The image quality, amyloid-β detectability, and acquisition time of clinical florbetapir positron emission tomography in Alzheimer’s disease and healthy adults
Introduction
Alzheimer’s disease (AD) is a category of neurodegenerative disease characterized by progressive cognitive impairment in multiple domains that can seriously affect the quality of daily life over time (1). AD has become a global health burden estimated to affect approximately 46 million people worldwide, and the prevalence is projected to double over the next 2 decades with population ageing (2). At present, it is hypothesized that the abnormal metabolism of amyloid-β (Aβ) initiates the pathological cascade leading to AD (3). In the central nervous system (CNS), Aβ is generated by the cleavage of amyloid precursor protein in neurons, which subsequently enters the extracellular fluid and is transported to the cerebrospinal fluid and venous blood (3). More importantly, it is universally acknowledged that Aβ deposition likely begins approximately 20 years before the appearance of symptoms (4,5). Therefore, detecting abnormal Aβ accumulation in the CNS plays an essential role in early diagnosis and intervention.
Aβ-positron emission tomography (PET) has become an important tool to identify Aβ deposition in the brain given its noninvasive and direct visualization advantages. Florbetapir F18 (18F-AV45) is one of the tracers used for Aβ-PET and has been approved by the US and European regulatory bodies for in vivo imaging (6). Additionally, 18F-AV45 shows high reliability and affinity-specific binding to amyloid plaques in general clinical work (7,8). However, exclusively modal Aβ-PET imaging can only provide qualitative information, and the binary diagnosis (positive or negative Aβ deposition) basically depends on visual inspection by nuclear medicine physicians. With the help of magnetic resonance imaging (MRI), especially high-resolution T1-weighted images, the degree of Aβ deposition in regional cortices can be approximately semiquantitative by calculating the standardized uptake value ratio (SUVR) with reference to the cerebellar cortex (9).
Notably, the scanning time for 18F-AV45 brain PET imaging currently ranges from 10 to 20 minutes (10-12). Although a relatively short scanning time may increase the image noise and adversely affect the diagnostic accuracy, a relatively long duration could cause discomfort and slow down the clinical workflow. More importantly, patients with severe AD likely cannot keep their heads still in continuous scanning due to impaired cognitive function with/without psychiatric symptoms. Therefore, it is worthwhile to explore a proper scanning duration to achieve both discomfort reduction and good image quality for diagnosis. With the help of a deep learning-based denoising algorithm, the scanning duration could be reduced to 5 minutes with both acceptable image quality and good diagnostic accuracy (13). However, in general clinical work, the potential shortest duration has not been reported thus far.
In this study, we aimed to investigate the image quality and Aβ detectability of 18F-AV45 cranial PET with a short acquisition time in clinical work. To achieve this goal, we enrolled both AD patients and healthy participants and performed a PET/MRI examination. The scanning duration of PET imaging was 15 minutes, and the obtained data were subsequently reconstructed into 15-, 10-, 8-, 6-, 4-, 2-, and 1-minute images as different groups (G15, G10, G8, G6, G4, G2, and G1). We hypothesized that, compared with the 15-minute group, a shorter scanning time could maintain image quality with good Aβ detectability. We present this article in accordance with the STROBE reporting checklist (14) (available at https://qims.amegroups.com/article/view/10.21037/qims-23-268/rc).
Methods
Participants
This cross-sectional study was conducted in accordance with the Declaration of Helsinki (as revised in 2013). The study was approved by the Ethics Committee of West China Hospital of Sichuan University (No. 2021-1056), and written informed consent was obtained from all participants or their proxies before their participation in the study. The inclusion criteria for patients were as follows: (I) impaired cognitive function (15) and (II) positive 18F-AV45 PET images (16). The following exclusion criteria were employed: (I) non-AD neurological disease; (II) systemic illnesses that could interfere with cognition; and (III) general PET/MRI contraindications. Healthy adults were recruited according to the following criteria: (I) Mini-Mental State Examination (MMSE) score ≥27/30; (II) normal neuropsychological assessment; (III) negative 18F-AV45 PET images; and (IV) no PET/MRI contraindications. In total, 29 patients and 13 healthy adults were included and underwent structured interviews and cranial PET/MRI scanning.
PET/MRI examination
To ensure the segmentation accuracy of PET images, we performed a high-resolution structural MRI protocol instead of computed tomography (CT). Each participant received an injection of 18F-AV45 according to body weight (3.7 MBq/kg). At 40 minutes after injection, simultaneous integrated cranial PET/MRI was performed using the GE 3T scanner (SIGNA PET/MR; GE Healthcare, Chicago, IL, USA). The acquisition time of emission images was 15 minutes, and PET images were reconstructed into 15-, 10-, 8-, 6-, 4-, 2-, and 1-minute. All PET images were reconstructed using an ordered subset expectation maximization algorithm with the following parameters: time-of-flight (ToF) modelling, 5 iterations, 28 subsets, matrix =192×192, slice thickness =2.8 mm, field of view (FOV) =30 cm, and filter cut-off =4 mm. Corrections were applied for attenuation and scatter. The whole-brain T1-weighted 3-dimensional (3D) BRAVO (BRAin VOlume) sequence was implemented using the following parameters: repetition time (TR) =8 ms, echo time (TE) =3 ms, FOV =24×24, slice thickness =1 mm, and flip angle =12°. For each case in the 7 groups, visual inspection was first performed on PET and PET/MRI fusion images to verify the absence of suspicious head motion or other artifacts by 2 independent reviewers.
Image quality analysis
The subjective PET image assessment was performed independently by 2 experienced nuclear radiologists. Based on the 5-point Likert scale (17), a score of 1 was given to images with nondiagnostic quality or excessive noise; a score of 2 was given to images with tolerable quality or suboptimal noise; a score of 3 was given to images with average quality for diagnosis in our center; a score of 4 was given to images with quality that was superior to the average; and a score of 5 was given to images with excellent quality. A senior nuclear medicine physician was consulted to unify the scores from the 2 radiologists performing the assessment for the comparison between different time groups. To minimize bias, the participant information was hidden, and the reading order of the images was randomized.
The objective PET image quality was quantified using the signal-to-noise ratio (SNR) value, which was defined as the uniformity of the region of interest (ROI) and calculated as SNR = mean/standard deviation (SD) (18). The quality assessment was performed by 2 experienced technicians as described in the PET/MRI Image Quality Control (GE Healthcare) procedure. Briefly, a 3D circular ROI (Figure 1) was placed on the center of the left cerebellar hemisphere, and the volume of the ROI was maintained at 1 cm3 (19). All ROIs were placed on G15 images and copied-and-pasted to other groups to ensure that the location and size of the ROIs were identical in all groups. We recorded the mean standardized uptake value (SUV) values of the ROIs to obtain the SNR values.
Aβ detectability
The CortexID Suite (GE Healthcare) is an approved tool for clinical use in quantitatively evaluating the deposition of Aβ in brain ROIs (20,21). We utilized it to calculate the SUVR values using the cerebellar grey matter as a reference region in 15 conventional brain regions, including the bilateral prefrontal, anterior cingulate, posterior cingulate/precuneus, sensorimotor, parietal, temporal, occipital, and composite cortices (20,22,23). Then, we compared the SUVR values of each region between G15 and the other groups (G1 to G10). We also calculated the ΔSUVR values between the G15 and other groups to describe the effects of scanning time on SUVR values (24). Finally, we used the obtained SUVR value of the composite cortex in the G15 group as a reference to calculate the sensitivity, specificity, positive predictive value (PPV), and negative predictive value (NPV) in the G1 to G10 groups to further analyze the differences between G15 and other groups and to make a diagnosis and evaluate the diagnostic accuracy based on the SUVR threshold for each group.
Visual inspection was conducted by 2 nuclear physicians in a joint session who were required to give a binary diagnosis (positive or negative Aβ deposition). To reduce potential bias, the reading order was randomized, and the participant information of all images was hidden. To evaluate the diagnostic accuracy of visual inspection, we calculated the sensitivity, specificity, NPV, and PPV in the G1 to G10 groups. The diagnostic results in the G15 group were considered the gold standard for each case. We also provided 2 cases [an AD patient and a healthy control (HC)] in different groups showing 18F-AV45 uptake in the brain (Figure 2A). Notably, the cases exhibiting excessive background noise or poor image quality that hindered a definitive diagnosis were labelled as undefined and subsequently excluded from the analysis of diagnostic accuracy.
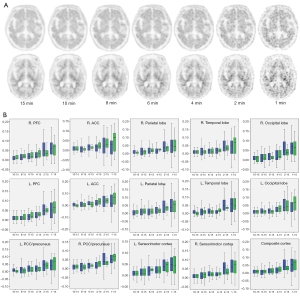
Statistical analysis
Statistical analysis was performed in SPSS 22.0 (IBM Corp., Armonk, NY, USA). The Kruskal-Wallis rank sum test and Dunn’s post-hoc test for multiple comparisons were used to compare the subjective image quality scores and SNR values between the groups in all, AD, and HC participants (17). A statistical description of the SUVR of each group was presented as the mean ± SD. The paired t-test was adopted to compare the SUVR between the groups. A threshold of α<0.05 was considered statistically significant.
Results
Participant characteristics
In total, 29 AD patients and 13 HCs were enrolled in this study. The mean age and MMSE score were 63.76±10.36 years and 15.52±4.55 in the AD group and 58.31±5.69 years and 29.08±0.76 in the HC group. The injected 18F-AV45 doses were 216.04±37.37 and 251.20±42.45 MBq, respectively. The details of the patient characteristics are listed in Table 1.
Table 1
Characteristics | Alzheimer’s disease | Healthy control |
---|---|---|
Total | 29 | 13 |
Male/female | 12/17 | 4/9 |
Age (years) | 63.76±10.36 | 58.31±5.69 |
Weight (kg) | 55.90±9.96 | 63.31±10.37 |
BMI (kg/m2) | 21.52±2.49 | 23.81±2.33 |
MMSE score | 15.52±4.55 | 29.08±0.76 |
Injected dose (MBq) | 216.04±37.37 | 251.20±42.45 |
Injected dose/weight (MBq/kg) | 3.87±0.21 | 3.97±0.30 |
Data are presented as number or mean ± standard deviation. BMI, body mass index; MMSE, Mini-Mental State Examination.
Image quality
The subjective quality scores and SNR values were significantly decreased in the G1 to G10 groups compared to the G15 group (all P<0.05). For both AD patients and HCs, a downwards trend of subjective scores and SNR values was observed from G15 to G1. Notably, the subjective quality score in the G6 group exceeded 3 points (average image quality for diagnosis). The detailed results are listed in Table 2.
Table 2
Participant | Image quality | Group | ||||||
---|---|---|---|---|---|---|---|---|
15 min | 10 min | 8 min | 6 min | 4 min | 2 min | 1 min | ||
Total | Subjective scores | 4.67±0.48 | 4.40±0.63 | 3.86±0.68 | 3.14±0.52 | 2.60±0.50 | 1.67±0.48 | 1.31±0.47 |
SNR values | 11.22±2.61 | 10.06±2.32 | 9.23±2.13 | 8.61±1.93 | 7.48±1.96 | 5.53±1.08 | 4.00±0.73 | |
AD | Subjective scores | 4.66±0.48 | 4.48±0.63 | 3.97±0.73 | 3.21±0.56 | 2.69±0.47 | 1.72±0.45 | 1.41±0.50 |
SNR values | 11.05±2.79 | 9.84±2.44 | 8.96±2.12 | 8.38±1.85 | 7.22±1.85 | 5.43±1.12 | 4.00±0.72 | |
HC | Subjective scores | 4.69±0.48 | 4.23±0.60 | 3.62±0.51 | 3.00±0.41 | 2.38±0.51 | 1.54±0.52 | 1.07±0.28 |
SNR values | 11.62±2.19 | 10.54±2.02 | 9.83±2.12 | 9.12±2.10 | 8.08±2.13 | 5.75±1.01 | 3.98±0.77 |
Data are presented as mean ± standard deviation. All P values of subjective scores and SNR values were <0.05 compared with the group 15 min. SNR, signal-to-noise ratio; AD, Alzheimer’s disease; HC, healthy control.
Aβ detectability
SUVR quantitative analysis
Using the cerebellum as the reference region, the SUVR values of 15 regions were obtained, the details of which are listed in Table 3. For both AD patients and HCs, the majority of the SUVR values significantly increased as the scan time was reduced (P<0.05). The differences in SUVR (ΔSUVR) between G10 and G15, G8 and G15, G6 and G15, G4 and G15, G2 and G15, and G1 and G15 (noted as 10–15, 8–15, 6–15, 4–15, 2–15, and 1–15, respectively) are plotted in Figure 2B. The values of ΔSUVR were also increased, and the deviation expanded as the scan time decreased.
Table 3
Type | Brain regions | Group | ||||||
---|---|---|---|---|---|---|---|---|
15 min | 10 min | 8 min | 6 min | 4 min | 2 min | 1 min | ||
AD | Composite cortex | 1.48±0.16 | 1.50±0.16* | 1.50±0.17* | 1.51±0.18* | 1.52±0.22* | 1.56±0.26* | 1.58±0.30* |
R. prefrontal cortex | 1.45±0.18 | 1.47±0.19* | 1.47±0.19* | 1.48±0.19* | 1.50±0.24* | 1.53±0.29* | 1.55±0.32* | |
L. prefrontal cortex | 1.46±0.18 | 1.47±0.19* | 1.48±0.20* | 1.49±0.20* | 1.50±0.25* | 1.54±0.31* | 1.56±0.34* | |
R. ACC | 1.44±0.20 | 1.45±0.20* | 1.46±0.21* | 1.47±0.21* | 1.48±0.27* | 1.50±0.32* | 1.52±0.36* | |
L. ACC | 1.55±0.20 | 1.56±0.20* | 1.56±0.21* | 1.57±0.21* | 1.59±0.26* | 1.61±0.31* | 1.62±0.35* | |
R. PCC/precuneus | 1.55±0.21 | 1.56±0.21 | 1.56±0.22* | 1.57±0.22* | 1.58±0.27* | 1.62±0.33* | 1.64±0.36* | |
L. PCC/precuneus | 1.60±0.22 | 1.62±0.22* | 1.62±0.23* | 1.63±0.24* | 1.64±0.29* | 1.68±0.35* | 1.70±0.39* | |
R. sensorimotor cortex | 1.35±0.15 | 1.37±0.15* | 1.37±0.16* | 1.39±0.17* | 1.39±0.20* | 1.43±0.25* | 1.45±0.29* | |
L. sensorimotor cortex | 1.35±0.16 | 1.37±0.17* | 1.38±0.18* | 1.38±0.18* | 1.40±0.21* | 1.43±0.25* | 1.44±0.28* | |
R. parietal lobe | 1.47±0.16 | 1.48±0.17* | 1.49±0.17* | 1.49±0.17* | 1.50±0.20* | 1.53±0.24* | 1.55±0.26* | |
L. parietal lobe | 1.41±0.15 | 1.43±0.16* | 1.43±0.17* | 1.44±0.18* | 1.45±0.21* | 1.48±0.25* | 1.50±0.28* | |
R. temporal lobe | 1.52±0.15 | 1.54±0.14* | 1.54±0.15* | 1.55±0.15* | 1.56±0.18* | 1.59±0.22* | 1.61±0.24* | |
L. temporal lobe | 1.48±0.14 | 1.50±0.15* | 1.50±0.16* | 1.51±0.16* | 1.52±0.19* | 1.56±0.22* | 1.58±0.26* | |
R. occipital lobe | 1.49±0.15 | 1.51±0.16* | 1.49±0.15* | 1.52±0.16* | 1.53±0.19* | 1.57±0.25* | 1.58±0.26* | |
L. occipital lobe | 1.53±0.18 | 1.54±0.19* | 1.55±0.19* | 1.56±0.20* | 1.57±0.23* | 1.60±0.27* | 1.61±0.29* | |
HC | Composite cortex | 1.13±0.09 | 1.14±0.09 | 1.14±0.09 | 1.14±0.09* | 1.16±0.09* | 1.18±0.12* | 1.20±0.13* |
R. prefrontal cortex | 1.07±0.10 | 1.08±0.10 | 1.08±0.09* | 1.09±0.10* | 1.10±0.10* | 1.13±0.12* | 1.14±0.14* | |
L. prefrontal cortex | 1.06±0.12 | 1.07±0.12 | 1.07±0.11* | 1.08±0.12* | 1.09±0.12* | 1.12±0.140* | 1.14±0.15* | |
R. ACC | 1.04±0.08 | 1.04±0.08 | 1.04±0.08 | 1.05±0.08 | 1.06±0.08* | 1.08±0.09* | 1.10±0.10* | |
L. ACC | 1.13±0.10 | 1.14±0.10 | 1.14±0.09 | 1.15±0.09 | 1.17±0.09* | 1.20±0.12* | 1.22±0.15* | |
R. PCC/precuneus | 1.09±0.10 | 1.10±0.11 | 1.10±0.11 | 1.10±0.11* | 1.12±0.11* | 1.15±0.13* | 1.17±0.55* | |
L. PCC/precuneus | 1.15±0.12 | 1.16±0.13 | 1.16±0.12 | 0.17±0.12 | 1.18±0.12* | 1.21±0.14* | 1.22±0.15* | |
R. sensorimotor cortex | 1.13±0.10 | 1.14±0.11 | 1.14±0.10 | 1.15±0.10* | 1.16±0.11* | 1.19±0.13* | 1.21±0.14* | |
L. sensorimotor cortex | 1.11±0.10 | 1.12±0.10 | 1.12±0.97 | 1.13±0.09* | 1.14±0.10* | 1.17±0.12* | 1.17±0.13* | |
R. parietal lobe | 1.15±0.09 | 1.16±0.10* | 1.16±0.09* | 1.17±0.09* | 1.18±0.10* | 1.20±0.12* | 1.22±0.14* | |
L. parietal lobe | 1.09±0.11 | 1.10±0.11 | 1.10±0.10 | 1.10±0.11* | 1.11±0.11* | 1.14±0.13* | 1.16±0.14* | |
R. temporal lobe | 1.24±0.08 | 1.25±0.08 | 1.25±0.08 | 1.26±0.08* | 1.27±0.08* | 1.30±0.12* | 1.32±0.13* | |
L. temporal lobe | 1.22±0.08 | 1.22±0.09 | 1.23±0.08 | 1.23±0.08* | 1.24±0.08* | 1.27±0.12* | 1.29±0.13* | |
R. occipital lobe | 1.22±0.09 | 1.23±0.09 | 1.24±0.09 | 1.24±0.10* | 1.25±0.10* | 1.29±0.13* | 1.31±0.15* | |
L. occipital lobe | 1.26±0.09 | 1.27±0.08 | 1.27±0.08* | 1.27±0.08* | 1.29±0.09* | 1.32±0.11* | 1.34±0.13* |
Data are presented as mean ± standard deviation. *, P<0.05 compared with the 15-min group. SUVR, standardized uptake value ratio; AD, Alzheimer’s disease; R., right; L., left; ACC, anterior cingulate cortex; PCC, posterior cingulate cortex; HC, healthy control.
In G15, the maximum SUVR values for AD patients and HCs were 1.42–2.20 and 1.15–1.41, respectively. According to the SUVR values obtained from the G15 data, an SUVR threshold of 1.41 gave the best separation of AD patients and HCs. The sensitivity and specificity of each group were evaluated based on an SUVR threshold of 1.41, and the results are listed in Table 4. The diagnostic accuracy could be maintained at 100% until G6. Impressively, a sensitivity of 97% and specificity of 70% could be achieved in G1.
Table 4
Project | Groups | Pooled number | Undefined number | Sensitivity (%) | Specificity (%) | PPV (%) | NPV (%) |
---|---|---|---|---|---|---|---|
Threshold of SUVR =1.41 | 15 min | 42 | 0 | Ref | Ref | Ref | Ref |
10 min | 42 | 0 | 100 | 100 | 100 | 100 | |
8 min | 42 | 0 | 100 | 100 | 100 | 100 | |
6 min | 42 | 0 | 100 | 100 | 100 | 100 | |
4 min | 42 | 0 | 97 | 92 | 97 | 92 | |
2 min | 42 | 0 | 93 | 70 | 87 | 82 | |
1 min | 42 | 0 | 97 | 70 | 88 | 90 | |
Visual inspection | 15 min | 42 | 0 | Ref | Ref | Ref | Ref |
10 min | 42 | 0 | 100 | 100 | 100 | 100 | |
8 min | 42 | 0 | 100 | 100 | 100 | 100 | |
6 min | 42 | 0 | 100 | 100 | 100 | 100 | |
4 min | 41 | 1 | 100 | 100 | 100 | 100 | |
2 min | 31 | 11 | 82 | 78 | 90 | 64 | |
1 min | 22 | 20 | 69 | 67 | 85 | 44 |
Aβ, amyloid-β; PET, positron emission tomography; PPV, positive predictive value; NPV, negative predictive value; SUVR, standardized uptake value ratio; Ref, reference.
Visual qualitative analysis
Using the diagnostic results in the G15 group as the standard, the sensitivity and specificity of other groups were obtained based on visual (Table 4). Poor sensitivity and specificity could be observed in G1 and G2. Undefined cases existed in G1, G2, and G4. When the scan time exceeded 6 minutes (in G6, G8, G10, and G15), all cases were included in the analysis of diagnostic accuracy and showed satisfactory sensitivity and specificity.
Discussion
Reducing the clinical scanning duration of PET imaging has been a research hotspot in recent years. For 18F-AV45 PET, our results showed that acceptable subjective image quality could be achieved in the G6 group (image quality scores, 3.14±0.52), and good image quality scores were attained in the G10 and G15 groups (image quality scores, 4.40±0.63 and 4.67±0.48, respectively). Additionally, although the image quality scores and SNR were decreased with a shorter acquisition time, the diagnostic accuracy of both visual inspection and quantitative analysis could be maintained up to the G6 group. These findings suggested that the shortest duration of 6-minute cranial 18F-AV45 PET imaging might achieve a balance between short-term scanning and acceptable image quality and Aβ detectability.
The injected dose is an important factor that needs to be considered in the pursuit of optimal image quality. For 18F-AV45 PET, various injection doses have been reported in recent studies. Generally, 40 MBq·min/kg and a 10-minute acquisition of PET imaging were adopted, with good imaging quality (25). Several researchers used an intravenous bolus of approximately 370 MBq of 18F-AV45 for each participant regardless of body weight (7,26). In our study, a dose of 3.87±0.21 MBq/kg was used for AD patients, 3.97±0.30 MBq/kg for HCs, and 3.90±0.24 MBq/kg for all cases. After multiplying by 15-, 10-, 8-, 6-, and 4-minute acquisition times, the values became 59.55, 39.70, 31.76, 22.82, and 15.88 MBq·min/kg, respectively, which was in accordance with or lower than that noted in the previous study. This result suggested that the injection dose of 18F-AV45 could be reduced, which could help to reduce the effective radiation dose for patients.
SUVR is valuable for studies and clinical trials in AD. However, it is resource intensive, requiring coregistered MRI data and specialized segmentation software. Research has revealed that deep learning algorithms can estimate SUVR and use this information for diagnosis (9). Such software can enable quantitative measurements rapidly and in settings without extensive image processing manpower and expertise. In our study, similar image processing software provided by the GE workstation CortexID Suite was used to obtain SUVR, enabling us to obtain SUVR data for each group which helped to reduce image processing manpower and maintain consistency.
The clinical evaluation of amyloid PET imaging employs visual assessment for binary classification, which has been validated to be approximately 90% accurate (27,28). Notably, visual assessment can be influenced by the readers’ experience, and some cases are classified as equivocal when amyloid deposition is focal or at an early stage. Therefore, incorporating adjunct quantitative measures of Aβ deposition by calculating the SUVR value can provide valuable clinical benefits for diagnosis. Currently, cerebellar grey matter usually serves as the reference region, and the SUVR threshold ranges from 1.10 to 1.22 in AV45 PET (29-31). Other studies also followed an SUVR threshold of 0.79 based on the cerebral white matter reference region (32-34). In this study, we used a threshold of SUVR =1.41 and obtained the maintained diagnostic accuracy until the G6 group. While achieving a robust SUVR cut-off value remains an ongoing topic, future studies should consider several potential factors affecting the thresholds, such as sample size, population variability, image acquisition, and data analysis techniques.
When the scan time exceeded 6 minutes, 100% diagnostic accuracy could be achieved for both visual inspection and the SUVR threshold method. As the scan time decreased, the sensitivity and specificity showed a slight reduction in both quantitative and qualitative analyses. Our results indicated that the deviation of ΔSUVR increased from G10-G15 to G1-G15, resulting in a slight reduction in sensitivity and specificity based on the SUVR threshold. Based on quantitative categorization based on an SUVR threshold of 1.41, sensitivity and specificity values of 97% and 92%, respectively, could be achieved with a 4-minute acquisition time. Acceptable sensitivity and specificity values of 97% and 70%, respectively, could be obtained with a 1-minute acquisition time. However, the sensitivity and specificity were considerably reduced in the qualitative categorization based on visual characteristics when the acquisition time was less than 4 minutes (sensitivity of 69% and specificity of 67% for 1-minute acquisition, sensitivity of 82% and specificity of 78% for 2-minute acquisition). In addition, 11 and 20 cases were marked as undefined for 1- and 2-minute acquisition, respectively. We consider that the high noise and poor image quality affect the visual characteristics and result in a low diagnostic accuracy. However, the accuracy of the quantitative method based on SUVR obtained from CortexID Suite software was minimally affected. These results may reveal that if the acquisition time is less than 4 minutes, the quantitative method based on SUVR showed a better diagnostic accuracy, and qualitative visual diagnosis showed a better accuracy in the opposite site.
Limitations
Several limitations exist in our study. First, the sample size was relatively small. To determine the specific minimum scan time, further research involving large multicenter samples is needed. Second, we used a relatively simple method to segment the whole-brain cortex, which might have affected the accuracy of SUVR values. Although the tool is sufficient for heavy clinical work, future studies should consider a more reliable tool for segmentation. Third, in this study, we set the 15-minute group as a gold standard reference. In the future, we suggest that dynamic imaging might better reveal the timepoint with the shortest scanning duration and excellent imaging quality.
Conclusions
The PET scanning duration of clinical cranial 18F-AV45 PET can be significantly reduced while maintaining image quality and diagnostic performance in both AD patients and HCs. In terms of image quality, a more than 6-minute acquisition time can obtain PET images with good subjective evaluation and SNR values. In terms of Aβ detectability, a 10-minute acquisition time in the AD group and a 6-minute acquisition time in the HC group can contribute to statically different SUVR values. Importantly, these SUVR differences might not affect diagnostic accuracy because the sensitivity, specificity, PPV, and NPV were all maintained using both visual inspection and the SUVR threshold of 1.41. In summary, our results suggest that a 6-minute acquisition time of AV45 PET could be sufficient for clinical practice, which can reduce the burden on nuclear medicine physicians and shorten patient waiting time.
Acknowledgments
Funding: This study was supported by
Footnote
Reporting Checklist: The authors have completed the STROBE reporting checklist. Available at https://qims.amegroups.com/article/view/10.21037/qims-23-268/rc
Conflicts of Interest: All authors have completed the ICMJE uniform disclosure form (available at https://qims.amegroups.com/article/view/10.21037/qims-23-268/coif). ZJ reports that this study was supported by the National Natural Science Foundation of China (Nos. 82271947 and 81971595), the CAMS Innovation Fund for Medical Sciences (CIFMS; No. 2022-I2M-C&T-B-104), and the Key R&D Support Plan of Chengdu Science and Technology Bureau (No. 2022-YF05-01766-SN). RT reports that this study was supported by the National Key R&D Program of China (No. 2022YFC2009902/2022YFC2009900). The other authors have no conflicts of interest to declare.
Ethical Statement: The authors are accountable for all aspects of the work in ensuring that questions related to the accuracy or integrity of any part of the work are appropriately investigated and resolved. The study was conducted in accordance with the Declaration of Helsinki (as revised in 2013). The study was approved by the Ethics Committee of West China Hospital of Sichuan University (Reference No. 2021-1056). Written informed consent was provided by all participants or their proxies before their participations in the study.
Open Access Statement: This is an Open Access article distributed in accordance with the Creative Commons Attribution-NonCommercial-NoDerivs 4.0 International License (CC BY-NC-ND 4.0), which permits the non-commercial replication and distribution of the article with the strict proviso that no changes or edits are made and the original work is properly cited (including links to both the formal publication through the relevant DOI and the license). See: https://creativecommons.org/licenses/by-nc-nd/4.0/.
References
- Scheltens P, De Strooper B, Kivipelto M, Holstege H, Chételat G, Teunissen CE, Cummings J, van der Flier WM. Alzheimer's disease. Lancet 2021;397:1577-90. [Crossref] [PubMed]
- Steele NZ, Carr JS, Bonham LW, Geier EG, Damotte V, Miller ZA, Desikan RS, Boehme KL, Mukherjee S, Crane PK, Kauwe JS, Kramer JH, Miller BL, Coppola G, Hollenbach JA, Huang Y, Yokoyama JS. Fine-mapping of the human leukocyte antigen locus as a risk factor for Alzheimer disease: A case-control study. PLoS Med 2017;14:e1002272. [Crossref] [PubMed]
- Roberts KF, Elbert DL, Kasten TP, Patterson BW, Sigurdson WC, Connors RE, Ovod V, Munsell LY, Mawuenyega KG, Miller-Thomas MM, Moran CJ, Cross DT 3rd, Derdeyn CP, Bateman RJ. Amyloid-β efflux from the central nervous system into the plasma. Ann Neurol 2014;76:837-44. [Crossref] [PubMed]
- Bateman RJ, Xiong C, Benzinger TL, Fagan AM, Goate A, Fox NC, et al. Clinical and biomarker changes in dominantly inherited Alzheimer's disease. N Engl J Med 2012;367:795-804. [Crossref] [PubMed]
- Villemagne VL, Pike KE, Chételat G, Ellis KA, Mulligan RS, Bourgeat P, Ackermann U, Jones G, Szoeke C, Salvado O, Martins R, O'Keefe G, Mathis CA, Klunk WE, Ames D, Masters CL, Rowe CC. Longitudinal assessment of Aβ and cognition in aging and Alzheimer disease. Ann Neurol 2011;69:181-92. [Crossref] [PubMed]
- Heurling K, Leuzy A, Zimmer ER, Lubberink M, Nordberg A. Imaging β-amyloid using [(18)F]flutemetamol positron emission tomography: from dosimetry to clinical diagnosis. Eur J Nucl Med Mol Imaging 2016;43:362-73.
- Johnson KA, Sperling RA, Gidicsin CM, Carmasin JS, Maye JE, Coleman RE, Reiman EM, Sabbagh MN, Sadowsky CH, Fleisher AS, Murali Doraiswamy P, Carpenter AP, Clark CM, Joshi AD, Lu M, Grundman M, Mintun MA, Pontecorvo MJ, Skovronsky DM. AV45-A11 study group. Florbetapir (F18-AV-45) PET to assess amyloid burden in Alzheimer's disease dementia, mild cognitive impairment, and normal aging. Alzheimers Dement 2013;9:S72-83. [Crossref] [PubMed]
- Camus V, Payoux P, Barré L, Desgranges B, Voisin T, Tauber C, et al. Using PET with 18F-AV-45 (florbetapir) to quantify brain amyloid load in a clinical environment. Eur J Nucl Med Mol Imaging 2012;39:621-31. [Crossref] [PubMed]
- Reith F, Koran ME, Davidzon G, Zaharchuk G. Alzheimer′s Disease Neuroimaging Initiative. Application of Deep Learning to Predict Standardized Uptake Value Ratio and Amyloid Status on (18)F-Florbetapir PET Using ADNI Data. AJNR Am J Neuroradiol 2020;41:980-6. [Crossref] [PubMed]
- Kolanko MA, Win Z, Loreto F, Patel N, Carswell C, Gontsarova A, Perry RJ, Malhotra PA. Amyloid PET imaging in clinical practice. Pract Neurol 2020;20:451-62. [Crossref] [PubMed]
- Wu KY, Liu CY, Chen CS, Chen CH, Hsiao IT, Hsieh CJ, Lee CP, Yen TC, Lin KJ. Beta-amyloid deposition and cognitive function in patients with major depressive disorder with different subtypes of mild cognitive impairment: (18)F-florbetapir (AV-45/Amyvid) PET study. Eur J Nucl Med Mol Imaging 2016;43:1067-76. [Crossref] [PubMed]
- Lilamand M, Cesari M, del Campo N, Cantet C, Soto M, Ousset PJ, Payoux P, Andrieu S, Vellas BMAPT Study Group. Brain Amyloid Deposition Is Associated With Lower Instrumental Activities of Daily Living Abilities in Older Adults. Results From the MAPT Study. J Gerontol A Biol Sci Med Sci 2016;71:391-7. [Crossref] [PubMed]
- Peng Z, Ni M, Shan H, Lu Y, Li Y, Zhang Y, Pei X, Chen Z, Xie Q, Wang S, Xu XG. Feasibility evaluation of PET scan-time reduction for diagnosing amyloid-β levels in Alzheimer's disease patients using a deep-learning-based denoising algorithm. Comput Biol Med 2021;138:104919. [Crossref] [PubMed]
- von Elm E, Altman DG, Egger M, Pocock SJ, Gøtzsche PC, Vandenbroucke JP. STROBE Initiative. The Strengthening the Reporting of Observational Studies in Epidemiology (STROBE) statement: guidelines for reporting observational studies. PLoS Med 2007;4:e296. [Crossref] [PubMed]
- Dubois B, Feldman HH, Jacova C, Hampel H, Molinuevo JL, Blennow K, et al. Advancing research diagnostic criteria for Alzheimer's disease: the IWG-2 criteria. Lancet Neurol 2014;13:614-29. [Crossref] [PubMed]
- Grothe MJ, Ewers M, Krause B, Heinsen H, Teipel SJAlzheimer's Disease Neuroimaging Initiative. Basal forebrain atrophy and cortical amyloid deposition in nondemented elderly subjects. Alzheimers Dement 2014;10:S344-53. [Crossref] [PubMed]
- Zhang YQ, Hu PC, Wu RZ, Gu YS, Chen SG, Yu HJ, Wang XQ, Song J, Shi HC. The image quality, lesion detectability, and acquisition time of (18)F-FDG total-body PET/CT in oncological patients. Eur J Nucl Med Mol Imaging 2020;47:2507-15. [Crossref] [PubMed]
- Wagatsuma K, Miwa K, Kamitaka Y, Koike E, Yamao T, Yoshii T, Kobayashi R, Nezu S, Sugamata Y, Miyaji N, Imabayashi E, Ishibashi K, Toyohara J, Ishii K. Determination of optimal regularization factor in Bayesian penalized likelihood reconstruction of brain PET images using [18 F]FDG and [11 C]PiB. Med Phys 2022;49:2995-3005.
- Shao X, Xu M, Qiu C, Niu R, Wang Y, Wang X. Application of siemens SMART neuro attenuation correction in brain PET imaging. Medicine (Baltimore) 2018;97:e12502. [Crossref] [PubMed]
- Imabayashi E, Tamamura N, Yamaguchi Y, Kamitaka Y, Sakata M, Ishii K. Automated semi-quantitative amyloid PET analysis technique without MR images for Alzheimer's disease. Ann Nucl Med 2022;36:865-75. [Crossref] [PubMed]
- Pemberton HG, Buckley C, Battle M, Bollack A, Patel V, Tomova P, Cooke D, Balhorn W, Hegedorn K, Lilja J, Brand C, Farrar G. Software compatibility analysis for quantitative measures of [18F]flutemetamol amyloid PET burden in mild cognitive impairment. EJNMMI Res 2023;13:48.
- Bucci M, Savitcheva I, Farrar G, Salvadó G, Collij L, Doré V, Gispert JD, Gunn R, Hanseeuw B, Hansson O, Shekari M, Lhommel R, Molinuevo JL, Rowe C, Sur C, Whittington A, Buckley C, Nordberg A. A multisite analysis of the concordance between visual image interpretation and quantitative analysis of [18F]flutemetamol amyloid PET images. Eur J Nucl Med Mol Imaging 2021;48:2183-99.
- Müller EG, Stokke C, Stokmo HL, Edwin TH, Knapskog AB, Revheim ME. Evaluation of semi-quantitative measures of (18)F-flutemetamol PET for the clinical diagnosis of Alzheimer's disease. Quant Imaging Med Surg 2022;12:493-509. [Crossref] [PubMed]
- Murray ME, Lowe VJ, Graff-Radford NR, Liesinger AM, Cannon A, Przybelski SA, Rawal B, Parisi JE, Petersen RC, Kantarci K, Ross OA, Duara R, Knopman DS, Jack CR Jr, Dickson DW. Clinicopathologic and 11C-Pittsburgh compound B implications of Thal amyloid phase across the Alzheimer's disease spectrum. Brain 2015;138:1370-81. [Crossref] [PubMed]
- Vanhoutte M, Landeau B, Sherif S, de la Sayette V, Dautricourt S, Abbas A, Manrique A, Chocat A, Chételat G. Evaluation of the early-phase [18F]AV45 PET as an optimal surrogate of [18F]FDG PET in ageing and Alzheimer's clinical syndrome. Neuroimage Clin 2021;31:102750.
- Brendel M, Sauerbeck J, Greven S, Kotz S, Scheiwein F, Blautzik J, Delker A, Pogarell O, Ishii K, Bartenstein P, Rominger AAlzheimer’s Disease Neuroimaging Initiative. Serotonin Selective Reuptake Inhibitor Treatment Improves Cognition and Grey Matter Atrophy but not Amyloid Burden During Two-Year Follow-Up in Mild Cognitive Impairment and Alzheimer's Disease Patients with Depressive Symptoms. J Alzheimers Dis 2018;65:793-806. [Crossref] [PubMed]
- Salloway S, Gamez JE, Singh U, Sadowsky CH, Villena T, Sabbagh MN, et al. Performance of [18F]flutemetamol amyloid imaging against the neuritic plaque component of CERAD and the current (2012) NIA-AA recommendations for the neuropathologic diagnosis of Alzheimer's disease. Alzheimers Dement (Amst) 2017;9:25-34.
- Clark CM, Pontecorvo MJ, Beach TG, Bedell BJ, Coleman RE, Doraiswamy PM, Fleisher AS, Reiman EM, Sabbagh MN, Sadowsky CH, Schneider JA, Arora A, Carpenter AP, Flitter ML, Joshi AD, Krautkramer MJ, Lu M, Mintun MA, Skovronsky DM. AV-45-A16 Study Group. Cerebral PET with florbetapir compared with neuropathology at autopsy for detection of neuritic amyloid-β plaques: a prospective cohort study. Lancet Neurol 2012;11:669-78. [Crossref] [PubMed]
- Sevigny J, Suhy J, Chiao P, Chen T, Klein G, Purcell D, Oh J, Verma A, Sampat M, Barakos J. Amyloid PET Screening for Enrichment of Early-Stage Alzheimer Disease Clinical Trials: Experience in a Phase 1b Clinical Trial. Alzheimer Dis Assoc Disord 2016;30:1-7. [Crossref] [PubMed]
- Fakhry-Darian D, Patel NH, Khan S, Barwick T, Svensson W, Khan S, Perry RJ, Malhotra P, Carswell CJ, Nijran KS, Win Z. Optimisation and usefulness of quantitative analysis of (18)F-florbetapir PET. Br J Radiol 2019;92:20181020. [Crossref] [PubMed]
- Sato C, Barthélemy NR, Mawuenyega KG, Patterson BW, Gordon BA, Jockel-Balsarotti J, Sullivan M, Crisp MJ, Kasten T, Kirmess KM, Kanaan NM, Yarasheski KE, Baker-Nigh A, Benzinger TLS, Miller TM, Karch CM, Bateman RJ. Tau Kinetics in Neurons and the Human Central Nervous System. Neuron 2018;97:1284-1298.e7. [Crossref] [PubMed]
- Reith FH, Mormino EC, Zaharchuk G. Predicting future amyloid biomarkers in dementia patients with machine learning to improve clinical trial patient selection. Alzheimers Dement (N Y) 2021;7:e12212. [Crossref] [PubMed]
- Landau SM, Fero A, Baker SL, Koeppe R, Mintun M, Chen K, Reiman EM, Jagust WJ. Measurement of longitudinal β-amyloid change with 18F-florbetapir PET and standardized uptake value ratios. J Nucl Med 2015;56:567-74. [Crossref] [PubMed]
- Chen K, Roontiva A, Thiyyagura P, Lee W, Liu X, Ayutyanont N, Protas H, Luo JL, Bauer R, Reschke C, Bandy D, Koeppe RA, Fleisher AS, Caselli RJ, Landau S, Jagust WJ, Weiner MW, Reiman EMAlzheimer’s Disease Neuroimaging Initiative. Improved power for characterizing longitudinal amyloid-β PET changes and evaluating amyloid-modifying treatments with a cerebral white matter reference region. J Nucl Med 2015;56:560-6. [Crossref] [PubMed]