Artificial intelligence system-based histogram analysis of computed tomography features to predict tumor invasiveness of ground-glass nodules
Introduction
Ground-glass nodules (GGNs) are defined as dense and hazy lung lesions that do not obscure the bronchial structures or pulmonary vascular structures on computed tomography (CT) images (1). Lung adenocarcinoma (LUAD) is a common type of lung cancer, which could present as GGNs on CT scans (2). Preinvasive lesions, such as atypical adenomatous hyperplasia (AAH) and adenocarcinoma in situ (AIS), and invasive lesions, such as minimally invasive adenocarcinoma (MIA) and invasive adenocarcinoma (IAC), represent different stages of LUAD (3,4). Notably, AAH, AIS, MIA, and IAC can be detected as varying degrees of ground glass opacities on CT scans (5,6). Many studies indicate that the size and mass of the nodules are also significant factors in differentiating precancerous lesions (PLs) and those of IAC (7-9). Furthermore, the early detection and diagnosis of LUAD presenting as GGNs can improve the survival of patients.
With the widespread application of CT in lung disease screening, the detection rate of GGNs has gradually increased (10). Moreover, the identification of the qualitative and quantitative features of GGNs on chest CT has allowed for the evaluation of tumor invasiveness in clinical practice (11). Previous studies have suggested that different texture features from each CT image could predict whether lesions are preinvasive or invasive (7,12). However, the accuracy of diagnosis based on nonenhanced CT (NECT) images is limited. Recently, contrast-enhanced CT (CECT) has been reported to provide enhanced diagnosis of malignant tumors such as lung and brain tumors and liver cancer. Yang et al. showed that quantitative parameters on iodine-enhanced images of dual-source DECT could accurately and reliably differentiate adenocarcinoma lesions from PLs (13). However, the value of CECT images may vary due to the breath holding of patients and the local volume effects of image acquisition (14,15), and whether enhanced CT is sufficiently sensitive to distinguish PLs from adenocarcinoma lesions remains controversial.
For clinicians and radiologists, manually interpreting hundreds of CT images of GGNs constitutes highly burdensome workload. In recent years, artificial intelligence (AI) systems have been applied in the medical imaging field for variety of purposes, such as in assisting in the detection of pulmonary nodules (16,17), in the preoperative planning of lung cancer resection (18), in the quantitative assessment of acute pulmonary thromboembolism (19), and in the triage of patients with coronavirus disease 2019 (COVID-19) (20,21). It has been reported that AI can automatically and quantitatively analyze the characteristics of pulmonary nodules on CT images, which may include maximum CT value, minimum CT value, mean CT value, and solid component volume (22). Nevertheless, only a few studies have scrutinized the applicability of AI-measured CT parameters in screening LUAD and differentiating the its pathological subtypes (23,24). A recently published article explored the role of AI-based histogram analyses on plain chest CT images in predicting the tumor invasiveness of lung adenocarcinoma manifesting as part-solid nodules (24). Yet, the validation of AI system performance and the benefit of CECT scans for the differentiation of invasiveness among LUAD presenting as GGNs is lacking. Therefore, this study aimed to evaluate the value of an AI diagnostic system in screening LUAD and the capacity of a multivariable logistic regression model based on automatically AI-measured histogram CT parameters to predict the tumor invasiveness of GGNs. The added value of CECT scans compared with NECT scans in the predicting the tumor invasiveness of GGNs was also examined. We present this article in accordance with the TRIPOD reporting checklist (available at https://qims.amegroups.com/article/view/10.21037/qims-23-31/rc).
Methods
Study design and participants
This study employed a retrospective, cross-sectional design based on routine care data. In addition to paired NECT and CECT images, demographic and clinical characteristics of patients were collected, including gender, age, and pathological examination results. Given the requirement of data categories and the availability of existing data, patients with persistent GGNs (those that did not disappear after 3 months of follow-up) who visited the Department of Radiology, General Hospital of Ningxia Medical University between January 2018 and December 2020 were enrolled based on following inclusion and exclusion criteria described below. This study was conducted in accordance with the Declaration of Helsinki (as revised in 2013) and was reviewed and approved by the Ethics Committee of General Hospital of Ningxia Medical University (No. KYLL-2021-535). Informed consent was waived due to the retrospective nature of the study.
The inclusion criteria were the following: (I) patients with persistent GGNs confirmed by surgery and pathology; (II) nodules appearing as GGNs on the lung window setting (width 1,500 HU; level −700 HU) with an image thickness ≤1.25 mm, and (III) GGNs not shrinking or disappearing during 3 months of follow-up. Meanwhile, the exclusion criteria were as follows: (I) incomplete pathological results, (II) patients without preoperative paired NECT and CECT scans, (III) patients with severe organ dysfunction, (IV) patients with radiotherapy or chemotherapy, (V) patients with lung metastases, (VI) poor quality images with respiratory motion artifacts, and (VII) patients with cavities in the nodules. The flowchart in Figure 1 displays the details of the patient enrollment.
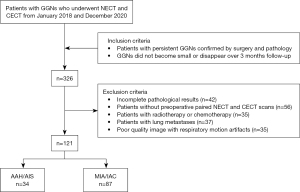
Ultimately, a total of 116 patients with persistent GGNs who underwent thoracic surgeries were enrolled in this study, including 33 males and 83 females. The age range was 31–78 years, and the mean age was 57.17±0.92 years. Since 5 patients were diagnosed with 2 LUAD GGNs, 121 GGNs were analyzed in this study, including 32 pure GGNs and 89 subsolid nodules. Postsurgery pathological examination results were collected from the electronic medical record of each enrolled patient. According to the 2021 World Health Organization (WHO) classification of thoracic tumors (25), 34 lesions with a pathological diagnosis of AAH or AIS were categorized as precursor glandular lesions and placed into a PL group (noninvasive lesions), while 87 lesions diagnosed as MIA or IAC were placed into an adenocarcinoma group (invasive lesions). Detailed clinical characteristics of patients in the 2 groups are shown in Table 1.
Table 1
Characteristics | PL (n=34) | Adenocarcinoma lesion (n=87) | P value |
---|---|---|---|
Gender | 0.032 | ||
Male | 10 (31.25) | 23 (27.4) | |
Female | 22 (68.75) | 61 (72.6) | |
Age, years | 56.56±12.16 | 57.40±9.34 | 0.994 |
Nonenhanced CT | |||
Max CT value (HU) | −175.82±170.64 | −3.50 (−108, 61) | <0.001 |
Min CT value (HU) | −793.88±53.68 | −752.50 (−792, −673) | <0.001 |
Mean CT value (HU) | −627.67±94.28 | −529 (−613, −389) | 0.001 |
SCV (mm3) | 0 (0, 31.51) | 33.55 (0.72, 303.52) | 0.001 |
Arterial-phase enhanced CT | |||
Max CT value (HU) | −71.41±233.22 | 66.48±188.43 | 0.49 |
Min CT value (HU) | −772.79±57.24 | −730 (−783, −642) | 0.001 |
Mean CT value (HU) | −604.44±97.03 | −505 (−598, −364) | 0.001 |
SCV (mm3) | 4.07 (0, 47.65) | 65.60 (9.02, 323.64) | 0.001 |
Venous-phase enhanced CT | |||
Max CT value (HU) | −90.76±213.01 | 84.50 (−24, 173) | 0.001 |
Min CT value (HU) | −790.50 (−810, −735) | −715.50 (−775, −610) | 0.001 |
Mean CT value (HU) | −598.58±103.11 | −511 (−587, −341) | 0.001 |
SCV (mm3) | 2.09 (0, 55.36) | 64.18 (9.67, 376.92) | 0.001 |
Delayed-phase enhanced CT | |||
Max CT value (HU) | −100.91±210.20 | 56 (−38, 135) | 0.002 |
Min CT value (HU) | −776.14±58.54 | −733 (−788, −627) | 0.001 |
Mean CT value (HU) | −620.50 (−681, −542.25) | −496.50 (−597, −344) | 0.001 |
SCV (mm3) | 1.25 (0, 42.86) | 81.08 (6.79, 379.85) | 0.001 |
Continuous data with a normal distribution are presented as mean ± SD; data with skewed distribution are presented as median (P25, P75). CT, computed tomography; GGN, ground-glass nodule; PL, precancerous lesion; SCV, solid component volume; HU, Hounsfield unit; SD, standard deviation.
AI system-based analyses were performed on the collected NECT and CECT scans from the enrolled patients and was followed by target lesion identification. Upon validation of the AI system performance, histogram features were analyzed and used to construct a model for invasiveness differentiation among the LUAD GGNs. The details of this process are shown in Figure 2.
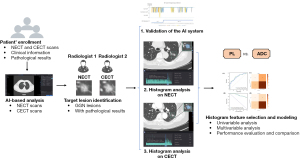
Chest CT acquisition
For chest CT acquisition, the patient was placed in a supine position with the head advanced, and a whole lung scan was completed after 1 inhalation and breath hold. The scanning range was from the thoracic entrance to the bottom of the lung. The protocol parameters were as follows: tube voltage, 120 kVp; tube current, 200 mA; thickness, 8 mm; pitch, 1.375; field of view, 387 mm; and acquisition matrix, 512×512. After the nonenhanced scan, contrast media (300 mgI/mL) was injected through the cubital vein for an enhanced scan at a flow rate of 3.0 mL/s with a flow volume of 60–80 mL. After contrast agent injection, 100 mL of normal saline was injected. Three scanning phases were performed at 30 s (arterial phase), 60 s (venous phase), and 90 s (delayed phase), respectively. After the scans, the data were reconstructed by using the standard algorithm (B31) with a layer thickness of 1.0 mm. Image observation conditions included a lung window (width 2,000 HU, window position −500 HU) and a mediastinal window (width 400 HU, window level 45 HU), with appropriate window adjustments being performed as necessary.
Detection, auxiliary diagnosis, and histogram analysis of GGNs with the AI diagnostic system
A commercial AI system, InferRead CT Lung (Infervision Medical Technology Co., Ltd., Beijing, China), was used to automatically perform nodule detection (16), nodule malignancy prediction (17), nodule segmentation(17), and subsequent histogram analyses. In particular, all thin-layer original axial CT images were first sent to the server of the deep learning (DL)-based pulmonary nodule auxiliary detection system for automatic detection of all pulmonary nodules. Meanwhile, the detected nodules were automatically precisely segmented for measurement tasks. Subsequently, a DL algorithm trained on pathologically confirmed lesions predicted the malignancy probability of a single nodule based on the nodule’s features and its surrounding microenvironment (detection bounding box) and output the malignancy probability in terms of percentage. According to malignancy, GGNs were categorized into low-risk, intermediate-risk, and high-risk groups based on the thresholds of 50% and 70% probabilities. To ensure an accurate selection of targeted GGNs with pathological results from all those detected by the AI system on CT images, a radiologist with 5 years of experience and a senior radiologist with 10 years of experience reviewed the detected pulmonary nodules independently; targeted GGNs were then manually selected according to ‘the construction of pulmonary nodules data set of chest CT and the consensus of experts on quality control’ (26), and the pathological examination results. Any disagreements between them, especially concerning the nodule’s attenuation, were settled through discussion. Of note, once the AI diagnostic system detected the pulmonary nodules, histogram analysis was automatically performed based on precise segmentation contours of the nodules being examined. In particular, the maximum CT value, minimum CT value, mean CT value, nonsolid component volume, solid component volume, kurtosis, and skewness of the interested GGNs in each scanning period were automatically calculated and recorded for analysis (Figure 3). For the solid component volume, the algorithm differentiated solid components and nonsolid components with a threshold of –145 HU, and voxels with a density over −145 HU were regarded as the solid components. The volume was calculated using the voxel-counting method, with the detailed formulae listed in Figure 3.
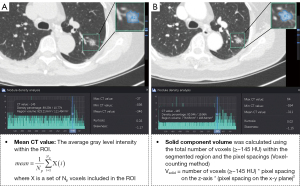
Statistical analysis
All data were analyzed with SPSS 23.0 software (IBM Corp.), and the chi-squared test was used to analyze categorical variables. The Kolmogorov-Smirnov normality test was used for the continuous data. Continuous data with a normal distribution were compared with a t test and are presented as the mean ± standard deviation (SD). Continuous data with a skewed distribution were compared with the Mann-Whitney test and are presented as the median (P25, P75). Of note, since each GGN was considered an independent lesion, within-patient correlations were not considered when a patient had multiple GGNs (27). CT parameters with P values of less than 0.05 on univariable analyses were considered as candidate input variables. After the examination of the collinearity of candidate CT parameters, multivariable logistic regression analyses were performed to identify the independent factors for the stratification of PLs and invasive adenocarcinoma. Sensitivity, specificity, receiver operating characteristic (ROC) curve, precision-recall (PR) curve, and confusion matrix were used to evaluate the performance. The cutoff value was determined by the geometric mean. Delong test was used to compare the area under the ROC curve (AUC) and PR curve (AUPR) of the NECT and CECT models. P<0.05 was considered statistically significant.
Results
Performance evaluation of the AI diagnostic system
Both NECT and CECT scans were collected from the enrolled 116 patients for pulmonary nodule detection via the AI diagnostic system. Two radiologists participated in identifying targeted GGNs from the AI-detected pulmonary nodules. The intraobserver intraclass correlation coefficient (ICC) ranged from 0.861 to 0.972 based on 2 rounds of results from radiologist A. The ICC ranged from 0.821 to 0.921 for radiologist B. These results indicated that the interobserver reproducibility of GGN screening was good. Of note, DL-based AI diagnostic system achieved a sensitivity of 100%, as it detected all the targeted GGNs on both NECT and CECT images, as confirmed by the 2 radiologists.
In addition, the AI diagnostic system predicted the probability of malignancy for these targeted GGNs. Notably, the AI system alerted radiologists to at least an intermediate risk for all lesions (100%), either based on NECT or CECT (artery phase and delayed phase) images; meanwhile, this proportion was 99.2% (120/121) on venous-phase images of CECT. When assigning PLs and adenocarcinoma lesions intermediate- and high-risk grades, the AI auxiliary diagnosis system tended to predict both groups as high-risk (Figure 4), and the prediction accuracy of the adenocarcinoma group was significantly higher than that of the PL group (P<0.05).
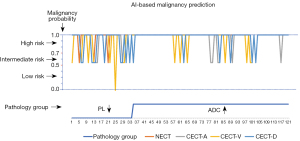
Selection of CT parameters from AI-based histogram analysis
For screened GGNs, the DL-based AI diagnostic system automatically measured related CT parameters, including maximum CT value, minimum CT value, mean CT value, solid component volume, nonsolid component volume, kurtosis, and skewness. We noticed that most CT parameters varied on NECT and CECT images and were significantly different between the PL group and adenocarcinoma group (all P values <0.05) (Table 1). Univariable analysis revealed that maximum CT value, minimum CT value, mean CT value, and solid component volume were significantly related to GGN tumor invasiveness (P<0.05). The detailed results of the univariable analyses are summarized in Table 1.
Given the collinearity among CT value-related parameters, mean CT value combined with solid component volume was eventually selected and used for further analysis. The multivariable logistic regression analysis revealed that the mean CT value and solid component volume of NECT and CECT were independent factors for the differentiation of PLs and adenocarcinoma lesions [NECT: odds ratio (OR) 1.009, 95% confidence interval (CI): 1.004–1.013, P<0.001; OR 1.005, 95% CI: 1.000–1.010, P=0.032; arterial-phase CECT: OR 1.008, 95% CI: 1.004–1.013, P<0.001; OR 1.003, 95% CI: 1.000–1.006, P=0.038; venous-phase CECT: OR 1.008, 95% CI: 1.004–1.012, P<0.001; OR 1.003, 95% CI: 1.000–1.005, P=0.039; delayed-phase CECT: OR 1.008, 95% CI: 1.004–1.012, P<0.001; OR 1.004, 95% CI: 1.002–1.015, P=0.010) (Table 2).
Table 2
Quantitative parameter | P value | OR | 95% CI |
---|---|---|---|
Nonenhanced CT | |||
Mean CT value | <0.001 | 1.009 | 1.004–1.013 |
SCV | 0.032 | 1.005 | 1.000–1.010 |
Arterial-phase enhanced CT | |||
Mean CT value | <0.001 | 1.008 | 1.004–1.013 |
SCV | 0.038 | 1.003 | 1.000–1.006 |
Venous-phase enhanced CT | |||
Mean CT value | <0.001 | 1.008 | 1.004–1.012 |
SCV | 0.039 | 1.003 | 1.000–1.005 |
Delayed-phase enhanced CT | |||
Mean CT value | <0.001 | 1.008 | 1.004–1.012 |
SCV | 0.010 | 1.004 | 1.002–1.015 |
CT, computed tomography; OR, odds ratio; SCV, solid component volume; CI, confidence interval.
Comparison of NECT and CECT parameters in predicting the invasiveness of GGNs tumors
To elucidate the potential value of CECT histogram characteristics in predicting GGN tumor invasiveness, we compared the performance of the NECT model and the CECT model. In particular, the AUC and AUPR of the NECT-based model were 0.765 (95% CI: 0.679–0.837) and 0.898 (95% CI: 0.815–0.946), respectively (Figure 5), showing no significant difference with CECT models. The optimal performance was observed in the CECT delayed-phase in which the AUC and AUPR reached 0.772 (95% CI: 0.687–0.843) and 0.907 (95% CI: 0.825–0.953), respectively (Figure 5). The AUC of CECT artery phase and venous phase were 0.757 (95% CI: 0.670–0.830) and 0.894 (95% CI: 0.810–0.943), respectively. The AUPR of the CECT artery phase and venous phase were 0.764 (95% CI: 0.678–0.836) and 0.895 (95% CI: 0.812–0.944), respectively. Additionally, other metrics, including sensitivity, specificity, accuracy, and precision, did not differ significantly between the NECT and CECT models. Details of these metrics are summarized in Table 3. The confusion matrices of the NECT model and CECT delayed-phase model are shown in Figure 5B,5D.
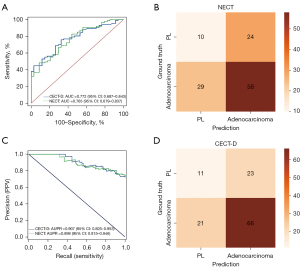
Table 3
Group | AUC | Sensitivity | Specificity | Accuracy | Precision | F1-score | G-Mean |
---|---|---|---|---|---|---|---|
NECT | 0.765 | 0.667 | 0.706 | 0.562 | 0.707 | 0.687 | 0.686 |
CECT-D | 0.772 | 0.759 | 0.676 | 0.636 | 0.742 | 0.750 | 0.716 |
P value | 0.661 | 0.180 | 0.793 | 0.238 | 0.616 | – | – |
CECT-D, delayed phase of contrast-enhanced computed tomography; NECT, nonenhanced computed tomography; AUC, area under the receiver operating characteristic curve; G-Mean, geometric mean.
Discussion
In this study, the performance of the DL-based AI diagnostic system in detecting GGNs and identifying malignancies was validated in the enrolled cohort. Additionally, the mean CT value and solid component volume were identified as independent predictors of adenocarcinoma invasiveness. Furthermore, compared with NECT scans, CECT scans did not significantly improve the performance of the AI diagnostic system or the CT parameter-based multiple logistic regression models for differentiating the invasiveness of GGNs tumors.
With the rapid development of AI technology, convolutional neural network models have been used to improve the accuracy of nodular detection and reduce false positives (28). DL algorithms can overcome the disadvantages of traditional machine learning algorithms, such as manual segmentation measurement error and doctors’ subjectivity (29,30). In this study, the end-to-end DL-based AI diagnostic system displayed excellent performance in detecting pulmonary nodules and aiding in the diagnosis of its invasiveness as evidenced by the 100% detection sensitivity of targeted lesions and the 100% alert rate for the malignancy risk of targeted lesions on NECT and artery-phase and delayed-phase CECT images. In this case, the AI diagnostic system exhibited a slightly different performance between NECT and CECT images in distinguishing the malignancy of targeted lesions, which should be further validated in larger data sets.
CT scans are of great value in differentiating GGNs, and the CT-based identification of GGNs has gradually gained more attention in clinical practice (10,31). Recently, CT features of GGN growth, including mean CT value, kurtosis, skewness, and solid component volume, have been gradually revealed. A previous study reported solid component volume to be positively correlated with the invasiveness of GGNs, which may have a certain degree of predictive value for the invasiveness of GGNs (32). Chae et al. indicated that CT-based texture analysis has a certain predictive value in distinguishing IAC lesions from noninvasive lesions in patients with GGN tumors (7). Li et al. demonstrated that quantitative analysis of CT imaging, including the maximum nodule diameter and 100th percentile on the CT number histogram, could predict IAC presenting as GGNs (33). In this study, we used automatically AI-measured CT parameters to perform multivariable regression analysis and found that the mean CT value and solid component volume of NECT images were significant predictors for differentiating PLs from invasive adenocarcinoma lesions, which can guide long-term follow-up and selective surgical management. Furthermore, the automatically calculated histogram features could help avoid subjectivity in doctors’ evaluation, reduce their workload, and increase the repeatability of measurements between medical centers, which might in turn enhance these features’ applicability in clinical practice. Of note, in addition to the automatic histogram analysis, the AI system could predict the malignancy probabilities of the detected lesions, which could be used to not only alert clinicians of suspicious lesions (100% in our study) but also potentially aid in the differentiation of lesion invasiveness. The commonly used risk stratification threshold of 50% and 75% could ideally differentiate the PL and adenocarcinoma groups, but the optimal malignancy probability threshold for differentiating invasiveness needs to be further determined.
Notably, many previous studies have focused on the differentiation of invasive and noninvasive pulmonary lesions using radiomics analysis and have achieved decent performance, with AUCs ranging from 0.72 to 0.98. Some studies utilized multiple features, such as ground-glass and solid features, as well as the SD of attenuation, mass, kurtosis, and entropy, yielding an AUC of 0.98 (7,34). Moreover, mixed density and fractal dimension, radiomics features, and mean CT values were all used for modeling, achieving an AUC of 0.76 and 0.716 for discriminating preinvasive and invasive pulmonary adenocarcinoma lesions, respectively (35,36), which is equivalent to the performance of our proposed models. Key feature abundance might greatly impact the model performance, as we noticed that the combination of 4 radiomics features with lesion shape enhanced the model performance in differentiating MIA and IAC as compared with models trained with either of them alone (37). In contrast to the studies mentioned above, we took advantage of automatically AI-calculated histogram features to develop models which, to some extent, appear suitable for clinical practice compared with the time-consuming lesion delineation procedures described in other studies. Of note, given that the AI system could segment lesions accurately, radiomics feature extraction and selection modules might be incorporated into the clinically deployed AI auxiliary system, which would allow for the enrichment of features for modeling and the improvement of model performance. Moreover, the absence of solid components in some of the included lesions might also have impacted the model performance in our study since solid component volume is one of the features for modeling. The possible influence of pure GGNs needs to be validated on a larger sample size in the near future.
Several studies have reported that energy spectrum CT iodide parameters compared to NECT provided more value for radiomic prediction in distinguishing adenocarcinoma from IAC and AIS/MIA (38,39). Dennie et al. showed that the sensitivity of the CECT in differentiating primary lung tumors from granulomatous pulmonary lesions was lower than that of NECT (40). This reduction may be attributable to the presence of a contrast agent masking the texture characteristics of the lesions (39). In our study, mean CT values were extracted from enhanced CT images, and solid component volume was significant in differentiating PLs from adenocarcinoma lesions. Notably, we found that there was no significant difference between the CECT and NECT diagnostic models in differentiating PLs from adenocarcinoma lesions, which is consistent with a previous report by Gao et al. (41). A possible explanation for this finding is the less vascular invasion of GGNs in histopathology (42). In addition, the gas content of GGNs can offset part of the increase in contrast agents. The finding further indicates that NECT scans might be sufficient to predict the invasiveness of GGNs tumors, and patients with GGN tumors might be able to avoid extra radiation exposure, allergic reaction to contrast agent, or side effects of enhanced CT scanning. Despite the slightly variable performance of the AI diagnostic system between NECT and CECT images in identifying malignancy risks, we can conclude that AI-measured CT indicators could help assess the invasiveness of GGN tumors and that NECT scans, to some extent, can facilitate an adequate performance as compared to CECT scans.
It should be noted that a recently published study by Gao et al. also used AI-based histogram analyses to predict the invasiveness of part-solid nodules (PSNs) in LUAD (24). In comparison, we focused on the GGN tumors instead of PSN tumors, as PLs and adenocarcinoma lesions can be detected as various degrees of GGNs on CT scans (5,6). Furthermore, AIS and MIA were categorized into the same group while invasive IAC was placed in a separate group in their study design. In sharp contrast, we categorized the lesion invasiveness groups according to the recent (year 2021) WHO classification of lung tumors (25), in which AAH and AIS are defined as precursor glandular lesions (PGLs; the PL group in our study), while MIA and IAC are categorized as adenocarcinoma (the invasive group). Obviously, direct comparisons of model performance were not appropriate at the time of the study. Additionally, the paired NECT and CECT scans were used in our study to investigate the additional benefit of CECT scans in the histogram feature-based prediction of GGN tumor invasiveness. Importantly, although our and Gao et al.’s studies have different designs and focuses, each uniquely contributes to realizing the potential practicability of AI-based histogram analyses in clinical practice.
There are some limitations to this study. First, the sample size was small, and it could not completely reflect the full disease characteristics of AAH, AIS, MIA, or IAC. Second, all cases were scanned with the same CT instrument, and the images were constructed via the standard B31algorithm. The use of a single instrument type and set of reconstruction parameters might limit the generalizability of the proposed model. Hence, additional cases scanned with different CT instruments and the use of different reconstruction algorithms should be implemented in future studies. Moreover, our study employed a single-center, retrospective design, and a multicenter study needs to be conducted to validate and optimize the prediction model performance.
Conclusions
This study validated the performance of an AI diagnostic system in detecting GGNs, identifying malignancy risk, and predicting invasiveness based on automatically measured CT histogram features. Particularly, the mean CT value and solid component volume were identified as independent risk factors of adenocarcinoma. In addition, NECT images, when compared with enhanced CECT scans, were found to be sufficient for the AI diagnostic system in differentially diagnosing adenocarcinoma lesions and PLs presented as GGNs.
Acknowledgments
We would like to thank Dr. Yun Shen (GE HealthCare, USA) and Ms. Shihan Zhang (Infervision Medical Technology Co., Ltd., Beijing, China) for their contributions to this work.
Funding: This work was supported by
Footnote
Reporting Checklist: The authors have completed the TRIPOD reporting checklist. Available at https://qims.amegroups.com/article/view/10.21037/qims-23-31/rc
Conflicts of Interest: All authors have completed the ICMJE uniform disclosure form (available at https://qims.amegroups.com/article/view/10.21037/qims-23-31/coif). DW is a current employee of Infervision Medical Technology Co., Ltd. The other authors have no conflicts of interest to declare.
Ethical Statement: The authors are accountable for all aspects of the work in ensuring that questions related to the accuracy or integrity of any part of the work are appropriately investigated and resolved. This study was conducted in accordance with the Declaration of Helsinki (as revised in 2013) and was reviewed and approved by the Ethics Committee of General Hospital of Ningxia Medical University (No. KYLL-2021-535). Informed consent was waived due to the retrospective nature of the study.
Open Access Statement: This is an Open Access article distributed in accordance with the Creative Commons Attribution-NonCommercial-NoDerivs 4.0 International License (CC BY-NC-ND 4.0), which permits the non-commercial replication and distribution of the article with the strict proviso that no changes or edits are made and the original work is properly cited (including links to both the formal publication through the relevant DOI and the license). See: https://creativecommons.org/licenses/by-nc-nd/4.0/.
References
- Mets OM, de Jong PA, Chung K, Lammers JJ, van Ginneken B, Schaefer-Prokop CM. Fleischner recommendations for the management of subsolid pulmonary nodules: high awareness but limited conformance - a survey study. Eur Radiol 2016;26:3840-9. [Crossref] [PubMed]
- Torre LA, Bray F, Siegel RL, Ferlay J, Lortet-Tieulent J, Jemal A. Global cancer statistics, 2012. CA Cancer J Clin 2015;65:87-108. [Crossref] [PubMed]
- Kimura K, Matsumoto S, Harada T, Morii E, Nagatomo I, Shintani Y, Kikuchi A. ARL4C is associated with initiation and progression of lung adenocarcinoma and represents a therapeutic target. Cancer Sci 2020;111:951-61. [Crossref] [PubMed]
- Yatabe Y, Borczuk AC, Powell CA. Do all lung adenocarcinomas follow a stepwise progression? Lung Cancer 2011;74:7-11. [Crossref] [PubMed]
- Xiang W, Xing Y, Jiang S, Chen G, Mao H, Labh K, Jia X, Sun X. Morphological factors differentiating between early lung adenocarcinomas appearing as pure ground-glass nodules measuring ≤10 mm on thin-section computed tomography. Cancer Imaging 2014;14:33. [Crossref] [PubMed]
- Lee HY, Lee KS. Ground-glass opacity nodules: histopathology, imaging evaluation, and clinical implications. J Thorac Imaging 2011;26:106-18. [Crossref] [PubMed]
- Chae HD, Park CM, Park SJ, Lee SM, Kim KG, Goo JM. Computerized texture analysis of persistent part-solid ground-glass nodules: differentiation of preinvasive lesions from invasive pulmonary adenocarcinomas. Radiology 2014;273:285-93. [Crossref] [PubMed]
- Fu F, Zhang Y, Wang S, Li Y, Wang Z, Hu H, Chen H. Computed tomography density is not associated with pathological tumor invasion for pure ground-glass nodules. J Thorac Cardiovasc Surg 2021;162:451-459.e3. [Crossref] [PubMed]
- Meng Y, Liu CL, Cai Q, Shen YY, Chen SQ. Contrast analysis of the relationship between the HRCT sign and new pathologic classification in small ground glass nodule-like lung adenocarcinoma. Radiol Med 2019;124:8-13. [Crossref] [PubMed]
- Niu R, Shao X, Shao X, Jiang Z, Wang J, Wang Y. Establishment and verification of a prediction model based on clinical characteristics and positron emission tomography/computed tomography (PET/CT) parameters for distinguishing malignant from benign ground-glass nodules. Quant Imaging Med Surg 2021;11:1710-22. [Crossref] [PubMed]
- Lee SM, Park CM, Goo JM, Lee HJ, Wi JY, Kang CH. Invasive pulmonary adenocarcinomas versus preinvasive lesions appearing as ground-glass nodules: differentiation by using CT features. Radiology 2013;268:265-73. [Crossref] [PubMed]
- Yagi T, Yamazaki M, Ohashi R, Ogawa R, Ishikawa H, Yoshimura N, Tsuchida M, Ajioka Y, Aoyama H. HRCT texture analysis for pure or part-solid ground-glass nodules: distinguishability of adenocarcinoma in situ or minimally invasive adenocarcinoma from invasive adenocarcinoma. Jpn J Radiol 2018;36:113-21. [Crossref] [PubMed]
- Yang Y, Li K, Sun D, Yu J, Cai Z, Cao Y, Wu J. Invasive Pulmonary Adenocarcinomas Versus Preinvasive Lesions Appearing as Pure Ground-Glass Nodules: Differentiation Using Enhanced Dual-Source Dual-Energy CT. AJR Am J Roentgenol 2019;213:W114-22. [Crossref] [PubMed]
- Liugang G, Kai X, Chunying L, Zhengda L, Jianfeng S, Tao L, Xinye N, Jianrong D. Generation of Virtual Non-Contrast CT From Intravenous Enhanced CT in Radiotherapy Using Convolutional Neural Networks. Front Oncol 2020;10:1715. [Crossref] [PubMed]
- Choi Y, Gil BM, Chung MH, Yoo WJ, Jung NY, Kim YH, Kwon SS, Kim J. Comparing attenuations of malignant and benign solitary pulmonary nodule using semi-automated region of interest selection on contrast-enhanced CT. J Thorac Dis 2019;11:2392-401. [Crossref] [PubMed]
- Liu K, Li Q, Ma J, Zhou Z, Sun M, Deng Y, Tu W, Wang Y, Fan L, Xia C, Xiao Y, Zhang R, Liu S. Evaluating a Fully Automated Pulmonary Nodule Detection Approach and Its Impact on Radiologist Performance. Radiol Artif Intell 2019;1:e180084. [Crossref] [PubMed]
- Wang Y, Yan F, Lu X, Zheng G, Zhang X, Wang C, et al. IILS: Intelligent imaging layout system for automatic imaging report standardization and intra-interdisciplinary clinical workflow optimization. EBioMedicine 2019;44:162-81. [Crossref] [PubMed]
- Chen X, Wang Z, Qi Q, Zhang K, Sui X, Wang X, Weng W, Wang S, Zhao H, Sun C, Wang D, Zhang H, Liu E, Zou T, Hong N, Yang F. A fully automated noncontrast CT 3-D reconstruction algorithm enabled accurate anatomical demonstration for lung segmentectomy. Thorac Cancer 2022;13:795-803. [Crossref] [PubMed]
- Zhang H, Cheng Y, Chen Z, Cong X, Kang H, Zhang R, Guo X, Liu M. Clot burden of acute pulmonary thromboembolism: comparison of two deep learning algorithms, Qanadli score, and Mastora score. Quant Imaging Med Surg 2022;12:66-79. [Crossref] [PubMed]
- Wang M, Xia C, Huang L, Xu S, Qin C, Liu J, et al. Deep learning-based triage and analysis of lesion burden for COVID-19: a retrospective study with external validation. Lancet Digit Health 2020;2:e506-15. [Crossref] [PubMed]
- Zhang X, Wang D, Shao J, Tian S, Tan W, Ma Y, Xu Q, Ma X, Li D, Chai J, Wang D, Liu W, Lin L, Wu J, Xia C, Zhang Z. A deep learning integrated radiomics model for identification of coronavirus disease 2019 using computed tomography. Sci Rep 2021;11:3938. [Crossref] [PubMed]
- Zimmerman KC, Sharma G, Parchur AK, Joshi A, Schmidt TG. Experimental investigation of neural network estimator and transfer learning techniques for K-edge spectral CT imaging. Med Phys 2020;47:541-51. [Crossref] [PubMed]
- Zhang T, Pu XH, Yuan M, Zhong Y, Li H, Wu JF, Yu TF. Histogram analysis combined with morphological characteristics to discriminate adenocarcinoma in situ or minimally invasive adenocarcinoma from invasive adenocarcinoma appearing as pure ground-glass nodule. Eur J Radiol 2019;113:238-44. [Crossref] [PubMed]
- Gao J, Qi Q, Li H, Wang Z, Sun Z, Cheng S, Yu J, Zeng Y, Hong N, Wang D, Wang H, Yang F, Li X, Li Y. Artificial-intelligence-based computed tomography histogram analysis predicting tumor invasiveness of lung adenocarcinomas manifesting as radiological part-solid nodules. Front Oncol 2023;13:1096453. [Crossref] [PubMed]
- Nicholson AG, Tsao MS, Beasley MB, Borczuk AC, Brambilla E, Cooper WA, Dacic S, Jain D, Kerr KM, Lantuejoul S, Noguchi M, Papotti M, Rekhtman N, Scagliotti G, van Schil P, Sholl L, Yatabe Y, Yoshida A, Travis WD. The 2021 WHO Classification of Lung Tumors: Impact of Advances Since 2015. J Thorac Oncol 2022;17:362-87. [Crossref] [PubMed]
- Zhang T, Pu XH, Yuan M, Zhong Y, Li H, Wu JF, Yu TF. Histogram analysis combined with morphological characteristics to discriminate adenocarcinoma in situ or minimally invasive adenocarcinoma from invasive adenocarcinoma appearing as pure ground-glass nodule. Eur J Radiol 2019;113:238-44. [Crossref] [PubMed]
- Son JY, Lee HY, Kim JH, Han J, Jeong JY, Lee KS, Kwon OJ, Shim YM. Quantitative CT analysis of pulmonary ground-glass opacity nodules for distinguishing invasive adenocarcinoma from non-invasive or minimally invasive adenocarcinoma: the added value of using iodine mapping. Eur Radiol 2016;26:43-54. [Crossref] [PubMed]
- Monkam P, Qi S, Xu M, Han F, Zhao X, Qian W. CNN models discriminating between pulmonary micro-nodules and non-nodules from CT images. Biomed Eng Online 2018;17:96. [Crossref] [PubMed]
- Beig N, Khorrami M, Alilou M, Prasanna P, Braman N, Orooji M, Rakshit S, Bera K, Rajiah P, Ginsberg J, Donatelli C, Thawani R, Yang M, Jacono F, Tiwari P, Velcheti V, Gilkeson R, Linden P, Madabhushi A. Perinodular and Intranodular Radiomic Features on Lung CT Images Distinguish Adenocarcinomas from Granulomas. Radiology 2019;290:783-92. [Crossref] [PubMed]
- Zhu X, Dong D, Chen Z, Fang M, Zhang L, Song J, Yu D, Zang Y, Liu Z, Shi J, Tian J. Radiomic signature as a diagnostic factor for histologic subtype classification of non-small cell lung cancer. Eur Radiol 2018;28:2772-8. [Crossref] [PubMed]
- Luo T, Xu K, Zhang Z, Zhang L, Wu S. Radiomic features from computed tomography to differentiate invasive pulmonary adenocarcinomas from non-invasive pulmonary adenocarcinomas appearing as part-solid ground-glass nodules. Chin J Cancer Res 2019;31:329-38. [Crossref] [PubMed]
- Xi J, Yin J, Liang J, Zhan C, Jiang W, Lin Z, Xu S, Wang Q. Prognostic Impact of Radiological Consolidation Tumor Ratio in Clinical Stage IA Pulmonary Ground Glass Opacities. Front Oncol 2021;11:616149. [Crossref] [PubMed]
- Li Q, Fan L, Cao ET, Li QC, Gu YF, Liu SY. Quantitative CT analysis of pulmonary pure ground-glass nodule predicts histological invasiveness. Eur J Radiol 2017;89:67-71. [Crossref] [PubMed]
- Wu G, Woodruff HC, Shen J, Refaee T, Sanduleanu S, Ibrahim A, Leijenaar RTH, Wang R, Xiong J, Bian J, Wu J, Lambin P. Diagnosis of Invasive Lung Adenocarcinoma Based on Chest CT Radiomic Features of Part-Solid Pulmonary Nodules: A Multicenter Study. Radiology 2020;297:451-8. [Crossref] [PubMed]
- Xue X, Yang Y, Huang Q, Cui F, Lian Y, Zhang S, Yao L, Peng W, Li X, Pang P, Yan J, Chen F. Use of a Radiomics Model to Predict Tumor Invasiveness of Pulmonary Adenocarcinomas Appearing as Pulmonary Ground-Glass Nodules. Biomed Res Int 2018;2018:6803971. [Crossref] [PubMed]
- Zhao W, Xu Y, Yang Z, Sun Y, Li C, Jin L, Gao P, He W, Wang P, Shi H, Hua Y, Li M. Development and validation of a radiomics nomogram for identifying invasiveness of pulmonary adenocarcinomas appearing as subcentimeter ground-glass opacity nodules. Eur J Radiol 2019;112:161-8. [Crossref] [PubMed]
- Weng Q, Zhou L, Wang H, Hui J, Chen M, Pang P, Zheng L, Xu M, Wang Z, Ji J. A radiomics model for determining the invasiveness of solitary pulmonary nodules that manifest as part-solid nodules. Clin Radiol 2019;74:933-43. [Crossref] [PubMed]
- Hong D, Xu K, Zhang L, Wan X, Guo Y. Radiomics Signature as a Predictive Factor for EGFR Mutations in Advanced Lung Adenocarcinoma. Front Oncol 2020;10:28. [Crossref] [PubMed]
- Phillips I, Ajaz M, Ezhil V, Prakash V, Alobaidli S, McQuaid SJ, South C, Scuffham J, Nisbet A, Evans P. Clinical applications of textural analysis in non-small cell lung cancer. Br J Radiol 2018;91:20170267. [Crossref] [PubMed]
- Dennie C, Thornhill R, Sethi-Virmani V, Souza CA, Bayanati H, Gupta A, Maziak D. Role of quantitative computed tomography texture analysis in the differentiation of primary lung cancer and granulomatous nodules. Quant Imaging Med Surg 2016;6:6-15. [Crossref] [PubMed]
- Gao C, Xiang P, Ye J, Pang P, Wang S, Xu M. Can texture features improve the differentiation of infiltrative lung adenocarcinoma appearing as ground glass nodules in contrast-enhanced CT? Eur J Radiol 2019;117:126-31. [Crossref] [PubMed]
- Lim HJ, Ahn S, Lee KS, Han J, Shim YM, Woo S, Kim JH, Yie M, Lee HY, Yi CA. Persistent pure ground-glass opacity lung nodules ≥ 10 mm in diameter at CT scan: histopathologic comparisons and prognostic implications. Chest 2013;144:1291-9. [Crossref] [PubMed]