A tri-phasic relationship between T2 relaxation time and magnetic resonance imaging (MRI)-derived apparent diffusion coefficient (ADC)
We recently described a negative correlation between T2 relaxation time (T2 time) and magnetic resonance imaging (MRI)-derived apparent diffusion coefficient (ADC) when T2 time is in the range of those of liver and spleen (1,2). However, body fluid such as gallbladder fluid has both very long T2 time and very high ADC (Figure 1) (3,5). When myometrium tumors are very highly hypertensive such as the cases of leiomyoma cystic degeneration and myxoid degeneration, the relationship between T2 weighted signal intensity and diffusion is similar to that of a normal gallbladder, i.e., T2 weighted signal highly hypertensive without diffusion restriction (1,6). Therefore, when a tissue has a long T2 time, longer T2 may be associated with higher diffusion measures. That is, depending on the T2 time value, a positive correlation may exist between long T2 time and ADC (2). This point is further supported by examples of parotid tumors, as illustrated in Figure 2 (2,7-9). Figure 2 is based on our parotid tumor literature search results where we searched articles reporting both quantitative ADC measure and T2 time value or quantitative T2 signal intensity. Figure 2 shows almost linear relationships between parotid tumor mean T2 relaxation time (or T2 weighted mean image signal) and parotid tumor mean ADC.
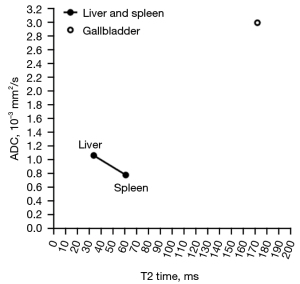
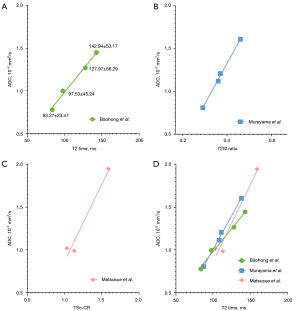
We add the data of Baohong et al. (7) onto Figure 1, and the results are shown in Figure 3. Figure 3 tentatively shows a tri-phasic (or bi-phasic) correlation between T2 time and ADC.
To further clarify the possible tri-phasic correlation between T2 time and ADC, we added more data onto Figure 3, and thus we further have Figure 4 (10-15). We arbitrarily divide T2 time into short T2 time band [<60 millisecond (ms)], intermediate T2 time band (60–80 ms), and long T2 time band (>80 ms). For the short T2 time band, we argue that ‘there is a negative correlation between T2 time and ADC’. This is evidently shown with normal liver tissue and normal spleen tissue (1,2,16). In addition to the data shown in Figure 4, it is well known that most of the liver and pancreas cancerous tissues have increased T2 time and decreased ADC (17-21) (Figures S1,S2). Liver fibrosis has been well shown to have increased T2 time (10,11,22-24) and lowered ADC (20).
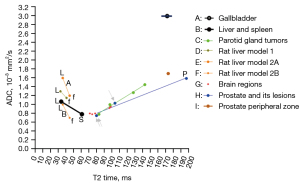
For the long T2 time band, we can argue that ‘there is a positive correlation between T2 time and ADC’. In addition to parotid gland tumors, there are numerous examples to support this claim (25-30), and the correlations between T2 time and ADC are mostly strong (Figures 2,5-7). In fact, the strong correlation between T2 time and ADC value may suggest, for the long T2 time band, T2 time’s contribution to ADC may be dominant, while tissue diffusion differences may be much smaller than ADC measure demonstrates (Figure 2).

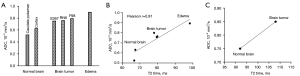
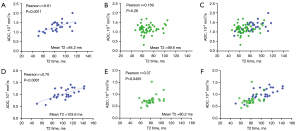
The range of intermediate T2 time band cannot be validated yet, but it is likely that the association between T2 time and ADC may be insensitive around the T2 time of 70 ms (at 3T). Data of Figure 7 support this notion (13,28). For MRI hyperintense uterine fibroid (Figure 7A, mean T2 time =84.2 ms), there was a strong positive correlation between T2 time and ADC. For MRI intermediate-intensity uterine fibroid (Figure 7B, mean T2 time =69.6 ms), the correlation between T2 time and ADC was almost non-existent. For prostatitis (Figure 7C, mean T2 time =103.6 ms), there was a strong positive correlation between T2 time and ADC. For prostate cancer (Figure 7E, mean T2 time =80.62 ms), there was a weak correlation between T2 time and ADC. Note that mean T2 time =69.6 ms for intermediate-intensity uterine fibroid is right in the middle of the T2 time insensitive band [at the T2 time of around 70 ms, 1.5T value and 3.0 value are similar (4)], while T2 time =80.2 ms for prostate cancer is close to the long T2 time band. Whether the intermediate T2 time band spans from 60 to 80 ms at 3T requires further studies.
Perfusion of course does contribute to ADC measure. One typical example is the richly perfused kidneys. Both kidney cortex and medulla have a similar ADC of around 1.8×10-3 mm2/s (31,32). While kidney cortex has much higher blood perfusion than kidney medulla (for example, Vallée et al.: 2.54 vs. 1.08 mL/min/g; Winter et al.: 2.98 vs. 1.53 mL/min/g) (33,34). Kidney medulla has a longer T2 time than the cortex (138 vs. 121 ms at 3T) (4,35,36). It is possible that longer medulla T2 time also contributes to the medulla’s not-lower ADC than that of the cortex. Kidney cortex ADC of around 1.8×10-3 mm2/s is much higher than those of liver and spleen. Kidney ADC is distinctly high compared with other issues of similar T2 time. It is likely that the high kidney cortex perfusion (more than double that of liver perfusion) (33,34,37) contributes to the kidney cortex high ADC.
Another example of T2 time contribution to ADC measurement is mucinous fluid/semi-fluid containing tissues. Myxoid degenerated leiomyoma has long T2 time and high ADC value (1,6). Another example is prostate peripheral zone, which has an ADC of around 1.7×10-3 mm2/s (15,38) and this value is much higher than those of liver and spleen. The prostate peripheral zone contains the majority of prostatic glandular tissue and has a much longer T2 value than those of liver and spleen (Figure 4) (13,14). It is highly likely that the long T2 value of prostate peripheral zone contributes to its high ADC value. On the other hand, despite the long T2 time of the prostate peripheral zone, tissue structures still hinder diffusion, thus the prostate peripheral zone still has a lower ADC than those of free body water (such as gallbladder fluid).
Human brain tumors commonly have mixed components including active tumor tissue, necrosis and cystic change, hemorrhage and hemosiderin deposition, calcification, edema, etc. Knowing that brain tumors have increased ADC (as compared to normal brain tissues) (26,27,30,39) has clinical implications. Some authors choose the lowest value region of ADC map to quantify ADC value for the tumor (40). Our analysis suggests this may not be the most appropriate approach, as active tumor may have high ADC value.
In conclusion, while ADC measure is influenced by numerous factors including perfusion, tissue structure and cellularity, T2* effect (41,42), viscosity of internal fluid or semi-fluid, etc., empirical observations support a tri-phasic (or bi-phasic) relationship between T2 time and ADC. When T2 time is longer than 80 ms (at 3T), a strong positive correlation between T2 time and ADC is observed, and it is possible that T2 time contributes dominantly to ADC measure in many scenarios. For interpretation of ADC value of any tissue, this tissue’s T2 time should be always referred. Low ADC measure is not necessarily associated with true diffusion restriction. A tissue likely measures a low ADC if its T2 time is close to 70 ms (or its equivalent values at other magnetic fields). On the other hand, a tissue likely measures a high ADC if its T2 time is far away from 70 ms (or its equivalent values at other magnetic fields). A cancerous tissue may not be necessarily associated with diffusion restriction even when its ADC measure is low.
Acknowledgments
The authors thank Dr. Wei-Ling Yu, at the Chinese University of Hong Kong, for the help during the manuscript preparation.
Funding: This work was supported by
Footnote
Conflicts of Interest: Both authors have completed the ICMJE uniform disclosure form (available at https://qims.amegroups.com/article/view/10.21037/qims-23-1342/coif). YXJW serves as the Editor-in-Chief of Quantitative Imaging in Medicine and Surgery. FZM has no conflicts of interest to declare.
Ethical Statement: The authors are accountable for all aspects of the work in ensuring that questions related to the accuracy or integrity of any part of the work are appropriately investigated and resolved.
Open Access Statement: This is an Open Access article distributed in accordance with the Creative Commons Attribution-NonCommercial-NoDerivs 4.0 International License (CC BY-NC-ND 4.0), which permits the non-commercial replication and distribution of the article with the strict proviso that no changes or edits are made and the original work is properly cited (including links to both the formal publication through the relevant DOI and the license). See: https://creativecommons.org/licenses/by-nc-nd/4.0/.
References
- Wáng YXJ, Zhao KX, Ma FZ, Xiao BH. The contribution of T2 relaxation time to MRI-derived apparent diffusion coefficient (ADC) quantification and its potential clinical implications. Quant Imaging Med Surg 2023; [Crossref]
-
.Wang YXJ Complicated relationships between tissue T2 relaxation time and in vivo tissue diffusion measures, depending on the ranges of T2 value. [Submitted on 19 Jun 2023] arXiv:2306.10657 - Kim BR, Song JS, Choi EJ, Hwang SB, Hwang HP. Diffusion-Weighted Imaging of Upper Abdominal Organs Acquired with Multiple B-Value Combinations: Value of Normalization Using Spleen as the Reference Organ. Korean J Radiol 2018;19:389-396. [Crossref] [PubMed]
- de Bazelaire CM, Duhamel GD, Rofsky NM, Alsop DC. MR imaging relaxation times of abdominal and pelvic tissues measured in vivo at 3.0 T: preliminary results. Radiology 2004;230:652-9. [Crossref] [PubMed]
- Mohajeri S, Ijare OB, Bezabeh T, King SB, Thomas MA, Minuk G, Lipschitz J, Kirkpatrick I, Smith M, Smith IC. In vivo 1H MRS of human gallbladder bile at 3 T in one and two dimensions: detection and quantification of major biliary lipids. NMR Biomed. 2014;27:1192-202. [Crossref] [PubMed]
- DeMulder D, Ascher SM. Uterine Leiomyosarcoma: Can MRI Differentiate Leiomyosarcoma From Benign Leiomyoma Before Treatment? AJR Am J Roentgenol 2018;211:1405-15. [Crossref] [PubMed]
- Baohong W, Jing Z, Zanxia Z, Kun F, Liang L, Eryuan G, Yong Z, Fei H, Jingliang C, Jinxia Z. T2 mapping and readout segmentation of long variable echo-train diffusion-weighted imaging for the differentiation of parotid gland tumors. Eur J Radiol 2022;151:110265. [Crossref] [PubMed]
- Murayama Y, Kamitani T, Sagiyama K, Yamasaki Y, Hida T, Matsuura Y, Yasumatsu R, Yamamoto H, Yabuuchi H, Ishigami K. Evaluation of MR imaging findings differentiating parotid basal cell adenomas from other parotid tumors. Eur J Radiol 2021;144:109980. [Crossref] [PubMed]
- Matsusue E, Fujihara Y, Matsuda E, Tokuyasu Y, Nakamoto S, Nakamura K, Ogawa T. Differentiating parotid tumors by quantitative signal intensity evaluation on MR imaging. Clin Imaging 2017;46:37-43. [Crossref] [PubMed]
- Liu JY, Cai YY, Ding ZY, Zhou ZY, Lv M, Liu H, Zheng LY, Li L, Luo YH, Xiao EH. Characterizing Fibrosis and Inflammation in a Partial Bile Duct Ligation Mouse Model by Multiparametric Magnetic Resonance Imaging. J Magn Reson Imaging 2022;55:1864-1874. [Crossref] [PubMed]
- Zhang H, Yang Q, Yu T, Chen X, Huang J, Tan C, Liang B, Guo H. Comparison of T2, T1rho, and diffusion metrics in assessment of liver fibrosis in rats. J Magn Reson Imaging 2017;45:741-750. [Crossref] [PubMed]
- Ma S, Nguyen CT, Han F, Wang N, Deng Z, Binesh N, Moser FG, Christodoulou AG, Li D. Three-dimensional simultaneous brain T1, T2, and ADC mapping with MR Multitasking. Magn Reson Med 2020;84:72-88. [Crossref] [PubMed]
- Hepp T, Kalmbach L, Kolb M, Martirosian P, Hilbert T, Thaiss WM, Notohamiprodjo M, Bedke J, Nikolaou K, Stenzl A, Kruck S, Kaufmann S. T2 mapping for the characterization of prostate lesions. World J Urol 2022;40:1455-1461. [Crossref] [PubMed]
- Han D, Choi MH, Lee YJ, Kim DH. Feasibility of Novel Three-Dimensional Magnetic Resonance Fingerprinting of the Prostate Gland: Phantom and Clinical Studies. Korean J Radiol 2021;22:1332-1340. [Crossref] [PubMed]
- Hambrock T, Somford DM, Huisman HJ, van Oort IM, Witjes JA, Hulsbergen-van de Kaa CA, Scheenen T, Barentsz JO. Relationship between apparent diffusion coefficients at 3.0-T MR imaging and Gleason grade in peripheral zone prostate cancer. Radiology 2011;259:453-61. [Crossref] [PubMed]
- Yu WL, Xiao BH, Ma FZ, Zheng CJ, Tang SN, Wáng YXJ. Underestimation of the spleen perfusion fraction by intravoxel incoherent motion MRI. NMR Biomed 2023;36:e4987. [Crossref] [PubMed]
- Cao L, Chen J, Duan T, Wang M, Jiang H, Wei Y, Xia C, Zhou X, Yan X, Song B. Diffusion kurtosis imaging (DKI) of hepatocellular carcinoma: correlation with microvascular invasion and histologic grade. Quant Imaging Med Surg 2019;9:590-602. [Crossref] [PubMed]
- Kartalis N, Manikis GC, Loizou L, Albiin N, Zöllner FG, Del Chiaro M, Marias K, Papanikolaou N. Diffusion-weighted MR imaging of pancreatic cancer: A comparison of mono-exponential, bi-exponential and non-Gaussian kurtosis models. Eur J Radiol Open 2016;3:79-85. [Crossref] [PubMed]
- Padhani AR, Liu G, Koh DM, Chenevert TL, Thoeny HC, Takahara T, Dzik-Jurasz A, Ross BD, Van Cauteren M, Collins D, Hammoud DA, Rustin GJ, Taouli B, Choyke PL. Diffusion-weighted magnetic resonance imaging as a cancer biomarker: consensus and recommendations. Neoplasia 2009;11:102-25. [Crossref] [PubMed]
- Wáng YXJ, Wang X, Wu P, Wang Y, Chen W, Chen H, Li J. Topics on quantitative liver magnetic resonance imaging. Quant Imaging Med Surg 2019;9:1840-90. [Crossref] [PubMed]
- Wang Y, Chen ZE, Nikolaidis P, McCarthy RJ, Merrick L, Sternick LA, Horowitz JM, Yaghmai V, Miller FH. Diffusion-weighted magnetic resonance imaging of pancreatic adenocarcinomas: association with histopathology and tumor grade. J Magn Reson Imaging 2011;33:136-42. [Crossref] [PubMed]
- Guimaraes AR, Siqueira L, Uppal R, Alford J, Fuchs BC, Yamada S, Tanabe K, Chung RT, Lauwers G, Chew ML, Boland GW, Sahani DV, Vangel M, Hahn PF, Caravan P. T2 relaxation time is related to liver fibrosis severity. Quant Imaging Med Surg 2016;6:103-14. [Crossref] [PubMed]
- Kreft B, Dombrowski F, Block W, Bachmann R, Pfeifer U, Schild H. Evaluation of different models of experimentally induced liver cirrhosis for MRI research with correlation to histopathologic findings. Invest Radiol 1999;34:360-6. [Crossref] [PubMed]
- Takayama Y, Nishie A, Ishimatsu K, Ushijima Y, Fujita N, Kubo Y, Yoshizumi T, Kouhashi KI, Maehara J, Akamine Y, Ishigami K. Diagnostic potential of T1ρ and T2 relaxations in assessing the severity of liver fibrosis and necro-inflammation. Magn Reson Imaging 2022;87:104-12. [Crossref] [PubMed]
- Niu G, Yang J, Wang R, Dang S, Wu EX, Guo Y. MR imaging assessment of lumbar intervertebral disk degeneration and age-related changes: apparent diffusion coefficient versus T2 quantitation. AJNR Am J Neuroradiol 2011;32:1617-23. [Crossref] [PubMed]
- Eis M, Els T, Hoehn-Berlage M. High resolution quantitative relaxation and diffusion MRI of three different experimental brain tumors in rat. Magn Reson Med 1995;34:835-44. [Crossref] [PubMed]
- Wang S, Chen L, Feng Y, Yin T, Yu J, De Keyzer F, Peeters R, Van Ongeval C, Bormans G, Swinnen J, Soete J, Wevers M, Li Y, Ni Y. Development and characterization of a rat brain metastatic tumor model by multiparametric magnetic resonance imaging and histomorphology. Clin Exp Metastasis 2022;39:479-93. [Crossref] [PubMed]
- Verpalen IM, Anneveldt KJ, Vos PC, Edens MA, Heijman E, Nijholt IM, Dijkstra JR, Schutte JM, Franx A, Bartels LW, Moonen CTW, Boomsma MF. Use of multiparametric MRI to characterize uterine fibroid tissue types. MAGMA 2020;33:689-700. [Crossref] [PubMed]
- Kapoor R, Rangankar VP, Kumar D, Raina S, Revikumar A, Mohanan K. Apparent diffusion coefficient and T2* mapping on 3T MRI in normal and degenerative lumbar intervertebral discs. Pol J Radiol 2023;88:e275-85. [Crossref] [PubMed]
- Zhang H, Liu K, Ba R, Zhang Z, Zhang Y, Chen Y, Gu W, Shen Z, Shu Q, Fu J, Wu D. Histological and molecular classifications of pediatric glioma with time-dependent diffusion MRI-based microstructural mapping. Neuro Oncol 2023;25:1146-56. [Crossref] [PubMed]
- Rankin AJ, Allwood-Spiers S, Lee MMY, Zhu L, Woodward R, Kuehn B, Radjenovic A, Sattar N, Roditi G, Mark PB, Gillis KA. Comparing the interobserver reproducibility of different regions of interest on multi-parametric renal magnetic resonance imaging in healthy volunteers, patients with heart failure and renal transplant recipients. MAGMA 2020;33:103-12. [Crossref] [PubMed]
- Dillman JR, Benoit SW, Gandhi DB, Trout AT, Tkach JA, VandenHeuvel K, Devarajan P. Multiparametric quantitative renal MRI in children and young adults: comparison between healthy individuals and patients with chronic kidney disease. Abdom Radiol (NY) 2022;47:1840-52. [Crossref] [PubMed]
- Vallée JP, Lazeyras F, Khan HG, Terrier F. Absolute renal blood flow quantification by dynamic MRI and Gd-DTPA. Eur Radiol 2000;10:1245-52. [Crossref] [PubMed]
- Winter JD, St Lawrence KS, Cheng HL. Quantification of renal perfusion: comparison of arterial spin labeling and dynamic contrast-enhanced MRI. J Magn Reson Imaging 2011;34:608-15. [Crossref] [PubMed]
- Li X, Bolan PJ, Ugurbil K, Metzger GJ. Measuring renal tissue relaxation times at 7 T. NMR Biomed 2015;28:63-9. [Crossref] [PubMed]
- de Boer A, Harteveld AA, Stemkens B, Blankestijn PJ, Bos C, Franklin SL, Froeling M, Joles JA, Verhaar MC, van den Berg N, Hoogduin H, Leiner T. Multiparametric Renal MRI: An Intrasubject Test-Retest Repeatability Study. J Magn Reson Imaging 2021;53:859-73. [Crossref] [PubMed]
- Lemoine S, Papillard M, Belloi A, Rognant N, Fouque D, Laville M, Rouvière O, Juillard L. Renal perfusion: noninvasive measurement with multidetector CT versus fluorescent microspheres in a pig model. Radiology 2011;260:414-20. [Crossref] [PubMed]
- Morgan VA, Riches SF, Giles S, Dearnaley D, deSouza NM. Diffusion-weighted MRI for locally recurrent prostate cancer after external beam radiotherapy. AJR Am J Roentgenol 2012;198:596-602. [Crossref] [PubMed]
- Maier SE, Sun Y, Mulkern RV. Diffusion imaging of brain tumors. NMR Biomed 2010;23:849-64. [Crossref] [PubMed]
- Stuart C, Rabiei P, Lugo A, Arevalo O, Ocasio L, Mumtaz Syed M, Roy Riascos R, Zhu JJ, Cai C, Kamali A. Use of quantitative diffusion-weighted MR imaging (DWI) in differentiating between glioblastoma and primary central nervous system lymphoma in real-time exam interpretation. Neuro Neurosurg 2019;2: [Crossref]
- Chandarana H, Do RK, Mussi TC, Jensen JH, Hajdu CH, Babb JS, Taouli B. The effect of liver iron deposition on hepatic apparent diffusion coefficient values in cirrhosis. AJR Am J Roentgenol 2012;199:803-8. [Crossref] [PubMed]
- Xiao BH, Wáng YXJ. Different tissue types display different signal intensities on b = 0 images and the implications of this for intravoxel incoherent motion analysis: Examples from liver MRI. NMR Biomed 2021;34:e4522. [Crossref] [PubMed]