Predicting the microvascular invasion and tumor grading of intrahepatic mass-forming cholangiocarcinoma based on magnetic resonance imaging radiomics and morphological features
Introduction
The incidence of intrahepatic cholangiocarcinoma (IHC) has risen progressively worldwide (1,2), and the most common morphological subtype is intrahepatic mass-forming cholangiocarcinoma (IMCC) (3,4). Surgical resection is the first-line treatment option; however, even though surgical techniques have greatly improved in recent decades, patients still have a poor outcome, with a 5-year survival rate of approximately 30–35% (5). The poor outcome is mainly related to the high postoperative recurrence rate, with recurrence occurring in approximately 50–70% of cases (6).
Microvascular invasion (MVI) and tumor grading are regarded as 2 of the most important prognostic factors that strongly influence not only tumor recurrence but also patients’ survival (7-10). MVI refers to the presence of tumor cells within the within a vascular lumen which is covered by endothelium that is visible only by microscopy (11). MVI is a potential source of intrahepatic metastases and distant metastatic spread, and also is associated with aggressive and progressive tumor behaviors (12). It has been accepted that for patients with a high probability of MVI, large safety margins are needed at surgical resection (13). Considering the strong correlation between tumor grading and lymph node metastases, although it may not be required by all patients, the high tumor grading of IMCC should prompt surgeons to consider portal lymphadenectomy (14). Therefore, the early and precise detection of MVI and tumor grading before treatment is necessary to provide patients with appropriate surgical decisions and prognoses. Generally, biopsy is not a satisfactory method for the diagnosis of MVI or tumor grading preoperatively because of the inadequate tumor specimen obtained and its invasive nature (15).
Medical imaging has provided a noninvasive method for the preoperative assessment of IHC. Specifically, magnetic resonance imaging (MRI) is a common imaging method for IMCC. Some studies have evaluated the correlations between MRI morphological features and MVI for IMCC, and large tumor size and intrahepatic duct dilatation were reported as independent predictors of MVI (16-18). However, the interobserver agreements of MRI morphological features lack further analyses, and the evaluation of these features is highly subjective and variable. Additionally, due to the limitation of the number of patients, those studies lacked sufficient validation. Radiomics can extract high-throughput imaging features from biomedical images and convert them into mineable data for quantitative analysis, with the aim of predicting the pathological characteristics of tumors (19). Recently, MRI-based radiomics nomograms have been found to be helpful in predicting MVI in preliminary studies (18,20). With the increase of the radiomics signatures (rad_scores), the possibility of MVI has increased gradually (18,20). To date, few studies have described the relationship between tumor grading in IMCC and MRI-based radiomics features.
Therefore, this study aimed to develop and validate noninvasive MRI-based radiomics nomograms to predict the biological MVI and tumor grading of IMCC, which are essential for a complete understanding of the stratification of the tumor and to guide more accurate personalized treatment in the future. We present this article in accordance with the TRIPOD reporting checklist (available at https://qims.amegroups.com/article/view/10.21037/qims-23-11/rc).
Methods
The study was conducted in accordance with the Declaration of Helsinki (as revised in 2013). The study was approved by the Ethics Committee of the National Cancer Center/Cancer Hospital, Chinese Academy of Medical Sciences and Peking Union Medical College, and the requirement for individual consent for this retrospective analysis was waived.
Eligibility criteria and patients
A total of 285 consecutive IHC patients who underwent hepatic resection at the Chinese Academy of Medical Sciences and Peking Union Medical College were enrolled in the study from 1 February 2010 to 2 February 2022. The inclusion criteria were as follows: (I) patients with a pathological confirmation of IHC from liver resection specimens; and (II) patients who underwent a preoperative MRI examination before surgery at the Chinese Academy of Medical Sciences and Peking Union Medical College. The excluded patients met the following criteria: (I) patients under neoadjuvant treatment before surgery (n=20); (II) other types of IHC (n=15; 7 for periductal-infiltrating type and 8 for intraductal growing type); (III) patients with positive resection margins (n=10); and (IV) inadequate imaging quality for analysis (n=5). Ultimately, 235 eligible patients [median age, 60 years; interquartile range (IQR), 53–66; age range, 36–80 years; 146 men] were enrolled in our study and randomly divided into training (n=167) and validation (n=68) cohorts at a ratio of around 5:2 (Figure 1). The same cohort was used to predict both MVI status and tumor grading. The median time between the MRI examination and hepatectomy was 15 days (IQR, 9–21 days). The tumor specimens of all patients were obtained through pathological detection for MVI and tumor grading postoperatively.
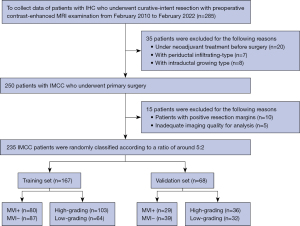
MRI protocols
MRI examinations were acquired with 8-channel phased array coil 3.0-T MRI scanners (Discovery MR 750 for 43 patients, SIGNA Excite HDxt for 92 patients, SIGNA Pioneer for 46 patients, and SIGNA Architect for 25 patients; GE Healthcare, Waukesha, WI, USA; Magnetom Spectra for 17 patients, and Magnetom Prisma for 12 patients; Siemens, Erlangen, Germany). The patients fasted for at least 4 hours before examination. All patients underwent routine abdominal MRI performed using the following sequences: non-CE T1-weighted in-phase and opposed-phase, T2 weighted imaging-fat-suppressed (T2WI-FS) on transverse, and T2WI on coronal. Diffusion-weighted imaging (DWI) was obtained at b values of 0 and 800 s/mm2. A total of 157 patients received 0.2 mmol/kg body weight of extracellular gadolinium contrast agent (Magnevist; Bayer AG, Leverkusen, Germany) at an infusion rate of 1.5–2 mL/s; the other patients received gadoxetic acid (Primovist; Bayer AG) injected at a dose of 0.025 mmol/kg at an infusion rate of 1 mL/s. Both were followed by a wash with 20 mL of 0.9% physiological saline flush, after which multiphasic dynamic images were obtained. The images in the arterial phase (AP), portal venous phase (PVP), and delayed phase (DP; for extracellular gadolinium contrast agents) or transitional phase (for gadoxetic acid) were acquired during suspended respiration at 25–35, 60–75, and 150–180 s, respectively, on transverse. Additionally, the hepatobiliary phase was acquired 10–15 minutes after the injection of gadoxetate disodium. For patients with cirrhosis, a 20-minute hepatobiliary phase is preferred. The last phase was scanned in the coronal direction after the transverse scan. Detailed technical specifications are shown in Table S1.
Preoperative information collecting
Preoperative information mainly included 20 items that were categorized into the following groups: (I) the standard demographics of the patients (including age and sex); (II) 7 laboratory variables, including hepatitis Be antigen (HBeAg), α-fetoprotein (AFP), carbohydrate antigen 19-9 (CA19-9), carcinoembryonic antigen (CEA), alanine aminotransferase (ALT), aspartate aminotransferase (AST), and gamma-glutamyl transpeptidase (GGT); and (III) 11 MRI morphological features, including tumor maximum diameter, number of tumors, tumor margin, necrosis, bile duct dilatation, capsule retraction, peritumoral enhancement, DWI signal characteristics, AP enhancement patterns, targetoid appearance, and lymph node status. The detailed classifications and definitions of each MRI feature are shown in Table S2. All MRI features were independently evaluated on a picture archiving and communication system by 2 radiologists (radiologists 1 and 2, with 10 and 8 years of experience in abdominal imaging, respectively). Any discrepancy was discussed to reach a final consensus.
Radiomic feature extraction
Radiomics features were extracted from T2WI-FS and DWI (b=800 s/mm2) sequences. Both sequences were resampled and aligned to the same resolution, spacing, and position by ITK-SNAP (http://www.itksnap.org) to offset the interference caused by the uneven spatial resolution. The trilinear interpolation algorithm was used to resample the T2WI/FS and DWI with a new image resolution of 1 mm × 1 mm × 1 mm. Tumor volumes of interest (VOIs) covering the total volume of tumor tissue (hyperintense signal intensity on T2WI-FS, and restricted diffusion on DWI) were manually segmented by radiologist 1 on transverse slices using ITK-SNAP. VOIs also included cystic necrotic regions, which are considered one of the manifestations of tumor biological behaviors (21). The original MRI images and VOIs were saved as medical digital imaging files in Neuroimaging Informatics Technology Initiative (NII) format. To assess the intraobserver repeatability and reliability of the extracted features, 70 patients (approximately 30% of the total patients) were randomly selected, and the same segmentation procedure was repeated 1 month after the first evaluation by the same radiologist to reduce recall bias. The intraclass correlation coefficient (ICC) was used to assess the reproducibility of the radiomics features (22). All image segmentations were reviewed by radiologist 2. The PyRadiomics software package (version 3.0.1; http://www.radiomics.io/pyradiomics.html) was used to extract radiomics features from VOIs (23). The radiomics features were obtained on the original images using the wavelet and Laplacian of Gaussian filters with sigma values of 1.0, 2.0, 3.0, 4.0, and 5.0. A single-level directional discrete wavelet transform with high-pass (H) and low-pass (L) filters was used for wavelet transform (24). In total, 8 wavelet-decomposition images were created from each MRI sequence input, including HHH, HHL, HLH, HLL, LHH, LHL, LLH, and LLL. Finally, we extracted 1,132 radiomic features, including first-order statistical features (234 features), shape features (14 features), gray-level co-occurrence matrix (GLCM) features (286 features), gray-level run-length matrix (GLRLM) features (208 features), gray-level size zone matrix (GLSZM) features (208 features), and gray-level dependence matrix (GLDM) features (182 features), from the original images and wavelet-transformed images. In the process of MRI characteristic evaluation and VOI delineation, both radiologists were aware of the diagnosis of IMCC but were blinded to the clinicopathologic traits. For multinodular tumors (including satellite nodules and intrahepatic metastasis), only the targeted lesion (the largest nodule) was selected for further analysis. The average time needed for segmentation was 7 minutes (range, 4–10 minutes). The radiomics workflow is depicted in Figure 2.
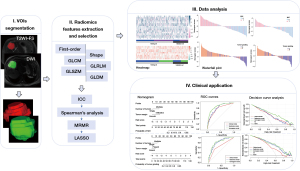
Feature selection
The VOIs were saved as label maps and then used to obtain the radiomics features. To render the parameters of different dimensions comparable, the values of the extracted features in the training set were normalized by the Z score, and the mean and standard deviation (SD) values based on the training set were used to standardize the values of the validation set. We followed a 4-step procedure to identify robust radiomic features (Figure S1). First, we used ICC to evaluate the repeatability of radiomics features. The features with ICC ≥0.8 were chosen as having high reproducibility for further analysis and the other features were discarded as low reproducibility. Second, Spearman’s analysis was used to calculate the correlation between radiomics features in a single sequence (T2WI/FS or DWI). Features with |ρ| ≥0.9 were defined as highly correlated. If correlation factor was high, any of the features were randomly selected for further analysis, and the other features were discarded. Third, minimum redundancy maximum relevance (MRMR) was used to remove the redundant and irrelevant features in the training set (30 features were retained). Finally, we used least absolute shrinkage and selection operator (LASSO) regression in the training set to select the optimized features with nonzero coefficients with 10-fold cross-validation.
Models construction and evaluation
The clinical data, and MRI morphological features were subsequently compared by univariate logistic analysis. The clinical data included the standard demographics of the patients (age and sex), and laboratory variables (HBeAg, AFP, CA19-9, CEA, ALT, AST, and GGT). Significant features with P values <0.05 were included in the multivariable logistic regression analysis. The clinical models were established based on significant features of demographics, laboratory, and MRI morphological features. The radiomics models were built based on the selected radiomics features. Then, the rad_score of each patient was calculated based on a linear combination of the selected features with the coefficients weighted by the LASSO regression. The independent features in the clinical models and rad_scores were combined to establish nomogram models for the prediction of MVI and tumor grading, respectively. The validation group was used to test the internal validation of the nomograms. A nomogram score was calculated for each patient in the training and validation sets.
Performance and validation of the prediction model
The areas under the receiver operating characteristic (ROC) curve (AUCs) were used to predict the accuracy of each model. The difference in the ROC curves between various models were assessed by the DeLong test. The sensitivity, specificity, and positive predictive value (PPV), and negative predictive value (NPV) of the predictive models were calculated by cross-tabulation. The model calibration was assessed by using calibration curves with the Hosmer-Lemeshow test. Additionally, decision curve analysis (DCA) was used to evaluate the clinical utility of the prediction model by computing the net benefits for a range of threshold probabilities.
Histopathologic analysis
The pathological examination results were recorded according to the hospital clinical records. The pathological slices of IMCC were subjected to postoperative hematoxylin and eosin (H&E) staining. MVI was defined as the presence of nested clusters of cancer cells within the endothelium-covered vessels diagnosed only by microscopy (25). The histologic grading of IMCC was divided into 3 categories: G1 (well differentiated), G2 (moderately differentiated), and G3 (poorly differentiated) (26). In our study, we defined G1 and/or G2 as “low grading” and G3 as “high grading”.
Statistical analysis
Clinical, histopathologic, and MRI morphological features are expressed as numbers (frequency %) or the mean ± SD as appropriate. The Mann-Whitney U test or unpaired t-test was used for continuous variables according to the normal distribution test, and the chi-square test or Fisher’s exact test was used for categorical variables. Interobserver agreement was analyzed for each qualitative MRI feature by the κ statistic. The level of agreement was assessed as slight (κ of 0.01 to 0.20), fair (0.21 to 0.40), moderate (0.41 to 0.60), good (0.61 to 0.80), and excellent (0.81 to 1). Associated odds ratios (ORs) and 95% confidence intervals (CIs) were estimated. Statistical significance was defined as P values <0.05 (2-tailed). Statistical analysis was carried out by SPSS 23.0 (IBM Corp., Armonk, NY, USA) and R statistical software (version 4.0.3, https://www.r-project.org).
Results
Interobserver agreements
The percentage of MRI morphological features identified for feature classification by the 2 radiologists as well as their interobserver agreements for each imaging feature are shown in Table S3. The κ values for MRI morphological features were good to excellent (κ=0.634–0.888).
Clinical and MRI characteristics of the patients
A total of 235 patients were enrolled in this study, among whom 167 patients (MVI+ =80, MVI− =87; high grading =103, low grading =64) were enrolled in the training set. In the validation set, 68 patients were enrolled, including 29 patients with MVI+, 39 with MVI−, 36 with high grading and 32 with low grading, showing no significant difference in tumor pathological behaviors in the distribution among patients (P=0.464 for MVI; P=0.217 for tumor grading). Additionally, there were no significant differences in clinical and MRI characteristics between the training and validation sets except for AFP (P=0.014). The demographic and tumor characteristics are summarized in Table 1.
Table 1
Variables | Training set (n=167) | Validation set (n=68) | P-Inter value | |||||||||||
---|---|---|---|---|---|---|---|---|---|---|---|---|---|---|
MVI (+), n=80 | MVI (−), n=87 | P-Intra value | High grade, n=103 |
Low grade, n=64 | P-Intra value | MVI (+), n=29 | MVI (−), n=39 | P-Intra value | High grade, n=36 |
Low grade, n=32 | P-Intra value | |||
Age (year)† | 59±9.7 | 60±8.7 | 0.833 | 59±9.6 | 59±8.7 | 0.890 | 59.0±9.2 | 60.3±8.9 | 0.539 | 58.8±8.5 | 61.1±9.6 | 0.621 | 0.120 | |
Sex | 0.328 | 0.916 | 0.841 | 0.773 | 0.266 | |||||||||
Male | 51 (63.8) | 49 (56.3) | 62 (60.2) | 38 (59.4) | 20 (69.0) | 26 (66.7) | 27 (75.0) | 19 (59.4) | ||||||
Female | 29 (36.2) | 38 (43.7) | 41 (39.8) | 26 (40.6) | 9 (31.0) | 13 (33.3) | 9 (25.0) | 13 (40.6) | ||||||
HBsAg | 0.965 | 0.053 | 0.904 | 0.511 | 0.662 | |||||||||
Positive | 26 (32.5) | 28 (32.2) | 39 (37.9) | 15 (23.4) | 10 (34.5) | 14 (35.9) | 14 (38.9) | 10 (31.2) | ||||||
Negative | 54 (67.5) | 59 (67.8) | 64 (62.1) | 49 (76.6) | 19 (65.5) | 25 (64.1) | 22 (61.1) | 22 (68.8) | ||||||
CA19-9, U/mL | 0.028 | 0.477 | 0.013 | 0.219 | 0.400 | |||||||||
>37 | 53 (66.2) | 43 (49.4) | 57 (55.3) | 39 (60.9) | 20 (69.0) | 15 (38.5) | 16 (44.4) | 19 (59.4) | ||||||
≤37 | 27 (33.8) | 44 (50.6) | 46 (44.7) | 25 (39.1) | 9 (31.0) | 24 (61.5) | 20 (55.6) | 13 (40.6) | ||||||
AFP, ng/mL | 0.200 | 0.834 | – | – | 0.014 | |||||||||
>20 | 9 (11.2) | 5 (5.7) | 9 (8.7) | 5 (7.8) | 0 (0) | 0 (0) | 0 (0) | 0 (0) | ||||||
≤20 | 71 (88.8) | 82 (94.3) | 94 (91.3) | 59 (92.2) | 29 (100.0) | 39 (100.0) | 36 (100.0) | 32 (100.0) | ||||||
CEA, ng/mL | 0.051 | 0.182 | 0.721 | 0.931 | 0.852 | |||||||||
>5 | 22 (27.5) | 13 (14.9) | 25 (24.3) | 10 (15.6) | 7 (24.1) | 8 (20.5) | 8 (22.2) | 7 (21.9) | ||||||
≤5 | 58 (72.5) | 74 (85.1) | 78 (75.7) | 54 (84.4) | 22 (75.9) | 31 (79.5) | 28 (77.8) | 25 (78.1) | ||||||
ALT, U/L | 0.753 | 0.796 | 0.887 | 0.575 | 0.373 | |||||||||
>40 | 15 (18.7) | 18 (20.7) | 21 (20.4) | 12 (18.7) | 7 (24.1) | 10 (25.6) | 10 (27.8) | 7 (21.9) | ||||||
≤40 | 65 (81.3) | 69 (79.3) | 82 (79.6) | 52 (81.3) | 22 (75.9) | 29 (74.4) | 26 (72.2) | 25 (78.1) | ||||||
AST, U/L | 0.534 | 0.672 | 0.940 | 0.092 | 0.695 | |||||||||
> 40 | 11 (13.7) | 15 (17.2) | 17 (16.5) | 9 (14.1) | 5 (17.2) | 7 (17.9) | 9 (25.0) | 3 (9.4) | ||||||
≤40 | 69 (86.3) | 72 (82.8) | 86 (83.5) | 55 (85.9) | 24 (82.8) | 32 (82.1) | 27 (75.0) | 29 (90.6) | ||||||
GGT | 0.088 | 0.125 | 0.025 | 0.726 | 0.979 | |||||||||
> 55 | 37 (46.2) | 29 (33.3) | 36 (35.0) | 30 (46.9) | 16 (55.2) | 11 (28.2) | 15 (41.7) | 12 (37.5) | ||||||
≤55 | 43 (53.8) | 58 (66.7) | 67 (65.0) | 34 (53.1) | 13 (44.8) | 28 (71.8) | 21 (58.3) | 20 (62.5) | ||||||
Tumor diameter, cm | 0.009 | 0.051 | 0.418 | 0.100 | 0.139 | |||||||||
>5 | 51 (63.8) | 38 (43.7) | 61 (59.2) | 28 (43.7) | 14 (48.3) | 15 (38.5) | 12 (33.3) | 17 (53.1) | ||||||
≤5 | 29 (36.2) | 49 (56.3) | 42 (40.8) | 36 (56.3) | 15 (51.7) | 24 (61.5) | 24 (66.7) | 15 (46.9) | ||||||
Numbers of tumor | 0.001 | 0.009 | 0.022 | 0.109 | 0.081 | |||||||||
Single | 49 (61.3) | 79 (90.8) | 72 (69.9) | 56 (87.5) | 22 (75.9) | 37 (94.9) | 29 (80.6) | 30 (93.8) | ||||||
Multiple | 31 (38.7) | 8 (9.2) | 31 (30.1) | 8 (12.5) | 7 (24.1) | 2 (5.1) | 7 (19.4) | 2 (6.2) | ||||||
Tumor margin | <0.001 | 0.397 | 0.002 | 0.438 | 0.858 | |||||||||
Infiltrative | 62 (77.5) | 31 (35.6) | 60 (58.3) | 33 (51.6) | 22 (75.9) | 15 (38.5) | 18 (50.0) | 19 (59.4) | ||||||
Smooth | 18 (22.5) | 56 (64.4) | 43 (41.7) | 31 (48.4) | 7 (24.1) | 24 (61.5) | 18 (50.0) | 13 (40.6) | ||||||
Necrosis | 0.304 | 0.007 | 0.841 | <0.001 | 0.461 | |||||||||
Absent | 55 (68.8) | 66 (75.9) | 67 (65.0) | 54 (84.4) | 20 (69.0) | 26 (66.7) | 17 (47.2) | 29 (90.6) | ||||||
Present | 25 (31.2) | 21 (24.1) | 36 (35.0) | 10 (15.6) | 9 (31.0) | 13 (33.3) | 19 (52.8) | 3 (9.4) | ||||||
Bile duct dilatation | 0.301 | 0.713 | 0.106 | 0.557 | 0.104 | |||||||||
Absent | 52 (65.0) | 63 (72.4) | 72 (69.9) | 43 (67.2) | 17 (58.6) | 30 (76.9) | 26 (72.2) | 21 (65.6) | ||||||
Present | 28 (35.0) | 24 (27.6) | 31 (30.1) | 21 (32.8) | 12 (41.4) | 9 (23.1) | 10 (27.8) | 11 (34.4) | ||||||
Hepatic capsule retraction | 0.292 | 0.398 | 0.240 | 0.193 | 0.240 | |||||||||
Absent | 19 (23.7) | 27 (31.0) | 26 (25.2) | 20 (31.2) | 10 (34.5) | 19 (48.7) | 18 (50.0) | 11 (34.4) | ||||||
Present | 61 (76.3) | 60 (69.0) | 77 (74.8) | 44 (68.8) | 19 (65.5) | 20 (51.3) | 18 (50.0) | 21 (65.6) | ||||||
Peritumoral enhancement | 0.586 | 0.979 | 0.322 | 0.575 | 0.232 | |||||||||
Absent | 52 (65.0) | 60 (69.0) | 69 (67.0) | 43 (67.2) | 20 (69.0) | 31 (79.5) | 28 (77.8) | 23 (71.9) | ||||||
Present | 28 (35.0) | 27 (31.0) | 34 (33.0) | 21 (32.8) | 9 (31.0) | 8 (20.5) | 8 (22.2) | 9 (28.1) | ||||||
DWI signal characteristics | 0.526 | 0.107 | 0.583 | 0.702 | 0.441 | |||||||||
Diffuse hypo-enhancement | 37 (46.2) | 36 (41.4) | 40 (38.8) | 33 (51.6) | 10 (34.5) | 16 (41.0) | 13 (36.1) | 13 (40.6) | ||||||
Diffuse hyper-enhancement | 43 (53.8) | 51 (58.6) | 63 (61.2) | 31 (48.4) | 19 (65.5) | 23 (59.0) | 23 (63.9) | 19 (59.4) | ||||||
AP enhancement pattern | 0.234 | 0.001 | 0.014 | 0.910 | 0.338 | |||||||||
Diffuse hypo-enhancement | 13 (16.3) | 9 (10.3) | 11 (10.7) | 11 (17.2) | 9 (31.0) | 3 (7.7) | 7 (19.4) | 5 (15.6) | ||||||
Rim-enhancement | 60 (75.0) | 64 (73.6) | 83 (80.6) | 41 (64.1) | 18 (62.1) | 26 (66.7) | 23 (63.9) | 21 (65.6) | ||||||
Diffuse hyper-enhancement | 7 (8.7) | 14 (16.1) | 9 (8.7) | 12 (18.7) | 2 (6.9) | 10 (25.6) | 6 (16.7) | 6 (18.8) | ||||||
Targetoid appearance | 0.864 | 0.069 | 0.734 | 0.586 | 0.667 | |||||||||
Absent | 13 (16.3) | 15 (17.2) | 13 (12.6) | 15 (23.4) | 5 (17.2) | 8 (20.5) | 6 (16.7) | 7 (21.9) | ||||||
Present | 67 (83.7) | 72 (82.8) | 90 (87.4) | 49 (76.6) | 24 (82.8) | 31 (79.5) | 30 (83.3) | 25 (78.1) | ||||||
Lymph node status | 0.012 | 0.104 | 0.003 | 0.147 | 0.098 | |||||||||
Positive | 35 (43.7) | 22 (25.3) | 40 (38.8) | 17 (26.6) | 12 (41.4) | 4 (10.3) | 11 (30.6) | 5 (15.6) | ||||||
Negative | 45 (56.3) | 65 (74.7) | 63 (61.2) | 47 (73.4) | 17 (58.6) | 35 (89.7) | 25 (69.4) | 27 (84.4) | ||||||
Rad_score, median (interquartile range) | 0.639 (−0.122, 1.678) | −1.102 (−1.834, 0.221) | <0.001 | 1.132 (0.472, 1.921) | −0.258 (−1.246, 0.595) | <0.001 | 0.661 (−0.012, 1.765) | −0.922 (−1.380, 0.548) | <0.001 | 1.214 (0.668, 1.597) | −0.169 (−0.566, 0.879) | <0.001 | – |
Categorical variables are expressed as frequency with percentages in parentheses. †, the mean ± standard deviation. Qualitative variables are analyzed using Pearson’s χ2 test or Fisher’s exact test as appropriate, and quantitative variables are analyzed using the independent t-test. P-Intra is the result of univariate analyses between the MVI+ and MVI− groups, high-grade and low-grade groups; P-Inter value represents the comparisons of characteristics between training and validation set. MVI, microvascular invasion; HBsAg, hepatitis Be antigen; CA19-9, carbohydrate antigen 19-9; AFP, α-fetoprotein; CEA, carcinoembryonic antigen; ALT, alanine aminotransferase; AST, aspartate aminotransferase; GGT, gamma-glutamyl transpeptidase; DWI, diffusion-weighted imaging; AP, arterial phase; rad_score, radiomics score.
Selection of predictive factors
In univariable logistic regression analysis, 6 and 2 variables were significantly associated with an increased risk for MVI and tumor grading, respectively (Table 2). Multivariable logistic regression analysis identified number of tumors (P=0.002, OR =4.590, 95% CI: 1.736–12.136) and tumor margin (P<0.001, OR =5.472, 95% CI: 2.538–11.797) as independent predictors of MVI. For tumor grading, number of tumors (P=0.022, OR =2.752, 95% CI: 1.201–5.947) and tumor necrosis (P=0.016, OR =2.156, 95% CI: 1.157–6.547) were identified as independent risk factors in multivariable logistic regression analysis (Table 2). These independent risk factors were used for clinical models’ construction for the prediction of MVI and tumor grading.
Table 2
Variables | Univariate analysis | Multivariate analysis | |||
---|---|---|---|---|---|
Odds ratio (95% CI) | P value | Odds ratio (95% CI) | P value | ||
MVI-status | |||||
CA19-9 (>37 vs. ≤37 U/mL) | 2.009 (1.074–3.755) | 0.029* | 1.245 (0.566–2.742) | 0.586 | |
CEA (>5 vs. ≤5 ng/mL) | 2.159 (1.003–4.649) | 0.049* | 1.216 (0.479–3.082) | 0.681 | |
Tumor diameter (>5 vs. ≤5 cm) | 2.268 (1.217–4.225) | 0.010* | 1.198 (0.553–2.598) | 0.647 | |
Number of tumors (multiple vs. single) | 6.247 (2.657–14.691) | <0.001* | 4.590 (1.736–12.136) | 0.002* | |
Tumor margin (infiltrative vs. smooth) | 6.222 (3.140–12.331) | <0.001* | 5.472 (2.538–11.797) | <0.001* | |
Lymph node status (positive vs. negative) | 2.314 (1.200–4.464) | 0.012* | 1.182 (0.522–2.680) | 0.688 | |
Tumor grading | |||||
Numbers of tumor (multiple vs. single) | 3.014 (1.285–7.066) | 0.011* | 2.752 (1.201–5.947) | 0.022* | |
Necrosis (present vs. absent) | 2.901 (1.321–6.374) | 0.008* | 2.156 (1.157–6.547) | 0.016* |
*, P<0.05. CI, confidence interval; MVI, microvascular invasion; CA19-9, carbohydrate antigen 19-9; CEA, carcinoembryonic antigen.
Feature selection and construction of radiomics models
Each MRI sequence contained 1,132 features, for a total of 2,264 features (T2WI-FS and DWI). Finally, 16 radiomics features (11 from T2WI-FS, 5 from DWI) for MVI prediction and 9 radiomics features (6 from T2WI-FS, 3 from DWI) for tumor grading detection were selected with nonzero coefficients with optimal regulation weights λ of 0.0398 and 0.0588, respectively, under the 1-SE criterion, and rad_scores were constructed (Figure 3). The selected radiomics features and their corresponding coefficients are detailed in Table S4. Figure S2 shows the heatmap of the selected radiomics features and scatter plots of the rad_scores for the MVI and tumor grading of IMCC in the training and validation sets. These represent the values of the rad_scores for all patients. The rad_scores showed statistically significant differences between MVI+ and MVI− and between high grading and low grading in the training and validation sets (all P<0.001) (Table 1). The rad_score yielded AUCs of 0.806 (95% CI: 0.742–0.870) in the training set and 0.783 (95% CI: 0.674–0.893) in the validation set for the prediction of MVI. For the prediction of tumor grading, the AUCs were 0.806 (95% CI: 0.737–0.874) and 0.779 (95% CI: 0.664–0.894) in the training and validation sets, respectively (Table 3).
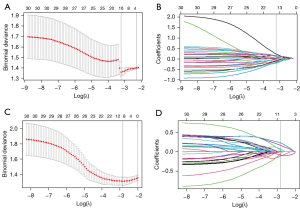
Table 3
Model | AUC | Accuracy, % | Sensitivity, % | Specificity, % | PPV, % | NPV, % |
---|---|---|---|---|---|---|
MVI | ||||||
Training set | ||||||
Clinical model | 0.787 (0.716, 0.857) |
71.26 (63.76, 77.99) |
83.75 (73.82, 91.05) |
59.77 48.71, 70.15) |
65.69 (55.63, 74.81) |
80.00 (68.23, 88.90) |
Radiomics model | 0.806 (0.742, 0.870) |
71.26 (63.76, 77.99) |
71.25 (60.05, 80.82) |
71.26 (60.57, 80.46) |
69.51 (58.36, 79.20) |
72.94 (62.21, 82.01) |
Nomogram model | 0.874 (0.822, 0.926) |
77.25 (70.13, 83.37) |
73.75 (62.71, 82.96) |
80.46 (70.57, 88.19) |
77.63 (66.62, 86.40) |
76.92 (66.91, 85.11) |
Validation set | ||||||
Clinical model | 0.738 (0.618, 0.858) |
69.12 (56.74, 79.76) |
82.76 (64.23, 94.15) |
58.97 (42.10, 74.43) |
60.00 (43.33, 75.14) |
82.14 (63.11, 93.93) |
Radiomics model | 0.783 (0.674, 0.893) |
69.12 (56.74, 79.76) |
62.07 (42.26, 79.31) |
74.36 (57.87, 86.96) |
64.29 (44.06, 81.36) |
72.50 (56.11, 85.40) |
Nomogram model | 0.869 (0.783, 0.955) |
79.41 (67.88, 88.26) |
75.86 (56.46, 89.70) |
82.05 (66.47, 92.46) |
75.86 (56.46, 89.70) |
82.05 (66.47, 92.46) |
Tumor grading | ||||||
Training set | ||||||
Clinical model | 0.650 (0.565, 0.734) |
63.47 (55.68, 70.78) |
55.34 (45.22, 65.14) |
76.56 (64.31, 86.25) |
79.17 (67.98, 87.84) |
51.58 (41.10, 61.96) |
Radiomics model | 0.806 (0.737, 0.874) |
74.25 (66.92, 80.70) |
85.44 (77.12, 91.61) |
56.25 (43.28, 68.63) |
75.86 (67.04, 83.32) |
70.59 (56.17, 82.51) |
Nomogram model | 0.827 (0.763, 0.891) |
76.65 (69.49, 82.84) |
85.44 (77.12, 91.61) |
62.50 (49.51, 74.30) |
78.57 (69.81, 85.76) |
72.73 (59.04, 83.86) |
Validation set | ||||||
Clinical model | 0.734 (0.614, 0.854) |
70.59 (58.29, 81.02) |
58.33 (40.76, 74.49) |
84.38 (67.21, 94.72) |
80.77 (60.65, 93.45) |
64.29 (48.03, 78.45) |
Radiomics model | 0.779 (0.664, 0.894) |
72.06 (59.85, 82.26) |
80.56 (63.98, 91.81) |
62.50 (43.69, 78.90) |
70.73 (54.46, 83.87) |
74.07 (53.71, 88.89) |
Nomogram model | 0.848 (0.759, 0.937) |
73.52 (61.42, 83.50) |
77.78 (60.85, 89.88) |
68.75 (49.99, 83.88) |
73.68 (56.90, 86.60) |
73.33 (54.11, 87.72) |
Data in parentheses are 95% confidence intervals. MVI, microvascular invasion; AUC, area under the receiver operating characteristic curve; PPV, positive predictive value; NPV, negative predictive value.
Performance and comparison of the nomograms
Nomograms were constructed by incorporating significant MRI features and the rad_scores (Figure 4). All of the calibration curves for the nomograms showed that the predicted probabilities of the nomogram were closely aligned with the actual MVI and tumor grading estimates, with P values of 0.895 and 0.451 in the training set, respectively (Figure S3). The AUCs of the nomogram for predicting MVI were 0.874 (95% CI: 0.822–0.926) for the training set and 0.869 (95% CI: 0.783–0.955) for the validation set, whereas the AUCs of the nomogram for predicting tumor grading were 0.827 (95% CI: 0.763–0.891) for the training set and 0.848 (95% CI: 0.759–0.937) for the validation set (Table 3). Furthermore, the nomograms had significantly higher AUCs than the clinical models in both the training set (MVI, 0.874 vs. 0.787, P<0.001; tumor grading, 0.827 vs. 0.650, P<0.001) and validation set (MVI, 0.869 vs. 0.738, P=0.008; tumor grading, 0.848 vs. 0.734, P=0.002) for the prediction of both MVI and tumor grading (Figure 5, Table S5). The AUC difference between each model is provided in Table S5. The nomogram score for each patient is shown in Figure S4. The corresponding accuracy, sensitivity, specificity, PPV, and NPV are presented in Table 3.
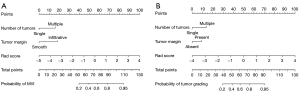
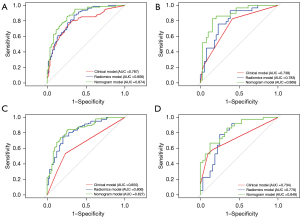
Clinical utility
DCA was performed for the nomograms for predicting the presence of MVI and tumor grading, which reflected that the clinical utility and was presented in the training set. As shown in Figure S5, using the nomogram and rad_scores for MVI prediction added more benefit than using the “treat-all strategy” or “treat-none strategy” within a certain range of thresholds (the range of the radiomics model and nomogram was between 10% and 95%). Similar results were found in the prediction of tumor grading (radiomics model, between 10% and 95%; nomogram, >10%).
Discussion
In our study, we constructed 2 nomograms incorporating the rad_scores and MRI morphological risk factors for the prediction of MVI and tumor grading of IMCC. Our findings demonstrated that the radiomics features and MRI morphological features had an acceptable ability to discriminate the pathological features of the tumor. The calibration curves and DCA showed the good clinical utility of these easy-to-use nomogram prediction models for MVI and tumor grading.
The rad_scores based on T2WI-FS and DWI showed better performance outcomes (AUCs of 0.783 and 0.806, respectively) in the prediction of MVI than the clinical model (AUCs of 0.787 and 0.738, respectively) in the study. The nomogram combining rad_scores and significant MRI morphological features had the highest diagnostic performance for the prediction of MVI, with AUCs of 0.874 and 0.869 in the training and validation sets, respectively. Zhou et al. (20) utilized a set of 7 wavelet features based on gadolinium ethoxybenzyl diethylenetriamine pentaacetic acid (Gd-DTPA)-enhanced MRI for the prediction of MVI, and the radiomics model reached AUCs of 0.850–0.873. Qian et al. (18) established a nomogram incorporating intrahepatic duct dilatation, tumor size, and rad_score (DWI, T2WI-FS, T1WI, AP, PVP, and DP) with an AUC range of 0.819 to 0.953, and the diagnostic performance was similar to that of our nomogram.
To date, to the best of our knowledge, no study has employed radiomics features based on MRI to predict tumor grading of IMCC. In our study, the radiomics model achieved AUCs of 0.806 and 0.779 in the training and validation sets, respectively, and the nomogram achieved the best predictive ability (AUCs of 0.827 and 0.848, respectively). Rad_scores based on positron emission tomography/computed tomography (PET/CT) (27) and ultrasound (28) have been used to preoperatively predict tumor grading, with AUCs ranging from 0.71 to 0.78. However, the case number was limited (74 to 128 patients) and a validation test was lacking, which may have led to a possible risk of data overfitting. We hypothesized that the radiomics features of MRI are superior to those of other examination devices, but further validation is needed.
MRMR is used to select radiomics features that can provide less redundancy and more reliable coefficients in the classifier (29). In addition, the LASSO method is an excellent approach for feature screening, obtaining good features of both ridge regression and subset screening and enabling the panel of selected features to be combined into rad_scores (30). Therefore, optimal radiomics features were selected in this way in our study. Alternatively, it is worth mentioning that the radiomics features extracted from T2WI-FS (11/16 for MVI and 6/9 for tumor grading) and wavelet features (11/16 for MVI and 6/9 for tumor grading) exhibited higher weights in the rad_scores. These results showed that wavelet transformation can further reflect the spatial heterogeneity across multiple dimensions, which is consistent with previous studies (31,32). We also assume that radiomics features extracted from T2WI-FS are more useful for predicting the aggressive behaviors of tumors than those extracted from DWI. This could be attributed to the fact that T2WI-FS has a higher signal-to-noise ratio, spatial resolution, and image contrast than DWI images (33).
Multivariate logistic regression analysis showed that multiple tumors was an independent factor in the prediction of both MVI and tumor grading, which was consistent with previous studies (9,34,35). The presence of multiple tumors is a visible sign of invasiveness and an external manifestation of the tumor’s poor biological behavior. Tumor margin is an important predictor of MVI. Xiang et al. (34) reported that a blurry or infiltrative contour of the tumor on CT is a significant risk factor for MVI. Our results concur with this report. Tumor necrosis is another important predictive factor associated with pathological grading in the study. A common hypothesis is that the rapid growth of malignant cells, especially in the more aggressive types of cancer, with the subsequent generation of hypoxic conditions, exceeds their own blood supply and leads to necrosis of tissue areas (36). The most reliable criterion for evaluating tumor necrosis is pathological diagnosis; therefore, radiographically assessing the degree of consistency of necrosis with pathological specimens requires validation.
Our study has several limitations. First, this was a retrospective study at a single institute and may have selection bias. Therefore, external validation is anticipated in the future through a public database through a multisite study to assess the practicability of the results. Second, we did not include contrast-enhanced (CE) sequences because the patients enrolled in our study used different liver contrast agents, including liver-specific contrast agents and extracellular contrast agents. Although the nomograms in our study obtained a favorable performance in the prediction of MVI and tumor grading, CE sequences, which would show further characteristics of IMCC, might contain more information and needs further analysis. Finally, this study was also limited by the heterogeneity of the MRI protocols, which were not standardized because of the retrospective design of the study.
Conclusions
The nomograms based on MRI radiomics features and morphological features may be helpful for preoperatively predicting MVI and tumor grading in IMCC, which may help clinicians to choose individual and optimal surgical strategies to improve clinical outcomes.
Acknowledgments
Funding: None.
Footnote
Reporting Checklist: The authors have completed the TRIPOD reporting checklist. Available at https://qims.amegroups.com/article/view/10.21037/qims-23-11/rc
Conflicts of Interest: All authors have completed the ICMJE uniform disclosure form (available at https://qims.amegroups.com/article/view/10.21037/qims-23-11/coif). The authors have no conflicts of interest to declare.
Ethical Statement: The authors are accountable for all aspects of the work in ensuring that questions related to the accuracy or integrity of any part of the work are appropriately investigated and resolved. The study was conducted in accordance with the Declaration of Helsinki (as revised in 2013). The study was approved by the Ethics Committee of the National Cancer Center/Cancer Hospital, Chinese Academy of Medical Sciences and Peking Union Medical College, and the requirement for individual consent for this retrospective analysis was waived.
Open Access Statement: This is an Open Access article distributed in accordance with the Creative Commons Attribution-NonCommercial-NoDerivs 4.0 International License (CC BY-NC-ND 4.0), which permits the non-commercial replication and distribution of the article with the strict proviso that no changes or edits are made and the original work is properly cited (including links to both the formal publication through the relevant DOI and the license). See: https://creativecommons.org/licenses/by-nc-nd/4.0/.
References
- Cerrito L, Ainora ME, Borriello R, Piccirilli G, Garcovich M, Riccardi L, Pompili M, Gasbarrini A, Zocco MA. Contrast-Enhanced Imaging in the Management of Intrahepatic Cholangiocarcinoma: State of Art and Future Perspectives. Cancers (Basel) 2023;15:3393. [Crossref] [PubMed]
- Kupietzky A, Ariche A. Surgical Aspects of Intrahepatic Cholangiocarcinoma. Cancers (Basel) 2022;14:6265. [Crossref] [PubMed]
- Razumilava N, Gores GJ. Cholangiocarcinoma. Lancet 2014;383:2168-79. [Crossref] [PubMed]
- Guglielmi A, Ruzzenente A, Campagnaro T, Pachera S, Valdegamberi A, Nicoli P, Cappellani A, Malfermoni G, Iacono C. Intrahepatic cholangiocarcinoma: prognostic factors after surgical resection. World J Surg 2009;33:1247-54. [Crossref] [PubMed]
- de Jong MC, Nathan H, Sotiropoulos GC, Paul A, Alexandrescu S, Marques H, et al. Intrahepatic cholangiocarcinoma: an international multi-institutional analysis of prognostic factors and lymph node assessment. J Clin Oncol 2011;29:3140-5. [Crossref] [PubMed]
- Doussot A, Gonen M, Wiggers JK, Groot-Koerkamp B, DeMatteo RP, Fuks D, Allen PJ, Farges O, Kingham TP, Regimbeau JM, D'Angelica MI, Azoulay D, Jarnagin WR. Recurrence Patterns and Disease-Free Survival after Resection of Intrahepatic Cholangiocarcinoma: Preoperative and Postoperative Prognostic Models. J Am Coll Surg 2016;223:493-505.e2. [Crossref] [PubMed]
- Xu X, Zhang HL, Liu QP, Sun SW, Zhang J, Zhu FP, Yang G, Yan X, Zhang YD, Liu XS. Radiomic analysis of contrast-enhanced CT predicts microvascular invasion and outcome in hepatocellular carcinoma. J Hepatol 2019;70:1133-44. [Crossref] [PubMed]
- Atanasov G, Dietel C, Feldbrügge L, Benzing C, Krenzien F, Brandl A, Mann E, Englisch JP, Schierle K, Robson SC, Splith K, Morgul MH, Reutzel-Selke A, Jonas S, Pascher A, Bahra M, Pratschke J, Schmelzle M. Tumor necrosis and infiltrating macrophages predict survival after curative resection for cholangiocarcinoma. Oncoimmunology 2017;6:e1331806. [Crossref] [PubMed]
- Chen Y, Liu H, Zhang J, Wu Y, Zhou W, Cheng Z, Lou J, Zheng S, Bi X, Wang J, Guo W, Li F, Wang J, Zheng Y, Li J, Cheng S, Zeng Y, Liu J. Prognostic value and predication model of microvascular invasion in patients with intrahepatic cholangiocarcinoma: a multicenter study from China. BMC Cancer 2021;21:1299. [Crossref] [PubMed]
- Ji GW, Jiao CY, Xu ZG, Li XC, Wang K, Wang XH. Development and validation of a gradient boosting machine to predict prognosis after liver resection for intrahepatic cholangiocarcinoma. BMC Cancer 2022;22:258. [Crossref] [PubMed]
- Jiang C, Zhao L, Xin B, Ma G, Wang X, Song S. (18)F-FDG PET/CT radiomic analysis for classifying and predicting microvascular invasion in hepatocellular carcinoma and intrahepatic cholangiocarcinoma. Quant Imaging Med Surg 2022;12:4135-50. [Crossref] [PubMed]
- Erstad DJ, Tanabe KK. Prognostic and Therapeutic Implications of Microvascular Invasion in Hepatocellular Carcinoma. Ann Surg Oncol 2019;26:1474-93. [Crossref] [PubMed]
- Lu WF, Chen PQ, Yan K, Wu YC, Liang L, Yuan JY, Fu Y, Zhang HB. Synergistic impact of resection margin and microscopic vascular invasion for patients with HBV-related intrahepatic cholangiocarcinoma. Expert Rev Gastroenterol Hepatol 2021;15:575-82. [Crossref] [PubMed]
- Martin SP, Ruff S, Diggs LP, Drake J, Ayabe RI, Brown ZJ, Wach MM, Steinberg SM, Davis JL, Hernandez JM. Tumor grade and sex should influence the utilization of portal lymphadenectomy for early stage intrahepatic cholangiocarcinoma. HPB (Oxford) 2019;21:419-24. [Crossref] [PubMed]
- Heimbach JK, Sanchez W, Rosen CB, Gores GJ. Trans-peritoneal fine needle aspiration biopsy of hilar cholangiocarcinoma is associated with disease dissemination. HPB (Oxford) 2011;13:356-60. [Crossref] [PubMed]
- Ma X, Liu L, Fang J, Rao S, Lv L, Zeng M, Shi Y, Yang C. MRI features predict microvascular invasion in intrahepatic cholangiocarcinoma. Cancer Imaging 2020;20:40. [Crossref] [PubMed]
- Zhou Y, Wang X, Xu C, Zhou G, Liu X, Gao S, Xu P. Mass-forming intrahepatic cholangiocarcinoma: Can diffusion-weighted imaging predict microvascular invasion? J Magn Reson Imaging 2019;50:315-24. [Crossref] [PubMed]
- Qian X, Lu X, Ma X, Zhang Y, Zhou C, Wang F, Shi Y, Zeng M. A Multi-Parametric Radiomics Nomogram for Preoperative Prediction of Microvascular Invasion Status in Intrahepatic Cholangiocarcinoma. Front Oncol 2022;12:838701. [Crossref] [PubMed]
- Lambin P, Leijenaar RTH, Deist TM, Peerlings J, de Jong EEC, van Timmeren J, Sanduleanu S, Larue RTHM, Even AJG, Jochems A, van Wijk Y, Woodruff H, van Soest J, Lustberg T, Roelofs E, van Elmpt W, Dekker A, Mottaghy FM, Wildberger JE, Walsh S. Radiomics: the bridge between medical imaging and personalized medicine. Nat Rev Clin Oncol 2017;14:749-62. [Crossref] [PubMed]
- Zhou Y, Zhou G, Zhang J, Xu C, Wang X, Xu P. Radiomics signature on dynamic contrast-enhanced MR images: a potential imaging biomarker for prediction of microvascular invasion in mass-forming intrahepatic cholangiocarcinoma. Eur Radiol 2021;31:6846-55. [Crossref] [PubMed]
- Li H, Wang L, Zhang J, Duan Q, Xu Y, Xue Y. Evaluation of microvascular invasion of hepatocellular carcinoma using whole-lesion histogram analysis with the stretched-exponential diffusion model. Br J Radiol 2022;95:20210631. [Crossref] [PubMed]
- Koo TK, Li MY. A Guideline of Selecting and Reporting Intraclass Correlation Coefficients for Reliability Research. J Chiropr Med 2016;15:155-63. Erratum in: J Chiropr Med 2017;16:346. [Crossref] [PubMed]
- van Griethuysen JJM, Fedorov A, Parmar C, Hosny A, Aucoin N, Narayan V, Beets-Tan RGH, Fillion-Robin JC, Pieper S, Aerts HJWL. Computational Radiomics System to Decode the Radiographic Phenotype. Cancer Res 2017;77:e104-7. [Crossref] [PubMed]
- Wang JZ. Wavelets and imaging informatics: a review of the literature. J Biomed Inform 2001;34:129-41. [Crossref] [PubMed]
- Shao C, Chen J, Chen J, Shi J, Huang L, Qiu Y. Histological classification of microvascular invasion to predict prognosis in intrahepatic cholangiocarcinoma. Int J Clin Exp Pathol 2017;10:7674-81.
- Washington MK, Berlin J, Branton PA, Burgart LJ, Carter DK, Compton CC, Frankel WL, Jessup JM, Kakar S, Minsky B, Nakhleh RE, Vauthey JN. Protocol for the examination of specimens from patients with carcinoma of the intrahepatic bile ducts. Arch Pathol Lab Med 2010;134:e14-8. [Crossref] [PubMed]
- Fiz F, Masci C, Costa G, Sollini M, Chiti A, Ieva F, Torzilli G, Viganò L. PET/CT-based radiomics of mass-forming intrahepatic cholangiocarcinoma improves prediction of pathology data and survival. Eur J Nucl Med Mol Imaging 2022;49:3387-400. [Crossref] [PubMed]
- Peng YT, Zhou CY, Lin P, Wen DY, Wang XD, Zhong XZ, Pan DH, Que Q, Li X, Chen L, He Y, Yang H. Preoperative Ultrasound Radiomics Signatures for Noninvasive Evaluation of Biological Characteristics of Intrahepatic Cholangiocarcinoma. Acad Radiol 2020;27:785-97. [Crossref] [PubMed]
- Lu W, Zhong L, Dong D, Fang M, Dai Q, Leng S, Zhang L, Sun W, Tian J, Zheng J, Jin Y. Radiomic analysis for preoperative prediction of cervical lymph node metastasis in patients with papillary thyroid carcinoma. Eur J Radiol 2019;118:231-8. [Crossref] [PubMed]
- Huang YQ, Liang CH, He L, Tian J, Liang CS, Chen X, Ma ZL, Liu ZY. Development and Validation of a Radiomics Nomogram for Preoperative Prediction of Lymph Node Metastasis in Colorectal Cancer. J Clin Oncol 2016;34:2157-64. [Crossref] [PubMed]
- Wilson R, Devaraj A. Radiomics of pulmonary nodules and lung cancer. Transl Lung Cancer Res 2017;6:86-91. [Crossref] [PubMed]
- Zheng YM, Yuan MG, Zhou RQ, Hou F, Zhan JF, Liu ND, Hao DP, Dong C. A computed tomography-based radiomics signature for predicting expression of programmed death ligand 1 in head and neck squamous cell carcinoma. Eur Radiol 2022;32:5362-70. [Crossref] [PubMed]
- Xu Q, Zhu Q, Liu H, Chang L, Duan S, Dou W, Li S, Ye J. Differentiating Benign from Malignant Renal Tumors Using T2- and Diffusion-Weighted Images: A Comparison of Deep Learning and Radiomics Models Versus Assessment from Radiologists. J Magn Reson Imaging 2022;55:1251-9. [Crossref] [PubMed]
- Xiang F, Wei S, Liu X, Liang X, Yang L, Yan S. Radiomics Analysis of Contrast-Enhanced CT for the Preoperative Prediction of Microvascular Invasion in Mass-Forming Intrahepatic Cholangiocarcinoma. Front Oncol 2021;11:774117. [Crossref] [PubMed]
- Addeo P, Jedidi I, Locicero A, Faitot F, Oncioiu C, Onea A, Bachellier P. Prognostic Impact of Tumor Multinodularity in Intrahepatic Cholangiocarcinoma. J Gastrointest Surg 2019;23:1801-9. [Crossref] [PubMed]
- Atanasov G, Schierle K, Hau HM, Dietel C, Krenzien F, Brandl A, Wiltberger G, Englisch JP, Robson SC, Reutzel-Selke A, Pascher A, Jonas S, Pratschke J, Benzing C, Schmelzle M. Prognostic Significance of Tumor Necrosis in Hilar Cholangiocarcinoma. Ann Surg Oncol 2017;24:518-25. [Crossref] [PubMed]