Carotid artery perivascular adipose tissue on magnetic resonance imaging: a potential indicator for carotid vulnerable atherosclerotic plaque
Introduction
Stroke is the second leading cause of death worldwide, accounting for 11.6% of all deaths (1). Carotid vulnerable atherosclerotic plaque is the major cause of ischemic stroke (2,3). Studies have shown that vulnerable features of carotid atherosclerotic plaques, such as intraplaque hemorrhage (IPH), fibrous cap rupture (FCR), and large lipid-rich necrotic core (LRNC), are effective predictors for ischemic stroke (2-4). Therefore, it is important to determine the indicators for carotid vulnerable plaques prior to occurrence of cerebrovascular events.
Biological studies have confirmed that inflammation is the key to the initiation and progression of atherosclerosis (5). In addition, due to the complex bidirectional interaction between the arterial vessel wall and its corresponding perivascular adipose tissue (PVAT), the inflammatory changes of PVAT are closely related to atherosclerotic vulnerable plaques (6,7). Pathophysiologically, the pro-inflammatory adipokine secreted by PVAT can spread directly to the blood vessel wall through paracrine and vascular secretion signaling pathways, thus causing vascular inflammation and atherosclerosis (8). Vascular inflammation will also induce the morphological changes of PVAT. Therefore, inflammation of PVAT might be a potential marker for vulnerable atherosclerosis (9,10).
Increasing evidence has shown that computed tomography angiography (CTA) can accurately identify and quantify inflammatory changes of PVAT associated with atherosclerosis and ischemic events. Antonopoulos et al. (10) measured fat attenuation index (FAI) based on CTA which represents the inflammatory changes of coronary PVAT and found that FAI was positively correlated with coronary atherosclerotic plaque burden. Investigators evaluated the perivascular fat density (PFD) of carotid artery on CTA and found that the increase of PFD was associated with unstable atherosclerosis (11,12) and the increased risk of cerebrovascular events (13,14). Since non-contrast enhanced computed tomography (CT) imaging can only provide Hounsfield unit (HU) value of tissues, it is not a comprehensive measurement for inflammatory status of PVAT. In contrast, magnetic resonance imaging (MRI) has better soft tissue contrast which might be beneficial for characterization of inflammatory status of PVAT. However, it is unclear if the signal intensity of PVAT measured by MRI could indicate the vulnerability of carotid atherosclerotic plaques. The aim of this study was to investigate the association between signal intensity of PVAT and vulnerable plaques in carotid arteries using multi-contrast magnetic resonance (MR) vessel wall imaging. We present this article in accordance with the STROBE reporting checklist (available at https://qims.amegroups.com/article/view/10.21037/qims-23-280/rc).
Methods
Study population
In this cross-sectional study, patients with symptomatic moderate-to-severe atherosclerotic stenosis (stenosis range, 50–99%) or asymptomatic severe atherosclerotic stenosis (stenosis range, 70–99%) in unilateral carotid artery referred to carotid endarterectomy (CEA) were recruited. The inclusion criteria pertained the procedure of CEA. Carotid multi-contrast MR vessel wall imaging was performed for all recruited patients within 1 week before CEA. The exclusion criteria were as follows: (I) hemorrhagic stroke within the recent 1 year; (II) acute ischemic stroke within the recent 6 weeks; (III) intracranial aneurysm and severe intracranial stenosis; (IV) history of carotid stenting; (V) brain tumor; (VI) history of radiotherapy in the neck; and (VII) contraindications to MRI examinations. The clinical information including age, sex, history of smoking, diabetes, hypertension, hyperlipidemia, and coronary heart disease was collected from the medical records. The study was conducted in accordance with the Declaration of Helsinki (as revised in 2013). The study was approved by the Medical Ethics Committee of Peking University Third Hospital, and all patients provided written informed consent before participation.
Carotid MRI
All recruited patients underwent carotid MR vessel wall imaging on a 3.0T MR scanner (uMR 780; United Imaging, Shanghai, China) with 8-channel carotid coil. The imaging protocol included 3-dimensional (3D) time-of-flight (ToF), 2-dimensional (2D) black-blood T1-weighted (T1w), 2D black-blood T2-weighted (T2w), and 3D simultaneous non-contrast angiography and intraplaque hemorrhage (SNAP) imaging sequences. All imaging sequences were acquired centered to the bifurcation of the index carotid artery which is defined as arteries with the moderate-to-severe atherosclerotic stenosis referred to CEA. The imaging parameters are detailed in Table 1. In addition, the image acquisition plane of all sequences was transverse, the excitation number of each sequence was 1, the slice gap of each sequence was 0, and the fat saturation technique was applied to T1w and T2w imaging sequences.
Table 1
Imaging parameters | Multi-contrast imaging protocol | |||
---|---|---|---|---|
ToF | T1w | T2w | SNAP | |
Sequence | GRE | FSE | FSE | GRE |
Fat saturation | No | Yes | Yes | No |
Repetition time, ms | 20.0 | 855.0 | 2,500.0 | 10.0 |
Echo time, ms | 4.1 | 11.9 | 72.8 | 3.8 |
Flip angle, ° | 20 | 90 | 90 | 11 |
Field of view, cm2 | 14×14 | 14×14 | 14×14 | 14×14 |
In plane resolution, mm2 | 0.55×0.55 | 0.55×0.55 | 0.55×0.55 | 0.73×0.73 |
Slice thickness, mm | 2 | 2 | 2 | 2 |
MRI, magnetic resonance imaging; ToF, time-of-flight; T1w, T1-weighted; T2w, T2-weighted; SNAP, simultaneous non-contrast angiography and intraplaque hemorrhage; GRE, gradient-recalled echo; FSE, fast spin echo.
MRI analysis
The multi-contrast MR vessel wall images of the index carotid artery were reviewed by 2 radiologists with >5-year experience in neurovascular imaging utilizing custom-designed software (Vessel Explorer 2; TS Imaging Healthcare, Beijing, China) with consensus blinded to clinical information. A radiologist (Huo R) performed the primary review and the other radiologist (Xu H) conducted secondary review for each axial slice. If there was disagreement between primary and secondary review, a peer review was performed by a senior radiologist (Zhao X) with >10-year experience in neurovascular imaging. According to the sharpness and contrast between the vessel wall and the surrounding fat tissues and between normal wall and plaque components, the quality of carotid MR vessel wall images was evaluated using a 4-point scale: 1, poor; 2, marginal; 3, good; 4, excellent. The images with image scale <2 were excluded. The boundaries of lumen and outer wall of each axial slice were delineated and the plaque components of calcification (CA), IPH, LRNC, loose matrix (LM), and FCR were identified using the published criteria (15). An example for identification of carotid artery and plaque components is shown in Figure 1. In addition, the T1w images were further resampled and registered with ToF images using 3D slicer, a medical image computing platform (16), in which the registration was performed using the Elastix medical image registration toolbox (17) available in 3D slicer. Vulnerable plaque was defined as lesions with presence of IPH, FCR, or larger LRNC with an area ratio of more than 40% to the arterial wall.
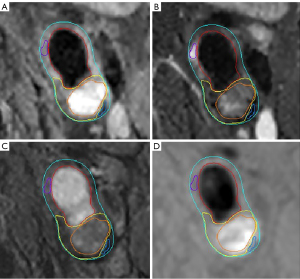
The outer wall boundaries on ToF magnetic resonance angiography (MRA) images were magnified 1.5 times which will be considered as the region of interest (ROI) for quantification of carotid artery PVAT. According to the fat suppression of T1w imaging and the difference in signal intensity between normal vessel wall and fat tissues, a k-means clustering algorithm was used to automatically segment the fat mask on the registered T1w images. The optimal number of clusters was determined as 4 using the elbow method. The first group represented background and vessels, the second group represented fat, the third group represented muscle, and the fourth group represented lymph nodes and other high-intensity tissues, respectively. The PVAT of the carotid artery was identified as the component of the second group within the ROI after clustering. An example of carotid artery PVAT segmentation of ToF images is shown in Figure 2. In addition, 2 circular regions with a radius of 1 mm were generated in the background region at the same position in each slice. The center of the 2 circular regions was automatically placed in the region of 5 pixels away from the edge of the image. Another circular region with a radius of 1 mm was also semi-automatically generated in the sternocleidomastoid muscle region on the same side of the index carotid artery. The signal-to-noise ratio (SNR) and contrast-to-noise ratio (CNR) of carotid artery PVAT at each axial slice were calculated, where SNR was the ratio of the signal intensity of carotid artery PVAT to the standard deviation (SD) of signal intensity of the background noise, and CNR was the ratio of the signal intensity difference of carotid artery PVAT and sternocleidomastoid muscle to the SD of signal intensity of background noise. The automatic analysis and quantification of carotid artery PVAT were conducted using MATLAB R2022a software (MathWorks, Natick, MA, USA).
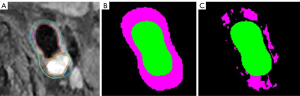
Reproducibility study
A total of 20 cases were randomly selected for reproducibility study. The PVAT of all axial slices of the 20 cases was segmented twice with a time interval of 3 months. The agreement in measuring SNR and CNR of PVAT between 2 times of segmentation was determined.
Statistical analysis
Continuous variables were described as mean ± SD, whereas categorical variables were presented as absolute counts and percentages. The SNR and CNR of carotid artery PVAT were compared between axial slices with and without carotid plaque components using Mann-Whitney U test. To explore the associations between SNR and CNR of carotid artery PVAT and the presence of carotid plaque components, the generalized linear mixed model (GLMM) with random effects was conducted to account for clustering of slices from the same patient. All GLMM models with fixed intercept, random intercept, and logit link function were adjusted for age, sex, body mass index (BMI), history of smoking, diabetes, hypertension, hyperlipidemia, and coronary heart disease. The value of P calculated by GLMM models was corrected by false discovery rate (FDR) correction for multiple comparisons. In addition, the intraclass correlation coefficient (ICC) was calculated for analyzing the agreement of measurements of SNR and CNR between the first and the second segmentation of PVAT with an identical method. Excellent agreement was determined when ICC >0.75. A 2-tailed value of P<0.05 was considered statistically significant. All statistical analyses were performed utilizing SPSS 26.0 (IBM Corp., Armonk, NY, USA).
Results
Clinical characteristics of study population
A total of 122 patients were recruited from April 2018 to December 2020 at Department of Neurosurgery of Peking University Third Hospital, of whom 18 were excluded because of the following reasons (Figure 3): (I) acute brain infract (n=2); (II) severe intracranial stenosis (n=2); (III) intracranial artery aneurysm (n=1); (IV) poor image quality (n=10); (V) misregistration of different imaging sequences due to motion (n=3). Of the remaining 104 patients, the mean age was 64.9±7.0 years, the mean BMI was 25.9±11.1 kg/m2, 86 (82.7%) were males, 61 (58.7%) had history of smoking, 72 (69.2%) had hypertension, 46 (44.2%) had hyperlipidemia, 37 (35.6%) had diabetes, and 21 (20.2%) had coronary heart disease. The clinical characteristics of the study population are summarized in Table 2.
Table 2
Clinical characteristics | Mean ± SD or n (%) |
---|---|
Age, years | 64.9±7.0 |
Male | 86 (82.7) |
BMI, kg/m2 | 25.9±11.1 |
Smoke | 61 (58.7) |
Diabetes | 37 (35.6) |
Hypertension | 72 (69.2) |
Hyperlipidemia | 46 (44.2) |
Coronary heart disease | 21 (20.2) |
SD, standard deviation; BMI, body mass index.
Comparison of SNR and CNR of PVAT between slices with and without plaque components
Of 1,594 slices of the index carotid artery of 104 patients, 365 (22.9%) had CA, 236 (14.8%) had IPH, 194 (12.2%) had FCR, 755 (47.4%) had LRNC, 233 (14.6%) had large LRNC, 143 (9.0%) had LM, and 479 (30.1%) had vulnerable plaque, respectively. Table 3 summarizes the comparison results of SNR and CNR of PVAT between slices with and without plaque components. Carotid artery slices with IPH (30.93±14.56 vs. 27.34±10.02; P<0.001), FCR (30.35±13.82 vs. 27.53±10.37; P=0.006), and vulnerable plaque (29.15±12.52 vs. 27.32±10.05; P=0.016) had significantly higher value of SNR of PVAT compared to those without, whereas such differences were not found between slices with and without CA and LRNC (all P>0.05). However, carotid artery slices with CA (−1.62±5.53 vs. 0.31±6.88; P<0.001), LRNC (−0.99±6.21 vs. 0.63±6.92; P<0.001), LM (−2.20±5.36 vs. 0.07±6.72; P<0.001) and larger LRNC (−1.00±5.25 vs. 0.01±6.85; P=0.006) had significantly lower value of CNR of PVAT compared to those without, whereas such differences cannot be found between slices with and without IPH and FCR (both P>0.05).
Table 3
Presence of plaque component | SNR of PVAT | CNR of PVAT | |||
---|---|---|---|---|---|
Mean ± SD | P value | Mean ± SD | P value | ||
CA | 0.386 | <0.001 | |||
− | 28.09±11.27 | 0.31±6.88 | |||
+ | 27.15±9.41 | −1.62±5.53 | |||
IPH | <0.001 | 0.241 | |||
− | 27.34±10.02 | −0.12±6.42 | |||
+ | 30.93±14.56 | −0.21±7.83 | |||
FCR | 0.006 | 0.646 | |||
− | 27.53±10.37 | −0.03±6.44 | |||
+ | 30.35±13.82 | −0.86±7.96 | |||
LRNC | 0.231 | <0.001 | |||
− | 27.44±10.35 | 0.63±6.92 | |||
+ | 28.35±11.42 | −0.99±6.21 | |||
LM | 0.589 | <0.001 | |||
− | 27.84±10.85 | 0.07±6.72 | |||
+ | 28.16±11.20 | −2.20±5.36 | |||
Large LRNC | 0.865 | 0.006 | |||
− | 27.91±11.06 | 0.01±6.85 | |||
+ | 27.64±9.81 | −1.00±5.25 | |||
Vulnerable plaque | 0.016 | 0.484 | |||
− | 27.32±10.05 | 0.04±6.67 | |||
+ | 29.15±12.52 | −0.54±6.56 |
“+” and “−” represent the slices with and without the corresponding plaque component, respectively. SNR, signal-to-noise ratio; CNR, contrast-to-noise ratio; PVAT, perivascular adipose tissue; SD, standard deviation; CA, calcification; IPH, intraplaque hemorrhage; FCR, fibrous cap rupture; LRNC, lipid-rich necrotic core; LM, loose matrix.
Correlation between PVAT and carotid plaque components
The GLMM models were established to explore the association between SNR or CNR of PVAT and carotid plaque components respectively (Table 4). After adjusting for clinical confounding factors of age, sex, BMI, history of smoking, diabetes, hypertension, hyperlipidemia and coronary heart disease, the SNR of carotid artery PVAT was significantly associated with the presence of IPH [odds ratio (OR) =0.627, 95% confidence interval (CI): 0.465–0.847, Puncorr=0.002, PFDR=0.016], and vulnerable plaque (OR =0.762, 95% CI: 0.629–0.924, Puncorr=0.006, PFDR=0.020). No significant association was found between SNR of carotid artery PVAT and presence of carotid plaque CA, FCR, LM, LRNC, and large LRNC (all P>0.05). The CNR of carotid artery PVAT was found to be significantly associated with the presence of CA (OR =1.409, 95% CI: 1.153–1.722, Puncorr=0.001, PFDR=0.006) and LRNC (OR =1.255, 95% CI: 1.075–1.465, Puncorr=0.004, PFDR=0.014), but not with other plaque components (all P>0.05), after adjusting for the above confounding factors.
Table 4
Presence of plaque feature | Predictor (SNR of PVAT) | Predictor (CNR of PVAT) | |||||||
---|---|---|---|---|---|---|---|---|---|
OR | 95% CI | Puncorr | PFDR | OR | 95% CI | Puncorr | PFDR | ||
CA | 1.114 | 0.901–1.378 | 0.320 | 0.373 | 1.409 | 1.153–1.722 | 0.001 | 0.006 | |
IPH | 0.627 | 0.465–0.847 | 0.002 | 0.016 | 0.845 | 0.655–1.090 | 0.194 | 0.340 | |
FCR | 0.791 | 0.606–1.033 | 0.085 | 0.148 | 0.936 | 0.735–1.193 | 0.595 | 0.694 | |
LRNC | 0.842 | 0.714–0.992 | 0.040 | 0.094 | 1.255 | 1.075–1.465 | 0.004 | 0.014 | |
LM | 0.793 | 0.569–1.105 | 0.170 | 0.238 | 1.120 | 0.810–1.548 | 0.492 | 0.689 | |
Large LRNC | 0.900 | 0.707–1.145 | 0.391 | 0.391 | 1.207 | 0.960–1.519 | 0.108 | 0.252 | |
Vulnerable plaque | 0.762 | 0.629–0.924 | 0.006 | 0.020 | 1.034 | 0.868–1.231 | 0.710 | 0.710 |
Adjustment for: age, sex, BMI, history of smoking, diabetes, hypertension, hyperlipidemia and coronary heart disease; the increment of SNR and CNR of carotid artery PVAT is 1 standard deviation. SNR, signal-to-noise ratio; CNR, contrast-to-noise ratio; PVAT, perivascular adipose tissue; OR, odds ratio; CI, confidence interval; Puncorr, uncorrected P value; PFDR, P value corrected by FDR; FDR, false discovery rate; CA, calcification; IPH, intraplaque hemorrhage; FCR, fibrous cap rupture; LRNC, lipid-rich necrotic core; LM, loose matrix; BMI, body mass index.
Reproducibility
Of the 20 cases selected for reproducibility study, 304 axial slices were included for analysis. There was excellent agreement in CNR (ICC =0.998, 95% CI: 0.998–0.999, P<0.001) and SNR (ICC =0.999, 95% CI: 0.999–1.000, P<0.001) between first and second time of segmentation of PVAT.
Discussion
This study investigated the relationship between carotid artery PVAT measured by ToF MRA and carotid vulnerable plaque features determined by multi-contrast MR vessel wall imaging. We found that carotid artery slices with vulnerable plaque features, particularly IPH and FCR, had significantly higher SNR of PVAT on ToF MRA compared to those without. The GLMM analysis showed that the SNR of PVAT on ToF MRA was independently associated with vulnerable plaque features in carotid arteries. Our findings suggest that the SNR of carotid artery PVAT measured by ToF MRA might be an effective indicator for carotid vulnerable plaques.
In the present study, the SNR of carotid artery PVAT on ToF MRA was found to be independently associated with carotid vulnerable plaque features. Previous studies have reported that density of carotid PVAT on CTA was associated with carotid vulnerable plaque. Saba et al. (11) found that there was a positive association between PFD on CTA and contrast plaque enhancement (r=0.658, P=0.001). Zhang et al. (12) reported that the density of carotid PVAT on CTA was strongly associated with carotid IPH (OR =1.96, 95% CI: 1.41–2.73, P<0.001). Therefore, both density on CTA and SNR on MRA of PVAT may represent the inflammatory status of fat. Investigators demonstrated that the IPH, as the result of rupture of immature neovessels, is associated with increased influx of inflammatory mediators within the plaques (18-20). Pathophysiologically, most of the neovessels in atherosclerotic plaques originate from the adventitial vasa vasorum (21,22). Recent studies have demonstrated that the inflammation of PVAT and adventitia will precede the formation of endothelial dysfunction and atherosclerotic plaques (23,24), and is closely associated with the sprouting of vasa vasorum (25,26). These findings suggest that the inflammation of PVAT may play an important role in the formation of IPH. In pathological conditions, the pro-inflammatory adipokines secreted by PVAT can spread directly to the vessel wall through paracrine and vascular secretion signaling pathways (8), leading to the increase of oxidative stress and inflammation in the adventitial layer (27), thus promoting the secretion of vascular endothelial growth factor (VEGF) (28). Then, the vasa vasorum grows into the lesion and mediates IPH (22). VEGF produced by dysfunctional PVAT may also promote the proliferation of vascular smooth muscle cells (VSMCs) (8,29,30). VSMCs are key to the formation and rupture of fiber caps. After phenotypic transformation, VSMCs can generate extracellular matrix to form fiber caps (31). As the fibroatheroma develops, VSMCs are continuously apoptotic or necrotic, leading to thinning and eventual rupture of fiber caps (31). In other ways, inflammatory mediators and oxidation products produced by local vascular lesions can also directly lead to changes in PVAT phenotype (32). Therefore, the inflammation of PVAT may increase the risk of developing IPH and FCR and the measurements of the density or signal intensity of PVAT might be effective indicators for vulnerable atherosclerotic plaques.
Our results also suggested that the CNR of PVAT on ToF MRA might not be a potential indicator for atherosclerotic plaque vulnerability. We hypothesized that the muscle tissue may also variate along with the inflammation of PVAT and arteriosclerotic plaques. Recent studies have shown that cholesterol crystals (CCs) are strongly associated with atherosclerosis and play a key role in plaque rupture (33-35). A variety of plaque cells, such as macrophages and smooth muscle cells, are active in producing CCs (33). The necrotic core expands during cholesterol crystallization, leading to rupture of the fibrous cap (34,35). As a result of plaque rupture, CCs are released into the circulation and embolize various tissues, ultimately causing local and systemic inflammation (35,36). Particularly, it has been established that embolization of CCs caused by plaque rupture can cause localized or generalized muscle inflammation and injury (37,38). Thus, rupture of atherosclerotic plaques can directly induce local muscle inflammation, which is consistent with our hypothesis. Our findings indicate that using muscle signal intensity as a reference may counteract to some extent the inflammatory changes in PVAT associated with atherosclerosis.
The present study has several limitations. First, since there is no unified definition of PVAT, the selection of the region for measuring carotid artery PVAT may not be optimal in this study. Second, the accuracy of carotid artery PVAT segmentation might be affected by the fat suppression effect of T1w imaging. Third, although ToF MRA has no fat suppression, its saturation effect has a potential impact on fat signal. In the future, quantitative fat imaging techniques, such as Dixon (39), can be used for better determining the inflammatory status of PVAT. Finally, because all included patients had moderate-to-severe carotid atherosclerotic stenosis, the data were inevitably biased to some extent. Therefore, a larger cohort with broader range of stenosis should be included in future studies.
Conclusions
The SNR of carotid artery PVAT measured by ToF MRA is independently associated with vulnerable atherosclerotic plaque features, suggesting that the signal intensity of PVAT might be an effective indicator for vulnerable plaque.
Acknowledgments
Funding: This work was supported by
Footnote
Reporting Checklist: The authors have completed the STROBE reporting checklist. Available at https://qims.amegroups.com/article/view/10.21037/qims-23-280/rc
Conflicts of Interest: All authors have completed the ICMJE uniform disclosure form (available at https://qims.amegroups.com/article/view/10.21037/qims-23-280/coif). The authors have no conflicts of interest to declare.
Ethical Statement: The authors are accountable for all aspects of the work in ensuring that questions related to the accuracy or integrity of any part of the work are appropriately investigated and resolved. The study was conducted in accordance with the Declaration of Helsinki (as revised in 2013). The study was approved by the Medical Ethics Committee of Peking University Third Hospital, and all participants provided written informed consent.
Open Access Statement: This is an Open Access article distributed in accordance with the Creative Commons Attribution-NonCommercial-NoDerivs 4.0 International License (CC BY-NC-ND 4.0), which permits the non-commercial replication and distribution of the article with the strict proviso that no changes or edits are made and the original work is properly cited (including links to both the formal publication through the relevant DOI and the license). See: https://creativecommons.org/licenses/by-nc-nd/4.0/.
References
- Global, regional, and national burden of stroke and its risk factors, 1990-2019: a systematic analysis for the Global Burden of Disease Study 2019. Lancet Neurol 2021;20:795-820. [Crossref] [PubMed]
- Zhang D, Wang M, Wu L, Zhao Y, Wang S, Yin X, Wu X. Assessing the characteristics and diagnostic value of plaques for patients with acute stroke using high-resolution magnetic resonance imaging. Quant Imaging Med Surg 2022;12:1529-38. [Crossref] [PubMed]
- Hoyer UCI, Lennartz S, Abdullayev N, Fichter F, Jünger ST, Goertz L, Laukamp KR, Gertz RJ, Grunz JP, Hohmann C, Maintz D, Persigehl T, Kabbasch C, Borggrefe J, Weiss K, Pennig L. Imaging of the extracranial internal carotid artery in acute ischemic stroke: assessment of stenosis, plaques, and image quality using relaxation-enhanced angiography without contrast and triggering (REACT). Quant Imaging Med Surg 2022;12:3640-54. [Crossref] [PubMed]
- Howard DP, van Lammeren GW, Redgrave JN, Moll FL, de Vries JP, de Kleijn DP, de Borst GJ, Pasterkamp G, Rothwell PM. Histological features of carotid plaque in patients with ocular ischemia versus cerebral events. Stroke 2013;44:734-9. [Crossref] [PubMed]
- Ross R. Atherosclerosis--an inflammatory disease. N Engl J Med 1999;340:115-26. [Crossref] [PubMed]
- Ridker PM, Everett BM, Thuren T, MacFadyen JG, Chang WH, Ballantyne C, et al. Antiinflammatory Therapy with Canakinumab for Atherosclerotic Disease. N Engl J Med 2017;377:1119-31. [Crossref] [PubMed]
- Ridker PM, Libby P, MacFadyen JG, Thuren T, Ballantyne C, Fonseca F, Koenig W, Shimokawa H, Everett BM, Glynn RJ. Modulation of the interleukin-6 signalling pathway and incidence rates of atherosclerotic events and all-cause mortality: analyses from the Canakinumab Anti-Inflammatory Thrombosis Outcomes Study (CANTOS). Eur Heart J 2018;39:3499-507. [Crossref] [PubMed]
- Nosalski R, Guzik TJ. Perivascular adipose tissue inflammation in vascular disease. Br J Pharmacol 2017;174:3496-513. [Crossref] [PubMed]
- Tanaka K, Sata M. Roles of Perivascular Adipose Tissue in the Pathogenesis of Atherosclerosis. Front Physiol 2018;9:3. [Crossref] [PubMed]
- Antonopoulos AS, Sanna F, Sabharwal N, Thomas S, Oikonomou EK, Herdman L, et al. Detecting human coronary inflammation by imaging perivascular fat. Sci Transl Med 2017;9:eaal2658. [Crossref] [PubMed]
- Saba L, Zucca S, Gupta A, Micheletti G, Suri JS, Balestrieri A, Porcu M, Crivelli P, Lanzino G, Qi Y, Nardi V, Faa G, Montisci R. Perivascular Fat Density and Contrast Plaque Enhancement: Does a Correlation Exist? AJNR Am J Neuroradiol 2020;41:1460-5. [Crossref] [PubMed]
- Zhang S, Gu H, Yu X, Kang B, Yuan X, Wang X. Association Between Carotid Artery Perivascular Fat Density and Intraplaque Hemorrhage. Front Cardiovasc Med 2021;8:735794. [Crossref] [PubMed]
- Baradaran H, Myneni PK, Patel P, Askin G, Gialdini G, Al-Dasuqi K, Kamel H, Gupta A. Association Between Carotid Artery Perivascular Fat Density and Cerebrovascular Ischemic Events. J Am Heart Assoc 2018;7:e010383. [Crossref] [PubMed]
- Zhang S, Yu X, Gu H, Kang B, Guo N, Wang X. Identification of high-risk carotid plaque by using carotid perivascular fat density on computed tomography angiography. Eur J Radiol 2022;150:110269. [Crossref] [PubMed]
- Saam T, Ferguson MS, Yarnykh VL, Takaya N, Xu D, Polissar NL, Hatsukami TS, Yuan C. Quantitative evaluation of carotid plaque composition by in vivo MRI. Arterioscler Thromb Vasc Biol 2005;25:234-9. [Crossref] [PubMed]
- Fedorov A, Beichel R, Kalpathy-Cramer J, Finet J, Fillion-Robin JC, Pujol S, Bauer C, Jennings D, Fennessy F, Sonka M, Buatti J, Aylward S, Miller JV, Pieper S, Kikinis R. 3D Slicer as an image computing platform for the Quantitative Imaging Network. Magn Reson Imaging 2012;30:1323-41. [Crossref] [PubMed]
- Klein S, Staring M, Murphy K, Viergever MA, Pluim JP. elastix: a toolbox for intensity-based medical image registration. IEEE Trans Med Imaging 2010;29:196-205. [Crossref] [PubMed]
- de Vries MR, Quax PH. Plaque angiogenesis and its relation to inflammation and atherosclerotic plaque destabilization. Curr Opin Lipidol 2016;27:499-506. [Crossref] [PubMed]
- Wu MY, Li CJ, Hou MF, Chu PY. New Insights into the Role of Inflammation in the Pathogenesis of Atherosclerosis. Int J Mol Sci 2017;18:2034. [Crossref] [PubMed]
- Brinjikji W, Huston J 3rd, Rabinstein AA, Kim GM, Lerman A, Lanzino G. Contemporary carotid imaging: from degree of stenosis to plaque vulnerability. J Neurosurg 2016;124:27-42. [Crossref] [PubMed]
- Virmani R, Kolodgie FD, Burke AP, Finn AV, Gold HK, Tulenko TN, Wrenn SP, Narula J. Atherosclerotic plaque progression and vulnerability to rupture: angiogenesis as a source of intraplaque hemorrhage. Arterioscler Thromb Vasc Biol 2005;25:2054-61. [Crossref] [PubMed]
- Xu J, Lu X, Shi GP. Vasa vasorum in atherosclerosis and clinical significance. Int J Mol Sci 2015;16:11574-608. [Crossref] [PubMed]
- Herrmann J, Lerman LO, Rodriguez-Porcel M, Holmes DR Jr, Richardson DM, Ritman EL, Lerman A. Coronary vasa vasorum neovascularization precedes epicardial endothelial dysfunction in experimental hypercholesterolemia. Cardiovasc Res 2001;51:762-6. [Crossref] [PubMed]
- Skiba DS, Nosalski R, Mikolajczyk TP, Siedlinski M, Rios FJ, Montezano AC, Jawien J, Olszanecki R, Korbut R, Czesnikiewicz-Guzik M, Touyz RM, Guzik TJ. Anti-atherosclerotic effect of the angiotensin 1-7 mimetic AVE0991 is mediated by inhibition of perivascular and plaque inflammation in early atherosclerosis. Br J Pharmacol 2017;174:4055-69. [Crossref] [PubMed]
- van Hinsbergh VW, Eringa EC, Daemen MJ. Neovascularization of the atherosclerotic plaque: interplay between atherosclerotic lesion, adventitia-derived microvessels and perivascular fat. Curr Opin Lipidol 2015;26:405-11. [Crossref] [PubMed]
- Manka D, Chatterjee TK, Stoll LL, Basford JE, Konaniah ES, Srinivasan R, Bogdanov VY, Tang Y, Blomkalns AL, Hui DY, Weintraub NL. Transplanted perivascular adipose tissue accelerates injury-induced neointimal hyperplasia: role of monocyte chemoattractant protein-1. Arterioscler Thromb Vasc Biol 2014;34:1723-30. [Crossref] [PubMed]
- Queiroz M, Sena CM. Perivascular adipose tissue in age-related vascular disease. Ageing Res Rev 2020;59:101040. [Crossref] [PubMed]
- Chistiakov DA, Orekhov AN, Bobryshev YV. Contribution of neovascularization and intraplaque haemorrhage to atherosclerotic plaque progression and instability. Acta Physiol (Oxf) 2015;213:539-53. [Crossref] [PubMed]
- Ahmadieh S, Kim HW, Weintraub NL. Potential role of perivascular adipose tissue in modulating atherosclerosis. Clin Sci (Lond) 2020;134:3-13. [Crossref] [PubMed]
- Schlich R, Willems M, Greulich S, Ruppe F, Knoefel WT, Ouwens DM, Maxhera B, Lichtenberg A, Eckel J, Sell H. VEGF in the crosstalk between human adipocytes and smooth muscle cells: depot-specific release from visceral and perivascular adipose tissue. Mediators Inflamm 2013;2013:982458. [Crossref] [PubMed]
- Basatemur GL, Jørgensen HF, Clarke MCH, Bennett MR, Mallat Z. Vascular smooth muscle cells in atherosclerosis. Nat Rev Cardiol 2019;16:727-44. [Crossref] [PubMed]
- Mancio J, Oikonomou EK, Antoniades C. Perivascular adipose tissue and coronary atherosclerosis. Heart 2018;104:1654-62. [Crossref] [PubMed]
- Baumer Y, Mehta NN, Dey AK, Powell-Wiley TM, Boisvert WA. Cholesterol crystals and atherosclerosis. Eur Heart J 2020;41:2236-9. [Crossref] [PubMed]
- Janoudi A, Shamoun FE, Kalavakunta JK, Abela GS. Cholesterol crystal induced arterial inflammation and destabilization of atherosclerotic plaque. Eur Heart J 2016;37:1959-67. [Crossref] [PubMed]
- Ghanem F, Vodnala D, K, Kalavakunta J, Durga S, Thormeier N, Subramaniyam P, Abela S, S, Abela G. Cholesterol crystal embolization following plaque rupture: a systemic disease with unusual features. J Biomed Res 2017;31:82-94. [Crossref] [PubMed]
- Abela GS. Cholesterol crystals piercing the arterial plaque and intima trigger local and systemic inflammation. J Clin Lipidol 2010;4:156-64. [Crossref] [PubMed]
- Pervaiz MH, Durga S, Janoudi A, Berger K, Abela GS. PET/CTA detection of muscle inflammation related to cholesterol crystal emboli without arterial obstruction. J Nucl Cardiol 2018;25:433-40. [Crossref] [PubMed]
- Haygood TA, Fessel WJ, Strange DA. Atheromatous microembolism simulating polymyositis. JAMA 1968;203:423-5.
- Ma J. Dixon techniques for water and fat imaging. J Magn Reson Imaging 2008;28:543-58. [Crossref] [PubMed]