers in the control group (Figure S1), we confirmed that these were from the same individual and therefore excluded this individual before performing correlations and running a linear regression. VAT areas of pelvic sections had great correlations with PFV (correlation coefficients: 0.624–0.745) whereas the correlations between abdominal sections and PFV were rather poor (correlation coefficient maximum: 0.596). VAT area at the level of FH had the best correlation with PFV (r=0.745). The linear regression models were established using VAT areas of 7 sections of the abdomen and the 5 sections of the pelvic cavity as the independent variables respectively and PFV as the dependent variable (Table 4, Figure S3).
Table 4
Slice of VAT areas | PFV | ||
---|---|---|---|
r value | R square | P value | |
L−94 | 0.570 | 0.318 | <0.001 |
L−95 | 0.585 | 0.342 | <0.001 |
L−96 | 0.586 | 0.336 | <0.001 |
L−97 | 0.589 | 0.346 | <0.001 |
L−98 | 0.591 | 0.350 | <0.001 |
L−99 | 0.594 | 0.353 | <0.001 |
L−100 | 0.596 | 0.355 | <0.001 |
S1 | 0.624 | 0.389 | <0.001 |
S2 | 0.653 | 0.427 | <0.001 |
S3 | 0.715 | 0.511 | <0.001 |
S4 | 0.694 | 0.482 | <0.001 |
FH | 0.745 | 0.555 | <0.001 |
VAT, visceral adipose tissue; PFV, pelvic fat volume; FH, femoral head.
Power estimates for different anatomic locations
Established linear regression model by VAT areas of FH:
In addition to binary regression models, we also developed multiple regression models using an enter linear regression algorithm, with independent variables including VAT area at 7 sections of the abdomen and 5 sections of the pelvis, and the independent variable of the obtained model still only contained FH.
We made Bland-Altman plots of the equation to compare the estimates and the real values. Figure 5A shows the corresponding Bland-Altman plots of actual PFV versus estimated PFV computed with the obtained VAT areas at FH. Figure 5B provides an overview of standard deviations Sd% of the percent differences (PFV~ − PFV) / PFV ×100% for all landmarks in the pelvic cavity. A minimal Sd% value (6.91% for the binary regression model including FH) was observed. The second smallest Sd% value was located at the level of S3 (Sd% value 7.34%).

Prediction of PL with VAT areas
The logistic regression equations were established using VAT areas of the 5 sections of the pelvic cavity as the independent variables. The logistic regression equations including S4 or FH had great efficiency to predict PL (Table 5).
Table 5
Variables included in the logistic regression equation | AUC | 95% CI | P value |
---|---|---|---|
S1 | 0.655 | 0.547–0.762 | 0.008 |
S2 | 0.699 | 0.595–0.803 | <0.001 |
S3 | 0.756 | 0.660–0.852 | <0.001 |
S4 | 0.800 | 0.711–0.889 | <0.001 |
FH | 0.893 | 0.830–0.956 | <0.001 |
S4 + FH | 0.927 | 0.874–0.980 | <0.001 |
PFV | 0.834 | 0.751–0.916 | <0.001 |
AUC, area under the curve; 95% CI, 95% confidence interval; FH, femoral head; PFV, pelvic fat volume.
Furthermore, we developed a binary logistic regression model that combined VAT areas of S4 and FH.
The AUC of the logistic regression model was 0.927 [95% confidence interval (CI): 0.874–0.980, SEN =90%, SPE =88%, threshold P0>0.462]. The overall accuracy of the logistic regression model in predicting PL was 88% (Figure 6A,6B). We also performed univariate logistic regression analysis using the area ratio of different locations in the pelvic cavity between the patients and controls. FH/S1 ratio had the largest AUC (0.823, 95% CI: 0.742–0.904, P<0.001), followed by S4/S1 ratio (AUC: 0.696, 95% CI: 0.592–0.799, P<0.001). The ROC curve demonstrated that the best threshold for PFV to predict PL was 733.42 cm3 (AUC =0.834, 95% CI: 0.751–0.916, SEN =80%, SPE =82%).
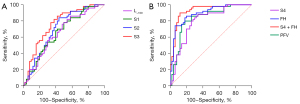
Discussion
This study, conducted in the largest sample population of PL to date, investigated the association between SAT and VAT in a single image with PFV in PL patients and controls. The results suggested that VAT areas of some specific levels and PFV were significantly different between patients and controls. We found that VAT area measured at a single slice located just above the level of the FH can be used to estimate PFV. Furthermore, VAT areas at the level of FH and S4 can be applied to predict the diagnosis of PL as a simplified and accurate approach.
The etiology and natural course of PL remain unclear, but are possibly related to obesity (11). However, in our study, weight, BMI, and body width between patients and controls were not statistically different. Craig et al. argued that it is reasonable to consider lipomatosis as a local manifestation of obesity, but the theory fails to explain its gender and racial preference, as well as its rarity (12).
We also endeavored to investigate fat distribution differences between patients and controls. Zhang et al. found that excessive fat in patients with PL was primarily distributed in perivesical and perirectal areas and gradually spread upward to the abdominal cavity, unlike obese patients whose excessive fat can be randomly distributed in the belly, pelvic cavity, or subcutaneous space (7). Our study also strongly supported this point. There were no statistical differences in abdominal SAT areas between the 2 groups, but there were statistical differences in VAT areas of the lower abdomen [L−94 − L−100 (unmatching) or L−87 − L−100 (matching BMI)] and pelvic cavity (S1–S4 and FH) between the 2 groups. Although the slices of L+79 − L+100 (unmatching) or L+93 − L+100 (matching) also indicated a statistical difference between patients and controls, these slices equivalent to 7.9–10 cm above the L3 vertebra or 9.3–10 cm above the L3 vertebra were located in the upper abdomen near the inferior boundary of the lung and were susceptible to respiratory movement. Therefore, these sections of the upper abdomen were not included in the following model construction. Besides, for the lower abdomen, sections where the 2 methods intersected were selected as these parameters were more robust, resulting in 7 sections. The 7 slices of L−94 − L−100 represented 9.4–10 cm below the L3 vertebra approximately near the L5/S1 disc, so it manifested that abdominal fat deposits in PL patients mainly in the lower abdomen.
Some studies have shown that a single-slice area of AT can strongly predict total AT volume and VAT volume in normal people, obese adults, and cancer patients (8,9,13-15). These studies just measured several bony landmarks such as L3 or the L4–5 level as the central point. We utilized a deep learning method for image segmentation, which could automatically segment SAT and VAT regions of each CT image, thereby obtaining abdominal SAT and VAT areas and pelvic VAT areas. To ensure reproducibility, slice selection protocols should use specific bony landmarks rather than soft tissue (15), so we selected the levels of S1–4 and the level above the bilateral FH in the pelvic cavity.
PFV can directly and precisely reflect the content of pelvic AT but the measurement is a time-consuming process. The MRIcron and Siemens Syngo Volume software that we used to measure volume are not that simple and convenient. Hence, the study used a simplified way of AT area measurement to replace PFV and then make a diagnosis of PL. For scientific research, we utilized a deep architecture to segment SAT and VAT regions to save time and economize manpower to acquire a mass of data, but in clinical practice, physicians are able to choose available workstations to measure areas instead of volumes.
Using VAT areas at the level of FH estimated the value of PFV with minimal Sd% (6.91%). In clinical practice, we can use the AT area of a single image to estimate PFV for measurement convenience, but it is generally discouraged to predict PL with AT area from a single image. Instead, we recommend the use of a combined regression model of S4 and FH to predict PL, which has better diagnostic efficacy than any single level.
The diagnosis of PL is made by imaging examinations in clinical practice (16) as its clinical symptoms are non-specific. Our study provides a quantitative and accurate method to diagnose PL. A study reported evidence of significantly improved symptoms in a patient after diet management and weight loss (17), which suggested conservative treatments can delay or even reverse the course of the disease. Consequently, recognizing PL correctly in the early stage can benefit patients more. Close monitoring of renal function is widely recommended for patients with mildly symptomatic cases of lipomatosis with no impact on renal function. Radical cystoprostatectomy with urinary diversion and reimplantation of ureters should be considered when diseases progress due to obstruction (12,18). Local fat extirpation can be helpful in the relief of urinary obstruction (19-21).
The general limitations of this study are the retrospective, single-center, and mono-ethnic design. All SAT and VAT regions were segmented automatically using deep learning and were not validated by an independent reference. Additionally, limited by a small sample of the rare disease, the available patient data may have introduced a selection bias. Our sample was composed of males, so applicability to females remains to be investigated.
Conclusions
We recommend the use of a single CT slice centered at the level of FH to estimate PFV. This choice is characterized by a small standard deviation and minimal assessment time. The logistic regression model including VAT areas of S4 and FH provides greater power for the diagnosis of PL. The conclusion should be restricted to Asian men with a BMI of 19.20–37.04 kg/m2.
Acknowledgments
Funding: This work was supported by
Footnote
Reporting Checklist: The authors have completed the STARD reporting checklist. Available at https://qims.amegroups.com/article/view/10.21037/qims-23-128/rc
Conflicts of Interest: All authors have completed the ICMJE uniform disclosure form (available at https://qims.amegroups.com/article/view/10.21037/qims-23-128/coif). The authors have no conflicts of interest to declare.
Ethical Statement: The authors are accountable for all aspects of the work in ensuring that questions related to the accuracy or integrity of any part of the work are appropriately investigated and resolved. The study was conducted in accordance with the Declaration of Helsinki (as revised in 2013) and approved by the Institutional Review Board of Peking Union Medical College Hospital. The study is a retrospective study and the requirement for written informed consent was waived by the Institutional Review Board of Peking Union Medical College Hospital.
Open Access Statement: This is an Open Access article distributed in accordance with the Creative Commons Attribution-NonCommercial-NoDerivs 4.0 International License (CC BY-NC-ND 4.0), which permits the non-commercial replication and distribution of the article with the strict proviso that no changes or edits are made and the original work is properly cited (including links to both the formal publication through the relevant DOI and the license). See: https://creativecommons.org/licenses/by-nc-nd/4.0/.
References
- Heyns CF. Pelvic Lipomatosis: A Review of Its Diagnosis and Management. J Urol 1991;146:267-73. [Crossref] [PubMed]
- Engels EP. Sigmoid colon and urinary bladder in high fixation: roentgen changes simulating pelvic tumor. Radiology 1959;72:419-22. [Crossref] [PubMed]
- Fogg LB, Smyth JW. Pelvic lipomatosis: a condition simulating pelvic neoplasm. Radiology 1968;90:558-64. [Crossref] [PubMed]
- Hermie I, Hermie L, Coenegrachts K. Pelvic Lipomatosis Causing Renal Failure. J Belg Soc Radiol 2016;100:55. [Crossref] [PubMed]
- Bååth L, Nyman U, Aspelin P, Wadström L. Computed tomography of pelvic lipomatosis. Report of a case. Acta Radiol Diagn (Stockh) 1986;27:311-4. [Crossref] [PubMed]
- Bai X, Zhang G, Xu L, Zhang X, Zhang X, Jin Z, Sun H. Diagnostic accuracy of CT imaging parameters in pelvic lipomatosis. Abdom Radiol (NY) 2021;46:2779-88. [Crossref] [PubMed]
- Zhang Y, Wu S, Xi Z, Wang X, Jiang X. Measuring diagnostic accuracy of imaging parameters in pelvic lipomatosis. Eur J Radiol 2012;81:3107-14. [Crossref] [PubMed]
- Shen W, Punyanitya M, Wang Z, Gallagher D, St-Onge MP, Albu J, Heymsfield SB, Heshka S. Total body skeletal muscle and adipose tissue volumes: estimation from a single abdominal cross-sectional image. J Appl Physiol (1985) 2004;97:2333-8. [Crossref] [PubMed]
- Shen W, Punyanitya M, Wang Z, Gallagher D, St-Onge MP, Albu J, Heymsfield SB, Heshka S. Visceral adipose tissue: relations between single-slice areas and total volume. Am J Clin Nutr 2004;80:271-8. [Crossref] [PubMed]
- Gut D, Tabor Z, Szymkowski M, Rozynek M, Kucybala I, Wojciechowski W. Benchmarking of Deep Architectures for Segmentation of Medical Images. IEEE Trans Med Imaging 2022;41:3231-41. [Crossref] [PubMed]
- Morettin LB, Wilson M. Pelvic lipomatosis. Am J Roentgenol Radium Ther Nucl Med 1971;113:181-4. [Crossref] [PubMed]
- Craig WD, Fanburg-Smith JC, Henry LR, Guerrero R, Barton JH. Fat-containing lesions of the retroperitoneum: radiologic-pathologic correlation. Radiographics 2009;29:261-90. [Crossref] [PubMed]
- Mourtzakis M, Prado CM, Lieffers JR, Reiman T, McCargar LJ, Baracos VE. A practical and precise approach to quantification of body composition in cancer patients using computed tomography images acquired during routine care. Appl Physiol Nutr Metab 2008;33:997-1006. [Crossref] [PubMed]
- Linder N, Michel S, Eggebrecht T, Schaudinn A, Blüher M, Dietrich A, Denecke T, Busse H. Estimation of abdominal subcutaneous fat volume of obese adults from single-slice MRI data - Regression coefficients and agreement. Eur J Radiol 2020;130:109184. [Crossref] [PubMed]
- MacDonald AJ, Greig CA, Baracos V. The advantages and limitations of cross-sectional body composition analysis. Curr Opin Support Palliat Care 2011;5:342-9. [Crossref] [PubMed]
- Sun Y, Wang J, Chiang M, Li H, Liu JB, Wang S. Value of Multimode Sonography for Assessment of Pelvic Lipomatosis Compared With Computed Tomography. J Ultrasound Med 2016;35:1143-8. [Crossref] [PubMed]
- Sacks SA, Drenick EJ. Pelvic lipomatosis: effect of diet. Urology 1975;6:609-15. [Crossref] [PubMed]
- Prabakaran R, Abraham G, Kurien A, Mathew M, Parthasarathy R. Pelvic lipomatosis. Kidney Int 2016;90:453. [Crossref] [PubMed]
- Carpenter AA. Pelvic Lipoivlactosis: Successful Surgical Treatment. J Urol 1973;110:397-9. [Crossref] [PubMed]
- SanjayPrakash J. Mathisekaran T, Jain N, Bafna S, Paul R, Selvaraj N. Robotic Management of Pelvic Lipomatosis-Experience with Difficulties Encountered and the Techniques to Successful Outcomes. Eur Urol Open Sci 2020;21:33-40. [Crossref] [PubMed]
- Chiruvella M, Syed GM, Darga S, Kondakindi PR, Enganti B, Adapala RKR. Robotic Local Fat Extirpation and Ureteric Reimplantation for Pelvic Lipomatosis with Ureteric Obstruction: Technical Considerations. Turk J Urol 2022;48:385-8. [Crossref] [PubMed]