Role of combined clinical-radiomics model based on contrast-enhanced MRI in predicting the malignancy of breast non-mass enhancements without an additional diffusion-weighted imaging sequence
Introduction
The fifth edition of the American College of Radiology (ACR) Breast Imaging-Reporting and Data System (BI-RADS) Atlas divides the possibility of breast malignancy into 7 categories (1). There is a wide range of malignancy rates (from >2% to <95%) for BI-RADS category 4 lesions, which may result in overlap between benign and malignant assessment. Although BI-RADS 4 lesions are further subdivided into 4a, 4b, and 4c, a biopsy or surgical resection should be performed for patients with a BI-RADS category of 4 and followed by a pathological examination. However, there are many benign pathologic results for BI-RADS 4 lesions (2), and of these, non-mass enhancements (NMEs) in breast magnetic resonance (MR) images are the leading cause of false-positive diagnoses and unnecessary biopsies (3,4). In addition, NME is the most common finding of ductal carcinoma in situ (DCIS) on contrast-enhanced MR imaging (CE-MRI) (5). DCIS may be underestimated preoperatively and only confirmable with the invasive component of surgery (6). The presence of an invasive component may influence the choice of treatment. It should be noted that both pure DCIS and invasive cancer can appear as NME (7). To make noninvasive and accurate differentiation between pure DCIS and invasive cancer preoperatively would be of great benefit for treatment planning including lymph node biopsy. However, this possibility remains a considerable challenge for both radiologists and pathologists because NME lesions have poorly defined boundaries and are mixed with normal fibroglandular tissue (8).
In our previous study, we developed a combined diagnostic model based on timeintensity curve (TIC) types and a radiomics signature on CE-MRI (9). Even without an additional diffusion-weighted imaging (DWI) sequence, this model had a high diagnostic ability in differentiation. However, several studies have reported that the apparent diffusion coefficient (ADC) is valuable for the reclassification of BI-RADS 4 lesions (8), is able to detect the potential invasive components in cases with DCIS (10), and can be used to predict treatment responses and breast cancer prognoses (11). In this context, it remains unclear whether the combined model without additional ADC values has a comparable or superior performance compared to ADC.
Therefore, in this study, we compared the diagnostic value of the TIC-radiomics signature combined model derived from contrast-enhanced MRI and the minimal ADC values in distinguishing benign and malignant lesions among the BI-RADS 4 lesions. We also analyzed the diagnostic value of the radiomics signature in distinguishing DCIS and invasive breast carcinoma. In addition, as the prediction of estrogen receptor (ER), progesterone receptor (PR), epidermal growth factor (HER2), and Ki-67 expression biomarkers would be of substantive value for ascertaining the effects of neoadjuvant chemotherapies (12), we further explored the value of the combined model in predicting the expression level of biomarkers. We present this article in accordance with the STARD reporting checklist (available at https://qims.amegroups.com/article/view/10.21037/qims-22-1199/rc).
Methods
Patients
This retrospective study was conducted in accordance with the Declaration of Helsinki (as revised in 2013) and approved by the Ethics Committee of Tongji Hospital. Informed consent was waived due to the retrospective nature of the study. This study reviewed 4,519 consecutive patients who underwent breast DCE-MRI in our hospital between December 2017 and November 2021 (including the patients examined between December 2017 and April 2021 described in our previous study). As previously reported (9), the inclusion criteria included (I) lesions presenting as NMEs in DCE-MRI images, (II) complete clinical and pathological data, (III) the absence of lactation or pregnancy, and (IV) the absence of breast implants or previous treatments. Lesions with (I) maximal a diameter less than 5 mm, (II) apparent hemorrhage after biopsy, and (III) poor image quality were excluded. With regard to our previous study, 44 patients with 45 NME lesions were newly included in this study. Ultimately, 364 NME lesions from 343 female patients (mean age, 45.6±10.6 years) were enrolled in the study. Pathological data were obtained from hospital medical records as were the immunohistochemistry results within 1 week after surgery. The flowchart of patient enrollment is presented in Figure 1.
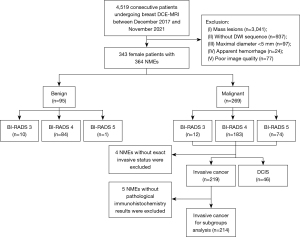
MRI protocol and interpretation
All MR images were obtained using the protocols described in our previous study (9). The imaging parameters for the multi-b value DWI with readout-segmented echo-planar imaging (RESOLVE DWI) sequence was as follows: repetition time (TR) =5,000 ms; echo time (TE) =70 ms; field of view (FOV) =169×280 mm2; matrix size =114×188; slice thickness =5.0 mm; readout segment =5, average =1; diffusion gradient mode =3-scan trace; and b values =0, 50, and 1,000 s/mm2. DCE-MR images were acquired using a time-resolved angiography with stochastic trajectories and volume-interpolated breath-hold examination (TWIST-VIBE) sequence with the following parameters: TR =5.24 ms, TE =2.46 ms, FOV =260×320 mm2, matrix size =182×320, slice thickness =1.5 mm without gap, flip angle =10°, and temporal resolution =5.74 s/phase. The contrast medium (Omniscan, GE HealthCare, Chicago, IL, USA) was intravenously injected with a power injector at the end of the third dynamic phase at a dose of 0.1 mmol/kg body weight and an injection rate of 2.5 mL/s, which was followed by a 20-mL saline flush.
Two radiologists (L.M.X. and Z.L.Y. with 15 and 3 years of experience in interpreting breast MRI, respectively) performed a blinded and independent analysis of the clinicopathological data and the breast DCE-MRI images of 343 patients. The morphologic features (internal enhancement and distribution), TICs, and pathological features based on immunohistochemistry results were recorded for each NME lesion. The maximal diameter of each lesion was measured 3 times using the DCE-MRI images with multiplanar reformation (MPR), and the average value was recorded. The type of TIC for each case was drawn using the Mean Curve software in the workstation (Siemens Healthcare, Germany) based on DCE-MRI with a region of interest (ROI) of approximately 0.2–0.4 cm2 placed on each slice at the brightest part of the lesions on images obtained in the early phase after the contrast injection. The TIC types were classified as persistent type (I), plateau type (II), and washout type (III). We recorded the high-level TIC curve types when different types were present in each lesion. The lowest ADC value in the darkest areas of the ADC maps was defined as the minimal ADC value, which was confirmed by agreement of the 2 radiologists. The lesions on the enhancement images were copied onto the ADC map if visible lesions were not observed on the DWI or the corresponding ADC maps, and multiple ROIs were placed to cover the whole area of the lesion (Figure 2). Based on the morphologic (distribution and internal enhancement patterns) and kinetic features, the NME lesions were classified by consensus according to the fifth edition of BI-RADS (1). Specific judging criteria are presented in Table 1. NMEs were classified as BI-RADS 2 if they fully met the criteria of benign characteristics, as BI-RADS 3 if they only met 1 criterion of malignant characteristics, as BI-RADS 4 if they met 2 or more criteria of malignant characteristics, and as BI-RADS 5 if they fully met the criteria of malignant characteristics. In this study, we considered BI-RADS 2 or 3 NMEs as benign lesions and BI-RADS 4 or 5 NMEs as malignant lesions.
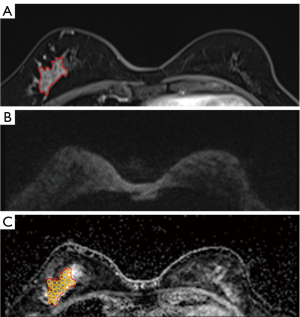
Table 1
Characteristics | Malignant characteristics | Benign characteristics |
---|---|---|
DWI | ||
ADC value (×10−6 mm2/s) | ≤1,250 | >1,250 |
Boundaries | Unclear | Clear |
Internal intensities | Heterogenous | Homogenous |
DCE-MRI | ||
TIC types | Washout/plateau | Persistent |
Distribution patterns | Segmental/linear | Diffuse/regional/focal |
Internal enhancement patterns | Heterogenous | Homogenous |
NME, non-mass enhancement; DWI, diffusion-weighted imaging; ADC, apparent diffusion coefficient; DCE-MRI, dynamic contrast-enhanced magnetic resonance imaging; TIC, time-signal intensity curve.
As described in the previous study, the radiomics workflow included ROI segmentation, feature extraction, feature selection, and radiomics score construction. Using Pyradiomics open-source software, we determined 6 features: morphological features, first-order features, and texture features including original_shape_SurfaceVolumeRatio, wavelet.HLL_glcm_Idn, original_firstorder_Skewness, wavelet.LLH_glcm_Idmn, original_glszm_SmallAreaEmphasis, and wavelet.LLL_firstorder_Kurtosis. Radiomics score (Radscore) was calculated with a linear equation of the coefficients for all selected features.
Diagnostic performance evaluation
Each corresponding measurement of all 364 NMEs was entered into following the logistic regression equation of the combined model: nomogram score = −1.774 + 0.0 × 0 (TIC type I) + 1.662 × 1 (TIC type II) + 2.941 × 1 (TIC type III) + 1.224 × radiomics score. We used the Sigmoid function (13) [S(x)=1/(1+e−x)] to convert nomogram score (x) into malignancy risk [S(x)] and defined the malignancy risk as nomogram score (NS) value, which was on a scale of 0–1 and favorable for statistical analysis. NME lesions were reclassified according to the cutoff value of NS and the minimal ADC. Histopathology was used as the reference standard. The NME with an NS value below the cutoff value was considered to be a benign lesion and was reclassified as BI-RADS 3, and that above the value was considered to be malignant and was reclassified as BI-RADS 5. The NME with ADC value below the cutoff value was considered to be malignant lesion and was reclassified as BI-RADS 5, and that above the value was considered to benign and reclassified as BI-RADS 3.
NME lesions classified as BI-RADS 4 category by the initial conventional imaging diagnosis were selected. The diagnostic performance of the NS values and the minimal ADC values were compared for downgrading the BI-RADS 4 category NMEs. Then, the malignant lesions were classified as invasive ductal carcinoma (IDC) or DCIS. The performances of the NS and the minimal ADC values in detecting the invasive components were evaluated via subgroup analysis. To verify the effectiveness of the minimal ADC values embedded in the combined model, we conducted an ablation experiment. Finally, the distribution of the NS and the minimal ADC values among various molecular biomarkers, including the expression of ER, PR, HER2 status, and Ki-67 of the malignant lesions, were evaluated.
Statistical analysis
SPSS 24.0. (IBM, Armonk, NY, USA), MedCalc Software version 20.0.4 (MedCalc Software, Mariakerke, Belgium), and GraphPad Prism software (version 7.0; GraphPad Software Inc., San Diego, CA, USA) were used for the statistical analysis. Continuous variables with normal distribution are expressed as mean ± standard deviation, non-normally distributed continuous variables are expressed as median [interquartile range (IQR)], and categorical variables are expressed as percentages. The Student t-test was used to compare the normally distributed continuous variables, the Mann-Whitney test was used to compare nonparametric data, and Wilcoxon rank-sum test was used for ordinal variables.
The discriminative ability of the NS and the minimal ADC values among distinct groups were analyzed using the area under the receiver operating characteristic (ROC) curve (AUC) values. The optimal cutoff values were obtained with Youden index, and AUCs were compared using the DeLong test. The McNemar test was used to compare the diagnostic performances for the BI-RADS 4 category NMEs before and after the assistance of the NS and the minimal ADC values.
The 95% confidence intervals (95% CIs) for the AUCs, sensitivity, specificity, and positive predictive value (PPV) were calculated using the Wilson score method. A 2-tailed P value less than 0.05 was considered statistically significant.
Results
Lesion characteristics
Table 2 shows the details of the 364 breast lesions examined in this study, including 269 NMEs pathologically confirmed as malignant and 95 NMEs confirmed as benign. The 269 malignant lesions included 46 (17.1%) case of DCIS, 219 (81.4%) of IDC or invasive lobular carcinoma (ILC) of no special type, 2 of mucinous carcinoma, and 2 of solid papillary carcinoma. The 95 benign lesions included 81 (85.2%) cases of adenosis, 1 (1.0%) of fibroadenoma/fibroadenomatous change, 2 (2.1%) of papilloma, and 11 (11.6%) of chronic inflammation. Additionally, 12 malignant lesions were classified as BI-RADS category 3 by the initial diagnosis, and 85 benign lesions were classified as BI-RADS 4 or 5 (Table 2). Of all the 364 NME lesions, 183 malignant and 84 benign breast lesions classified as BI-RADS 4 by the initial diagnosis were enrolled for differential analysis. As shown in the flowchart in Figure 1, other malignant lesions classified as BI-RADS 3 and 5 were also enrolled for differential analysis between DCIS and invasive cancer and further immunohistochemistry analysis. The median maximum diameters of DCIS and invasive cancer were 43.1 (IQR, 30.3–56.1) mm and 44.9 (IQR, 32.0–59.7) mm, respectively.
Table 2
Characteristics | Results | |
---|---|---|
Malignant (n=269) | Benign lesions (n=95) | |
Mean age (years) | 46.9±10.4 | 41.4±10.5 |
Pathology results | ||
DCIS | 46 (17.1) | NA |
IDC/ILC | 219 (81.4) | NA |
Mucinous carcinoma | 2 (0.7) | NA |
Solid papillary carcinoma | 2 (0.7) | |
Adenosis | NA | 81 (85.2) |
Fibroadenoma/fibroadenomatous change | NA | 1 (1.0) |
Papilloma | NA | 2 (2.1) |
Chronic inflammation | NA | 11 (11.6) |
BI-RADS category | ||
2 | 0 (0) | 0 (0) |
3 | 12 (4.5) | 10 (10.5) |
4 | 183 (68.0) | 84 (88.4) |
5 | 74 (27.5) | 1 (1.1) |
The data in parentheses are expressed as the number (%) of NMEs. The percentages may not add up to 100% due to rounding. The mean age is represented as the mean ± standard deviation. DCIS, ductal carcinoma in situ; IDC, invasive ductal carcinoma; ILC, invasive lobular carcinoma; BI-RADS, Breast Imaging-Reporting and Data System; NME, non-mass enhancement.
The detailed results of the minimal ADC and NS values among the different groups are shown in Table 3. In the group of NMEs classified as BI-RADS 4 by the initial diagnosis, the minimal ADC values for the malignant lesions were significantly lower than those of the benign lesions [(788.2±180.0)×10−6vs. (878.6±188.5)×10−6 mm2/s; P<0.001], whereas the NS values for the malignant lesions were significantly higher than those of the benign lesions [0.938 (0.843–0.972) vs. 0.346 (0.176–0.712); P<0.001]. The minimal ADC values for the IDC were significantly lower than those of DCIS [(855.6±182.5)×10−6vs. (745.3±151.8)×10−6 mm2/s; P<0.001], whereas the NS values of invasive cancers were significantly higher than those of DCIS [0.906 (0.643–0.952) vs. 0.945 (0.859–0.976); P<0.001] (Table 3).
Table 3
Parameter | Number | The minimal ADC (×10−6 mm2/s) | NS value | |||
---|---|---|---|---|---|---|
Mean ± SD | P value | Median (IQR) | P value | |||
BI-RADS 4 NME | <0.001 | <0.001 | ||||
Malignant | 183 | 788.2±180.0 | 0.938 (0.843–0.972) | |||
Benign | 84 | 878.6±188.5 | 0.346 (0.176–0.712) | |||
Malignant histological type | <0.001 | <0.001 | ||||
IDC/ILC | 219 | 745.3±151.8 | 0.945 (0.859–0.976) | |||
DCIS | 46 | 855.6±182.5 | 0.906 (0.643–0.952) | |||
Invasive carcinoma | ||||||
ER | 0.246 | 0.505 | ||||
Positive | 144 | 734.9±151.8 | 0.944 (0.862–0.976) | |||
Negative | 70 | 760.7±153.1 | 0.949 (0.855–0.981) | |||
PR | 0.021 | 0.296 | ||||
Positive | 136 | 725.2±139.8 | 0.949 (0.860–0.976) | |||
Negative | 78 | 775.1±168.3 | 0.949 (0.873–0.981) | |||
HER2 | 0.010 | 0.535 | ||||
Positive | 83 | 776.9±139.0 | 0.943 (0.878–0.979) | |||
Negative | 131 | 722.1±157.0 | 0.945 (0.859–0.978) | |||
Ki-67 | 0.212 | 0.002 | ||||
High | 151 | 733.4±149.5 | 0.954 (0.885–0.979) | |||
Low | 63 | 767.3±157.6 | 0.915 (0.840–0.953) |
ADC, apparent diffusion coefficient; NS, nomogram score; SD, standard deviation; IQR, interquartile range; BI-RADS, Breast Imaging-Reporting and Data System; NME, non-mass enhancement; IDC, invasive ductal carcinoma; ILC, invasive lobular carcinoma; DCIS, ductal carcinoma in situ; ER, estrogen receptor; PR, progesterone receptor; HER2, human epidermal growth factor receptor 2.
The subgroup analysis of the malignant lesions (n=214) demonstrated significantly higher NS values for the breast lesions with high Ki-67 expression compared with those with low Ki-67 expression (P=0.002; Table 3). However, significant differences were not observed between the minimal ADC values for the high and low Ki-67 expression groups (P=0.212) (Table 3), nor were they observed for NS values between groups with different ER, PR, and HER2 expression (all P values >0.05). NMEs with negative HER2 or positive PR expression showed significantly lower minimal ADC values compared with the NMEs with positive HER2 or negative PR expression (P<0.05; Table 3).
Among the 267 BI-RADS 4 NMEs, 68.5% (183/267) were malignant, and 31.4% (84/267) were benign. The mean maximal diameter of the malignant lesions was significantly larger than the benign lesions [43.1 (30.0–56.2) vs. 26.7 (17.1–45.3); P<0.001]. The characteristics of internal enhancement, morphologic distribution, and TIC types based on the breast DCE-MRI analysis showed statistically significant differences (all P values <0.05) between the malignant and benign lesions (Table 4).
Table 4
Characteristic | Malignant lesions (n=183) | Benign lesions (n=84) | P value |
---|---|---|---|
The maximal diameter (mm) [median (IQR)] | 43.1 (30.0–56.2) | 26.7 (17.1–45.3) | <0.001 |
Internal enhancement | 0.015 | ||
Homogeneous | 10 (5.5) | 16 (19.0) | |
Heterogeneous | 118 (64.5) | 48 (57.1) | |
Clumped | 45 (24.6) | 18 (21.4) | |
Clustered ring | 10 (5.5) | 2 (2.4) | |
Distribution | <0.001 | ||
Focal | 20 (10.9) | 22 (26.2) | |
Linear | 4 (2.2) | 9 (10.7) | |
Segmental | 50 (27.3) | 18 (21.4) | |
Regional | 68 (37.2) | 26 (31.0) | |
Multiple regions | 32 (17.5) | 8 (9.5) | |
Diffuse | 9 (4.9) | 1 (1.2) | |
TIC | <0.001 | ||
Persistent | 16 (8.7) | 41 (48.8) | |
Plateau | 104 (56.8) | 33 (39.3) | |
Washout | 63 (34.4) | 10 (11.9) |
Unless otherwise noted, the data in parentheses are expressed as the number (%) of NMEs. The percentages may not add up to 100% due to rounding. NME, non-mass enhancement; BI-RADS, Breast Imaging-Reporting and Data System; DCE-MRI, dynamic contrast-enhanced magnetic resonance imaging; TIC, time-signal intensity curve.
Diagnostic performance
For BI-RADS 4 NMEs classified by the initial diagnosis, the NS values showed better discrimination of the malignant and benign lesions (AUC =0.843; 95% CI: 0.789–0.896) compared with the minimal ADC values (AUC =0.662; 95% CI: 0.590–0.735) (P<0.001) based on the ROC curve analysis. The ablation experiment showed that the diagnostic performance of the combined NS-ADC model was significantly better than that of the minimal ADC values alone (P<0.001) and comparable to that of NS values alone (P=0.767). Neither parameter showed satisfactory performance in discriminating DCIS from invasive breast cancer presenting as NMEs (all AUCs <0.7). However, the diagnostic performance of the combined NS-ADC model (AUC =0.731; 95% CI: 0.655–0.806) was higher than that of the NS values alone and was comparable to that of the minimal ADC values (Table 5; Figure 3).
Table 5
Comparison | AUC (95% CI) | P value | |||||
---|---|---|---|---|---|---|---|
ADC | NS | NS-ADC | ADC vs. NS | ADC vs. NS-ADC |
NS vs. NS-ADC |
||
Benign vs. malignant (BI-RADS 4) | 0.662 (0.590–0.735) | 0.843 (0.789–0.896) | 0.845 (0.792–0.898) | <0.001 | <0.001 | 0.767 | |
DCIS vs. IDC/ILC | 0.691 (0.607–0.775) | 0.690 (0.609–0.771) | 0.731 (0.655–0.806) | 0.990 | 0.440 | 0.008 |
ROC, receiver operating characteristic curve; AUC, area under curve; CI, confidence interval; ADC, the minimal apparent diffusion coefficient value; NS, nomogram scores; NS-ADC, the combined NS and ADC model; BI-RADS, Breast Imaging–Reporting and Data System; DCIS, ductal carcinoma in situ; IDC, invasive ductal carcinoma; ILC, invasive lobular carcinoma.
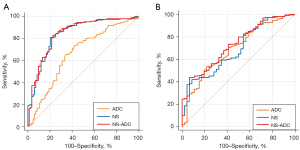
The minimal ADC value of 829.5×10−6 mm2/s and NS values of 0.772 were selected as the best cutoff values for the differential diagnosis of NMEs based on the ROC analysis. The final sensitivity, specificity, PPV, NPV, and accuracy values based on the cutoff values for reclassifying BI-RADS 4 NMEs for DWI were 65.6%, 65.5%, 80.5%, 46.6%, and 65.5%, respectively, while for the NS values, they were 80.3%, 79.8%, 89.6%, 65.0%, and 80.1%, respectively (McNemar test, both P values <0.001).
Of the 84 benign lesions, 55 (65.5%) categorized as BI-RADS 4 were accurately classified as nonsuspicious lesions based on the cutoff minimal ADC value. Therefore, the false-positive rate was 34.5%, with 29 of 84 NMEs misdiagnosed as malignant, including 22 diagnosed as adenosis, 2 as papilloma, and 5 as chronic inflammation lesions. Furthermore, among the 183 malignant lesions categorized as BI-RADS 4, 63 were classified as nonsuspicious lesions based on the cutoff value, including as 46 IDC, 2 classified as mucinous carcinoma, 1 as solid papillary carcinoma, and 14 as DCIS lesions.
Of the 84 benign lesions, 67 (79.8%) categorized as BI-RADS 4 were accurately classified as nonsuspicious lesions based on the NS cutoff value. Therefore, the false-positive rate was 20.2%, with 17 of 84 NMEs misdiagnosed as malignant, including 14 adenosis and 3 chronic inflammation cases. Furthermore, among the 183 malignant lesions categorized as BI-RADS 4, 36 were classified as nonsuspicious lesions, including 18 classified as IDC, 2 as mucinous carcinoma, 1 as ILC, and 15 as DCIS lesions.
Discussion
The results of our study showed that the diagnostic performance of the combined TIC–radiomics model was significantly better than that of DWI in reclassifying the BI-RADS 4 NMEs into malignant and benign lesions (sensitivity: 80.3% vs. 65.6%; specificity: 79.8% vs. 65.5%; accuracy: 80.1% vs. 65.5%; AUC: 0.843 vs. 0.662). The differential diagnostic performance of the combined model and DWI between DCIS and invasive cancer was comparable but not sufficiently high (AUC: 0.690 vs. 0.691).
DWI is a functional MRI method that is widely used in clinical practice (8,11,14,15). Minimal ADC values show good reliability and reproducibility as a quantitative imaging biomarker and can reflect the dense cellularity and heterogeneity of breast tissues (16). Partridge et al. showed that ADC values based on DWI could discriminate between malignant and benign breast lesions as well as DCIS and invasive cancer, thereby reducing the numbers of false positives based on the conventional DCE-MRI analysis (14). However, the differential diagnosis performance of ADC values for NME lesions remains controversial. Avendano et al. reported that 30% of NMEs on DCE-MRI were not visible on the ADC maps (17). ROIs of these lesions on the enhancement images should be pasted on the ADC maps to measure the ADC values. However, whether it is a required step depends on the additional utility of the ADC values for differential diagnosis. In this study, the diagnostic performance of the combined NS-ADC model for BI-RADS 4 NMEs was significantly better than that of the minimal ADC values alone—demonstrating a higher specificity (79.8%)—and comparable to that of the NS values alone (P=0.767). Our study demonstrated that the combined TIC-radiomics model can significantly reduce the false-positive rate and avoid unnecessary biopsies. Moreover, our approach only requires DCE-MRI images, with no additional DWI sequences being required.
The MRI features of mucinous breast carcinoma overlap with benign lesions, including cysts and fibroadenoma, thereby increasing the risk of misinterpretation (18). In our study, both the NS value and minimal ADC value failed to classify mucinous breast carcinoma lesions as malignant. Therefore, there is a need for better imaging methods to distinguish mucinous breast carcinoma from benign and other malignant breast cancer lesions.
Ki-67 is the most commonly used biomarker for estimating the proliferation index of breast cancer cells and is an independent prognostic biomarker in early-stage breast cancer (19). Our results suggested that the NS values of NMEs may be related to the expression level of Ki-67. However, further investigation is required in this regard. Our study also showed that the lower minimal ADC values correlated with positive PR and negative HER2 expression. However, HER2–positive breast cancers are more aggressive (20,21). The larger ADC value reported for these lesions compared to HER2 negative ones was unexpected since a more aggressive cancer should present a higher cell density and thus a lower ADC value. These conflicting results need further interpretation based on study of a larger cohort.
The differential diagnosis between DCIS and invasive breast cancer is challenging (22-25). Our study showed that the minimal ADC values for invasive breast cancer NMEs were significantly lower than the minimal ADC values of DCIS. These results were consistent with previous reports (6,10,26). However, ROC curve analysis did not demonstrate satisfactory performance for either minimal ADC values or the NS values in differentiating DCIS from invasive breast carcinoma NMEs (AUC <0.7). It is worth noting that the diagnostic performance of the combined NS-ADC model (AUC =0.731; 95% CI: 0.655–0.806) was higher than that of the NS values alone and was comparable to the minimal ADC values. Therefore, the diagnostic value of DWI was higher than the combined TIC-radiomics model in discriminating DCIS from invasive cancer. The radiomics signature in this study was optimized to discriminate between benign and malignant lesions but not to discriminate between DCIS and invasive breast carcinoma. Thus, a new radiomics score possibly using different radiomics features should be defined to solve this problem.
Our study has a few limitations. First, as we employed a retrospective design, a certain degree of selection bias might be inevitable. Second, this study included a small sample of benign lesions. This was because the patients enrolled in this study underwent breast MRI for second-look examinations of suspicious lesions or preoperative staging for known breast cancer. Third, 2 radiologists performed morphologic assessments and other MRI parameter measurements in consensus, precluding validation by interobserver repeatability. Fourth, we chose the MRI images from the early phase of disease to assess the maximal diameter and tumor morphology and avoid background parenchymal enhancements. However, some NMEs with progressive enhancement could not be evaluated accurately. Fifth, the effects of distortion in DWI was not considered in this study. Emerging DWI technological developments may improve the accuracy of NME discrimination. Sixth, factors such as histological grading and lymphovascular invasion are critical for describing breast cancer lesions, but we did not obtain this information during the study. Finally, the sensitivity of the radiomics analysis might be affected by certain imaging parameters, such as resolution, accelerated imaging, view sharing, scanner, and so on; thus, further well-designed multicenter studies are required.
Conclusions
The combined TIC–radiomics model based on CE-MRI showed better performance in downgrading the BI-RADS 4 NMEs as compared with DWI. The diagnostic model could achieve good performance in differentiating BI-RADS 4 NMEs without an additional DWI sequence. DWI may be of some value in differentiating DCIS and invasive cancer. However, further investigations and methodologic improvements are necessary to discriminate between DCIS and invasive breast cancer.
Acknowledgments
Funding: This study was financially supported by
Footnote
Reporting Checklist: The authors have completed the STARD reporting checklist. Available at https://qims.amegroups.com/article/view/10.21037/qims-22-1199/rc
Conflicts of Interest: All authors have completed the ICMJE uniform disclosure form (available at https://qims.amegroups.com/article/view/10.21037/qims-22-1199/coif). ZY reports financial support from the National Natural Science Foundation of China (No. 82001892). LX reports financial support from the National Natural Science Foundation of China (No. 82272109). WL reports a full-time employee of Julei Technology Company from 2021 to 2023, during the conduct of the study. XY reports a full-time employee of Siemens Healthcare Ltd. from 2021 to 2023, during the conduct of the study. TY reports a full-time employee of Siemens Healthineers Ltd. from 2021 to 2023, during the conduct of the study. The other authors have no conflicts of interest to declare.
Ethical Statement: The authors are accountable for all aspects of the work in ensuring that questions related to the accuracy or integrity of any part of the work are appropriately investigated and resolved. This retrospective study was conducted in accordance with the Declaration of Helsinki (as revised in 2013) and approved by the Ethics Committee of Tongji Hospital. Informed consent was waived due to the retrospective nature of the study.
Open Access Statement: This is an Open Access article distributed in accordance with the Creative Commons Attribution-NonCommercial-NoDerivs 4.0 International License (CC BY-NC-ND 4.0), which permits the non-commercial replication and distribution of the article with the strict proviso that no changes or edits are made and the original work is properly cited (including links to both the formal publication through the relevant DOI and the license). See: https://creativecommons.org/licenses/by-nc-nd/4.0/.
References
- ACR BI-RADS® ATLAS — BREAST MRI. Available online: https://www.acr.org/-/media/ACR/Files/RADS/BI-RADS/MRI-Reporting.pdf. 2013.
- Li J, Zheng H, Cai W, Wang Y, Zhang H, Liao M. Subclassification of BI-RADS 4 Magnetic Resonance Lesions: A Systematic Review and Meta-Analysis. J Comput Assist Tomogr 2020;44:914-20. [Crossref] [PubMed]
- Baltzer PA, Benndorf M, Dietzel M, Gajda M, Runnebaum IB, Kaiser WA. False-positive findings at contrast-enhanced breast MRI: a BI-RADS descriptor study. AJR Am J Roentgenol 2010;194:1658-63. [Crossref] [PubMed]
- Marino MA, Avendano D, Sevilimedu V, Thakur S, Martinez D, Lo Gullo R, Horvat JV, Helbich TH, Baltzer PAT, Pinker K. Limited value of multiparametric MRI with dynamic contrast-enhanced and diffusion-weighted imaging in non-mass enhancing breast tumors. Eur J Radiol 2022; Epub ahead of print. [Crossref]
- Shehata M, Grimm L, Ballantyne N, Lourenco A, Demello LR, Kilgore MR, Rahbar H. Ductal Carcinoma in Situ: Current Concepts in Biology, Imaging, and Treatment. J Breast Imaging 2019;1:166-76. [Crossref] [PubMed]
- Greenwood HI, Wilmes LJ, Kelil T, Joe BN. Role of Breast MRI in the Evaluation and Detection of DCIS: Opportunities and Challenges. J Magn Reson Imaging 2020;52:697-709. [Crossref] [PubMed]
- Machida Y, Shimauchi A, Tozaki M, Kuroki Y, Yoshida T, Fukuma E. Descriptors of Malignant Non-mass Enhancement of Breast MRI: Their Correlation to the Presence of Invasion. Acad Radiol 2016;23:687-95. [Crossref] [PubMed]
- Clauser P, Krug B, Bickel H, Dietzel M, Pinker K, Neuhaus VF, Marino MA, Moschetta M, Troiano N, Helbich TH, Baltzer PAT. Diffusion-weighted Imaging Allows for Downgrading MR BI-RADS 4 Lesions in Contrast-enhanced MRI of the Breast to Avoid Unnecessary Biopsy. Clin Cancer Res 2021;27:1941-8. [Crossref] [PubMed]
- Li Y, Yang ZL, Lv WZ, Qin YJ, Tang CL, Yan X, Guo YH, Xia LM, Ai T. Non-Mass Enhancements on DCE-MRI: Development and Validation of a Radiomics-Based Signature for Breast Cancer Diagnoses. Front Oncol 2021;11:738330. [Crossref] [PubMed]
- Mori N, Ota H, Mugikura S, Takasawa C, Tominaga J, Ishida T, Watanabe M, Takase K, Takahashi S. Detection of invasive components in cases of breast ductal carcinoma in situ on biopsy by using apparent diffusion coefficient MR parameters. Eur Radiol 2013;23:2705-12. [Crossref] [PubMed]
- Iima M, Honda M, Sigmund EE, Ohno Kishimoto A, Kataoka M, Togashi K. Diffusion MRI of the breast: Current status and future directions. J Magn Reson Imaging 2020;52:70-90. [Crossref] [PubMed]
- Cheang MC, Chia SK, Voduc D, Gao D, Leung S, Snider J, Watson M, Davies S, Bernard PS, Parker JS, Perou CM, Ellis MJ, Nielsen TO. Ki67 index, HER2 status, and prognosis of patients with luminal B breast cancer. J Natl Cancer Inst 2009;101:736-50. [Crossref] [PubMed]
- Ngah S, Bakar RA. Sigmoid function implementation using the unequal segmentation of differential lookup table and second order nonlinear function. Journal of Telecommunication Electronic and Computer Engineering 2017;9:103-8.
- Partridge SC, Nissan N, Rahbar H, Kitsch AE, Sigmund EE. Diffusion-weighted breast MRI: Clinical applications and emerging techniques. J Magn Reson Imaging 2017;45:337-55. [Crossref] [PubMed]
- Scaranelo AM. What's Hot in Breast MRI. Can Assoc Radiol J 2022;73:125-40. [Crossref] [PubMed]
- Donners R, Blackledge M, Tunariu N, Messiou C, Merkle EM, Koh DM. Quantitative Whole-Body Diffusion-Weighted MR Imaging. Magn Reson Imaging Clin N Am 2018;26:479-94. [Crossref] [PubMed]
- Avendano D, Marino MA, Leithner D, Thakur S, Bernard-Davila B, Martinez DF, Helbich TH, Morris EA, Jochelson MS, Baltzer PAT, Clauser P, Kapetas P, Pinker K. Limited role of DWI with apparent diffusion coefficient mapping in breast lesions presenting as non-mass enhancement on dynamic contrast-enhanced MRI. Breast Cancer Res 2019;21:136. [Crossref] [PubMed]
- Bitencourt AG, Graziano L, Osório CA, Guatelli CS, Souza JA, Mendonça MH, Marques EF. MRI Features of Mucinous Cancer of the Breast: Correlation With Pathologic Findings and Other Imaging Methods. AJR Am J Roentgenol 2016;206:238-46. [Crossref] [PubMed]
- de Azambuja E, Cardoso F, de Castro G Jr, Colozza M, Mano MS, Durbecq V, Sotiriou C, Larsimont D, Piccart-Gebhart MJ, Paesmans M. Ki-67 as prognostic marker in early breast cancer: a meta-analysis of published studies involving 12,155 patients. Br J Cancer 2007;96:1504-13. [Crossref] [PubMed]
- Kim JY, Kim JJ, Hwangbo L, Lee JW, Lee NK, Nam KJ, Choo KS, Kang T, Park H, Son Y, Grimm R. Diffusion-weighted MRI of estrogen receptor-positive, HER2-negative, node-negative breast cancer: association between intratumoral heterogeneity and recurrence risk. Eur Radiol 2020;30:66-76. [Crossref] [PubMed]
- Wang S, Wang Z, Li R, You C, Mao N, Jiang T, Wang Z, Xie H, Gu Y. Association between quantitative and qualitative image features of contrast-enhanced mammography and molecular subtypes of breast cancer. Quant Imaging Med Surg 2022;12:1270-80. [Crossref] [PubMed]
- Weaver O, Yang W. Imaging of Breast Cancers With Predilection for Nonmass Pattern of Growth: Invasive Lobular Carcinoma and DCIS-Does Imaging Capture It All? AJR Am J Roentgenol 2020;215:1504-11. [Crossref] [PubMed]
- Meurs CJC, van Rosmalen J, Menke-Pluijmers MBE, Siesling S, Westenend PJ. Predicting Lymph Node Metastases in Patients with Biopsy-Proven Ductal Carcinoma In Situ of the Breast: Development and Validation of the DCIS-met Model. Ann Surg Oncol 2023;30:2142-51. [Crossref] [PubMed]
- Grimm LJ, Rahbar H, Abdelmalak M, Hall AH, Ryser MD. Ductal Carcinoma in Situ: State-of-the-Art Review. Radiology 2022;302:246-55. [Crossref] [PubMed]
- Casasent AK, Almekinders MM, Mulder C, Bhattacharjee P, Collyar D, Thompson AM, Jonkers J, Lips EH, van Rheenen J, Hwang ES, Nik-Zainal S, Navin NE, Wesseling J. Learning to distinguish progressive and non-progressive ductal carcinoma in situ. Nat Rev Cancer 2022;22:663-78. [Crossref] [PubMed]
- Lee SA, Lee Y, Ryu HS, Jang MJ, Moon WK, Moon HG, Lee SH. Diffusion-weighted Breast MRI in Prediction of Upstaging in Women with Biopsy-proven Ductal Carcinoma in Situ. Radiology 2022;305:307-16. [Crossref] [PubMed]