Computed tomography angiography-based radiomics model to identify high-risk carotid plaques
Introduction
Stroke is the third leading cause of death and disability worldwide (1), and extracranial atherosclerosis is one of the major causes of stroke, contributing to 15–20% of ischemic stroke events (2,3). Traditionally, the assessment of the severity of carotid plaques (atherosclerosis) has been determined by the quantification of the degree of vascular stenosis, however, a growing number of studies have shown that ischemic stroke caused by high-risk plaques is not only related to the degree of vascular stenosis but also closely related to plaque components (4-7). Meanwhile, vascular inflammation plays a major role in the development of atherosclerosis (8,9), and the relationship between the vessel wall and perivascular adipose tissue (PVAT) is complex. A previous study reported that inflammation associated with high-risk carotid plaques can be identified by changes in PVAT density on computed tomographic (CT) images (10).
Carotid computed tomography angiography (CTA) is a widely used imaging modality that allows detailed assessments of plaque characteristics closely associated with ischemic stroke, such as plaque ulceration (11), plaque thickness (12), and remodeling index (13). Compared with the traditional manual measurement of plaque features on CT images, radiomics is a process that rapidly extracts massive quantifiable features from medical images, transforming digital medical images into extractable, high-dimensional data that can provide information indistinguishable to the naked eye (14,15). Radiomics model based on magnetic resonance imaging (MRI) has yielded good results in identifying high-risk carotid plaques (16). Meanwhile, Huang et al. achieved a high accuracy in identifying symptomatic carotid plaques by using clinical features and ultrasound radiomic features to construct a nomogram (17). Although carotid CTA exposes patients to ionizing radiation, it has advantages of rapidity, simplicity and low cost compared with MRI, and less dependence of operator and variability of inter-observer than ultrasound (5). Recently, Dong et al. (18) showed that CTA-based radiomics model can accurately identify symptomatic carotid plaques, but their study only included carotid plaques with >50% stenosis, whereas carotid plaques with 30–50% stenosis can be a cause of ischemic strokes of unknown origin (19).
This study aimed to develop and test radiomics models of carotid plaques with >30% stenosis and PVAT to improve the accuracy of identifying high-risk carotid plaques and compare the diagnostic value between radiomics models and traditional CTA model built by plaque features, which would result in distinguishing symptomatic from asymptomatic plaques. We present this article in accordance with the TRIPOD reporting checklist (available at https://qims.amegroups.com/article/view/10.21037/qims-23-158/rc).
Methods
Study patients
We consecutively screened neck CTA examinations at Affiliated Hospital of North Sichuan Medical College, China, from May 2016 to December 2021, to identify patients with extracranial carotid plaques. Atherosclerotic plaques are defined as thickening of the vessel wall or calcification of the vessel wall (11).
Inclusion criteria were as follows: (I) a diagnosis of extracranial carotid stenosis between 30% and 99% on CTA images based on North American Symptomatic Carotid Endarterectomy Trial (NASCET) criteria (20); (II) sufficient information of ascertaining cerebral ischemia symptoms in the clinical medical record; (III) adequate information regarding vascular risk factors in the clinical medical record.
Exclusion criteria were as follows (16): (I) cardiogenic stroke; (II) simultaneous bilateral anterior circulation events; (III) carotid dissection, aneurysm, primary intracranial disease, carotid stenosis caused by radiation therapy and vasculitis; (IV) stroke involving only the posterior circulation; and (V) poor image quality.
We eventually included 144 patients and randomly divided them into a training group (n=100) and a validation group (n=44) at a ratio of 7:3. This retrospective cohort study was conducted in accordance with the Declaration of Helsinki (as revised in 2013). The study was approved by the Institutional Review Board of Affiliated Hospital of North Sichuan Medical College (file number 2022ER377-1), and individual consent for this retrospective analysis was waived.
Classification of cerebral ischemia symptoms
In our study, patients were classified into symptomatic and asymptomatic groups according to whether they had acute ischemic stroke or transient ischemic attack (TIA) in the cerebral anterior circulation region within two weeks before CTA examination, which was diagnosed by the doctor (WT) who had no knowledge of carotid plaque features (16). Meanwhile, symptomatic patients with >50% carotid stenosis were considered as large-artery atherosclerosis type (21) and symptomatic patients with 30–50% carotid stenosis were considered as stroke of undetermined source (19) by combining clinical and relevant examination. Only one atherosclerotic carotid artery was included in each patient for the study. For the patients in the symptomatic group, we included the atherosclerotic carotid artery ipsilateral to the symptom (if the patient had an acute ischemic stroke in the anterior circulation of the right cerebral hemisphere, the right atherosclerotic carotid artery was included), and for the patients in the asymptomatic group, if the bilateral atherosclerotic carotid artery fulfilled the inclusion and exclusion criteria, only the side of the atherosclerotic carotid artery with more severe stenosis was included (22). Acute ischemic stroke is defined as neurological deficits lasting for more than 24 hours or neurological deficits with acute cerebral infarct manifestations on imaging (23). TIA is defined as focal arterial ischemia lasting for less than 24 hours without pathological or imaging evidence of infarction (23), and it may manifest as transient visual field loss, unilateral sensory deficits, or mild hemiparesis. Images of a patient with acute ischemic stroke are shown in Figure 1.
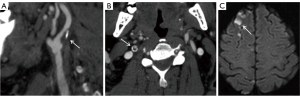
CTA techniques
All examinations were conducted on a 64-row CT scanner (SOMATOM Definition AS+, Siemens, Germany) at our institution. The scanning range covered from the aortic arch to the skull apex. A 60–80 mL volume of iodine contrast media was injected at a speed of 4.0–5.0 mL/s. The acquisition was triggered by using embolic tracking 2 seconds after an attenuation threshold of regions of interest (ROIs) of aortic arch at the level of the tracheal bifurcation reaching 100 Hounsfield unit (HU). The following were the scanning parameters: tube voltage, 100 kV; tube current, 300 mA; matrix, 512×512; field of view, 280 mm; slice thickness, 0.6 mm; slice interval, 0.5 mm.
Clinical information
Personal information and vascular risk factors of patients were collected from the clinical medical record, which included sex, age, diabetes (hemoglobin A1c >6.5% or taking glucose-lowering medication), hypertension (blood pressure >140/90 mmHg or taking antihypertensive medication), hyperlipidemia (low-density lipoprotein >100 mg/dL or taking lipid-lowering medication), smoking history [current smoking or stopped smoking within the last 3 months (10)] and coronary artery disease (presence of old myocardial infarction or after coronary stenting or definite diagnosis by coronary angiography).
Traditional CTA plaque analysis
Two radiologists with 4 years of experience in neuroradiology and no knowledge of patient information (WX and ZL) reconstructed the carotid artery on the picture archiving and communication system (PACS) (INFINITT Healthcare, Korea) by multi-planar reformation and measured the following radiological features of carotid plaques (traditional CTA features) in training and validation groups: (I) degree of stenosis (NASCET criteria) (20); (II) plaque type: if the mean attenuation of plaques is ≥130 HU and the volume is ≥50% of the total volume of plaques, it is considered calcified, otherwise, it is considered a noncalcified plaque (18); (III) total plaque thickness: the maximum plaque thickness on the CTA axis; (IV) calcified plaque thickness: the maximum thickness of calcified components of plaques on the CTA axis; (V) soft plaque thickness: the maximum thickness of noncalcified components of plaques on the CTA axis; (VI) total length of the plaque: the maximum longitudinal length of the plaque; (VII) plaque ulceration: Extension of the contrast media by >1.5 mm beyond the lumen of the vessel (24); (VIII) plaque enhancement: the threshold of plaque enhancement >10 HU after contrast injection (25). (IX) Remodeling index: (area of the vessel at the maximum stenosis of carotid plaques/area of the distal vessel unaffected by plaques) × 100% (26); (X) plaque burden: (1 − lumen area at the maximum stenosis/vessel area at the maximum stenosis) × 100% (16). Continuous variables were the average of the values measured by two radiologists (WX and ZL), including the degree of stenosis, plaque length, total plaque thickness, soft plaque thickness, calcified plaque thickness, plaque burden and remodeling index. Categorical variables, including plaque ulceration, plaque type and plaque enhancement, were agreed upon by the two radiologists (WX and ZL) when there was a disagreement. Radiological features of carotid plaques are shown in Figure S1.
Image segmentation, feature extraction, selection and model building
In this study, software dedicated to radiomics (3D Slicer) was used as the analysis platform, the 3D Slicer was a free and open source software package for image analysis. The ROIs, which included plaques and PVAT, were drawn at the level of the maximum plaque area in the arterial phase on CTA of which the two radiologists jointly determined before segmentation (16,18). PVAT was described as adipose tissue in the radial distance from the outer wall of vessel equal to the vessel diameter, and its Hounsfield unit (HU) value varied from −190 to −30 HU (27), so PVAT was drawn semiautomatically by setting an attenuation threshold. The ROIs of drawing are shown in Figure 2.
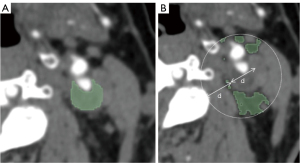
To reduce image heterogeneity, we resampled all CTA images to homogeneity (1 mm × 1 mm × 1 mm), used a fixed bin width of 25 HU, and added Laplacian of Gaussian (kernel sizes: 0.5, 1, 1.5, and 2) and Wavelet. Meanwhile, seven types of radiomics features were extracted, including shape, first order, gray-level co-occurrence matrix (GLCM), gray-level dependence matrix (GLDM), gray-level size-zone matrix (GLSZM), gray-level run-length matrix (GLRLM) and neighborhood gray-tone difference matrix (NGTDM), and we preprocessed the extracted data with z score normalization.
To avoid dimensional catastrophe and reduce bias of radiomic features, the best features were selected in the training group. First, we used independent samples t-test or Mann-Whitney U-test to filter out features with significant differences (P<0.05) according to whether the data met normal distribution. Then, we applied the least absolute shrinkage and selection operator (LASSO), in which the minimum variance was used to automatically regulate the regularization parameter (λ), and 10-fold cross-validation was performed for feature selection to reduce the dimensionality and select the best features (28). Finally, the selected optimal radiomics features were applied to build models.
The best radiomics features selected from the training groups of plaques and PVAT were used to construct radiomics models by a support vector machine (SVM) (29). To select the best model, we optimized the kernel size parameter {γ, gamma, [0.001,1]} and the regularization parameter {C, cost, [1, 1000]} of the SVM kernel function by 10-fold cross validation to select the best performing parameter. Finally, the best radiomics features were tested by SVM in the validation group. The receiver operating characteristic (ROC) curve and area under the curve (AUC) were applied to assess the predictive value of radiomics models. The classification accuracy, sensitivity, specificity, positive predictive value (PPV) and negative predictive value (NPV) were also calculated. The study flow chart and radiomics workflow are illustrated in Figures 3,4, respectively.
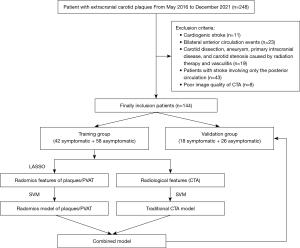
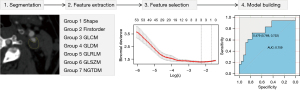
Intraobserver and interobserver agreement of radiomics features
Two radiologists with no knowledge of patient information (NY and CL) randomly sampled 50 patients and drew ROIs, including carotid plaques and PVAT. To assess intraobserver agreement, Observer 1 used the same method to perform two repeated drawings of the ROIs and extract radiomic features for the same patient within one week. To assess the interobserver agreement, observer 2 performed one drawing of the ROIs and feature extraction and then compared the extracted features with the results obtained by observer 1 the first time. The reproducibility of intra- and interobserver was assessed by intraclass correlation coefficient (ICC). ICC greater than 0.75 represented good agreement.
Statistical analysis
All statistical analyses were conducted by Statistical Product Service Solutions (SPSS) (Version 23.0, IBM) and R software (version 4.2.1). Means and standard deviations (SD) were used to record continuous variables, and frequencies and percentages were used for categorical variables. Each continuous variable was tested for normality by Shapiro-Wilk test, and the t-test was applied to the comparisons of continuous variables conforming to a normal distribution, otherwise, the Mann-Whitney u-test was used. The χ2 test was applied to the comparisons of categorical variables. We used multivariable logistic regression to analyze variables that were significantly different (P<0.05) in the univariate analysis of traditional CTA features and clinical information. LASSO regression was performed by using the “Glmnet” package, and “e1071” and “pROC” were applied to SVM modeling and ROC curves, respectively. The Delong method was applied to compare the AUC of each model. A P value <0.05 was considered significantly different.
Results
Clinical information
One hundred forty-four patients were enrolled in this study, including 60 symptomatic and 84 asymptomatic patients. Univariate analysis showed that only sex was associated with symptomatic plaques among personal information and vascular risk factors (P<0.05), but sex was not an independent predictor of symptomatic plaques in a multivariable logistic regression analysis (P>0.05). The clinical information is listed in Table 1. Meanwhile, the clinical information and traditional CTA features in training and validation groups show no statistical difference as displayed in Table 2.
Table 1
Clinical information/CTA features | Total (N=144) | Symptomatic (N=60) | Asymptomatic (N=84) | Univariate, P value | Multivariate | |
---|---|---|---|---|---|---|
OR (95% CI) | P value | |||||
Male | 110 (76.4) | 51 (85.0) | 59 (70.2) | 0.04* | 0.716 (0.249–2.054) | 0.534 |
Age (years) | 70.93±9.10 | 70.22±11.04 | 71.44±7.45 | 0.886 | ||
Smoking | 65 (45.1) | 28 (46.7) | 37 (44.0) | 0.756 | ||
Hypertension | 111 (77.1) | 45 (75.0) | 66 (78.6) | 0.615 | ||
Diabetes mellitus | 52 (36.1) | 18 (30.0) | 34 (40.5) | 0.197 | ||
Hyperlipidemia | 69 (47.9) | 30 (50.0) | 39 (46.4) | 0.672 | ||
Coronary artery disease | 40 (27.8) | 13 (21.7) | 27 (32.1) | 0.166 | ||
Degree of stenosis (%) | 63.69±18.75 | 68.29±20.19 | 60.39±17.02 | 0.017* | 1.016 (0.986–1.047) | 0.300 |
Calcified plaque | 39 (27.1) | 11 (18.3) | 28 (33.3) | 0.046* | 0.749 (0.204–2.743) | 0.662 |
Plaque ulceration | 26 (18.1) | 19 (31.7) | 7 (8.3) | <0.001* | 4.867 (1.718–13.791) | 0.003* |
Plaque length (mm) | 15.22±6.37 | 16.27±7.40 | 14.47±5.45 | 0.174 | ||
Total plaque thickness (mm) | 4.48±1.19 | 4.64±1.24 | 4.37±1.15 | 0.182 | ||
Soft plaque thickness (mm) | 3.74±1.71 | 4.10±1.61 | 3.48±1.74 | 0.032* | 0.901 (0.650–1.250) | 0.532 |
Calcified plaque thickness (mm) | 1.80±1.25 | 1.48±1.08 | 2.03±1.32 | 0.016* | 0.665 (0.427–1.036) | 0.071 |
Plaque burden (%) | 81.86±10.59 | 84.05±10.14 | 80.30±10.69 | 0.021* | 0.527 (0.002–133.526) | 0.821 |
Remodeling index | 2.50±0.71 | 2.68±0.67 | 2.36±0.72 | 0.008* | 2.143 (1.175–3.910) | 0.013* |
Plaque enhancement | 55 (38.2) | 27 (45.0) | 28 (33.3) | 0.155 |
Categorical variables shown with frequency and percentage; continuous variables shown with mean ± standard deviation (SD); *, P<0.05. CTA, computed tomography angiography; OR, odds ratio; CI, confidence interval.
Table 2
Clinical information/CTA features | Training group (N=100) | Validation group (N=44) | Univariate, P value |
---|---|---|---|
Male | 77 (77.0) | 33 (75.0) | 0.795 |
Age (years) | 70.21±11.19 | 71.41±8.90 | 0.682 |
Smoking | 44 (44.0) | 21 (47.7) | 0.679 |
Hypertension | 76 (76.0) | 35 (79.5) | 0.641 |
Diabetes mellitus | 38 (38.0) | 14 (31.8) | 0.477 |
Hyperlipidemia | 47 (47.0) | 22 (50.0) | 0.740 |
Coronary artery disease | 27 (27.0) | 13 (29.5) | 0.753 |
Degree of stenosis (%) | 63.55±18.48 | 64.44±19.52 | 0.733 |
Calcified plaque | 29 (29.0) | 10 (22.7) | 0.435 |
Plaque ulceration | 16 (16.0) | 10 (22.7) | 0.334 |
Plaque length (mm) | 14.94±5.60 | 16.13±7.73 | 0.615 |
Total plaque thickness (mm) | 4.49±1.16 | 4.53±1.20 | 0.741 |
Soft plaque thickness (mm) | 3.68±1.66 | 3.92±1.80 | 0.393 |
Calcified plaque thickness (mm) | 1.84±1.29 | 1.71±1.17 | 0.638 |
Plaque burden (%) | 81.76±10.66 | 82.55±10.10 | 0.709 |
Remodeling index | 2.48±0.72 | 2.56±0.70 | 0.501 |
Plaque enhancement | 37 (37.0) | 18 (40.9) | 0.657 |
Categorical variables shown with frequency and percentage; continuous variables shown with mean ± standard deviation. CTA, computed tomography angiography.
Assessment of carotid plaques on CTA
Univariate analysis showed that the degree of stenosis, calcified plaque thickness, soft plaque thickness, plaque burden, remodeling index, plaque type (calcified plaque), and plaque ulceration were associated with symptomatic plaques (P<0.05, Table 1). Multivariable logistic regression analysis demonstrated that plaque ulceration (OR =4.867; 95% CI: 1.718–13.791) and remodeling index (OR =2.143, 95% CI: 1.175–3.910) were independent predictors of symptomatic plaques. A traditional CTA model combining plaque ulceration and remodeling index was built by an SVM, in which the AUC values of training and validation groups were 0.624 and 0.624 (with an optimal γ value of 0.1 and C value of 10), respectively. Diagnostic value ability of traditional CTA model is listed in Table 3.
Table 3
Model | AUC (95% CI) | Accuracy, % | Sensitivity, % | Specificity, % | PPV, % | NPV, % |
---|---|---|---|---|---|---|
Training group | ||||||
Traditional CTA model | 0.624 (0.506–0.743) | 67.0 | 28.6 | 94.8 | 80.0 | 64.7 |
Plaque radiomics model | 0.766 (0.666–0.865) | 72.0 | 45.2 | 91.4 | 79.2 | 69.7 |
PVAT radiomics model | 0.740 (0.644–0.835) | 66.0 | 47.6 | 79.3 | 62.5 | 67.6 |
Plaque + PVAT radiomics model | 0.863 (0.792–0.934) | 78.0 | 59.5 | 91.4 | 83.3 | 75.7 |
Plaque + PVAT + Traditional CTA model | 0.883 (0.815–0.952) | 82.0 | 69.0 | 91.4 | 85.3 | 80.3 |
Validation group | ||||||
Traditional CTA model | 0.624 (0.431–0.817) | 70.5 | 38.9 | 92.3 | 77.8 | 68.6 |
Plaque radiomics model | 0.759 (0.606–0.911) | 75.0 | 55.6 | 88.5 | 76.9 | 74.2 |
PVAT radiomics model | 0.618 (0.440–0.794) | 61.3 | 22.2 | 88.5 | 57.1 | 62.2 |
Plaque + PVAT radiomics model | 0.784 (0.632–0.936) | 72.7 | 50.0 | 88.5 | 75.0 | 71.9 |
Plaque + PVAT + Traditional CTA model | 0.840 (0.710–0.970) | 79.5 | 66.7 | 88.5 | 80.0 | 79.3 |
AUC, area under curve; 95% CI, 95% confidence interval; PPV, positive predictive value; NPV, negative predictive value; CTA, computed tomography angiography; PVAT, perivascular adipose tissue.
Reproducibility of radiomics features and traditional CTA features
For the plaque radiomics features, 383 features with ICCs less than 0.75 were excluded, of which there were 335 features of intraobserver differences and 360 features of interobserver differences.
For the PVAT radiomics features, 519 features with ICCs less than 0.75 were excluded, of which 310 features showed intraobserver differences and 464 features showed interobserver differences.
The interclass correlation coefficients (ICCs) of the traditional CTA features for measuring the continuous variables, including degree of stenosis, plaque length, total plaque thickness, calcified plaque thickness, soft plaque thickness, plaque burden and remodeling index, were 0.842, 0.805, 0.827, 0.845, 0.803, 0.785 and 0.810, respectively. The Cohen’s kappa values of determining categorical variables including plaque ulceration, plaque type and plaque enhancement were 0.855, 0.818, 0.772, respectively.
Selection of radiomics features
For the plaque radiomics features, we extracted 851 radiomics features from ROI. There were 468 radiomics features after excluding features of intraobserver and interobserver differences. The next step was to remove the non-significant values, 29 features conformed to normal distribution, in which 18 features (P<0.05) were significantly different by t-test. 439 features did not conform to normal distribution, in which 389 features (P<0.05) were significantly different by Mann-Whitney u-test, so 407 features (P<0.05) were used to the LASSO algorithm. Finally, 3 radiomic features were retained (with the λ value of 0.103), and used to build plaque radiomics model in training group and test in validation group by SVM.
For the PVAT radiomics features, we extracted 851 radiomics features from ROI. There were 332 radiomics features after excluding features of intraobserver and interobserver differences. The next step was to remove the non-significant values, 52 features conformed to normal distribution, in which 1 feature (P<0.05) were significantly different by t-test. 280 features did not conform to normal distribution, in which 3 features (P<0.05) were significantly different by Mann-Whitney u-test, so 4 features (P<0.05) were used to the LASSO algorithm. Finally, all 4 radiomics features were retained (with the λ value of 0.015), and used to build PVAT radiomics model in training group and test in validation group by SVM.
Building models
Three plaque radiomics features and four PVAT radiomics features (Table S1) were used to build carotid plaques and PVAT radiomics models, respectively.
In the training and validation groups, the AUC values of plaque radiomics features were 0.766 and 0.759 with an optimal γ value of 0.001and C value of 10 (Table 3), which were significantly different in the training group (P=0.046) but not in the validation group (P=0.297) when plaque radiomics model was compared with the traditional CTA model (Figure 5). The AUC values of PVAT radiomics features were 0.740 and 0.618 in the training and validation groups (with an optimal γ value of 0.001 and C value of 1000), respectively, and there was no significant difference in the training (P=0.118) and validation groups (P=0.953) when PVAT radiomics model was compared with the traditional CTA model (Figure 6). When the model (Plaque + PVAT radiomics model) was constructed by combining the radiomics features of plaques and PVAT, the AUC values were 0.863 and 0.784 in the training and validation groups (with an optimal γ value of 0.01 and C value of 1), respectively, which were significantly different in the training group (P<0.001) but not in the validation group (P=0.182) when it was compared with the traditional CTA model (Figure 7).
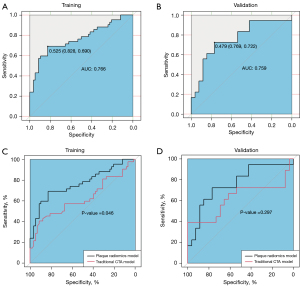
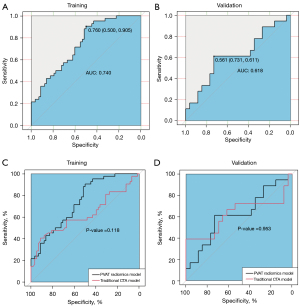
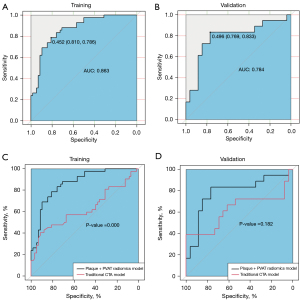
Finally, we combined plaque radiomics features, PVAT radiomics features, and traditional CTA features to build a new combinational model (Plaque + PVAT + Traditional CTA model) in which the AUC values were 0.883 and 0.840 in the training and validation groups (with an optimal γ value of 0.01 and C value of 10), respectively, and the ROC curves of the new combinational model were significantly better than those of the traditional CTA model in the training group (P<0.001) and validation group (P=0.029) (Figure 8).
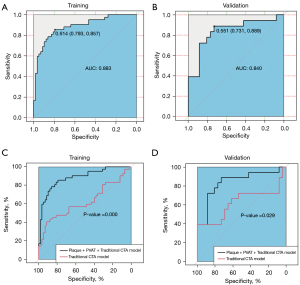
Discussion
In this study, we developed plaque radiomics features, PVAT radiomics features, and traditional CTA features to build models distinguishing symptomatic from asymptomatic carotid plaques. All models showed diagnostic values in the assessment of symptomatic carotid plaques, in which there were no significant differences when radiomics models were compared with traditional CTA model in the validation group. However, the combined model (Plaque + PVAT + Traditional CTA model) had a good diagnostic performance to identify symptomatic carotid plaques. Distinguishing between symptomatic and asymptomatic carotid plaques is important for the treatment of carotid plaques, and different management methods can be chosen for symptomatic and asymptomatic patients (30). Our finding contributes to setting up an effective treatment plan for patients with carotid plaques.
Carotid CTA can assess plaque features closely associated with cerebral ischemia symptoms by geometric parameters (25). Our study evaluated the correlation of CTA plaque features and clinical risk factors with cerebral ischemic symptoms and finally found that plaque ulceration and remodeling index were independent predictors of cerebral ischemic symptoms. Plaque ulceration, which CTA can accurately identify, is an important predictor of ischemic stroke (11,25). The sensitivity and specificity of CTA for examining plaque ulceration reached 94% and 99%, respectively (31), and plaque ulceration increased the risk of ipsilateral cerebral ischemia events by 2.2 times (32). Arterial remodeling is known to be involved in the development of atherosclerosis. The degree of expansive remodeling can indicate potential high-risk plaques, and symptomatic carotid plaques can show a greater degree of expansive remodeling (13,26), which is consistent with the results of this study. In our study, the percentage of plaque ulceration and the mean value of remodeling index in the symptomatic group were higher than those in the asymptomatic group, and the traditional CTA model consisting of plaque ulceration and remodeling index had a diagnostic value (AUC =0.624) for symptomatic carotid plaques.
Nowadays, the robustness of radiomics features is a major concern. In this study, we used the preprocessing method of resampling to maintain the stability of radiomics features, intra- and inter-observer reliability tests (ICC >0.75) to ensure the repeatability of features, and univariate analysis and LASSO to ensure the independence and importance of each feature, as well as 10-fold cross-validation to keep the robustness of features. Meanwhile, seven radiomics features were extracted from plaques and PVAT, which were Wavelet–LHL–GLCM–Joint Entropy, Wavelet–HLL–GLCM–Joint Energy, Wavelet–LLL–GLCM–MCC, Original–GLSZM–Size Zone Non-Uniformity, Wavelet–LHH–GLSZM–Small Area Emphasis, Wavelet–LHH–GLDM–Dependence Variance and Wavelet–LLL–GLCM–Sum Squares. Joint Entropy measures the randomness and variability of neighborhood intensity values. Energy measures homogeneous patterns of image. Size Zone Non-Uniformity measures the variability of the volume of size area on image, with lower values indicating a more uniform size area volume. Small Area Emphasis measures the distribution of small size areas, with larger values indicating smaller areas and finer textures. Dependence Variance measures the variance of dependence size of image. Sum Squares measures average intensity level in the distribution of adjacent intensity level pairs.
The combination of radiomics and machine learning has become a common approach. Zhang et al. (16) used radiomics and machine learning to identify symptomatic carotid plaque with the AUC values of 0.988 and 0.984 for training and validation group, the performance of their model is better than our plaque radiomics model, which may be caused by the difference between MRI and CTA. Meanwhile, Dong et al. (18) found that the CTA plaque radiomics model was better at differentiating symptomatic carotid plaques than the conventional CTA plaque model. In our study, there was no significant difference between the plaque radiomics model (AUC =0.759) and traditional CTA model (AUC =0.624) in validation groups; however, there was an upward trend in the diagnostic performance of the plaque radiomics model. Our results were different from those of Dong et al. (18), which may be caused by the inclusion of carotid plaques with 30–50% stenosis, which is gradually being recognized as an important factor in strokes of unknown etiology (33,34). The application of deep learning (DL) in carotid plaques is emerging (35). Saba et al. (36) used DL to classify and characterize carotid plaques based on ultrasound, showing the development potential of DL in carotid plaques. To our knowledge, there are no studies that have applied DL to identify high-risk carotid plaque on CT images. Combining DL and radiomics to identify high-risk carotid plaques based on CT may be a worth explored direction.
Vascular inflammation is a key component of the atherosclerotic process (8), and vascular inflammation induces transcriptional and structural changes to PVAT (37). These changes may be reflected by the increase in the HU values of PVAT on CT images, which is closely associated with intraplaque hemorrhage and cerebral ischemic symptoms (10,27). To our knowledge, there are no studies about the relationship between carotid PVAT radiomics and cerebral ischemic symptoms. In this study, the PVAT radiomics model showed diagnostic value but was not significantly different from the traditional CTA model. We think that this result can be brought about by the limited radiomics features that were extracted at a single level because the PVAT of carotid is relatively small and scattered. Currently, the application of artificial intelligence in pericardial adipose tissue has been shown to be a feasible approach (38), so it is necessary to combine radiomics and artificial intelligence to automatically evaluate PVAT as a marker of vascular and plaque inflammation in future studies.
Radiomics is not the only determinant of making clinical decisions, and radiomics data combined with other relevant data can produce reliable and accurate clinical decision support systems (39). Chen et al. (40) and Huang et al. (17) used radiomics and relevant clinical features to identify symptomatic carotid plaques by MRI and ultrasound, respectively. Although the combined models of their studies performed better than our combined model, similar to their study, the combined model (Plaque + PVAT + Traditional CTA model) performed better than the single relevant model (traditional CTA model), and radiomics features contribute to differentiating symptomatic from asymptomatic carotid plaques in this study. In our study, the AUC of a single factor with 0.624 (traditional CTA model) was raised to that of the combined model with 0.840 (Plaque + PVAT + Traditional CTA model). We think that the radiomics features of carotid plaques and PVAT together provide a good gaining effect in the combinational model. Furthermore, radiomics features of plaques and PVAT and traditional CTA features are complementary to each other in the identification of high-risk carotid plaques.
This study has some limitations. First, the study is a retrospective small sample study, so future prospective studies are needed to evaluate carotid plaques. Second, the ROIs in this study were manually segmented or semiautomatically segmented; full automatic segmentation can improve outline accuracy and reduce intraobserver and interobserver differences. Third, this is a single-centre study, and it is necessary to study the effects of different centres with different population and scanners on radiomics features of plaque and PVAT in the future. Finally, due to the large number of CTA images of carotid plaques and limited time, we performed radiomics analysis only at the level with the largest plaque area. The 3D segmentation method was not used, and some valid radiomics features may have been lost.
Conclusions
Radiomic features of plaques and PVAT and traditional CTA features are useful for distinguishing symptomatic from asymptomatic plaques. Furthermore, the combined model with three types of features significantly contributes to identifying high-risk carotid plaques compared with traditional CTA model.
Acknowledgments
Funding: This work was supported by grants from
Footnote
Reporting Checklist: The authors have completed the TRIPOD reporting checklist. Available at https://qims.amegroups.com/article/view/10.21037/qims-23-158/rc
Conflicts of Interest: All authors have completed the ICMJE uniform disclosure form (available at https://qims.amegroups.com/article/view/10.21037/qims-23-158/coif). All authors report that this work was supported by grants from North Sichuan Medical College (No. CBY21-PT01) and Affiliated Hospital of North Sichuan Medical College (No. 2022JB001). The authors have no other conflicts of interest to declare.
Ethical Statement: The authors are accountable for all aspects of the work in ensuring that questions related to the accuracy or integrity of any part of the work are appropriately investigated and resolved. This retrospective cohort study was conducted in accordance with the Declaration of Helsinki (as revised in 2013). The study was approved by the Institutional Review Board of Affiliated Hospital of North Sichuan Medical College (file number 2022ER377-1), and individual consent for this retrospective analysis was waived.
Open Access Statement: This is an Open Access article distributed in accordance with the Creative Commons Attribution-NonCommercial-NoDerivs 4.0 International License (CC BY-NC-ND 4.0), which permits the non-commercial replication and distribution of the article with the strict proviso that no changes or edits are made and the original work is properly cited (including links to both the formal publication through the relevant DOI and the license). See: https://creativecommons.org/licenses/by-nc-nd/4.0/.
References
- Global, regional, and national burden of stroke and its risk factors, 1990-2019: a systematic analysis for the Global Burden of Disease Study 2019. Lancet Neurol 2021;20:795-820. [Crossref] [PubMed]
- Sacco RL, Kargman DE, Gu Q, Zamanillo MC. Race-ethnicity and determinants of intracranial atherosclerotic cerebral infarction. The Northern Manhattan Stroke Study. Stroke 1995;26:14-20. [Crossref] [PubMed]
- Wityk RJ, Lehman D, Klag M, Coresh J, Ahn H, Litt B. Race and sex differences in the distribution of cerebral atherosclerosis. Stroke 1996;27:1974-80. [Crossref] [PubMed]
- Millon A, Mathevet JL, Boussel L, Faries PL, Fayad ZA, Douek PC, Feugier P. High-resolution magnetic resonance imaging of carotid atherosclerosis identifies vulnerable carotid plaques. J Vasc Surg 2013;57:1046-1051.e2. [Crossref] [PubMed]
- Brinjikji W, Huston J 3rd, Rabinstein AA, Kim GM, Lerman A, Lanzino G. Contemporary carotid imaging: from degree of stenosis to plaque vulnerability. J Neurosurg 2016;124:27-42. [Crossref] [PubMed]
- Yamada K, Kawasaki M, Yoshimura S, Shirakawa M, Uchida K, Shindo S, Nishida S, Iwamoto Y, Nakahara S, Sato Y. High-Intensity Signal in Carotid Plaque on Routine 3D-TOF-MRA Is a Risk Factor of Ischemic Stroke. Cerebrovasc Dis 2016;41:13-8. [Crossref] [PubMed]
- Schindler A, Schinner R, Altaf N, Hosseini AA, Simpson RJ, Esposito-Bauer L, Singh N, Kwee RM, Kurosaki Y, Yamagata S, Yoshida K, Miyamoto S, Maggisano R, Moody AR, Poppert H, Kooi ME, Auer DP, Bonati LH, Saam T. Prediction of Stroke Risk by Detection of Hemorrhage in Carotid Plaques: Meta-Analysis of Individual Patient Data. JACC Cardiovasc Imaging 2020;13:395-406. [Crossref] [PubMed]
- Ross R. Atherosclerosis--an inflammatory disease. N Engl J Med 1999;340:115-26. [Crossref] [PubMed]
- Chen X, Dang Y, Hu H, Ma S, Ma Y, Wang K, Liu T, Lu X, Hou Y. Pericoronary adipose tissue attenuation assessed by dual-layer spectral detector computed tomography is a sensitive imaging marker of high-risk plaques. Quant Imaging Med Surg 2021;11:2093-103. [Crossref] [PubMed]
- Baradaran H, Myneni PK, Patel P, Askin G, Gialdini G, Al-Dasuqi K, Kamel H, Gupta A. Association Between Carotid Artery Perivascular Fat Density and Cerebrovascular Ischemic Events. J Am Heart Assoc 2018;7:e010383. [Crossref] [PubMed]
- Homburg PJ, Rozie S, van Gils MJ, van den Bouwhuijsen QJ, Niessen WJ, Dippel DW, van der Lugt A. Association between carotid artery plaque ulceration and plaque composition evaluated with multidetector CT angiography. Stroke 2011;42:367-72. [Crossref] [PubMed]
- Gupta A, Baradaran H, Kamel H, Pandya A, Mangla A, Dunning A, Marshall RS, Sanelli PC. Evaluation of computed tomography angiography plaque thickness measurements in high-grade carotid artery stenosis. Stroke 2014;45:740-5. [Crossref] [PubMed]
- Hardie AD, Kramer CM, Raghavan P, Baskurt E, Nandalur KR. The impact of expansive arterial remodeling on clinical presentation in carotid artery disease: a multidetector CT angiography study. AJNR Am J Neuroradiol 2007;28:1067-70. [Crossref] [PubMed]
- Gillies RJ, Kinahan PE, Hricak H. Radiomics: Images Are More than Pictures, They Are Data. Radiology 2016;278:563-77. [Crossref] [PubMed]
- Shang J, Guo Y, Ma Y, Hou Y. Cardiac computed tomography radiomics: a narrative review of current status and future directions. Quant Imaging Med Surg 2022;12:3436-53. [Crossref] [PubMed]
- Zhang R, Zhang Q, Ji A, Lv P, Zhang J, Fu C, Lin J. Identification of high-risk carotid plaque with MRI-based radiomics and machine learning. Eur Radiol 2021;31:3116-26. [Crossref] [PubMed]
- Huang Z, Cheng XQ, Liu HY, Bi XJ, Liu YN, Lv WZ, Xiong L, Deng YB. Relation of Carotid Plaque Features Detected with Ultrasonography-Based Radiomics to Clinical Symptoms. Transl Stroke Res 2022;13:970-82. [Crossref] [PubMed]
- Dong Z, Zhou C, Li H, Shi J, Liu J, Liu Q, Su X, Zhang F, Cheng X, Lu G. Radiomics versus Conventional Assessment to Identify Symptomatic Participants at Carotid Computed Tomography Angiography. Cerebrovasc Dis 2022;51:647-54. [Crossref] [PubMed]
- Bulwa Z, Gupta A. Embolic stroke of undetermined source: The role of the nonstenotic carotid plaque. J Neurol Sci 2017;382:49-52. [Crossref] [PubMed]
- North American Symptomatic Carotid Endarterectomy Trial. Methods, patient characteristics, and progress. Stroke 1991;22:711-20. [Crossref] [PubMed]
- Adams HP Jr, Bendixen BH, Kappelle LJ, Biller J, Love BB, Gordon DL, Marsh EE 3rd. Classification of subtype of acute ischemic stroke. Definitions for use in a multicenter clinical trial. TOAST. Trial of Org 10172 in Acute Stroke Treatment. Stroke 1993;24:35-41. [Crossref] [PubMed]
- Saba L, Zucca S, Gupta A, Micheletti G, Suri JS, Balestrieri A, Porcu M, Crivelli P, Lanzino G, Qi Y, Nardi V, Faa G, Montisci R. Perivascular Fat Density and Contrast Plaque Enhancement: Does a Correlation Exist? AJNR Am J Neuroradiol 2020;41:1460-5. [Crossref] [PubMed]
- Sacco RL, Kasner SE, Broderick JP, Caplan LR, Connors JJ, Culebras A, et al. An updated definition of stroke for the 21st century: a statement for healthcare professionals from the American Heart Association/American Stroke Association. Stroke 2013;44:2064-89. [Crossref] [PubMed]
- Knight-Greenfield A, Quitlong Nario JJ, Vora A, Baradaran H, Merkler A, Navi BB, Kamel H, Gupta A. Associations Between Features of Nonstenosing Carotid Plaque on Computed Tomographic Angiography and Ischemic Stroke Subtypes. J Am Heart Assoc 2019;8:e014818. [Crossref] [PubMed]
- Baradaran H, Gupta A. Carotid Vessel Wall Imaging on CTA. AJNR Am J Neuroradiol 2020;41:380-6. [Crossref] [PubMed]
- Fukuda K, Iihara K, Maruyama D, Yamada N, Ishibashi-Ueda H. Relationship between carotid artery remodeling and plaque vulnerability with T1-weighted magnetic resonance imaging. J Stroke Cerebrovasc Dis 2014;23:1462-70. [Crossref] [PubMed]
- Zhang S, Gu H, Yu X, Kang B, Yuan X, Wang X. Association Between Carotid Artery Perivascular Fat Density and Intraplaque Hemorrhage. Front Cardiovasc Med 2021;8:735794. [Crossref] [PubMed]
- Chen Y, Chen TW, Wu CQ, Lin Q, Hu R, Xie CL, Zuo HD, Wu JL, Mu QW, Fu QS, Yang GQ, Zhang XM. Radiomics model of contrast-enhanced computed tomography for predicting the recurrence of acute pancreatitis. Eur Radiol 2019;29:4408-17. [Crossref] [PubMed]
- Lin Q, Ji YF, Chen Y, Sun H, Yang DD, Chen AL, Chen TW, Zhang XM. Radiomics model of contrast-enhanced MRI for early prediction of acute pancreatitis severity. J Magn Reson Imaging 2020;51:397-406. [Crossref] [PubMed]
- Naylor R, Rantner B, Ancetti S, de Borst GJ, De Carlo M, Halliday A, et al. Editor's Choice - European Society for Vascular Surgery (ESVS) 2023 Clinical Practice Guidelines on the Management of Atherosclerotic Carotid and Vertebral Artery Disease. Eur J Vasc Endovasc Surg 2023;65:7-111. [Crossref] [PubMed]
- Saba L, Caddeo G, Sanfilippo R, Montisci R, Mallarini G. Efficacy and sensitivity of axial scans and different reconstruction methods in the study of the ulcerated carotid plaque using multidetector-row CT angiography: comparison with surgical results. AJNR Am J Neuroradiol 2007;28:716-23.
- Baradaran H, Al-Dasuqi K, Knight-Greenfield A, Giambrone A, Delgado D, Ebani EJ, Kamel H, Gupta A. Association between Carotid Plaque Features on CTA and Cerebrovascular Ischemia: A Systematic Review and Meta-Analysis. AJNR Am J Neuroradiol 2017;38:2321-6. [Crossref] [PubMed]
- Gupta A, Gialdini G, Giambrone AE, Lerario MP, Baradaran H, Navi BB, Marshall RS, Iadecola C, Kamel H. Association Between Nonstenosing Carotid Artery Plaque on MR Angiography and Acute Ischemic Stroke. JACC Cardiovasc Imaging 2016;9:1228-9. [Crossref] [PubMed]
- Kamel H, Merkler AE, Iadecola C, Gupta A, Navi BB. Tailoring the Approach to Embolic Stroke of Undetermined Source: A Review. JAMA Neurol 2019;76:855-61. [Crossref] [PubMed]
- Saba L, Sanagala SS, Gupta SK, Koppula VK, Johri AM, Khanna NN, et al. Multimodality carotid plaque tissue characterization and classification in the artificial intelligence paradigm: a narrative review for stroke application. Ann Transl Med 2021;9:1206. [Crossref] [PubMed]
- Saba L, Sanagala SS, Gupta SK, Koppula VK, Johri AM, Sharma AM, Kolluri R, Bhatt DL, Nicolaides A, Suri JS. Ultrasound-based internal carotid artery plaque characterization using deep learning paradigm on a supercomputer: a cardiovascular disease/stroke risk assessment system. Int J Cardiovasc Imaging 2021;37:1511-28. [Crossref] [PubMed]
- Kotanidis CP, Antoniades C. Perivascular fat imaging by computed tomography (CT): a virtual guide. Br J Pharmacol 2021;178:4270-90. [Crossref] [PubMed]
- Greco F, Salgado R, Van Hecke W, Del Buono R, Parizel PM, Mallio CA. Epicardial and pericardial fat analysis on CT images and artificial intelligence: a literature review. Quant Imaging Med Surg 2022;12:2075-89. [Crossref] [PubMed]
- Lambin P, Leijenaar RTH, Deist TM, Peerlings J, de Jong EEC, van Timmeren J, Sanduleanu S, Larue RTHM, Even AJG, Jochems A, van Wijk Y, Woodruff H, van Soest J, Lustberg T, Roelofs E, van Elmpt W, Dekker A, Mottaghy FM, Wildberger JE, Walsh S. Radiomics: the bridge between medical imaging and personalized medicine. Nat Rev Clin Oncol 2017;14:749-62. [Crossref] [PubMed]
- Chen S, Liu C, Chen X, Liu WV, Ma L, Zha Y. A Radiomics Approach to Assess High Risk Carotid Plaques: A Non-invasive Imaging Biomarker, Retrospective Study. Front Neurol 2022;13:788652. [Crossref] [PubMed]