Comparison of artificial intelligence vs. junior dentists’ diagnostic performance based on caries and periapical infection detection on panoramic images
Introduction
Diagnosis is important and challenging in dentistry. Dental caries and infections are the most prevalent chronic dental diseases worldwide (1). Periapical tissue diseases are diffuse, liquefaction lesions that occur as an inflammatory response to tooth infection and necrotic pulp or non-microbial causes. Pulpal and periodontal infections can affect the alveolar bone, and they can spread to distant structures from the oral cavity via bone marrow, cortical bone and periosteum (2). Therefore, many techniques have been developed for the diagnosis of dental caries and infections. Visual and intraoral examination and radiographs (panoramic, bitewing, periapical) are usually used to diagnose caries and infections (3). In addition, transillumination is one of the oldest diagnosis methods offering moderate validity in the diagnosis of carious lesions in dentine (4). Based on this principle, various diagnostic technologies have been developed (Foti, Difoti, Nilt) (4).
As technology has advanced, AI has taken on important roles in health, providing convenience and superior success in several areas, with evident potential in diagnosis. AI continues to be researched and developed to facilitate the diagnosis of dental caries and infections, which are frequently encountered (5).
The software of AI aimed towards obtaining the best results in dentistry, patient examination, and treatments. It has been used to make diagnosis more accurate and efficient. The diagnostic speed of AI has a significant advantage when compared to dentists, reducing the time spent in the dental chair and the associated stress levels, of particular benefit to pediatric and adult patients with anxiety problems (6). To offer the best diagnosis, the most appropriate treatment plan and to predict the prognosis, dentists must draw on all their academic knowledge; however, in some cases, their knowledge may be insufficient (7).
There is no general-purpose AI, and no general solution method to serve as a comprehensive expert algorithm. Different solution methods are chosen for different tasks. Many tasks address mainly auditory and textual data that concern visual and natural language. Among the many AI technologies, classification is often handled by artificial neural networks (ANNs), and of the subtype called convolutional neural networks (CNNs).
ANNs start by assigning random weights to the connections between neurons, and, through the learning process, re-sets those weights so that the ANN mechanism works correctly. Each layer of an image recognition ANN conducts an abstraction process lines and corners are distinguished in the first layer, curvatures can be detected in later layers. Adding convolution to the network shifts the attention to low-level mechanisms such as curves and edges in an image (5).
As the network proceeds to learn, redundant data can be deleted, and, finally, the information is condensed into a one-dimensional vector by a fully interconnected layer. Once trained, the ANN is given an input image, and produces an output that indicates the presence of certain objects (8). CNN has already been the subject of much dental research on periodontology (8), orthodontics and dentofacial orthopedics (9), endodontics (10), oral and maxillofacial surgery (11), forensic odontology (12), and especially dental-maxillofacial radiology and diagnostic studies (13-15). In light of these studies, we have developed DentisToday, the new dental AI software in the year 2020.
In this study, our main purpose is to evaluate the diagnostic performance of our AI software in comparison with 3 junior dentists, while the diagnosis of specialist dentists’ diagnoses is set as a true diagnosis. In this context, diagnostic performance will be evaluated as true diagnosis, misdiagnosis, and underdiagnosis. We have assumed that the specialist-trained AI performance on basic diagnostic capabilities regarding tooth caries and periapical infection images on radiographic interpretation will be higher than junior dentists’ capabilities. Another important study outcome is to evaluate the diagnostic duration of specialist and junior dentists’ total evaluation time with regard to the AI software because we assumed that an AI-based system will aid professionals in saving time during their routine clinical procedures.
Methods
Experiment datasets and processing
A total of 500 digital panoramic radiographs were involved in the study, from the archive of Istanbul Medipol University, School of Dental Faculty. The entire dataset, consisting of adult panoramic X-rays, was obtained through databases of this university, without revealing gender and age information. The data obtained were anonymized and stored to protect patient confidentiality. In order to diversify the dataset so that the model would be more generalizable, each X-ray image was preferred from this dental library. Since the X-rays are anonymized without using patient information, informed consent was waived by the Non-Intervetional Clinical Trials Ethics Committee of Istanbul Medipol University. Approval was obtained from the the Non-Intervetional Clinical Trials Ethics Committee of Istanbul Medipol University (No. 635. Dated 1st August 2022). The study was conducted in accordance with the Declaration of Helsinki (as revised in 2013).
For the present study, a prior statistical power analysis was performed using G*Power statistical software (G*Power 3.1; Heinrich-Heine-Universität Düsseldorf, Germany). To achieve a confidence threshold of 0.05, a sample size of 495 was required for adequate statistical power (1−β=95%) and 0.2 effect size (d) to detect a satisfactory (i.e., ≥0.90) area under the curve (AUC) with a null hypothesis value of 0.50.
Test samples
The 500 panoramic X-ray images selected for our study were screened for caries and periapical infection by two specialist dentists with 10 years of experience, the new dental AI software, and three junior dentists. Detection success (true diagnosis, misdiagnosis, and underdiagnosis) and the total time spent in detection were recorded. The first round of diagnoses was made by two specialist dentists separately, and only radiographs with their consensus were included in the study, to make up a total of 500 radiographs.
We have used recently developed dental AI-based software that aims to interpret radiographs with high success through successful deep neural network architectures such as Fast R-CNN, Faster R-CNN, SSD, and YoLo. During development of this software, a large training and test data set was created from the radiological images over 5,100 adult and 4,800 pedodontic panoramic X-ray images. Many dental problems have been labeled in detail using these images by the five specialist dentists who are two endodontists, two pedodontists and one dento- maxillofacial radiologist in the project team. Among the trained topics are; dental caries, periapical lesions, root canal treatments, periodontal bone loss, root remnants, impacted tooth, implants, fillings, crowns, bridges, tooth germs, amputations, developing root apexes etc. Existing treated deep neural network architectures were adapted to panoramic X-ray images using a transfer learning approach and subjected to an extensive training process. Python-based Keras, Tensorflow, and Caffe deep learning environments are used for algorithm development in this AI software. It can apply World Dental Federation (FDI) notation for teeth enumeration and detect the afore mentioned diseases on adult and pediatric radiographs (Figure 1).
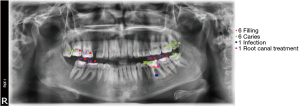
Detection of caries and periapical infections in the maxillo-mandibular area of panoramic radiographs were performed by specialist dentists, and these outcomes were stated as true diagnosis. Then the junior dentists were asked to observe the radiographs and perform their diagnosis regarding caries and periapical infections. Lastly, all radiographs were manually uploaded to the AI software to delineate and label the bounding boxes around locations of caries (Figure 2A) and infections (Figure 2B) for the detection of AI performance. All results were recorded to MS Excel, involving the diagnosis name (caries, periapical infection) and the regarded tooth number according to FDI annotation. These labelled radiographs were used to assess the success rate of the new AI software, in comparison with diagnoses from three junior dentists and two specialist dentists. True diagnosis, misdiagnosis, underdiagnosis were compared between all three groups as well as determination of the total diagnostic durations.
The diagnosis of the specialist dentists was set as the ground truth, and AI software and the three junior dentists have individually evaluated in terms of true diagnosis, misdiagnosis and underdiagnosis. The outcomes that are denoted for Junior Dentist 1, 2 and 3 (JD1, JD2 and JD3) for the three junior dentists, and also the new AI software; comprising caries detections, periapical lesion detections, and associated diagnosis times have been detected. An example of the AI response to an unlabelled X-ray is shown in Figure 3.
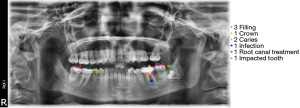
Statistical analysis and evaluation criteria
All statistical analyses were performed using SPSS 26.0 (IBM, Chicago, IL, USA). We evaluated diagnostic performance using the following metrics: sensitivity (SEN), specificity (SPEC), positive predictive value (PPV), negative predictive value (NPV), receiver operating characteristic (ROC) curves, and the area under the ROC curve (AUC).
SEN is used to indicate how well a test can classify subjects who actually have the outcome of interest and is calculated as the proportion of subjects correctly assigned as positive for the outcome among all subjects who are actually positive for the outcome. SPEC is used to indicate how well a test can classify subjects who do not actually have the outcome of interest and is calculated as the proportion of subjects correctly assigned as negative for the outcome among all subjects who are actually negative for the outcome.
PPV is the proportion of cases with positive test results who are already positive (patient). The NPV is the proportion of cases with negative test results who are already negative (healthy).
The ROC curve is an analytical method used to evaluate the performance of a binary diagnosis classification method and is displayed graphically. The AUC measures the entire two-dimensional area between (0,0) and (1,1) under the entire ROC curve (integral calculation). In addition, the F1-score was calculated as follows: 2 × (PPV × SEN)/(PPV + SEN).
Results
Interobserver correlation was performed with 50 samples to test the consistency of evaluation between two specialist dentists, based on Cohen’s κ coefficients.
The presence of caries and infection in the teeth was investigated through 500 X-ray images. In this study, the performance of junior dentists and AI was examined by comparison against the true base evaluation of two specialist dentists. Regarding interobserver correlation and Cohen’s κ coefficients; there is high consistency between the two specialists in terms of the evaluation of measurements (Table 1).
Table 1
Type of diagnosis | Interobserver correlation | Cohen’s κ | P |
---|---|---|---|
Caries | 0.937 | 0.57 | 0.001 |
Periapical lesion/infection | 0.961 | 0.673 | 0.001 |
Diagnostic duration of the dentists and AI software that is spent for diagnosing the X-ray images is presented in Table 2. It features the shortest average time, i.e., the greatest speed. The average time for a specialist dentist to evaluate an X-ray image was more than twice of the AI software (24.05 vs. 10 s).
Table 2
Detector | N | Minimum time (s) | Maximum time (s) | Mean time (s) ± Std Dev |
---|---|---|---|---|
JD1 | 500 | 10.00 | 65.00 | 31.97±15.13 |
JD2 | 500 | 5.00 | 198.00 | 57.72±31.01 |
JD3 | 500 | 2.00 | 70.00 | 26.94±1.80 |
AI | 500 | 10 | 10 | 10.00±0.00 |
Specialists (mean) | 500 | 4.00 | 70.00 | 24.05±12.96 |
AI, artificial intelligence; JD1, Junior Dentist 1; JD2, Junior Dentist 2; JD3, Junior Dentist 3.
Comparison for detection of caries
The specialists found no caries in 23.2% of the X-ray images, and no infection in 52% of them. The most common teeth for detected caries were 16, 17, 26, 36. The teeth most frequently identified as infected were 36 and 46 (Table 3).
Table 3
Tooth number | Caries | Infection/periapical lesion |
---|---|---|
None | 23.2% | 52.0% |
11 | 1.8% | 0.6% |
12 | 2.0% | 1.6% |
13 | 1.8% | 0.6% |
14 | 10.4% | 2.6% |
15 | 11.8% | 3.0% |
16 | 17.6% | 5.6% |
17 | 17.2% | 2.8% |
18 | 7.2% | 0.8% |
21 | 2.0% | 0.8% |
22 | 3.2% | 0.6% |
23 | 2.4% | 0.8% |
24 | 8.6% | 2.6% |
25 | 13.4% | 3.0% |
26 | 17.4% | 5.0% |
27 | 13.0% | 1.2% |
28 | 8.4% | 0.2% |
31 | 0.2% | 0.6% |
32 | 0.6% | 0.8% |
33 | 1.2% | 1.4% |
34 | 9.0% | 2.0% |
35 | 14.4% | 3.6% |
36 | 16.4% | 10.4% |
37 | 15.2% | 5.0% |
38 | 4.8% | 1.6% |
41 | 0.0% | 0.8% |
42 | 0.4% | 0.8% |
43 | 0.8% | 0.8% |
44 | 6.0% | 1.2% |
45 | 11.0% | 3.2% |
46 | 13.8% | 10.6% |
47 | 15.2% | 5.2% |
48 | 8.2% | 0.8% |
The AI detected dental caries at an SEN of 0.907, a SPEC of 0.760, a PPV of 0.693, an NPV of 0.505 and an F1-score of 0.786. Besides, JD1 has detected dental caries at an SEN of 0.889, a SPEC of 0.740, a PPV of 0.470, an NPV of 0.415 and an F1-score of 0.615. JD2 detected dental caries at an SEN of 0.491, a SPEC of 0.454, a PPV of 0.155, an NPV of 0.275 and an F1-score of 0.236. JD3 detected dental caries at an SEN of 0.907, a SPEC of 0.769660, a PPV of 0.666, an NPV of 0.367 and an F1-score of 0.768 (Table 4). According to the performance results, AI made the most successful detection (Figure 4). The counts for true diagnosis for JD1 is 141, for JD2 is 33, and for JD3 is 93 and AI is 194 true. The highest number of misdiagnoses was made by the 2nd junior dentist.
Table 4
Detector | True diagnosis | Underdiagnosis | Misdiagnosis | PPV | NPV | Sensitivity | Specificity | F1-score | AUC | Std. Error | 95% CI | P | |
---|---|---|---|---|---|---|---|---|---|---|---|---|---|
Lower | Upper | ||||||||||||
JD1 | 141 | 181 | 178 | 0.470 | 0.415 | 0.889 | 0.740 | 0.615 | 0.622 | 0.022 | 0.567 | 0.677 | 0.001 |
JD2 | 33 | 200 | 267 | 0.155 | 0.275 | 0.491 | 0.454 | 0.236 | 0.519 | 0.031 | 0.46 | 0.578 | 0.022 |
JD3 | 93 | 237 | 170 | 0.666 | 0.367 | 0.907 | 0.696 | 0.768 | 0.659 | 0.02 | 0.606 | 0.712 | 0.001 |
AI | 194 | 200 | 106 | 0.693 | 0.505 | 0.907 | 0.760 | 0.786 | 0.624 | 0.017 | 0.571 | 0.677 | 0.001 |
AI, artificial intelligence; PPV, positive predictive value; NPV, negative predictive value; AUC, area under the curve; CI, confidence interval; JD1, Junior Dentist 1; JD2, Junior Dentist 2; JD3, Junior Dentist 3.
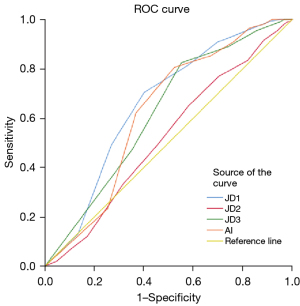
Comparison for detecting periapical infections
The AI detected infections at a SEN of 0.973, a SPEC of 0.629, a PPV of 0.861, a NPV of 0.689 and a F1-score of 0.914. Besides, JD1 detected infections at a SEN of 0.962, a SPEC of 0.421, a PPV of 0.651, a NPV of 0.673 and a F1-score of 0.777. JD2 detected infectious at a SEN of 0.758, a SPEC of 0.404, a PPV of 0.312, a NPV of 0.278 and a F1-score of 0.442. JD3 detected infectious at a SEN of 0.958, a SPEC of 0.621, a PPV of 0.648, a NPV of 0.546 and a F1-score of 0.773 (Table 5). According to the results obtained, AI was the most successful at detecting infections (Figure 5). JD1 has 307 true diagnoses, JD2 has 79, JD 3 has 294 and AI has 364 true diagnoses. The highest number of misdiagnoses was made by the 2nd junior dentist.
Table 5
Detector | True diagnosis | Underdiagnosis | Misdiagnosis | PPV | NPV | Sensitivity | Specificity | F1-score | AUC | Std. error | 95% CI | P | |
---|---|---|---|---|---|---|---|---|---|---|---|---|---|
Lower | Upper | ||||||||||||
JD1 | 307 | 56 | 137 | 0.651 | 0.673 | 0.962 | 0.421 | 0.777 | 0.773 | 0.022 | 0.73 | 0.816 | 0.001 |
JD2 | 79 | 126 | 295 | 0.312 | 0.278 | 0.758 | 0.404 | 0.442 | 0.641 | 0.022 | 0.593 | 0.719 | 0.001 |
JD3 | 294 | 86 | 120 | 0.648 | 0.546 | 0.958 | 0.621 | 0.773 | 0.833 | 0.019 | 0.796 | 0.87 | 0.001 |
AI | 364 | 67 | 69 | 0.861 | 0.689 | 0.973 | 0.629 | 0.914 | 0.872 | 0.017 | 0.838 | 0.905 | 0.001 |
AI, artificial intelligence; PPV, positive predictive value; NPV, negative predictive value; AUC, area under the curve; CI, confidence interval; JD1, Junior Dentist 1; JD2, Junior Dentist 2; JD3, Junior Dentist 3.
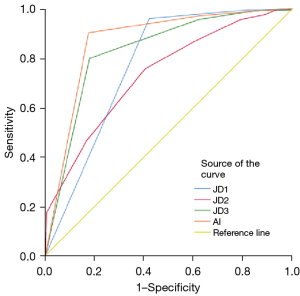
Discussion
AI in dentistry is used mainly to perform more accurate and efficient diagnosis, which is important in achieving the best results from the treatments provided, along with superior patient care. Under tight time constraints, dentists may lack in making the right clinical decision within a limited period. AI software can serve as their guide so that they can make better decisions and perform better (16). The AI models tested suggest prospects for a positive impact in assisting dental diagnostics, specifically in assisting dentists to achieve accurate interpretations of dental caries and infections (17).
AI diagnoses of periapical infection and caries have been evaluated and compared. In clinical practice, the caries diagnosis is performed through visual and tactile observation, and radiographs aid in identifying alterations in the appearance of teeth after the loss of enamel and dentin. CNNs have been demonstrated to correctly detect the presence of caries in approximately 4 out of 5 cases from these types of imaging data (18,19). Devito et al. used an ANN model for diagnosing proximal caries using bitewing radiographs and found quite encouraging results (20). Hung et al. demonstrated excellent outcomes from using AI technology to predict root caries (21).
Schwedicke et al. compared the cost-effectiveness of proximal caries detection on bitewing radiographs with vs. without AI, finding that AI was significantly more accurate than dentists and that AI was significantly more sensitive than dentists (7).
Mertens et al. reported that AI significantly increased the accuracy of caries detection using bitewing radiographs. They reported that AI can increase dentists’ diagnostic accuracy, mainly via increasing their sensitivity for detecting enamel lesions, but may also increase invasive therapy decisions (22).
In this study, the accuracy of detecting caries by new AI software was compared with that by junior dentists. AI software performed more successfully than the junior dentists.
In the case of dental pathologies, evidence of the presence of a periapical radiolucency on radiographs may be important in the detection of chronic inflammatory processes involving teeth. Periapical radiolucency can be detected through the presence and interpretation of specific radiographic signs. One study, using images cropped from panoramic radiographs to test CNNs for the detection of apical lesions, has concluded that the discriminatory ability of CNNs was satisfactory and highly sensitive in the imaging of molars, likely because of the reduced distortion of the images in the posterior areas of the jaw. Ekert et al. were successful in detecting apical lesion when they applied CNNs to this task on panoramic dental radiographs (23).
Geduk et al. evaluated the reliable detection of periapical pathologies using AI on panoramic radiographs. They stated that the AI software obtains results close to specialist dentists, and increases in the number of samples in AI-based commercial software prepared in this way. Retrospective detection would increase the accuracy, and this type of software would be more involved in clinical diagnosis (24). In this study, specialist dentists’ perceptions were set as the ground truth, and the accuracy of detecting periapical infection was compared among new AI software and junior dentists. AI performed more successful detection. It was observed that AI software tends to give more accurate results in periapical lesion detection compared to caries detection.
Song et al. presented a study based on panoramic radiographs testing detection of periapical lesions and found an F1-score value of 0.828 with an intersection over union (IoU) threshold of 0.3 (25). In this study, the F1-score of our AI software for detecting periapical lesions was found to be 0,914; while junior dentists scorings were found to be 0.77 for JD1, 0.442 for JD2 and 0,773 for JD3; that were all relatively lower than the AI outcomes. Both Song et al. (25) and we have proved to find very high results in detection of periapical lesions.
In addition, the total diagnosis speeds of specialist dentists, junior dentists and AI software were compared in this study. According to the results, AI software performed the evaluation most quickly. A study similar to this study was not found in the literature review. In this study, the most common teeth in which caries were detected were 16, 17, 26, and 36. Also, the number of teeth with the highest rate of infections was 36 and 46. These results are consistent with the results of similar studies (26-29).
Dentistry is an excellent discipline for applying AI because of its regular use of digitalized imaging and electronic health records. While there is plenty of active discussion on how AI may revolutionize dental practice, concerns remain regarding whether AI will eventually support dentists. Contemporary AI excels in utilizing formalized knowledge and extracting information from massive data sets. However, it fails to make associations like a human brain and can only partially perform complex decision-making in a clinical setting. Higher-level understanding that relies on the expertise of dentists is required, especially under ambiguous conditions, to conduct physical examinations, integrate medical histories, evaluate aesthetic outcomes, and facilitate discussion. AI should be viewed as an augmentation tool to enhance, and at times relieve, dentists so that they can perform more valuable tasks such as integrating patient information and improving professional interactions (30). We thought that AI would support junior dentists in dental radiologic diagnosis.
Conclusions
Caries and infections are frequently encountered in dentistry practice. According to the results obtained, we think that especially junior dentists should receive support from AI until they gain experience, both for the speed of diagnosis and in the correct diagnosis of diseases. With the new AI software, early and accurate diagnosis of caries and infections seems to be possible. Based on this study, we think that current AI software should be used more frequently in both endodontic practice and dentistry education.
Acknowledgments
We would like to thank the dental team at the radiological service of the Faculty of Dentistry at Medipol University for their invaluable technical support. The authors also thank Cambridge Proofreading Worldwide LLC, Chicago, Illinois, USA, for the English language editing of this manuscript.
Funding: None.
Footnote
Conflicts of Interest: All authors have completed the ICMJE uniform disclosure form (available at https://qims.amegroups.com/article/view/10.21037/qims-23-762/coif). The authors have no conflicts of interest to declare.
Ethical Statement: The authors are accountable for all aspects of the work in ensuring that questions related to the accuracy or integrity of any part of the work are appropriately investigated and resolved. This study was approved by the Non-Intervetional Clinical Trials Ethics Committee of Istanbul Medipol University (No. 635. Dated 1st August 2022). Informed consent was waived by the Non-Intervetional Clinical Trials Ethics Committee of Istanbul Medipol University. All methods were carried out in accordance with relevant guidelines and regulations of the Declaration of Helsinki (as revised in 2013).
Open Access Statement: This is an Open Access article distributed in accordance with the Creative Commons Attribution-NonCommercial-NoDerivs 4.0 International License (CC BY-NC-ND 4.0), which permits the non-commercial replication and distribution of the article with the strict proviso that no changes or edits are made and the original work is properly cited (including links to both the formal publication through the relevant DOI and the license). See: https://creativecommons.org/licenses/by-nc-nd/4.0/.
References
- Haque M, Sartelli M, Haque SZ. Dental Infection and Resistance-Global Health Consequences. Dent J (Basel) 2019.
- Ortiz R, Espinoza V. Odontogenic infection. Review of the pathogenesis, diagnosis, complications and treatment. Res Rep Oral Maxillofac Surg 2021;5:055.
- Walsh T, Macey R, Riley P, Glenny AM, Schwendicke F, Worthington HV, Clarkson JE, Ricketts D, Su TL, Sengupta A. Imaging modalities to inform the detection and diagnosis of early caries. Cochrane Database Syst Rev 2021;3:CD014545. [Crossref] [PubMed]
- Marmaneu-Menero A, Iranzo-Cortés JE, Almerich-Torres T, Ortolá-Síscar JC, Montiel-Company JM, Almerich-Silla JM. Diagnostic Validity of Digital Imaging Fiber-Optic Transillumination (DIFOTI) and Near-Infrared Light Transillumination (NILT) for Caries in Dentine. J Clin Med 2020;9:420. [Crossref] [PubMed]
- Corbella S, Srinivas S, Cabitza F. Applications of deep learning in dentistry. Oral Surg Oral Med Oral Pathol Oral Radiol 2021;132:225-38. [Crossref] [PubMed]
- Dahlander A, Soares F, Grindefjord M, Dahllöf G. Factors Associated with Dental Fear and Anxiety in Children Aged 7 to 9 Years. Dent J (Basel) 2019.
- Schwendicke F, Rossi JG, Göstemeyer G, Elhennawy K, Cantu AG, Gaudin R, Chaurasia A, Gehrung S, Krois J. Cost-effectiveness of Artificial Intelligence for Proximal Caries Detection. J Dent Res 2021;100:369-76. [Crossref] [PubMed]
- Lee JH, Kim DH, Jeong SN, Choi SH. Diagnosis and prediction of periodontally compromised teeth using a deep learning-based convolutional neural network algorithm. J Periodontal Implant Sci 2018;48:114-23. [Crossref] [PubMed]
- Xie X, Wang L, Wang A. Artificial neural network modeling for deciding if extractions are necessary prior to orthodontic treatment. Angle Orthod 2010;80:262-6. [Crossref] [PubMed]
- Saghiri MA, Asgar K, Boukani KK, Lotfi M, Aghili H, Delvarani A, Karamifar K, Saghiri AM, Mehrvarzfar P, Garcia-Godoy F. A new approach for locating the minor apical foramen using an artificial neural network. Int Endod J 2012;45:257-65. [Crossref] [PubMed]
- Aubreville M, Knipfer C, Oetter N, Jaremenko C, Rodner E, Denzler J, Bohr C, Neumann H, Stelzle F, Maier A. Automatic Classification of Cancerous Tissue in Laserendomicroscopy Images of the Oral Cavity using Deep Learning. Sci Rep 2017;7:11979. [Crossref] [PubMed]
- De Tobel J, Radesh P, Vandermeulen D, Thevissen PW. An automated technique to stage lower third molar development on panoramic radiographs for age estimation: a pilot study. J Forensic Odontostomatol 2017;35:42-54.
- Kaya E, Gunec HG, Gokyay SS, Kutal S, Gulum S, Ates HF. Proposing a CNN Method for Primary and Permanent Tooth Detection and Enumeration on Pediatric Dental Radiographs. J Clin Pediatr Dent 2022;46:293-8. [Crossref] [PubMed]
- Kaya E, Gunec HG, Aydin KC, Urkmez ES, Duranay R, Ates HF. A deep learning approach to permanent tooth germ detection on pediatric panoramic radiographs. Imaging Sci Dent 2022;52:275-81. [Crossref] [PubMed]
- Li S, Liu J, Zhou Z, Zhou Z, Wu X, Li Y, Wang S, Liao W, Ying S, Zhao Z. Artificial intelligence for caries and periapical periodontitis detection. J Dent 2022;122:104107. [Crossref] [PubMed]
- Khanagar SB, Al-Ehaideb A, Maganur PC, Vishwanathaiah S, Patil S, Baeshen HA, Sarode SC, Bhandi S. Developments, application, and performance of artificial intelligence in dentistry - A systematic review. J Dent Sci 2021;16:508-22. [Crossref] [PubMed]
- Ahmed N, Abbasi MS, Zuberi F, Qamar W, Halim MSB, Maqsood A, Alam MK. Artificial Intelligence Techniques: Analysis, Application, and Outcome in Dentistry-A Systematic Review. Biomed Res Int 2021;2021:9751564. [Crossref] [PubMed]
- Casalegno F, Newton T, Daher R, Abdelaziz M, Lodi-Rizzini A, Schürmann F, Krejci I, Markram H. Caries Detection with Near-Infrared Transillumination Using Deep Learning. J Dent Res 2019;98:1227-33. [Crossref] [PubMed]
- Schwendicke F, Elhennawy K, Paris S, Friebertshäuser P, Krois J. Deep learning for caries lesion detection in near-infrared light transillumination images: A pilot study. J Dent 2020;92:103260. [Crossref] [PubMed]
- Devito KL, de Souza Barbosa F, Felippe Filho WN. An artificial multilayer perceptron neural network for diagnosis of proximal dental caries. Oral Surg Oral Med Oral Pathol Oral Radiol Endod 2008;106:879-84. [Crossref] [PubMed]
- Hung M, Voss MW, Rosales MN, Li W, Su W, Xu J, Bounsanga J, Ruiz-Negrón B, Lauren E, Licari FW. Application of machine learning for diagnostic prediction of root caries. Gerodontology 2019;36:395-404. [Crossref] [PubMed]
- Mertens S, Krois J, Cantu AG, Arsiwala LT, Schwendicke F. Artificial intelligence for caries detection: Randomized trial. J Dent 2021;115:103849. [Crossref] [PubMed]
- Ekert T, Krois J, Meinhold L, Elhennawy K, Emara R, Golla T, Schwendicke F. Deep Learning for the Radiographic Detection of Apical Lesions. J Endod 2019;45:917-922.e5. [Crossref] [PubMed]
- Geduk G, Biltekin H, Şeker Ç. Artificial intelligence reliability assessment in the diagnosis of apical pathology in panoramic radiographs: a comparative study on different threshold values. Selcuk Dental Journal 2022;9:126-32.
- Song IS, Shin HK, Kang JH, Kim JE, Huh KH, Yi WJ, Lee SS, Heo MS. Deep learning-based apical lesion segmentation from panoramic radiographs. Imaging Sci Dent 2022;52:351-7. [Crossref] [PubMed]
- Marthaler TM. Changes in dental caries 1953-2003. Caries Res 2004;38:173-81. [Crossref] [PubMed]
- Akın H, Tugut F, Güney Ü, Akar T, Özdemir AK. Evaluation of the effects of age, gender, education and income levels on the tooth loss and prosthetic treatment. Cumhuriyet Dent J 2011;14:204-10.
- Dye BA, Hsu KL, Afful J. Prevalence and Measurement of Dental Caries in Young Children. Pediatr Dent 2015;37:200-16.
- Akbaş M, Akbulut MB. Prevalence of Apical Periodontitis and Quality of Root Canal Filling in a Selected Young Turkish Population. NEU Dent J 2020;2:52-8.
- Recht M, Bryan RN. Artificial Intelligence: Threat or Boon to Radiologists? J Am Coll Radiol 2017;14:1476-80. [Crossref] [PubMed]