Long-term reproducibility of opportunistically assessed vertebral bone mineral density and texture features in routine clinical multi-detector computed tomography using an automated segmentation framework
Introduction
Bone demineralization and deterioration of the trabecular microarchitecture are the hallmarks of osteoporosis, a potentially debilitating bone disease that leads to substantially decreased bone strength and increased vertebral fracture (VF) risk (1,2). According to the World Health Organization (WHO) criteria, approximately 21% of females aged 50 years and older suffer from osteoporosis (3). Despite being a considerable source of health care cost, the disease remains vastly underdiagnosed (3-5). Commonly, bone mineral density (BMD) measurements for osteoporosis diagnostics are assessed using dual-energy X-ray absorptiometry (DXA) or quantitative computed tomography (QCT) (6-8). However, both methods do not take into account changes in trabecular bone architecture and demand additional radiation exposure and specific scanning setups. Texture analysis (TA) is an image analysis approach that allows assessment of bone microarchitecture in three-dimensional (3D) computed tomography (CT) datasets (7,8). Since texture feature (TF) extraction from aggregate routine clinical CT scans is feasible opportunistically, it promises to overcome both aforementioned limitations of DXA- and QCT-based BMD measurements.
The usefulness of TFs in opportunistic osteoporosis screening and fracture risk assessment as well as fracture classification as measures of bone quality has been shown in previous studies (9-11). However, TA at the spine relies on exact labeling and accurate segmentations of trabecular bone to yield acceptable reproducibility. Recent advances in automated spine segmentation have streamlined this time-consuming process: A recently developed convolutional neural network (CNN)-based framework (https://anduin.bonescreen.de) allows for automated labeling and spine segmentation, asynchronous Hounsfield unit (HU)-to-BMD calibration, and correction for the intravenous contrast medium phase and has demonstrated its efficacy in predicting prevalent VFs and assessing risk for imminent fractures (12-16). Furthermore, Dieckmeyer et al. used this pipeline to establish reference values for TFs in females and males in the mid-thoracic, lower thoracic, and lumbar spine levels (17). Furthermore, the authors identified a set of six features with good scan-rescan reproducibility over a follow-up (FU) interval of up to 77 days.
However, before opportunistic volumetric BMD (vBMD) and TF measurements may be used to classify pathologic changes in the future, reference values and detailed information on reproducibility of measurements derived from a bone-healthy cohort are obligatory. Therefore, this study aimed to investigate TF and vBMD reproducibility over 6 to 26 months, using routine clinical multi-detector computed tomography (MDCT) data and to describe value changes over this time interval.
Methods
Patient selection
The study was conducted in accordance with the Declaration of Helsinki (as revised in 2013). The study was approved by institutional ethics committee of Klinikum rechts der Isar, Technical University of Munich and individual consent for this retrospective analysis was waived.
For this observational study, patients who underwent routine thoraco-abdominal MDCT scans with a single specific scanner at our institution to rule out tumor recurrence were retrospectively identified using our institute’s local picture archiving and communication system (PACS). The patient inclusion interval covered a time period of 6 years from July 2007 to July 2013 and patients included in the cohort had to have received two MDCT scans within 6–26 months. Included scans were manually checked for full coverage of the mid-thoracic to the lumbar spine (T5–L5).
Further, patients with VFs, history of use of bone-active medications (e.g., bisphosphonates), bone metastases, and diagnosis of osteoporosis/osteopenia or any other hematological disease or disease of bone metabolism prior to this study or during the FU interval were excluded. Thereby, we intended to generate a cohort with supposed bone-healthy subjects who were not at risk for pathological bone loss during FU. For quality assurance, scans were screened for severe beam hardening and motion artifacts. If present, these patients were excluded from further analyses.
Image acquisition and automated vertebral body segmentation
All scans were performed using a single MDCT scanner with 64 detector rows (Somatom Sensation Cardiac 64, Siemens Healthcare GmbH, Erlangen, Germany). Oral contrast medium was applied approximately 1 h prior to all scans (Barilux Scan; Sanochemia Diagnostics, Neuss, Germany). Scans were performed 70 s after intravenous administration of an iodine-based contrast agent (Fresenius Pilot C; Fresenius Kabi, Bad Homburg, Germany) with body-weight adjusted dosage (80, 90, and 100 mL for body weight 80, >80 to 100, >100 kg, respectively) and a flow-rate of 3 mL/s. For the dedicated scanning protocol, the average tube voltage and adapted tube load were set at 120 kVp and 200 mAs. Using a standard bone reconstruction kernel, sagittal views of the spine were reformatted for each scan (slice thickness of 3 mm).
Using the sagittal reformations, automated vertebral body segmentation was performed for T5–L5 using a CNN-based pipeline (Figure 1) (https://anduin.bonescreen.de) (12,13,15,18). This readily and freely available tool is based on a deep-learning algorithm and automatically labels and segments vertebrae from CT scans in a multi-step process (12): first, vertebral bodies are registered and annotated using an X-ray-like projection. Second, the tool segments vertebral bodies and separates cortical and trabecular compartments. In the next step, it separately creates subregion segmentation masks for the laminar regions, facet joints, and transverse and spinal processes.
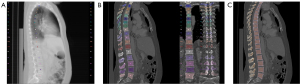
All segmentations were manually checked and corrected by a neuro-radiologist specialized in spine imaging, if necessary, as reported previously, with a correction ration of 1.1% (13,17). Simultaneously, the neuro-radiologist examined the segmented vertebrae for intra-osseous venous malformations and severe Modic-type endplate changes and excluded vertebrae presenting these anomalies.
Mean HU of trabecular bone was extracted from segmentation masks. The MDCT scanner was asynchronously calibrated to yield vBMD in mg/cm3 using a linear HU-to-vBMD-equation with a slope of 0.63 (14). In addition, automated correction of the contrast phase was performed by a two-dimensional anatomy-guided DenseNet model (16).
Extraction of texture features
Following generation of the vertebral segmentation masks, TA was performed using a customized version of a publicly available toolbox (https://github.com/mvallieres/radiomics), run on MatLAB (version R2021a; MathWorks Inc., Natick, MA, USA; RRID:SCR_001622) (19-21). We included TFs that have been shown to be highly reproducible (17). Analyzed TFs were varianceglobal, entropy, short-run emphasis (SRE), long-run emphasis (LRE), run-length non-uniformity (RLN), and run percentage (RP). These TFs were analyzed on the segmented trabecular compartments of the vertebral bodies.
Varianceglobal is a first-order feature calculated by performing a global gray-level histogram-based analysis, and it describes the spread of the gray-level distribution (22). Entropy is a gray-level co-occurrence matrix analysis (GLCM)-based feature of second order that quantifies randomness (23). While many more GLCM-based features have been described, they often exhibit substantial correlation among each other, resulting in redundance, or lack interpretability (17,23). Entropy has shown good reproducibility in the past and allows for a more intuitive interpretation (17). For this reason, and to limit multiple testing bias, we decided to investigate entropy as the only GLCM-based TF. The GLCM describes the occurrence of voxel pairs with the same given gray-level value and offset in an image. Entries of the GLCMs at different angular directions θ = (0°, 45°, 90°, and 135°) were computed as the joint probability of two adjacent voxel intensities at a given offset d = (dx, dy, dz) and given θ, with dx, dy, and dz denoting the displacement along the x-, y-, and z-axis, respectively. 3D GLCM analysis was performed by computing the co-occurrence probabilities of voxel intensities from the 26 neighbors aligned in 13 directions. Averaging over the 13 directions ensures rotational invariance. SRE, LRE, RLN, and RP are considered features of higher order and derived based on the analysis of gray-level run-length matrices (GLRLM) (24). A gray-level run is a set of consecutive voxels with identical gray-level values that are arranged collinearly in a certain direction. The run-length is the number of voxels in a gray-level run. GLRLM features are calculated based on the occurrence and distribution of such runs within the GLCM. These features quantify directional changes in the GLCM. By simultaneously adding up all possible run-lengths in the 13 directions of the 3D space, 3D GLRLMs were obtained. For both GLCM and GLRLM analysis, direction-dependent discretization length differences were taken into account when measurements were combined through averaging or summation.
Statistical analysis
All statistical analyses were performed using STATA v13.0 software (StataCorp LLC, College Station, TX, USA) with a two-sided significance threshold of 0.05. The normal distribution of dependent variables (vBMD and all TFs) was visually checked using histograms. In order to increase the robustness of the measurements and to reduce the number of observations, average values were calculated for the dependent variables and spine compartments by adding all measurements of the respective variable within one spine compartment of a single patient and dividing by the number of measurements. As QCT BMD measurements are typically derived from the lumbar spine, we assigned the lumbar vertebrae to a single compartment (lumbar: L1–L5) (25). Osteoporotic VFs typically occur between the T5 and L5 level, therefore we decided to include this non-superimposed thoracic spine segment, which was then divided into two equally sized compartments (mid-thoracic T5–T8 and lower thoracic T9–T12) (26).
To determine reproducibility, root mean square coefficient of variation (RMSCV) and root mean square error (RMSE) were calculated as measures of variability for vBMD and each TF, for males and females and each spine level. Further, to assess changes in vBMD and TFs, means and SDs were calculated for each variable, sorted by spine level and sex. In the same manner, the relative difference per year was calculated for each variable, following the equation:
with VARFU and VARBL denoting the value of a variable in a given spine compartment at baseline (BL) and FU, and BLFU denoting the time interval between BL and FU scans in months. Statistical significance of differences between BL and FU values was determined using paired t-tests for each variable, spine compartment, and sex.
Results
Cohort characteristics
Searching our PACS database, we retrospectively identified 2,480 patients, who underwent a thoracoabdominal MDCT for cancer staging purposes between July 2007 and July 2013. Of those, thoracoabdominal FU scans were available in 1,482. Further, 1,062 patients were excluded due to hematological diseases and bone metastases, bone active medication, or diagnosed osteoporosis. Lastly, 158 were excluded as they did not meet the defined FU interval of 6–26 months and 59 were excluded as they presented VFs at the FU scan. Thus, 203 patients were eligible for inclusion (131 males and 72 females). Detailed information on cohort demographics is presented in Table 1. The average age at BL was 63.0±8.8 years (range, 42–88 years) and the average time interval between BL and FU scans was 13.3±5.4 months. In total, TFs and vBMD were extracted from 2,639 vertebrae and manual correction of automatically generated volumes of interest (VOI) was performed in 28 vertebrae.
Table 1
Parameters | Males | Females | Combined |
---|---|---|---|
Patients, n | 131 | 72 | 203 |
Vertebral bodies, n | 1,703 | 936 | 2,639 |
Age (years) | |||
Mean ± SD | 63.0±8.8 | 61.6±10.2 | 62.5±9.3 |
Median [range] | 63 [42–88] | 63 [39–86] | 63 [39–88] |
FU interval (months) | |||
Mean ± SD | 13.3±5.4 | 12.7±5.8 | 13.1±5.6 |
Median [range] | 12 [6–25] | 11 [6–26] | 12 [6–26] |
SD, standard deviation; FU, follow-up.
Reproducibility of vBMD and texture features
RMSEs revealed notable vBMD variability ranging from 13.4 (L1–L5) to 16.8 mg CaHA/cm3 (T5–T8) in males and between 12.9 (T5–T8) and 16.8 mg CaHA/cm3 (L1–L5) in females (Table 2). Nonetheless a time-dependent decrease was observed for vBMD, irrespective of sex and vertebral level (Figure 2). While Females showed slight vBMD loss in all compartments over the FU interval, vBMD loss in males was more pronounced: maximum decrease per year in males was measured at T5–T8 with −3.9%±25.8% vBMD loss.
Table 2
Level | Sex | BL (mg CaHA/cm3), mean ± SD | FU (mg CaHA/cm3), mean ± SD | RMSCV (%) | RMSE (mg CaHA/cm3) |
Relative vBMD change per year (%), mean ± SD |
---|---|---|---|---|---|---|
T5–T8 | Females | 99.2±33.2 | 96.8±31.2 | 11.9 | 12.9 | −1.5±35.2 |
Males | 102.7±35.2 | 98.5±36.6 | 16.1 | 16.8 | −3.9±25.8* | |
T9–T12 | Females | 98.0±32.3 | 95.1±30.8 | 16.2 | 14.5 | −1.6±21.8 |
Males | 96.8±30.5 | 93.7±31.4 | 12.7 | 14.3 | −3.1±17.9* | |
L1–L5 | Females | 93.9±31.7 | 90.0±29.7 | 13.6 | 16.8 | −2.0±16.9* |
Males | 93.6±27.7 | 91.2±28.7 | 12.2 | 13.4 | −2.5±15.7* |
Statistical significance of differences between BL and FU values was determined using paired t-tests. Statistically significant changes (P value <0.05) are highlighted by an asterisk (*). vBMD, volumetric bone mineral density; BL, baseline; FU, follow-up; RMSCV, root-mean square coefficient of variation; RMSE, root-mean square error; CaHA, calcium hydroxylapatite; SD, standard deviation.
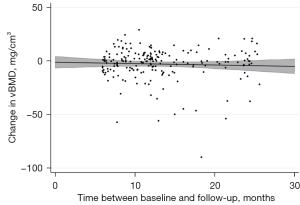
TF reproducibility differed substantially from vBMD findings: RMSCV was below 2% for all features of higher order (Table 3). Notably, despite low variability, changes between BL and FU were statistically significant for each one of these features. While LRE decreased over time, all remaining TFs of higher-order (SRE, RLN, and RP) increased, irrespective of sex and vertebral level (P<0.001) (Figures 3-5). With relative changes per year between 4.40%±5.78% (females, T5–T8) and 8.36%±8.66% (males, level L1–L5), entropy experienced the greatest variability, compared to higher-order TFs, ranging from 4.34% in females at the T5–T8 level to 7.36% in males at the L1–L5 level. Considering the magnitudes of BL and FU values, RMSEs were considerably low for all investigated TFs. Notably, no statistically significant changes were found for the first-order TF varianceglobal (P>0.05, for each level sex and vertebral level, respectively), although the variability was slightly greater compared to higher-order TFs (RMSCV =1.60–3.03%).
Table 3
Texture feature† | Level | Sex | BL, mean ± SD | FU, mean ± SD | RMSCV (%) | RMSE | Relative change per year (%), mean ± SD |
---|---|---|---|---|---|---|---|
Varianceglobal | T5–T8 | Females | 26.47±2.71 | 26.39±2.76 | 2.24 | 0.86 | −0.18±4.23 |
Males | 34.53±4.27 | 34.64±4.29 | 3.03 | 1.47 | 0.31±4.66 | ||
T9–T12 | Females | 39.97±3.90 | 39.79±3.79 | 1.60 | 0.91 | −0.50±2.81 | |
Males | 51.44±5.89 | 51.40±5.70 | 1.60 | 1.19 | 0.05±2.43 | ||
L1–L5 | Females | 56.82±5.30 | 56.67±5.19 | 1.73 | 1.40 | −0.04±3.21 | |
Males | 67.98±7.02 | 67.97±6.65 | 2.24 | 2.13 | 0.28±4.05 | ||
Entropy | T5–T8 | Females | 14.14±0.56 | 14.78±0.66 | 4.34 | 0.50 | 4.40±5.78** |
Males | 14.20±0.57 | 15.15±0.68 | 5.91 | 0.56 | 6.66±7.26** | ||
T9–T12 | Females | 14.32±0.61 | 15.02±0.81 | 4.92 | 0.54 | 4.75±6.93** | |
Males | 14.31±0.56 | 15.30±0.76 | 6.20 | 0.55 | 6.89±7.73** | ||
L1–L5 | Females | 14.54±0.62 | 15.68±0.89 | 5.73 | 0.58 | 6.07±7.86** | |
Males | 14.45±0.58 | 15.43±0.86 | 7.36 | 0.58 | 8.36±8.66** | ||
SRE | T5–T8 | Females | 0.9918±0.0021 | 0.9945±0.0024 | 0.27 | 0.0020 | 0.25±0.35** |
Males | 0.9909±0.0022 | 0.9949±0.0023 | 0.36 | 0.0023 | 0.38±0.40** | ||
T9–T12 | Females | 0.9913±0.0022 | 0.9942±0.0029 | 0.30 | 0.0021 | 0.27±0.40** | |
Males | 0.9903±0.0023 | 0.9944±0.0026 | 0.38 | 0.0024 | 0.40±0.43** | ||
L1–L5 | Females | 0.9914±0.0022 | 0.9947±0.0028 | 0.32 | 0.0022 | 0.30±0.39** | |
Males | 0.9907±0.0024 | 0.9950±0.0028 | 0.40 | 0.0024 | 0.41±0.43** | ||
LRE | T5–T8 | Females | 1.0338±0.0088 | 1.0222±0.0100 | 1.45 | 0.0086 | −1.01±1.39** |
Males | 1.0375±0.0096 | 1.0209±0.0095 | 1.11 | 0.0096 | −1.52±1.59** | ||
T9–T12 | Females | 1.0359±0.0093 | 1.0239±0.0120 | 1.21 | 0.0090 | −1.06±1.61** | |
Males | 1.0400±0.0100 | 1.0228±0.0108 | 1.54 | 0.0101 | −1.58±1.72** | ||
L1–L5 | Females | 1.0353±0.0095 | 1.0218±0.0117 | 1.28 | 0.0092 | −1.21±1.57** | |
Males | 1.0383±0.0101 | 1.0203±0.0115 | 1.61 | 0.0102 | −1.63±1.72** | ||
RLN | T5–T8 | Females | 0.9784±0.0054 | 0.9856±0.0062 | 0.72 | 0.0053 | 0.67±0.92** |
Males | 0.9762±0.0058 | 0.9865±0.0059 | 0.94 | 0.0058 | 1.01±1.06** | ||
T9–T12 | Females | 0.9771±0.0058 | 0.9846±0.0075 | 0.79 | 0.0056 | 0.71±1.05** | |
Males | 0.9747±0.0060 | 0.9853±0.0067 | 1.00 | 0.0061 | 1.05±1.14** | ||
L1–L5 | Females | 0.9775±0.0058 | 0.9859±0.0073 | 0.84 | 0.0057 | 0.81±1.04** | |
Males | 0.9757±0.0061 | 0.9869±0.0072 | 1.05 | 0.0061 | 1.09±1.14** | ||
RP | T5–T8 | Females | 0.9890±0.0028 | 0.9927±0.0032 | 0.38 | 0.0027 | 0.34±0.47** |
Males | 0.9878±0.0030 | 0.9931±0.0030 | 0.48 | 0.0030 | 0.52±0.54** | ||
T9–T12 | Females | 0.9883±0.0030 | 0.9922±0.0038 | 0.40 | 0.0029 | 0.36±0.54** | |
Males | 0.9870±0.0031 | 0.9925±0.0035 | 0.51 | 0.0032 | 0.53±0.58** | ||
L1–L5 | Females | 0.9885±0.0030 | 0.9929±0.0038 | 0.43 | 0.0029 | 0.41±0.53** | |
Males | 0.9876±0.0032 | 0.9934±0.0037 | 0.54 | 0.0032 | 0.55±0.58** |
†, texture features are dimensionless. Statistical significance of differences between BL and FU values was determined using paired t-tests. **, P<0.001. BL, baseline; SD, standard deviation; FU, follow-up; RMSCV, root-mean square coefficient of variation; RMSE, root-mean square error; SRE, short-run emphasis; LRE, long-run emphasis; RLN, run-length non-uniformity; RP, run percentage.
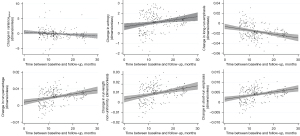
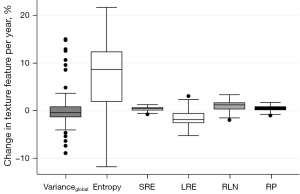
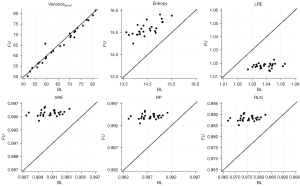
Discussion
This study provided evidence for reproducibility of a set of TFs and vBMD up to 26 months in a bone-healthy cohort and a single-scanner environment. Precise knowledge of physiological TF values and reproducibility thereof is warranted, as it may serve as the basis for future analyses, aiming to differentiate the healthy from osteopenic and osteoporotic subjects. However, TA is highly susceptible to both, changes in scan protocol and post-processing steps. This can result in inherently difficult comparability of absolute values between scans and studies. This study used an identical scan protocol for BL and FU scans to reduce related precision errors and provides reference values for relative annual TF changes. Following this principle, the observed changes of the TFs should also be comprehensible using other scanners and protocols, as long as the setup remains unchanged for BL and FU scans.
vBMD values decreased steadily in both sexes by about 2% per year, matching established models for trabecular bone loss in healthy subjects by Mazess et al. (13% per decade) and Block et al. (1.99–4.39 mg/mL per year) (27,28). The examined TFs had lower variability compared to extracted vBMD values, resulting in lower RMSCVs and RMSEs. The low variability of the analyzed TFs is in line with findings previously documented by Dieckmeyer et al., who found reproducibility errors of <5% over a period of 2 months, and magnitudes of TFs were also comparable (17). In contrast, varianceglobal values reported by Mannil et al. differ substantially from our findings (29). This may be explained by methodological differences, as we performed TA analogously to Dieckmeyer et al. on 3D regions of interest (ROIs) using an automated segmentation algorithm (17). At the same time, analyses by Mannil et al. was carried out two-dimensionally on mid-spine sagittal slices (29).
Varianceglobal measurements were reproducible in females and males, irrespective of the vertebral level, and our results match previous findings (11). While absolute values were greatest at the lumbar spine. As a TF of first-order, varianceglobal is a measure for the spread of gray-level distribution within the segmented vertebral bodies, and differences in trabecular bone architecture along the vertebral levels may explain the different values along the spine (22). However, a time-dependent decrease in varianceglobal, as expected in light of the results by Mannil et al., could not be confirmed (29). Nevertheless, considering the spatial distribution of varianceglobal values, Dieckmeyer et al. pointed out, that degenerative changes (typically most pronounced at the lumbar spine) and differences in vertebral body size may account for the observed differences (17).
Of all analyzed TFs, entropy showed greatest variability. The observed significant increases in entropy which occurred in both, females and males, may partially account for this finding. Similar to varianceglobal, we observed the greatest changes at the lumbar spine. The spatial dependence of our findings is supported by a previous study that observed significantly greater entropy in the lumbar spine compared to the thoracic spine (17). As this TF is a measure of inhomogeneity in gray-value distribution, it is thought to reflect trabecular deterioration, which could explain the observed annual increases. If this association is confirmed in future studies, entropy may be of interest as marker for the structural integrity, i.e., quality, of the trabecular bone. However, in contrast to our findings, Tabari et al. found positive associations between entropy values and vBMD by QCT in a study investigating trabecular microarchitectural changes in anorexia nervosa patients (30). A possible reason may be the different etiologies of BMD loss in anorexia nervosa patients versus a healthy, aging population. Nonetheless, the distinct biological correlate of this complex measure are yet to be determined.
Values of the higher-order TFs RLN, LRE, RP, and SRE demonstrated substantial reproducibility, although the observed changes were homogeneous and statistically significant. Notably, LRE was the only TF to decrease in both sexes and all vertebral compartments. However, an inverse relationship of SRE and LRE is non-surprising, as the greater emphasis on shorter run-lengths (captured by SRE) inherently describes less emphasis on longer run-lengths (captured by LRE). While it seems somewhat intuitive, that LRE may be a measure reflecting the quantity of long trabeculae and SRE of short trabeculae, it remains unclear how these findings correspond to actual microarchitectural changes in the trabecular bone. Further studies, e.g., with multiple FU time-points and a robust ground truth, are needed to further investigate the observed time-dependent changes in TFs and to determine their biologic equivalent. Thus, caution is warranted when interpreting the TFs.
The retrospective design of this study is the first limitation demanding attention: As data were collected retrospectively, important demographic factors and biomarkers of this chronic metabolic disease, e.g., race, diet, smoking status and body mass index that can impact the development and progression of osteopenia and osteoporosis, could not be assessed or adjusted for. However, the aim of this study is not to assess TFs as diagnostic tool for osteoporosis, but to investigate TF reproducibility and to describe the observed TF changes over time in a bone healthy population in order to lay the foundation for further, highly warranted studies concerning the value of TFs in osteoporosis diagnostics. Second, participants included in this study were scanned for cancer staging and/or FU purposes. Although we screened for and excluded patients receiving bone-active medications, the effects that drugs may have on bone microarchitecture rather than on BMD are largely unknown, introducing a possible bias into BMD measurements and TA. Third, the external validity is limited, as TF values are extremely susceptible to changes in scanning protocols and the scanning setup. However, this study investigated reproducibility of TFs and changes thereof, which ought to be reproducible in different scanning environments, as long as scan protocols are not altered between BL and FU scans. The manual correction performed during the vertebral body segmentation may introduce a bias into our data. Yet, only 28 out of 2,639 vertebral bodies required manual correction, therefore we regard this possible bias acceptable.
In conclusion, this study established reference reproducibility values for TFs for up to 26 months in a single-scanner environment. Overall, reproducibility was greater in TFs compared to opportunistic vBMD measurements. Entropy and all TFs of higher order showed characteristic time dependent changes. However, it remains unclear, to what extent TFs capture bone microarchitecture and changes thereof, thus cautious interpretation and further investigations are highly warranted.
Acknowledgments
The authors express their gratitude towards Malek El Husseini and Anjany Sekuboyina (co-founders of bonescreen GmbH) for developing the segmentation tool.
Funding: This work was supported by
Footnote
Conflicts of Interest: All authors have completed the ICMJE uniform disclosure form (available at https://qims.amegroups.com/article/view/10.21037/qims-23-19/coif). JSK is co-founder of bonescreen GmbH, received funding by the Deutsche Forschungsgemeinschaft and honoraria for lectures on multiple sclerosis by Novartis. TB received funding by the Deutsche Forschungsgemeinschaft. The other authors have no conflicts of interest to declare.
Ethical Statement: The authors are accountable for all aspects of the work in ensuring that questions related to the accuracy or integrity of any part of the work are appropriately investigated and resolved. The study was conducted in accordance with the Declaration of Helsinki (as revised in 2013). The study was approved by institutional ethics committee of Klinikum rechts der Isar, Technical University of Munich and individual consent for this retrospective analysis was waived.
Open Access Statement: This is an Open Access article distributed in accordance with the Creative Commons Attribution-NonCommercial-NoDerivs 4.0 International License (CC BY-NC-ND 4.0), which permits the non-commercial replication and distribution of the article with the strict proviso that no changes or edits are made and the original work is properly cited (including links to both the formal publication through the relevant DOI and the license). See: https://creativecommons.org/licenses/by-nc-nd/4.0/.
References
- Osteoporosis prevention, diagnosis, and therapy. JAMA 2001;285:785-95. [Crossref] [PubMed]
- Rachner TD, Khosla S, Hofbauer LC. Osteoporosis: now and the future. Lancet 2011;377:1276-87. [Crossref] [PubMed]
- Hernlund E, Svedbom A, Ivergård M, Compston J, Cooper C, Stenmark J, McCloskey EV, Jönsson B, Kanis JA. Osteoporosis in the European Union: medical management, epidemiology and economic burden. A report prepared in collaboration with the International Osteoporosis Foundation (IOF) and the European Federation of Pharmaceutical Industry Associations (EFPIA). Arch Osteoporos 2013;8:136. [Crossref] [PubMed]
- Chesnut CH 3rd. Osteoporosis, an underdiagnosed disease. JAMA 2001;286:2865-6. [Crossref] [PubMed]
- Löffler MT, Kallweit M, Niederreiter E, Baum T, Makowski MR, Zimmer C, Kirschke JS. Epidemiology and reporting of osteoporotic vertebral fractures in patients with long-term hospital records based on routine clinical CT imaging. Osteoporos Int 2022;33:685-94. [Crossref] [PubMed]
- Link TM, Kazakia G. Update on Imaging-Based Measurement of Bone Mineral Density and Quality. Curr Rheumatol Rep 2020;22:13. [Crossref] [PubMed]
- Link TM. Osteoporosis imaging: state of the art and advanced imaging. Radiology 2012;263:3-17. [Crossref] [PubMed]
- Löffler MT, Sollmann N, Mei K, Valentinitsch A, Noël PB, Kirschke JS, Baum T. X-ray-based quantitative osteoporosis imaging at the spine. Osteoporos Int 2020;31:233-50. [Crossref] [PubMed]
- Muehlematter UJ, Mannil M, Becker AS, Vokinger KN, Finkenstaedt T, Osterhoff G, Fischer MA, Guggenberger R. Vertebral body insufficiency fractures: detection of vertebrae at risk on standard CT images using texture analysis and machine learning. Eur Radiol 2019;29:2207-17. [Crossref] [PubMed]
- Mookiah MRK, Rohrmeier A, Dieckmeyer M, Mei K, Kopp FK, Noel PB, Kirschke JS, Baum T, Subburaj K. Feasibility of opportunistic osteoporosis screening in routine contrast-enhanced multi detector computed tomography (MDCT) using texture analysis. Osteoporos Int 2018;29:825-35. [Crossref] [PubMed]
- Valentinitsch A, Trebeschi S, Kaesmacher J, Lorenz C, Löffler MT, Zimmer C, Baum T, Kirschke JS. Opportunistic osteoporosis screening in multi-detector CT images via local classification of textures. Osteoporos Int 2019;30:1275-85. [Crossref] [PubMed]
- Sekuboyina A, Husseini ME, Bayat A, Löffler M, Liebl H, Li H, et al. VerSe: A Vertebrae labelling and segmentation benchmark for multi-detector CT images. Med Image Anal 2021;73:102166. [Crossref] [PubMed]
- Löffler MT, Jacob A, Scharr A, Sollmann N, Burian E, El Husseini M, Sekuboyina A, Tetteh G, Zimmer C, Gempt J, Baum T, Kirschke JS. Automatic opportunistic osteoporosis screening in routine CT: improved prediction of patients with prevalent vertebral fractures compared to DXA. Eur Radiol 2021;31:6069-77. [Crossref] [PubMed]
- Dieckmeyer M, Löffler MT, El Husseini M, Sekuboyina A, Menze B, Sollmann N, Wostrack M, Zimmer C, Baum T, Kirschke JS. Level-Specific Volumetric BMD Threshold Values for the Prediction of Incident Vertebral Fractures Using Opportunistic QCT: A Case-Control Study. Front Endocrinol (Lausanne) 2022;13:882163. [Crossref] [PubMed]
- Sollmann N, Löffler MT, El Husseini M, Sekuboyina A, Dieckmeyer M, Rühling S, Zimmer C, Menze B, Joseph GB, Baum T, Kirschke JS. Automated Opportunistic Osteoporosis Screening in Routine Computed Tomography of the Spine: Comparison With Dedicated Quantitative CT. J Bone Miner Res 2022;37:1287-96. [Crossref] [PubMed]
- Rühling S, Navarro F, Sekuboyina A, El Husseini M, Baum T, Menze B, Braren R, Zimmer C, Kirschke JS. Automated detection of the contrast phase in MDCT by an artificial neural network improves the accuracy of opportunistic bone mineral density measurements. Eur Radiol 2022;32:1465-74. [Crossref] [PubMed]
- Dieckmeyer M, Sollmann N, El Husseini M, Sekuboyina A, Löffler MT, Zimmer C, Kirschke JS, Subburaj K, Baum T. Gender-, Age- and Region-Specific Characterization of Vertebral Bone Microstructure Through Automated Segmentation and 3D Texture Analysis of Routine Abdominal CT. Front Endocrinol (Lausanne) 2021;12:792760. [Crossref] [PubMed]
- Rühling S, Scharr A, Sollmann N, Wostrack M, Löffler MT, Menze B, Sekuboyina A, El Husseini M, Braren R, Zimmer C, Kirschke JS. Proposed diagnostic volumetric bone mineral density thresholds for osteoporosis and osteopenia at the cervicothoracic spine in correlation to the lumbar spine. Eur Radiol 2022;32:6207-14. [Crossref] [PubMed]
- Vallières M, Kay-Rivest E, Perrin LJ, Liem X, Furstoss C, Aerts HJWL, Khaouam N, Nguyen-Tan PF, Wang CS, Sultanem K, Seuntjens J, El Naqa I. Radiomics strategies for risk assessment of tumour failure in head-and-neck cancer. Sci Rep 2017;7:10117. [Crossref] [PubMed]
- Zhou H, Vallières M, Bai HX, Su C, Tang H, Oldridge D, Zhang Z, Xiao B, Liao W, Tao Y, Zhou J, Zhang P, Yang L. MRI features predict survival and molecular markers in diffuse lower-grade gliomas. Neuro Oncol 2017;19:862-70. [Crossref] [PubMed]
- Vallières M, Freeman CR, Skamene SR, El Naqa I. A radiomics model from joint FDG-PET and MRI texture features for the prediction of lung metastases in soft-tissue sarcomas of the extremities. Phys Med Biol 2015;60:5471-96. [Crossref] [PubMed]
- Gaztañaga E, Croft RAC, Dalton GB. Variance, Skewness and Kurtosis: results from the APM Cluster Redshift Survey and Model Predictions. Mon Notices Royal Astron Soc 1995;276:336-46.
- Haralick RM, Shanmugam K, Dinstein I. Textural Features for Image Classification. IEEE Trans Syst Man Cybern 1973;SMC-3:610-21.
- Galloway MM. Texture analysis using gray level run lengths. Computer Graphics and Image Processing 1975;4:172-9.
- Engelke K, Adams JE, Armbrecht G, Augat P, Bogado CE, Bouxsein ML, Felsenberg D, Ito M, Prevrhal S, Hans DB, Lewiecki EM. Clinical use of quantitative computed tomography and peripheral quantitative computed tomography in the management of osteoporosis in adults: the 2007 ISCD Official Positions. J Clin Densitom 2008;11:123-62. [Crossref] [PubMed]
- Siminoski K, Lee KC, Jen H, Warshawski R, Matzinger MA, Shenouda N, Charron M, Coblentz C, Dubois J, Kloiber R, Nadel H, O'Brien K, Reed M, Sparrow K, Webber C, Lentle B, Ward LM. STOPP Consortium. Anatomical distribution of vertebral fractures: comparison of pediatric and adult spines. Osteoporos Int 2012;23:1999-2008. [Crossref] [PubMed]
- Block JE, Smith R, Glueer CC, Steiger P, Ettinger B, Genant HK. Models of spinal trabecular bone loss as determined by quantitative computed tomography. J Bone Miner Res 1989;4:249-57. [Crossref] [PubMed]
- Mazess RB. On aging bone loss. Clin Orthop Relat Res 1982;239-52.
- Mannil M, Eberhard M, Becker AS, Schönenberg D, Osterhoff G, Frey DP, Konukoglu E, Alkadhi H, Guggenberger R. Normative values for CT-based texture analysis of vertebral bodies in dual X-ray absorptiometry-confirmed, normally mineralized subjects. Skeletal Radiol 2017;46:1541-51. [Crossref] [PubMed]
- Tabari A, Torriani M, Miller KK, Klibanski A, Kalra MK, Bredella MA. Anorexia Nervosa: Analysis of Trabecular Texture with CT. Radiology 2017;283:178-85. [Crossref] [PubMed]