Relationships between the coronary fat attenuation index for patients with heart-related disease measured automatically on coronary computed tomography angiography and coronary adverse events and degree of coronary stenosis
Introduction
Coronary atherosclerotic heart disease (CAHD) has serious adverse effects on human health. Previous research has shown that monitoring and improving the risk factors for coronary heart disease can significantly reduce the mortality of patients and improve their quality of life (1).
Pericoronary adipose coronary tissue (PACT) is a type of epicardial fat primarily found surrounding the adventitia of the 3 primary branches of the coronary artery (2). Research indicates that PACT and coronary atherosclerosis have an interactive relationship (3) and that PACT can influence the progression of CAHD (4). Efforts have been made to detect early changes in coronary atherosclerosis through the visualization of alterations in fat attenuation around blood vessels using coronary computed tomography angiography (CCTA) (5). The perivascular fat attenuation index (FAI) is a recently proposed quantitative measure. The FAI is computed by evaluating the weighted mean attenuation of adipose tissues encompassing voxels [ranging from –190 to –30 Hounsfield units (HU)] situated within radial proximity from the outer boundaries of blood vessels having equal diameters (6).
Postprocessing algorithms embedded in user-friendly software interfaces are being widely used. This automatic workflow ensures both reproducibility and efficiency in the measurement of the image-derived quantitative parameters, which constitute the prerequisites for conducting medical imaging research with accuracy and precision. In previous studies on the quantitative analysis of pericoronal fat, manual delineation was used; however, the manual method is inefficient, involves inevitable measurement errors, and has poor stability and repeatability. Consequently, previous studies have primarily focused on measuring the local volume and thickness of the PACT.
To address the limitations associated with the quantification of the FAI, Siemens Healthineers has developed a software solution that uses machine learning algorithms. These algorithms enable automated measurement of the FAI and have been integrated into a systematic 4-step segmentation process. The first step involves the characterization of the heart and its anatomy using a machine learning approach developed by Zheng et al. (7). This step uses marginal space learning and steerable features to accurately identify the heart and its structures. The second step focuses on isolating the heart, employing a model- and data-driven approach also developed by Zheng et al. (8). This approach incorporates information pertaining to specific regions of interest relating to blood vessels for isolating the heart, which is the key to subsequent analysis. The third step, which involves robust centerline extraction, is critical for ensuring accurate measurements and is implemented using advanced techniques. In the fourth step, the inner and outer vessel walls are segmented. The process of inner wall segmentation involves ray-casting followed by Markov random field graph analysis with convex priors (9). To achieve outer vessel wall segmentation, an adaptive self-learning edge model is used, combining 3D and 2D active contour models (10). These algorithms are currently regarded as the best-performing fully automatic approaches in their respective areas, as demonstrated in the Rotterdam Coronary Challenge leaderboard (8). Rigorously evaluated, they have proven capable of providing efficient and accurate performance. By integrating these algorithms into a streamlined workflow with user-interaction elements to avoid any flawed measurement, Siemens’ software provides an enhanced and efficient approach to FAI quantification in medical imaging (11). This study aims to measure FAI using AI automation and analyze the relationship between FAI and different degrees of stenosis CAHD and coronary adverse events, which has been rarely explored by previous researchers. We present this article in accordance with the STROBE reporting checklist (available at https://qims.amegroups.com/article/view/10.21037/qims-23-326/rc).
Methods
Population
This study retrospectively analyzed the data of 395 patients who had undergone CCTA examinations at West China Hospital, Sichuan University. The patients included in the study met the following inclusion criteria: (I) underwent CCTA between January 2012 and December 2012 for reasons including coronary heart disease, chest pain, myocardial infarction, revascularization therapy, and death from heart disease; and (II) follow-up until September 2020.
The patient exclusion criteria were as follows: (I) patients who died from all causes other than non-heart-related diseases; and (II) patients whose image postprocessing analysis with AI software was hindered due to issues with image quality, such as motion artifacts, breathing artifacts, or stent artifacts.
The study was conducted in accordance with the Declaration of Helsinki (as revised in 2013) and was approved by ethics board of West China Hospital, Sichuan University. Individual consent for this retrospective analysis was waived.
Basic clinical information of the patients
During the CCTA examination, age, sex, and smoking history were recorded. Within a week after the CCTA examination, all patients underwent fasting venous blood collection for the assessment of blood glucose, total cholesterol, low-density cholesterol, high-density cholesterol, and triglyceride levels. The presence or absence of coronary adverse events was recorded at this time and during follow-up. Adverse coronary events were defined as coronary heart disease readmission, revascularization, myocardial infarction, and patient death due to CAHD.
CCTA scan performance
A 128-slice dual-source CT (DSCT) scanner (Somatom Definition Flash; Siemens Healthineers, Erlangen, Germany) was used for image acquisition, and retrospective electrocardiographic gating was used for heart-related motion elimination.
The imaging parameters were as follows: slice thickness of 0.6–0.75 mm; for patients with a body mass index (BMI) value below 25, a tube voltage of 100 kV and a tube current of 220 mAs were used, whereas for patients with a BMI value of 25 or higher, a tube voltage of 120 kV and a tube current of 300 mAs were applied. In total, the majority of CCTAs were performed with a tube voltage of 120 kV (n=300, 76%), and the remaining 95 (24%) were at 100 kV. The scanning range started from 1 to 2 cm below the point where the trachea splits into 2, and it extended down to the surface of the diaphragm, covering all the coronary arteries. The contrast protocol involved administering 20 mL of saline for testing, followed by iodine contrast (1–1.5 mL/kg) (Ultravist, Bayer, Leverkusen, Germany), and finally, 20 mL of physiological brine. The injection flow rates used were 6, 5, and 4 mL/s. The scan was triggered when the attenuation reached 100 HU in the ascending aorta.
Image postprocessing involved transferring the images, including the best diastolic and best systolic images, to a workstation (Extend Brilliance Workstation, Philips Medical Systems, Best, The Netherlands) for processing and reconstruction. The reconstruction parameters used were as follows: a slice thickness of 0.75 mm, an interval of 0.5 mm, and a matrix size of 512×512.
Stenosis of the coronary arteries on CCTA
Three experienced radiologists with more than 5 years of work experience were chosen to analyze the CCTA images of the patients according to the Coronary Artery Disease Reporting and Data System criteria. The degrees of coronary artery stenosis were categorized into 6 levels: not visible (0%), minimal (1–24%), mild (25–49%), moderate (50–69%), severe (70–99%), and occluded (100%) (12).
Measurement of FAI around the coronary artery
The postprocessing analysis of coronary CCTA images was carried out using software equipped with an automatic workflow (Siemens research prototype, CT Coronary Plaque Analysis, software version 5.0.2, Siemens Healthineers). This software was used to obtain the FAI values for all patients.
The volume of interest for measuring pericoronary FAI was defined as a region extending radially from the vessel wall. This region had a length equal to the diameter of the vessel and covered 40-mm segments of the proximal left anterior descending artery (LAD), left circumflex artery (LCX), and right coronary artery (RCA). To avoid unwanted effects of the aortic root, the initial 10-mm segment of the RCA and the left main (LM) coronary artery, were excluded from the analysis (6,13). Fat tissue within these volumes of interests were defined as voxels value with attenuation between –190 and –30 HU (Figure 1). Finally, the FAI values, or the averaged CT attenuation of this fat tissue (Figure 2), were automatically calculated by the software. Simultaneously, 3 measurements of the same blood vessel were recorded, and the average value was taken to ensure the reliability and repeatability of the measured data.
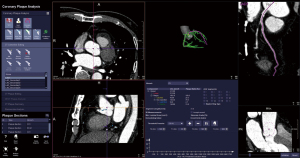
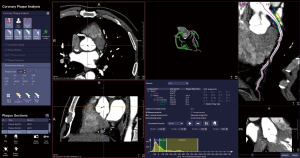
Statistical analysis
All statistical analyses and result plotting were performed in IBM SPSS software 20.0 (IBM Corp., Armonk, NY, USA). The data are presented as the average ± standard deviation (). Analysis of variance (ANOVA) was employed to assess the variations in the FAI among the groups in the LAD, LCX, and RCA, while the Bonferroni method was used for pairwise comparison correction among multiple groups. A 1-sided P value ≤0.05 indicated statistical significance. In the study, the clinical parameters affecting patients with coronary heart disease were adjusted by covariance analysis. This correction aimed to eliminate biases arising from individual factors, enabling a more precise assessment of the efficacy of AI functions in analyzing FAI, various degrees of coronary artery stenosis, and their association with adverse events related to CAHD in clinical settings.
Results
Population and baseline clinical characteristics
Based on the inclusion and exclusion criteria, a total of 338 patients were included in this study (as shown in Figure 3). These patients were categorized based on whether they experienced adverse coronary events during the follow-up period and whether the stenosis in any of their coronary arteries was moderately severe or higher. As a result, the patients were classified into 4 groups: those with less-than-moderate stenosis and no adverse events (Mb without adverse events), those with less-than-moderate stenosis but with adverse events (Mb with adverse events), those with moderate or above stenosis, and no adverse events (M without adverse events), and those with moderate or above stenosis and experiencing adverse events (M with adverse events). The baseline characteristics of these patients are presented in Table 1.
Table 1
Variable | Mb without adverse events (n=88) | Mb with adverse events (n=85) | M without adverse events (n=85) | M with adverse events (n=80) |
---|---|---|---|---|
Basic clinical data | ||||
Age (years) | 61.25±9.36 | 65.01±12.43 | 68.78±11.60 | 71.14±8.98 |
Sex (male:female) | 48:40 | 47:38 | 55:30 | 56:24 |
Smoking history (yes:no) | 32:56 | 31:54 | 35:50 | 39:41 |
Biochemical indicators | ||||
Fasting blood-glucose (mmol/L) | 5.80±1.74 | 5.66±1.41 | 7.03±3.36 | 6.54±2.20 |
Total cholesterol (mmol/L) | 4.63±1.07 | 4.13±1.05 | 4.21±1.13 | 4.52±1.49 |
Low-density (cholesterol mmol/L) | 2.63±0.83 | 2.42±0.87 | 2.51±0.93 | 2.70±1.38 |
High-density cholesterol (mmol/L) | 1.41±0.45 | 1.33±0.39 | 1.28±0.36 | 1.21±0.33 |
Triglycerides (mmol/L) | 1.76±1.66 | 1.39±0.88 | 1.42±0.78 | 1.72±1.10 |
Numerical variables are expressed as the average ± standard deviation. Mb, less-than-moderate stenosis; M, moderate-or-above stenosis.
The differences in sex or smoking status among the 4 groups were not significantly different (all P values >0.05). However, there were notable differences in age (P<0.001). The differences in blood glucose, total cholesterol, and high-density cholesterol among the 4 groups of patients were significant (all P values <0.05) (Table 1), but the differences in low-density cholesterol and triglycerides among the 4 groups were not significant (P>0.05).
Relationship between coronary FAI and adverse coronary events
Parts of vessels and branches were not evaluated among the 338 patients because of poor image quality (such as motion, breathing, and stent artifacts) and could not be recognized by AI software or undergo 3D reconstruction. Ultimately, 338 LADs, 337 LCXs, and 333 RCAs were evaluated. As shown in Table 2 and tested through ANOVA, statistically significant differences in FAI values were observed among the 4 groups for LAD, LCX, and RCA (P<0.001). Comparisons between any 2 groups showed that the differences in the FAI among the 4 groups for the LAD were significant (all P values <0.05). There were no significant differences between the Mb without adverse and M without adverse groups for the LCX (P>0.05). And for the remaining groups, FAI values exhibited statistically significant differences (P<0.05). There were no significant differences in FAI between the Mb without adverse events and M without adverse events groups or between the Mb with adverse events and M with adverse events groups in the RCA (P>0.05); however, the differences in FAI between the remaining groups were significant (P<0.05).
Table 2
Coronary artery branch | Mb without adverse events | Mb with adverse events | M without adverse events | M with adverse events | F | P | |||||||||||
---|---|---|---|---|---|---|---|---|---|---|---|---|---|---|---|---|---|
n | S | n | S | n | S | n | S | ||||||||||
LAD | 88 | −45.04 | 4.13 | 85 | −39.35 | 5.88 | 85 | −42.93 | 3.97 | 80 | −37.07 | 6.78 | 38.22 | <0.001 | |||
LCX | 88 | −36.51 | 4.11 | 84 | −33.75 | 5.36 | 85 | −36.40 | 5.57 | 80 | −31.14 | 6.62 | 18.04 | <0.001 | |||
RCA | 87 | −44.74 | 6.55 | 84 | −41.71 | 7.54 | 82 | −44.48 | 6.70 | 80 | −40.53 | 8.80 | 6.45 | <0.001 |
FAI, fat attenuation index; Mb, less-than-moderate stenosis; M, moderate-or-above stenosis; S, standard deviation; LAD, left anterior descending artery; LCX, left circumflex artery; RCA, right coronary artery.
The blood glucose, total cholesterol, and high-density cholesterol levels and age of these patients were used as covariates. After the influence of these factors’ effects were excluded through covariance analysis, significant differences in FAI values among the 4 groups persisted for LAD, LCX, and RCA (Table 3).
Table 3
Coronary artery branch | Mb without adverse events | Mb with adverse events | M without adverse events | M with adverse events | F | P | |||||||||||
---|---|---|---|---|---|---|---|---|---|---|---|---|---|---|---|---|---|
n | S | n | S | n | S | n | S | ||||||||||
LAD | 88 | −44.99 | 4.18 | 85 | −39.38 | 5.93 | 85 | −42.90 | 3.99 | 80 | −37.07 | 6.78 | 34.13 | <0.001 | |||
LCX | 88 | −36.44 | 4.12 | 84 | −33.76 | 5.42 | 85 | −36.46 | 5.58 | 80 | −31.14 | 6.62 | 18.31 | <0.001 | |||
RCA | 87 | −44.70 | 6.63 | 84 | −41.91 | 7.50 | 82 | −44.56 | 6.70 | 80 | −40.53 | 8.80 | 7.09 | <0.001 |
FAI, fat attenuation index; Mb, less-than-moderate stenosis; M, moderate-or-above stenosis; S, standard deviation; LAD, left anterior descending artery; LCX, left circumflex artery; RCA, right coronary artery.
Table 4 displays the distribution of FAIs among various groups and their corresponding results. The findings indicated a significantly greater occurrence of FAI <–40 in the group experiencing coronary adverse events compared to the group without such events.
Table 4
FAI | Mb without adverse events, (n=88) [%] | Mb with adverse events, (n=85) [%] | M without adverse events, (n=85) [%] | M with adverse events, (n=80) [%] | |||||||||||
---|---|---|---|---|---|---|---|---|---|---|---|---|---|---|---|
LAD | LCX | RCA | LAD | LCX | RCA | LAD | LCX | RCA | LAD | LCX | RCA | ||||
<−50 | 9 [10] | 0 [0] | 17 [20] | 2 [2] | 0 [0] | 14 [17] | 5 [6] | 2 [2] | 11 [13] | 0 [0] | 0 [0] | 15 [19] | |||
−40 to −50 | 71 [81] | 15 [17] | 52 [60] | 40 [47] | 10 [12] | 34 [40.5] | 54 [64] | 19 [23] | 53 [65] | 34 [42.5] | 7 [9] | 31 [39] | |||
−30 to −39 | 8 [9] | 67 [76] | 18 [20] | 35 [41] | 55 [65] | 34 [40.5] | 26 [30] | 57 [67] | 16 [20] | 36 [45] | 43 [54] | 23 [29] | |||
>−30 | 0 [0] | 6 [7] | 0 [0] | 8 [10] | 19 [23] | 2 [2] | 0 [0] | 7 [8] | 2 [2] | 10 [12.5] | 30 [37] | 11 [13] |
FAI, fat attenuation index; Mb, less-than-moderate stenosis; M, moderate-or-above stenosis; LAD, left anterior descending artery; LCX, left circumflex artery; RCA, right coronary artery.
In our other analysis of moderate-to-mild coronary artery stenosis, there were slight differences in data due to changes in follow-up times and included patients, but the final results nonetheless confirmed that the FAI of patients with coronary adverse events was significantly higher than that of patients without coronary adverse events (14).
Coronary FAI and coronary lumen stenosis
According to the degree of lumen stenosis, the patients were divided into groups according to the LAD, LCX, RCA, and the sum of the 3 vessels (TV).
There were significant differences in coronary FAI with different degrees of lumen stenosis of the TV, LAD, and LCX (P<0.05) (Table 5). In a comparison of any 2 groups, a notable distinction in coronary FAI was observed between the severe and moderate groups in TV (P<0.05). Nonetheless, for the remaining groups in TV, there were no statistically significant differences observed (P>0.05). Significant differences in coronary FAI were evident between the severe group and the not visible, minimal, and moderate groups in the LAD and LCX (P<0.05). However, no statistically significant differences were found among the other groups in the LAD and LCX (P>0.05). There were no significant differences in FAI among the 4 groups with different degrees of stenosis in the RCA (P>0.05).
Table 5
Coronary artery branch | Not visible | Minimal | Moderate | Severe | F | P | |||||||||||
---|---|---|---|---|---|---|---|---|---|---|---|---|---|---|---|---|---|
n | S | n | S | n | S | n | S | ||||||||||
TV | 562 | −39.64 | 7.38 | 156 | −39.49 | 8.23 | 144 | −40.65 | 5.92 | 146 | −38.02 | 8.28 | 3.12 | 0.025 | |||
LAD | 147 | −42.04 | 5.87 | 56 | −41.86 | 7.53 | 68 | −41.27 | 4.41 | 4.41 | −38.68 | 6.23 | 5.13 | 0.002 | |||
LCX | 218 | −34.86 | 5.59 | 52 | −34.06 | 6.82 | 32 | −36.06 | 4.94 | 35 | −31.69 | 6.05 | 3.89 | 0.009 | |||
RCA | 197 | −43.14 | 7.32 | 48 | −42.59 | 7.58 | 44 | −43.05 | 6.81 | 44 | −42.04 | 9.63 | 0.28 | 0.840 |
FAI, fat attenuation index; S, standard deviation; TV, sum of the 3 vessels; LAD, left anterior descending artery; LCX, left circumflex artery; RCA, right coronary artery.
The composition of the degrees of lumen stenosis in the LAD, LCX, and RCA is shown in Table 6.
- The χ2 test was used to compare the proportion of different degrees of stenosis in the lumens of 3 blood vessels, which revealed a statistically significant difference in the proportion of different degrees of stenosis in the lumens of the 3 blood vessels (χ2=39.80; P<0.001).
- After pairwise comparison through χ2 segmentation, it was found that there were statistically significant differences in the proportion of different degrees of stenosis between the LAD and LCX, as well as between the LAD and RCA (P<0.01). There was no statistically significant difference in the proportion of different degrees of stenosis between the LCX and RCA (P>0.01).
Table 6
Stenosis | LAD, n=338 (%) | LCX, n=337 (%) | RCA, n=333 (%) |
---|---|---|---|
Not visible | 147 (43.5) | 218 (64.7) | 197 (59.2) |
Minimal | 56 (16.6) | 52 (15.4) | 48 (14.4) |
Moderate | 68 (20.1) | 32 (9.5) | 44 (13.2) |
Severe | 67 (19.8) | 35 (10.4) | 44 (13.2) |
LAD, left anterior descending artery; LCX, left circumflex artery; RCA, right coronary artery.
Discussion
Pericardial fat and coronary FAI
Epicardial fat is a type of adipose tissue originating from the mesoderm and supplied by the coronary arteries (15,16). Pericardial adipose tissue is a form of epicardial fat located in close proximity to the myocardium, directly contacting the coronary arteries. PACT has a role in the occurrence, development, and destabilization of coronary atherosclerosis and coronary plaque (17). Through research, the concept of the interaction between the fat around the coronary arteries and the vessel wall has evolved. Coronary artery atherosclerosis and vascular damage may cause changes in fat around the coronary artery (13,18), and the fat around the coronary arteries may aggravate the process of coronary atherosclerosis (19).
The FAI is a recently proposed measurement index that consists of the average weighted attenuation of the adipose tissue in the region of interest after the CCTA images have been reconstructed in 3 dimensions (6,13). FAI potentially serves as an indicator of the phenotype and metabolic activity of PACT and offers supportive evidence for risk stratification in CAHD (20,21). Most previous studies on pericoronal fat used manual delineation, and so measurement errors were inevitable (2,4,22). Therefore, this study enrolled 338 patients who underwent CCTA and had follow-up results to evaluate the fat around the coronary arteries and its relationship with the status of CAHD. The FAI of coronary arteries was used as an indicator, and the FAI was acquired using intelligent algorithms embedded in a user-friendly interface for segmentation.
Coronary FAI and adverse coronary events
Our findings indicated that when patients were grouped according to whether they have coronary adverse events and the degree of stenosis, the pericoronal fat FAI values of patients among these 4 groups were significantly different; notably, the M with adverse events group had the highest FAI value while the Mb without adverse groups had the lowest FAI value. FAI values of the fat surrounding the 3 coronary arteries in the 2 groups with adverse events were also higher compared to those in the 2 groups without adverse events, and the FAI values of the 2 M groups were also higher than those of the 2 Mb groups.
Vascular inflammation is a factor that has been proven to have an important influence on coronary atherosclerosis (23-25). The initial description of this abnormal elevation in adipose tissue attenuation on CT images, attributed to edema, inflammatory, and/or vegetative infiltration, has been reported in abdominal pelvic CT (26). Vascular inflammation is a key factor in the development and progression of atherosclerosis (27). In recent years, PACT has been extensively researched due to its functions as a marker and promoter of local coronary inflammation (28,29). In previous studies, the density of PACT was associated with a variety of traditional risk factors for coronary heart disease, such as age, sex, BMI, hypertension, and diabetes (30,31). Lee et al. (32) confirmed that the PACT volume is a more reliable predictor than the Framingham risk score. However, due to the complexity of PACT measurement methods, this indicator has not been widely used in clinical practice.
Our research results showed that using AI to measure FAI in CCTA is an effective noninvasive examination method that can reflect vascular status, and the change of FAI value can reflect the severity of coronary vascular inflammation in patients to a certain extent. Compared to traditional coronary risk factors, FAI measurement may be a more objective and accurate monitoring method for reflecting the coronary artery status, which plays a valuable role in preventing the occurrence of CAHD and indicating the coronary artery health of patients.
Coronary FAI and coronary lumen stenosis
The degree of lumen stenosis plays a crucial role in the diagnosis and treatment decision-making process for CAHD and is closely associated with the occurrence of myocardial ischemia. Previous studies indicate that patients with CAHD and coronary stenosis ≥50% have increased pericoronal adipose tissue density (13,33). The overall PACT density is higher in patients with myocardial infarction than in patients with stable CAHD, and PACT density has been shown to be positively correlated with the occurrence of myocardial infarction (34). Gaibazz et al. (35) also found that coronary FAI was higher in a group of patients with coronary nonobstructive myocardial infarction than in a control group, with a sensitivity and specificity of 61.3% and 92.4%, respectively.
Although some researchers have previously reported that FAI is not related to the degree of coronary stenosis in patients (5,36), our results showed that in the LAD and LCX, the FAI was significantly higher in the moderate-or-above stenosis groups than in the other 2 groups. We speculate that this discrepancy may be due to 2 factors: (I) the exacerbation of stenosis in the patient's coronary lumen exacerbates inflammation in the patient’s coronary lumen, leading to an increase in FAI; (II) the extent of stenosis within the patient’s lumen leads to alterations in the corresponding coronary arteries, resulting in an elevation of FAI. This may also explain why the RCA has less lumen curvature and fewer branch vessels than do the LAD and LCX, so the degree of stenosis of the RCA lumen has less influence on its hemodynamics and thus has less influence on the FAI. This speculation needs to be confirmed by further research in a study with a larger sample size of patients and a combination of more examination results.
Study novelty
This study’s strengths include its substantial sample size and extended follow-up period. Another notable feature is the use of a combination of postprocessing algorithms to automatically quantify the FAI. The obtained measurement results reflect the entire coronary artery. The use of innovative software, designed with a user-friendly interface, demonstrates excellent repeatability, leading to more objective and time-efficient results, which should provide benefits in clinical application.
Limitations
Some limitations to our study should be acknowledged. First, it is important to note that this study was based on a retrospective analysis of patient images. During routine CCTA examinations, the tube voltage was adjusted according to the individual patient’s size. It is widely known that tube voltage can influence the measurement of FAI, introducing potential variation in the obtained values. In this study, the included images were predominantly acquired using a tube voltage of 120 kVp. A relevant study conducted by Ma et al. (37) compared the mean attenuation of FAI between 100 and 120 kVp and found no statistically significant difference. Based on this finding, it can be inferred that the variation in FAI measurements caused by different tube voltages could be disregarded in the context of this particular study. However, the generalizability of our study findings would have to be validated in CCTA performed at other kilovoltages. Second, resource limitations might have led to variations in patient classifications during follow-up, potentially introducing statistical bias. Third, the study being single-center in nature resulted in the recruitment of research participants being predominantly from the same geographical region.
Conclusions
As a noninvasive and convenient cardiovascular examination method, CCTA is currently the most widely used technique for the diagnosis, treatment, and follow-up of patients with CAHD. In this study, AI software was used to quantify pericoronal fat on CCTA images, which provided a more objective and comprehensive evaluation method with high repeatability. The FAI can reflect the state of coronary atherosclerosis to a certain extent and may be closely correlated with coronary adverse events. In clinical work, the changes in FAI and CCTA images may indicate pericoronary adverse events.
Acknowledgments
Funding: None.
Footnote
Reporting Checklist: The authors have completed the STROBE reporting checklist. Available at https://qims.amegroups.com/article/view/10.21037/qims-23-326/rc
Conflicts of Interest: All authors have completed the ICMJE uniform disclosure form (available at https://qims.amegroups.com/article/view/10.21037/qims-23-326/coif). XYC is an employee consultant of Siemens Healthineers. The other authors have no conflicts of interest to declare.
Ethical Statement: The authors are accountable for all aspects of the work in ensuring that questions related to the accuracy or integrity of any part of the work are appropriately investigated and resolved. The study was conducted in accordance with the Declaration of Helsinki (as revised in 2013) and was approved by ethics board of West China Hospital, Sichuan University. Individual consent for this retrospective analysis was waived.
Open Access Statement: This is an Open Access article distributed in accordance with the Creative Commons Attribution-NonCommercial-NoDerivs 4.0 International License (CC BY-NC-ND 4.0), which permits the non-commercial replication and distribution of the article with the strict proviso that no changes or edits are made and the original work is properly cited (including links to both the formal publication through the relevant DOI and the license). See: https://creativecommons.org/licenses/by-nc-nd/4.0/.
References
- Wu P, Gulati M, Kwok CS, Wong CW, Narain A, O'Brien S, Chew-Graham CA, Verma G, Kadam UT, Mamas MA. Preterm Delivery and Future Risk of Maternal Cardiovascular Disease: A Systematic Review and Meta-Analysis. J Am Heart Assoc 2018;7:e007809. [Crossref] [PubMed]
- Fitzgibbons TP, Czech MP. Epicardial and perivascular adipose tissues and their influence on cardiovascular disease: basic mechanisms and clinical associations. J Am Heart Assoc 2014;3:e000582. [Crossref] [PubMed]
- Antonopoulos AS, Margaritis M, Coutinho P, Shirodaria C, Psarros C, Herdman L, Sanna F, De Silva R, Petrou M, Sayeed R, Krasopoulos G, Lee R, Digby J, Reilly S, Bakogiannis C, Tousoulis D, Kessler B, Casadei B, Channon KM, Antoniades C. Adiponectin as a link between type 2 diabetes and vascular NADPH oxidase activity in the human arterial wall: the regulatory role of perivascular adipose tissue. Diabetes 2015;64:2207-19. [Crossref] [PubMed]
- Zhang M, Li ZP, Li WH, Li D, Liu LN, Feng XH, Gao W. Correlation between epicardial adipose tissue and coronary flow reserve in coronary heart disease patients with no chest pain. Beijing Da Xue Xue Bao Yi Xue Ban 2014;46:848-53.
- Oikonomou EK, Marwan M, Desai MY, Mancio J, Alashi A, Hutt Centeno E, et al. Non-invasive detection of coronary inflammation using computed tomography and prediction of residual cardiovascular risk (the CRISP CT study): a post-hoc analysis of prospective outcome data. Lancet 2018;392:929-39. [Crossref] [PubMed]
- Elnabawi YA, Oikonomou EK, Dey AK, Mancio J, Rodante JA, Aksentijevich M, Choi H, Keel A, Erb-Alvarez J, Teague HL, Joshi AA, Playford MP, Lockshin B, Choi AD, Gelfand JM, Chen MY, Bluemke DA, Shirodaria C, Antoniades C, Mehta NN. Association of Biologic Therapy With Coronary Inflammation in Patients With Psoriasis as Assessed by Perivascular Fat Attenuation Index. JAMA Cardiol 2019;4:885-91. [Crossref] [PubMed]
- Zheng Y, Barbu A, Georgescu B, Scheuering M, Comaniciu D. Four-chamber heart modeling and automatic segmentation for 3-D cardiac CT volumes using marginal space learning and steerable features. IEEE Trans Med Imaging 2008;27:1668-81. [Crossref] [PubMed]
- Zheng Y, Tek H, Funka-Lea G. Robust and accurate coronary artery centerline extraction in CTA by combining model-driven and data-driven approaches. Med Image Comput Comput Assist Interv 2013;16:74-81.
- Schaap M, Metz CT, van Walsum T, van der Giessen AG, Weustink AC, Mollet NR, et al. Standardized evaluation methodology and reference database for evaluating coronary artery centerline extraction algorithms. Med Image Anal 2009;13:701-14. [Crossref] [PubMed]
- Wels M, Lades F, Hopfgartner C, Schwemmer C, Sühling M. Intuitive and Accurate Patient-Specific Coronary Tree Modeling from Cardiac Computed-Tomography Angiography. In: Proceedings of the 3rd Interactive MIC Workshop, Athens, Greece: 2016;86-93.
- Denzinger F, Wels M, Hopfgartner C, Lu J, Schöbinger M, Maier A, Sühling M. Coronary Plaque Analysis for CT Angiography Clinical Research. In: Bildverarbeitung für die Medizin 2021. Informatik Aktuell; Palm C, Deserno TM, Handels H, Maier A, Maier-Hein K, Tolxdorff T, Editors. Vieweg, IL, USA; Wiesbaden, Germany: Springer; 2021.
- Cury RC, Abbara S, Achenbach S, Agatston A, Berman DS, Budoff MJ, Dill KE, Jacobs JE, Maroules CD, Rubin GD, Rybicki FJ, Schoepf UJ, Shaw LJ, Stillman AE, White CS, Woodard PK, Leipsic JA. CAD-RADS(TM) Coronary Artery Disease - Reporting and Data System. An expert consensus document of the Society of Cardiovascular Computed Tomography (SCCT), the American College of Radiology (ACR) and the North American Society for Cardiovascular Imaging (NASCI). Endorsed by the American College of Cardiology. J Cardiovasc Comput Tomogr 2016;10:269-81. [Crossref] [PubMed]
- Antonopoulos AS, Sanna F, Sabharwal N, Thomas S, Oikonomou EK, Herdman L, et al. Detecting human coronary inflammation by imaging perivascular fat. Sci Transl Med 2017;9:eaal2658. [Crossref] [PubMed]
- Zhang W, Li P, Chen X, He L, Zhang Q, Yu J. The Association of Coronary Fat Attenuation Index Quantified by Automated Software on Coronary Computed Tomography Angiography with Adverse Events in Patients with Less than Moderate Coronary Artery Stenosis. Diagnostics (Basel) 2023;13:2136. [Crossref] [PubMed]
- Dweck MR, Puntman V, Vesey AT, Fayad ZA, Nagel E. MR Imaging of Coronary Arteries and Plaques. JACC Cardiovasc Imaging 2016;9:306-16. [Crossref] [PubMed]
- Ueda Y, Shiga Y, Idemoto Y, Tashiro K, Motozato K, Koyoshi R, Kuwano T, Fujimi K, Ogawa M, Saku K, Miura SI. Association Between the Presence or Severity of Coronary Artery Disease and Pericardial Fat, Paracardial Fat, Epicardial Fat, Visceral Fat, and Subcutaneous Fat as Assessed by Multi-Detector Row Computed Tomography. Int Heart J 2018;59:695-704. [Crossref] [PubMed]
- Verhagen SN, Visseren FL. Perivascular adipose tissue as a cause of atherosclerosis. Atherosclerosis 2011;214:3-10. [Crossref] [PubMed]
- Takaoka M, Suzuki H, Shioda S, Sekikawa K, Saito Y, Nagai R, Sata M. Endovascular injury induces rapid phenotypic changes in perivascular adipose tissue. Arterioscler Thromb Vasc Biol 2010;30:1576-82. [Crossref] [PubMed]
- Morales-Portano JD, Peraza-Zaldivar JÁ, Suárez-Cuenca JA, Aceves-Millán R, Amezcua-Gómez L, Ixcamparij-Rosales CH, Trujillo-Cortés R, Robledo-Nolasco R, Mondragón-Terán P, Pérez-Cabeza de Vaca R, Hernández-Muñoz R, Melchor-López A, Vannan MA, Rubio-Guerra AF. Echocardiographic measurements of epicardial adipose tissue and comparative ability to predict adverse cardiovascular outcomes in patients with coronary artery disease. Int J Cardiovasc Imaging 2018;34:1429-37. [Crossref] [PubMed]
- Díaz-Rodríguez E, Agra RM, Fernández ÁL, Adrio B, García-Caballero T, González-Juanatey JR, Eiras S. Effects of dapagliflozin on human epicardial adipose tissue: modulation of insulin resistance, inflammatory chemokine production, and differentiation ability. Cardiovasc Res 2018;114:336-46. [Crossref] [PubMed]
- Dai X, Hou Y, Tang C, Lu Z, Shen C, Zhang L, Zhang J. Long-term prognostic value of the serial changes of CT-derived fractional flow reserve and perivascular fat attenuation index. Quant Imaging Med Surg 2022;12:752-65. [Crossref] [PubMed]
- Bhuiyan GR, Roy GC, Siddique MA, Rahman M, Ahmed K, Nahar F. Relationship between Echocardiographic Epicardial Adipose Tissue (EAT) Thickness and Angiographically Detected Coronary Artery Disease. Mymensingh Med J 2017;26:498-504.
- Frostegård J. Immunity, atherosclerosis and cardiovascular disease. BMC Med 2013;11:117. [Crossref] [PubMed]
- Kohchi K, Takebayashi S, Hiroki T, Nobuyoshi M. Significance of adventitial inflammation of the coronary artery in patients with unstable angina: results at autopsy. Circulation 1985;71:709-16. [Crossref] [PubMed]
- Hirata Y, Tabata M, Kurobe H, Motoki T, Akaike M, Nishio C, Higashida M, Mikasa H, Nakaya Y, Takanashi S, Igarashi T, Kitagawa T, Sata M. Coronary atherosclerosis is associated with macrophage polarization in epicardial adipose tissue. J Am Coll Cardiol 2011;58:248-55. [Crossref] [PubMed]
- Thornton E, Mendiratta-Lala M, Siewert B, Eisenberg RL. Patterns of fat stranding. AJR Am J Roentgenol 2011;197:W1-14. [Crossref] [PubMed]
- Raggi P, Genest J, Giles JT, Rayner KJ, Dwivedi G, Beanlands RS, Gupta M. Role of inflammation in the pathogenesis of atherosclerosis and therapeutic interventions. Atherosclerosis 2018;276:98-108. [Crossref] [PubMed]
- Franssens BT, Nathoe HM, Leiner T, van der Graaf Y, Visseren FL. Relation between cardiovascular disease risk factors and epicardial adipose tissue density on cardiac computed tomography in patients at high risk of cardiovascular events. Eur J Prev Cardiol 2017;24:660-70. [Crossref] [PubMed]
- Chen X, Dang Y, Hu H, Ma S, Ma Y, Wang K, Liu T, Lu X, Hou Y. Pericoronary adipose tissue attenuation assessed by dual-layer spectral detector computed tomography is a sensitive imaging marker of high-risk plaques. Quant Imaging Med Surg 2021;11:2093-103. [Crossref] [PubMed]
- Hell MM, Ding X, Rubeaux M, Slomka P, Gransar H, Terzopoulos D, Hayes S, Marwan M, Achenbach S, Berman DS, Dey D. Epicardial adipose tissue volume but not density is an independent predictor for myocardial ischemia. J Cardiovasc Comput Tomogr 2016;10:141-9. [Crossref] [PubMed]
- Milanese G, Silva M, Bruno L, Goldoni M, Benedetti G, Rossi E, Ferrari C, Grutta L, Maffei E, Toia P, Forte E, Bonadonna RC, Sverzellati N, Cademartiri F. Quantification of epicardial fat with cardiac CT angiography and association with cardiovascular risk factors in symptomatic patients: from the ALTER-BIO (Alternative Cardiovascular Bio-Imaging markers) registry. Diagn Interv Radiol 2019;25:35-41. [Crossref] [PubMed]
- Lee BC, Lee WJ, Lo SC, Hsu HC, Chien KL, Chang YC, Chen MF. The ratio of epicardial to body fat improves the prediction of coronary artery disease beyond calcium and Framingham risk scores. Int J Cardiovasc Imaging 2016;32:117-27. [Crossref] [PubMed]
- Konishi M, Sugiyama S, Sato Y, Oshima S, Sugamura K, Nozaki T, Ohba K, Matsubara J, Sumida H, Nagayoshi Y, Sakamoto K, Utsunomiya D, Awai K, Jinnouchi H, Matsuzawa Y, Yamashita Y, Asada Y, Kimura K, Umemura S, Ogawa H. Pericardial fat inflammation correlates with coronary artery disease. Atherosclerosis 2010;213:649-55. [Crossref] [PubMed]
- Mahabadi AA, Balcer B, Dykun I, Forsting M, Schlosser T, Heusch G, Rassaf T. Cardiac computed tomography-derived epicardial fat volume and attenuation independently distinguish patients with and without myocardial infarction. PLoS One 2017;12:e0183514. [Crossref] [PubMed]
- Gaibazzi N, Martini C, Botti A, Pinazzi A, Bottazzi B, Palumbo AA. Coronary Inflammation by Computed Tomography Pericoronary Fat Attenuation in MINOCA and Tako-Tsubo Syndrome. J Am Heart Assoc 2019;8:e013235. [Crossref] [PubMed]
- Oikonomou EK, West HW, Antoniades C. Cardiac Computed Tomography: Assessment of Coronary Inflammation and Other Plaque Features. Arterioscler Thromb Vasc Biol 2019;39:2207-19. [Crossref] [PubMed]
- Ma R, Ties D, van Assen M, Pelgrim GJ, Sidorenkov G, van Ooijen PMA, van der Harst P, van Dijk R, Vliegenthart R. Towards reference values of pericoronary adipose tissue attenuation: impact of coronary artery and tube voltage in coronary computed tomography angiography. Eur Radiol 2020;30:6838-46. [Crossref] [PubMed]