Development and validation of a nomogram for preoperative prediction of immature teratoma in children with teratoma: a retrospective, multicenter, diagnostic study
Introduction
Teratomas are derived from embryonic stem cells and are the most common germ cell tumors in children (1,2). Histologically, teratomas are classified as immature teratomas (ITs) or mature teratomas (MTs) based on the presence or absence of immature tissues within the tumor (3). Although both ITs and MTs are benign and have a good prognosis with surgery, an IT can present malignant behavior and metastasis when foci of malignant germ cell elements are present or the tumor is not completely resected (4), and the disease-free survival and overall survival of IT patients are much lower than those of MT patients (5,6). Hence, the preoperative work-up should include MT identification and IT identification as this may affect the surgical approach, the type of procedure, and future reproductive health. For example, laparoscopy and ovarian-sparing surgery are often performed in in cases of MT when compared to laparotomy, and ovariectomy is recommended in cases of IT (7). Unfortunately, due to the lack of accurate preoperative diagnosis, only 36% of the initial surgical procedures comply fully with surgical guidelines (8), and the choice of an operative technique (either laparoscopic or open) depends solely on a surgeon’s preference (9). Additionally, sufficient sampling of the resected specimens to identify immature tissues is the most important method for the diagnosis of ITs (10). However, the variety of tissue architecture in teratomas may create uncertainty in pathological diagnosis. Therefore, preoperative identification of MTs and ITs is helpful in determining treatment options and in performing tumor sampling.
Preoperative evaluations for teratomas include ultrasound, computed tomography (CT) or magnetic resonance imaging (MRI) and other appropriate laboratory examinations (6). MRI and CT are complementary to one another. MRI has excellent capabilities in soft tissue characterization and is preferred in pediatric patients to avoid radiation exposure. CT has the advantages of a short scan time, low sedation rate and malignant staging, and more importantly, it can detect characteristic intratumoral calcification. In some cases, CT can be recommended as a second-line imaging modality for pediatric patients.
CT or MRI can be used to easily diagnose teratomas based on the recognition of intratumoral fat and calcification (10). However, it remains challenging to differentiate ITs from MTs. Previous studies have shown that ITs usually present as larger tumors with a dominance of solid components containing scattered areas of fat and calcification (11-13). However, the imaging features of MTs and ITs sometimes overlap with each other. For example, MTs with dominance of solid parts and ITs with dominance of cystic parts are occasionally seen in clinical work (12,14). In addition, there has been a lack of systematic comparison between the imaging features of these two types of teratomas, and the diagnostic performance of these features in the identification of MTs and ITs remains unclear. Additionally, few studies have been conducted on the imaging features of ITs. Most of them are devoted to adult ovarian ITs, and few are concerned with ITs in children. It has been reported that ITs in children present different biological behaviors when compared with ITs in adult (15). Hence, a systematic study should be conducted on these imaging findings to expound their diagnostic value in distinguishing ITs from MTs in children.
There is no complete diagnostic criterion for ITs (16). Herein, this study was designed to establish and validate a diagnostic nomogram based on clinical and CT features for the preoperative prediction of ITs in children.
Methods
Study design and patients
We conducted a multicenter retrospective diagnostic study that involved 786 children with pathologically confirmed teratomas from two independent institutions (Institution I: Zhejiang University Children’s Hospital, Hangzhou, China; and Institution II: Anhui Provincial Children’s Hospital, Hefei, China). The eligibility of these patients was assessed based on the inclusion and exclusion criteria, and 632 patients were finally included. The 519 patients from Institution I, from January 2013 to October 2022, were randomly allocated to the training set (n=364) and the internal validation set (n=155) at a 7:3 ratio, with a seed of 78. The 113 patients at Institution II from February 2017 to October 2022 constituted an independent external validation set. The purpose of dividing the data into three sets was to better evaluate and optimize the performance of the model. The training set is responsible for training the model, the independent validation set is responsible for verifying whether the model is overfitting, and the external set is used to test performance. The research flow chart is shown in Figure 1. This retrospective research was approved by the ethics committees of Zhejiang University Children’s Hospital and Anhui Provincial Children’s Hospital. The requirement for informed consent was waived due to its retrospective nature of the study. The study was conducted in accordance with the Declaration of Helsinki (as revised in 2013).
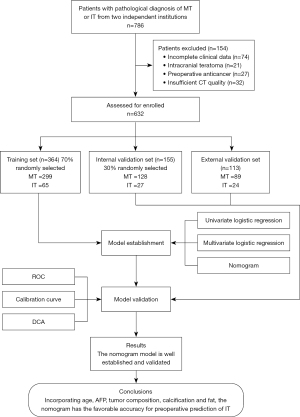
The following were the criteria for inclusion: (I) pathologically diagnosed with MT or IT; (II) having received CT scan within 1 month before surgery. The exclusion criteria were (I) incomplete data on clinical features or laboratory tests, (II) preoperative antitumor therapy, (III) intracranial teratoma, and (IV) insufficient CT image quality. According to the exclusion criteria, patients who had missing values for clinical features or laboratory tests were excluded.
Outcome measure
The outcome of interest was IT, defined as the presence of immature tissues within the tumor histologically (3). IT was confirmed by two pathologists who had 12 and 26 years of experience in pediatric pathology.
Clinical data extraction
Baseline clinical data were extracted from the patients’ medical records, including gender, age, preoperative serum AFP level and pathological results. Positive for serum AFP was defined as a serum AFP level above the age-related upper limit of normal that has been published (17).
CT examination and feature extraction
CT scans were performed at Institution I using 64-row and 16-row CT scanners, and a 64-row CT scanner was used at Institution II. The position of examination depended on the primary site, including the head and neck regions, chest, and abdominal and pelvic cavities. The level of total dose length product (DLP) was adjusted with the Chinese diagnostic reference levels in pediatric CT (18). The same imaging parameters were used at the two institutions: reconstruction using 5 mm thickness slices, 350–400 mm FOV, and 120-kV tube potential with automatic tube current modulation.
All images were interpreted by two pediatric radiologists independently (reader 1: 10 years of experience in pediatric radiology; reader 2: 20 years of experience in pediatric radiology). Disagreements were settled by consultation with a third radiologist (reader 3: having 25 years of experience in pediatric radiology). The three radiologists were blinded to the pathology results.
The following CT findings were analyzed: tumor site (gonadal or extragonadal), size, tumor composition (≥50% solid or <50% solid), calcification (nonpleomorphic, pleomorphic, or not visible), and fat (non-scattered, scattered, and not visible). Tumor size was represented by the maximum tumor diameter on axial, coronary or sagittal views. The solid component ratio of the tumor was ≥50% if the ratio of the area of solid components over the maximum cross-section of the tumor to the entire tumor area was greater than or equal to 50%. Pleomorphic calcification was defined as scattered calcification with varying size, morphology and density (Figure 2A). Scattered fat was defined as small foci of fat in a scattered distribution (Figure 2B). The intraclass correlation coefficient (ICC) was used to assess the interobserver reliability in the interpretation of CT findings.
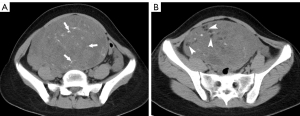
Risk factor screening and nomogram model building
Univariate logistic regression analysis was performed on the training set to screen candidate IT-related clinical and CT features, and the screening criterion was a P value <0.05. Then, multivariable logistic backward stepwise regression analysis was performed to further screen out the significant risk factors for IT on the criterion of a P value <0.05. The best diagnostic model was chosen based on the lowest Akaike’s information criterion (AIC). A nomogram was finally established based on the strength of the results of multivariate logistic regression analysis.
Evaluation of the screened risk factors
The chi-square statistic (χ2) and degree of freedom (df) of the risk factors involved in the nomogram model were calculated, and then the importance evaluation chart was drawn for the risk factors.
Assessment of nomogram performance
The performance of the nomogram model was assessed by discrimination, calibration, and clinical usefulness (19). The model’s discrimination was assessed using the area under the curve (AUC). AUCs and their 95% confidence interval (CI) were calculated. Calibration was assessed using the Hosmer-Lemeshow test and calibration curves. Decision curve analysis (DCA) curves were employed to assess the model’s clinical usefulness.
Validation of the nomogram model
The discrimination, calibration, and clinical usefulness of the model were validated on the internal and external validation sets using the same method.
Statistical analysis
Statistical analyses were performed using R (ver. 4.1.3), to establish and validate the nomogram. All statistical tests were two-sided, and a P value <0.05 was considered statistically significant. Continuous variables and categorical variables were expressed as medians (quartiles) and percentages. Intergroup differences between MT and IT were analyzed for these two types of variables using the Wilcoxon test and Fisher’s exact test, respectively. Optimal binning was performed for continuous variables to find high risk cutoffs using the “smbinning” package (20). Interobserver consistency was assessed using the “psych” package. The nomogram model was established, and the calibration curve was plotted using the “rms” package. The importance plot for risk factors was drawn using the “ggplot2” package. The receiver operating characteristic (ROC) curve was plotted using the “pROC” package. The DCA curve was plotted using the “ggDCA” package.
Results
Clinical characteristics of patients
Among 786 patients, 632 patients conformed to the inclusion criteria and were included. The training set and the internal validation set included 364 (MT, 82.1%; IT, 17.9%) and 155 patients (MT, 82.6%; IT, 17.4%), respectively. The external validation set included 113 patients (MT, 78.8%; IT, 21.2%). Based on the optimal binning method, the cutoffs for age were 2.47 and 6.57 months, and the cutoff for maximum tumor diameter was 106.1 mm. The clinical and CT features of the patients are detailed in Table 1. Both observers had high reliability in interpreting all of the CT findings (Table 2).
Table 1
Characteristics | Training set (N=364) | Internal validation set (N=155) | External validation set (N=113) | ||||||||
---|---|---|---|---|---|---|---|---|---|---|---|
MT (n=299) | IT (n=65) | P value | MT (n=128) | IT (n=27) | P value | MT (n=89) | IT (n=24) | P value | |||
AFP, ng/mL | <0.001 | <0.001 | <0.001 | ||||||||
Median (IQR) | 2.2 (1.1–30.8) |
474 (38.7–12,100) |
2.1 (1.1–16.9) |
120 (57.6–12,800) |
2.2 (1.5–42) |
289 (64.5–4,280) |
|||||
Negative | 281 (94.0) | 36 (55.4) | 120 (93.8) | 12 (44.4) | 84 (94.4) | 13 (54.2) | |||||
Positive | 18 (6.0) | 29 (44.6) | 8(6.3) | 15 (55.6) | 5 (5.6) | 11 (45.8) | |||||
Age, months | <0.001 | 0.019 | 0.052 | ||||||||
Median (IQR) | 36.0 (7.15–104) |
5.40 (2.00–12.0) |
41.5 (9.00–106) |
5.27 (2.70–79.0) |
63.8 (13.5–105) |
5.75 (1.63–107) |
|||||
≥6.57 | 229 (76.6) | 25 (38.5) | 104 (81.3) | 12 (44.4) | 76 (85.4) | 11 (45.8) | |||||
2.47–6.57 | 22 (7.4) | 23 (35.4) | 6 (4.7) | 8 (29.6) | 7(7.9) | 4 (16.7) | |||||
≤2.47 | 48 (16.1) | 17 (26.2) | 18 (14.1) | 7 (25.9) | 6 (6.7) | 9 (37.5) | |||||
Gender | 0.07 | 0.810 | 0.090 | ||||||||
Female | 221 (73.9) | 35(53.8) | 110 (85.9) | 20(74.1) | 74 (83.1) | 16 (66.7) | |||||
Male | 78 (26.1) | 30 (46.2) | 18 (14.1) | 7 (25.9) | 15 (16.9) | 8 (33.3) | |||||
Size, mm | <0.001 | <0.001 | 0.003 | ||||||||
Median (IQR) | 67.8 (42.0–98.9) |
103 (66.7–141) |
64.9 (38.9–98.5) |
120 (77.2–165) |
70.8 (47.5–102) |
117 (74.5–152) |
|||||
≤106.1 | 231 (77.3) | 34 (52.3) | 102 (79.7) | 11 (40.7) | 60 (67.4) | 10 (41.7) | |||||
>106.1 | 68 (22.7) | 31 (47.7) | 26 (20.3) | 16 (59.3) | 29 (32.6) | 14 (58.3) | |||||
Site | <0.001 | 0.508 | 0.006 | ||||||||
Extragonadal | 132 (44.1) | 42 (64.6) | 47 (36.7) | 16 (59.3) | 34 (38.2) | 17 (70.8) | |||||
Gonadal | 167 (55.9) | 23(35.4) | 81 (63.3) | 11 (40.7) | 55 (61.8) | 7 (29.2) | |||||
Fat | <0.001 | <0.001 | <0.001 | ||||||||
Non-scattered | 203 (67.9) | 10 (15.4) | 92 (71.9) | 5 (18.5) | 66 (74.2) | 1 (4.2) | |||||
Not visible | 52 (17.4) | 20 (30.8) | 23 (18.0) | 9 (23.3) | 10 (11.2) | 7 (29.2) | |||||
Scattered | 44 (14.7) | 35 (53.8) | 13 (10.2) | 13 (48.1) | 13 (14.6) | 16 (66.7) | |||||
Tumor composition | <0.001 | <0.001 | <0.001 | ||||||||
Solid <50% | 266 (89.0) | 15 (23.1) | 110 (85.9) | 7 (25.9) | 76 (85.4) | 5 (20.8) | |||||
Solid ≥50% | 33 (11.0) | 50 (76.9) | 18 (14.1) | 20 (74.1) | 13 (14.6) | 19 (79.2) | |||||
Calcification | <0.001 | <0.001 | <0.001 | ||||||||
Non-pleomorphic | 177 (59.2) | 6 (9.2) | 65 (50.8) | 3 (11.1) | 51 (57.3) | 3 (12.5) | |||||
Pleomorphic | 78 (26.1) | 50 (76.9) | 38 (29.7) | 22 (81.5) | 32 (36.0) | 21 (87.5) | |||||
Not visible | 44 (14.7) | 9 (13.8) | 25 (19.5) | 2 (7.4) | 6 (6.7) | 0 (0.0) |
Categorical and continuous variables are presented as frequency (percent) or median (IQR). Group differences in categorical and continuous variables are analyzed using Chi-squared test or Wilcoxon test as appropriate. CT, computed tomography; IQR, interquartile range; MT, mature teratoma; IT, immature teratoma; AFP, alpha-fetoprotein.
Table 2
Parameter | ICC value* (95% CI) | P value |
---|---|---|
Tumor composition | 0.90 (0.82–0.94) | <0.001 |
Calcification | 0.87 (0.77–0.93) | <0.001 |
Fat | 0.86 (0.75–0.92) | <0.001 |
Size | 0.96 (0.92–0.98) | <0.001 |
*, agreement for the ICC values was defined as follows: 0.00–0.20, slight agreement; 0.21–0.40, fair agreement; 0.41–0.60, moderate agreement; 0.61–0.80, substantial agreement; and 0.81–1.00, excellent agreement. ICC, intraclass correlation coefficient; CI, confidence interval.
Screening risk factors for the nomogram model
The univariate logistic regression analysis showed that age, AFP, site, size, tumor composition, calcification and fat were potential predictors of IT (P<0.05). All of the above candidate variables were subsequently included in the multivariate logistic regression analysis. Finally, five variables were included in the nomogram, namely, age [2.47–6.57 months, odds ratio (OR) =4.42], AFP (positive, OR =3.25), tumor composition (≥50% solid, OR =8.23), calcification (pleomorphic, OR =4.41), and fat (scattered, OR =1.84; not visible, OR =3.11) (Figure 3). The model with the lowest AIC value (value =202) was finally chosen.
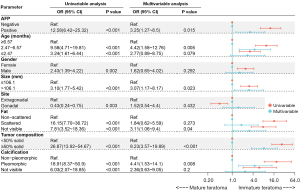
Evaluation of the screened risk factors
The relative importance of the five variables is shown in Figure 4. Among them, tumor composition was the most important risk factor (χ2-df =24.92), followed by serum AFP level (7.54) and age (6.44).
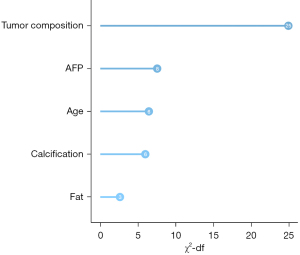
Construction and assessment of the nomogram model
Based on the results of the multivariate logistic regression, a nomogram incorporating the five significant risk factors was developed for predicting IT (Figure 5). The AUC of the nomogram was 0.92 (95% CI: 0.88–0.96) in the training set, with a sensitivity of 80% and specificity of 90% at the cutoff value of 0.262 (Figure 6). The calibration curve of the nomogram indicated good agreement between the predictive probability of the nomogram and the actual probability (Figure 7). The Hosmer-Lemeshow test was not significant (P=0.98), indicating a good fitting model. The DCA curves of the nomogram showed that if the threshold probability was 0.02–0.91, using this nomogram to identify IT could have a greater net benefit than either the treat-all tactic or the treat-none tactic. The net benefit of the nomogram was 11% in the training set at the threshold probability of 0.262 (Figure 8).
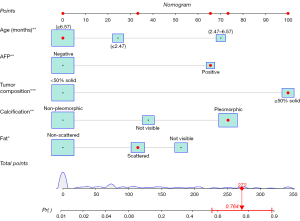
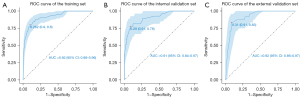
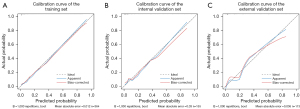
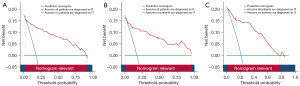
Validation of the nomogram model
The nomogram had satisfactory AUCs in both internal and external validation sets, with values of 0.91 (95% CI: 0.84–0.97) and 0.92 (95% CI: 0.86–0.97) (Figure 6), respectively. Likewise, the nomogram also had good calibration in the two validation sets (Figure 7) and was further supported by the nonsignificant result of the Hosmer-Lemeshow test (P=0.91 and 0.53, respectively). DCA curves showed good net benefits in the two sets at threshold probabilities of 0.01–0.96 and 0.02–0.78. At the threshold probability of 0.262, the net benefit was 10.6% and 14% in the two sets, respectively (Figure 8).
Discussion
A nomogram for predicting IT was established and validated in 653 teratoma cases from a multicenter database. The model, which had five independent risk factors for IT, was suitable for predicting the risk of IT in individual patients. These risk factors were ranked in descending order of importance as follows: tumor composition, AFP, age, calcification and fat. This model showed excellent performance in the training set, internal validation set, and external validation set. DCA curves suggested that the nomogram was a reliable tool for aiding treatment decision-making.
Imaging plays vital roles in the preoperative evaluation and postoperative follow-up of teratomas. On CT, an IT usually presents as a large irregular solid component containing coarse calcifications and small foci of fat, which is considered a useful feature for differentiating between MTs and ITs (11,14). Similarly, we found that tumor composition was the most important variable in the nomogram, and dominance of solid components had the highest risk score in the nomogram model, which may be related to its pathological composition. The solid components, which may be completely solid or mixed with cystic components on CT and MR, often represent immature tissues (16,21). Liu et al. reported that the presence of a solid portion with marked enhancement or the thick wall of the tumor indicates a malignant element (22). It was once believed that MTs and ITs were differentiated from each other by the distribution pattern of intratumoral fat. The fat within IT lesions is generally smaller and scattered and distributed amidst solid components, while the fat within MT lesions is largely spherical in shape and located on the periphery or in the center (23). Our study results were in agreement with the above, confirming that the scattered distribution of intratumoral fat was an independent risk factor for ITs. We also found that an absence of intratumoral fat was another important CT finding that differentiated ITs from MTs. In addition, the risk score of absence of fat was higher than that of the scattered distribution in the nomogram. According to another study, 6–7% of MT lesions did not contain fat (24), although the absence of fat in ITs has not yet been reported. We hypothesized that the pattern of intratumoral fat in ITs may be related to whether immature mesenchyme differentiated into adipocytes and the degree of differentiation. ITs contain abundant areas of immature mesenchyme that can differentiate into adipose tissue (25). On CT, intratumoral calcification was another important feature differentiating ITs from MTs (13) since it is more random or dispersed distributed and coarser in ITs when compared to the focally distributed pattern found in MTs (11,16). In the present study, pleomorphic calcification, defined as scattered calcification with varying size, morphology and density, was identified as an independent risk factor for ITs. This type of calcification may be attributed to fragments of calcified cartilage or bone (21).
Clinical features are indispensable for the identification of ITs. Both ITs and MTs can cause a mild increase in serum AFP levels. However, due to the low sensitivity of AFP, AFP alone is insufficient for the differential diagnosis between MT and IT (26). This problem can be resolved by combining the AFP level with imaging and other clinical features (27). In this study, the AFP level and proportion of AFP-positive patients in the IT group were higher than those in the MT group. The presence of immature endodermal elements has been reported to be the cause of elevated AFP levels (28). However, AFP levels higher than 1,000 ng/mL were more likely to indicate malignant elements within the tumor (29). Loh et al. recommend using age-related tables to determine whether serum AFP levels are elevated due to a broad range of AFP values in infants below the age of 1 year (26). Terenziani et al. reported a younger median age in children with ITs than in those with MTs (7 vs. 55 months), which is a useful feature for differentiating between the two types (1). We reported a similar finding, where the median age differed significantly between children with IT and MT (5.49 vs. 43 months, P<0.001). In the nomogram established in this study, age was the third most important predictor. In addition, the risk score of the age group of 2.47 to 6.57 months was higher than that of the other two age groups.
We developed a prediction model based on readily accessible and noninvasive factors and displayed it with a nomogram. As a type of clinical prediction model, nomograms transform complex regression equations into a visual graph, making clinical predictive models easy to use and understand (30,31). Using the dynamic nomogram by inputting the clinical and CT features can quickly determine the possibility of IT. This nomogram showed satisfactory discrimination in predicting IT in the training set (AUC =0.92), internal validation set (AUC =0.91) and external validation set (AUC =0.92) with good calibration and favorable clinical usefulness. The fact that the performance of the nomogram had been validated on an independent and external validation set suggests its bright prospects in clinical practice and supports its use in clinical referral across hospitals. We believe that the nomogram can assist radiologists and oncologists in making more accurate teratoma classification and treatment decisions, thus reducing unnecessary invasive surgeries for pediatric patients.
However, the present study had certain limitations. First, we excluded patients who had not received preoperative CT scans or those with poor-quality CT images, resulting in potential selection bias. To mitigate this problem, we validated the nomogram on an external validation set, and the nomogram demonstrated good predictive performance. Second, CT findings of MT and IT were subjectively, but independently, interpreted by two radiologists who had rich experience in pediatric radiology; the ICC was above 0.85, indicating good repeatability and reliability. Finally, we only differentiated between MT and IT due to limitations of the data and did not differentiate between either of the two and malignant mixed teratomas. In the future, we will collect more data to build a predictive model for malignant mixed teratomas.
Conclusions
A nomogram for the differentiation between MT and IT was established and validated. This nomogram involved five variables, namely, age, AFP, tumor composition, fat, and calcification and was proven to have favorable accuracy for the preoperative prediction of IT. As an easy-to-use and noninvasive tool, this nomogram may help in clinical decision-making and risk stratification.
Acknowledgments
Funding: This research was supported by
Footnote
Conflicts of Interest: All authors have completed the ICMJE uniform disclosure form (available at https://qims.amegroups.com/article/view/10.21037/qims-23-600/coif). JF reports that this work was supported by the national key research and development program of China (No. 2021YFC2701901). The other authors have no conflicts of interest to declare.
Ethical Statement: The authors are accountable for all aspects of the work in ensuring that questions related to the accuracy or integrity of any part of the work are appropriately investigated and resolved. This retrospective study was conducted in accordance with the Declaration of Helsinki (as revised in 2013) and was approved by the Ethics Committees of Zhejiang University Children’s Hospital and Anhui Provincial Children’s Hospital. The requirement for informed consent was waived due to the retrospective nature of the study.
Open Access Statement: This is an Open Access article distributed in accordance with the Creative Commons Attribution-NonCommercial-NoDerivs 4.0 International License (CC BY-NC-ND 4.0), which permits the non-commercial replication and distribution of the article with the strict proviso that no changes or edits are made and the original work is properly cited (including links to both the formal publication through the relevant DOI and the license). See: https://creativecommons.org/licenses/by-nc-nd/4.0/.
References
- Terenziani M, D'Angelo P, Inserra A, Boldrini R, Bisogno G, Babbo GL, Conte M. Dall' Igna P, De Pasquale MD, Indolfi P, Piva L, Riccipetitoni G, Siracusa F, Spreafico F, Tamaro P, Cecchetto G. Mature and immature teratoma: A report from the second Italian pediatric study. Pediatr Blood Cancer 2015;62:1202-8. [Crossref] [PubMed]
- Sharma S, Aronson DC, Gupta DK, Lakhoo K. Teratomas. In: Ameh EA, Bickler SW, Lakhoo K, Nwomeh BC, Poenaru D, editors. Pediatric Surgery: A Comprehensive Textbook for Africa. Cham: Springer International Publishing; 2020:1093-101.
- Harms D, Zahn S, Göbel U, Schneider DT. Pathology and molecular biology of teratomas in childhood and adolescence. Klin Padiatr 2006;218:296-302. [Crossref] [PubMed]
- Schneider DT, Terenziani M, Cecchetto G, Olson TA. Gonadal and Extragonadal Germ Cell Tumors, Sex Cord Stromal and Rare Gonadal Tumors. In: Schneider DT, Brecht IB, Olson TA, Ferrari A, editors. Rare Tumors In Children and Adolescents. Berlin, Heidelberg: Springer Berlin Heidelberg; 2012:327-402.
- Mann JR, Gray ES, Thornton C, Raafat F, Robinson K, Collins GS, Gornall P, Huddart SN, Hale JP, Oakhill AUK Children's Cancer Study Group Experience. Mature and immature extracranial teratomas in children: the UK Children's Cancer Study Group Experience. J Clin Oncol 2008;26:3590-7. [Crossref] [PubMed]
- PDQ Pediatric Treatment Editorial Board. Childhood Extracranial Germ Cell Tumors Treatment (PDQ®): Health Professional Version. In: PDQ Cancer Information Summaries [Internet]. Bethesda (MD): National Cancer Institute (US); 2002.
- Gkrozou F, Tsonis O, Vatopoulou A, Galaziou G, Paschopoulos M. Ovarian Teratomas in Children and Adolescents: Our Own Experience and Review of Literature. Children (Basel) 2022;9:1571. [Crossref] [PubMed]
- Cushing B, Giller R, Ablin A, Cohen L, Cullen J, Hawkins E, Heifetz SA, Krailo M, Lauer SJ, Marina N, Rao PV, Rescorla F, Vinocur CD, Weetman RM, Castleberry RP. Surgical resection alone is effective treatment for ovarian immature teratoma in children and adolescents: a report of the pediatric oncology group and the children's cancer group. Am J Obstet Gynecol 1999;181:353-8. [Crossref] [PubMed]
- Marina NM, Cushing B, Giller R, Cohen L, Lauer SJ, Ablin A, Weetman R, Cullen J, Rogers P, Vinocur C, Stolar C, Rescorla F, Hawkins E, Heifetz S, Rao PV, Krailo M, Castleberry RP. Complete surgical excision is effective treatment for children with immature teratomas with or without malignant elements: A Pediatric Oncology Group/Children's Cancer Group Intergroup Study. J Clin Oncol 1999;17:2137-43. [Crossref] [PubMed]
- Selvaggi SM. Tumors of the ovary, maldeveloped gonads, fallopian tube, and broad ligament. Arch Pathol Lab Med 2000;124:477.
- Saida T, Mori K, Masumoto T, Hoshiai S, Ishiguro T, Sakai M, Hara T, Ochi H, Satoh T, Minami M. Ovarian and non-ovarian teratomas: a wide spectrum of features. Jpn J Radiol 2021;39:143-58. [Crossref] [PubMed]
- Outwater EK, Siegelman ES, Hunt JL. Ovarian teratomas: tumor types and imaging characteristics. Radiographics 2001;21:475-90. [Crossref] [PubMed]
- Srisajjakul S, Prapaisilp P, Bangchokdee S. Imaging features of unusual lesions and complications associated with ovarian mature cystic teratoma. Clin Imaging 2019;57:115-23. [Crossref] [PubMed]
- Alotaibi MO, Navarro OM. Imaging of ovarian teratomas in children: a 9-year review. Can Assoc Radiol J 2010;61:23-8. [Crossref] [PubMed]
- Łuczak J, Bagłaj M, Dryjański P. What recent primary studies tell us about ovarian teratomas in children: a scoping review. Cancer Metastasis Rev 2020;39:321-9. [Crossref] [PubMed]
- Saba L, Guerriero S, Sulcis R, Virgilio B, Melis G, Mallarini G. Mature and immature ovarian teratomas: CT, US and MR imaging characteristics. Eur J Radiol 2009;72:454-63. [Crossref] [PubMed]
- Blohm ME, Vesterling-Hörner D, Calaminus G, Göbel U. Alpha 1-fetoprotein (AFP) reference values in infants up to 2 years of age. Pediatr Hematol Oncol 1998;15:135-42. [Crossref] [PubMed]
- Bai RJ CJ, Dong DD, Gao H. Expert consensus on the diagnostic reference level of CT radiation dose. Chin J Radiol 2017;51:817-22.
- Alba AC, Agoritsas T, Walsh M, Hanna S, Iorio A, Devereaux PJ, McGinn T, Guyatt G. Discrimination and Calibration of Clinical Prediction Models: Users' Guides to the Medical Literature. JAMA 2017;318:1377-84. [Crossref] [PubMed]
- Cleophas TJ, Zwinderman AH. Optimal Binning for Finding High Risk Cut-offs (1445 Families). In: Cleophas TJ, Zwinderman AH, editors. Machine Learning in Medicine – A Complete Overview. Cham: Springer International Publishing; 2020:473-9.
- Brammer HM 3rd, Buck JL, Hayes WS, Sheth S, Tavassoli FA. From the archives of the AFIP. Malignant germ cell tumors of the ovary: radiologic-pathologic correlation. Radiographics 1990;10:715-24. [Crossref] [PubMed]
- Liu Z, Lv X, Wang W, An J, Duan F, Feng X, Chen X, Ouyang B, Li S, Singh S, Qiu S. Imaging characteristics of primary intracranial teratoma. Acta Radiol 2014;55:874-81. [Crossref] [PubMed]
- Cho A, Kim SW, Choi J, Kang W, Lee JD, Yun M. The additional value of attenuation correction CT acquired during 18F-FDG PET/CT in differentiating mature from immature teratomas. Clin Nucl Med 2014;39:e193-6. [Crossref] [PubMed]
- Poncelet E, Delpierre C, Kerdraon O, Lucot JP, Collinet P, Bazot M. Value of dynamic contrast-enhanced MRI for tissue characterization of ovarian teratomas: correlation with histopathology. Clin Radiol 2013;68:909-16. [Crossref] [PubMed]
- Bonasoni MP, Comitini G, Barbieri V, Palicelli A, Salfi N, Pilu G. Fetal Presentation of Mediastinal Immature Teratoma: Ultrasound, Autopsy and Cytogenetic Findings. Diagnostics (Basel) 2021;11:1543. [Crossref] [PubMed]
- Loh AH, Gee KW, Chua JH. Diagnostic accuracy of preoperative alpha-fetoprotein as an ovarian tumor marker in children and adolescents: not as good as we thought? Pediatr Surg Int 2013;29:709-13. [Crossref] [PubMed]
- Lawrence AE, Fallat ME, Hewitt G, Hertweck P, Onwuka A, Afrazi A, et al. Understanding the Value of Tumor Markers in Pediatric Ovarian Neoplasms. J Pediatr Surg 2020;55:122-5. [Crossref] [PubMed]
- Busmanis I, Tay SK. Recurrent immature teratoma: lack of correlation between serum level and immunohistochemical detection of serum alpha-fetoprotein. Pathology 1998;30:77-9. [Crossref] [PubMed]
- Pashankar F, Hale JP, Dang H, Krailo M, Brady WE, Rodriguez-Galindo C, Nicholson JC, Murray MJ, Bilmire DF, Stoneham S, Arul GS, Olson TA, Stark D, Shaikh F, Amatruda JF, Covens A, Gershenson DM, Frazier AL. Is adjuvant chemotherapy indicated in ovarian immature teratomas? A combined data analysis from the Malignant Germ Cell Tumor International Collaborative. Cancer 2016;122:230-7. [Crossref] [PubMed]
- Jalali A, Alvarez-Iglesias A, Roshan D, Newell J. Visualising statistical models using dynamic nomograms. PLoS One 2019;14:e0225253. [Crossref] [PubMed]
- Park SY. Nomogram: An analogue tool to deliver digital knowledge. J Thorac Cardiovasc Surg 2018;155:1793. [Crossref] [PubMed]